Sports Performance Analysis PDF
Document Details
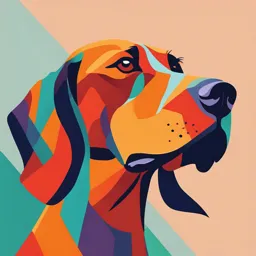
Uploaded by SoftCanyon8621
Tags
Summary
This document contains a statistical analysis of sports performance data. The analysis focuses on the relationship between hours of training and seasonal scores, including the calculation of correlation coefficients and OLS regressions. Categorical variables and other possible factors that may influence performance are also discussed.
Full Transcript
1. Get acquainted with the dataset and produce some summary statistics. Do you see anything that surprises you? 2. Make a scatterplot of the season score and hours trained and calculate the correlation between them. i From the scatterplot, it appears there is no clear or strong correlation bet...
1. Get acquainted with the dataset and produce some summary statistics. Do you see anything that surprises you? 2. Make a scatterplot of the season score and hours trained and calculate the correlation between them. i From the scatterplot, it appears there is no clear or strong correlation between “hours_trained” and “season_score.” The data points are widely scattered across the plot, without a discernible upward or downward trend. This suggests that the two variables are likely not strongly linearly related, and the relationship may be weak or non-existent. The correlation is -0.1121. This indicate a very weak negative linear relationship, where it hours trained increase, seasonal score decrease. 3. Estimate an OLS regression where test score is the dependent variable, and independent variable is hours studied: = 0 + 1ℎ + And interpret the coefficients. The coefficients can be interpreted as follows: For every additional hour of training, the seasonal score decrease by approximately 0,357 points. The constant is 71.89 which means that for a player that trains zero hours the score is 71.89 points T (this is a extrapolation). 4. State the null hypothesis that you want to test to answer our research question. Test the hypothesis using 1%, 5% and 10% significance levels, and interpret the results. 10 0 4A β β to The coefficient is significant at 5% (p < 0.05) and 10% (p < 0.01) but insignificant at 1%. For every additional hour of training, the seasonal score decrease by approximately 0,357 points. 5. You hear that some spotters counting the hours trained fell asleep during our data collection.You decide that you rather want to categorize students into three categories than rely on the precise estimates. Use: 1. Heavy training 2. Normal training and 3. Little training. Create this categorical variable (at levels you deem reasonable) and run the regression above but with the categories as the independent variables. Carefully interpret your new results. In this regression, we categorised the variable “hours trained” into three approximately equal groups based on the number of hours trained: 28-34 hours - little training 34-40 hours - normal training 41-46 hours - heavy training Since little training is the reference category, the constant represents the predicted value of the season score for individuals in this category. Therefore, the average season score for someone in the little training group is 60.13 points. The coefficient for normal training is -1.96, indicating that the average season score for someone in the normal training category is 1.96 points lower than for those in the reference group. Thus, the predicted score for someone in the normal training category is 60.13 - 1.96 = 58.17 points. Similarly, the coefficient for heavy training is -2.89, which means that the average season score for someone in the heavy training category is 2.89 points lower than for those in the reference group. Hence, the predicted score for someone in the heavy training category is 60.13 - 2.89 = 57.24 points. 6. You are very happy about your results and plan to present the findings. Your colleague raises the concern that what you are measuring is not a causal estimate. List some reasons why your friend might be right in this case and name the source of the endogeneity with the appropriate name. The endogenity problem: when Cov (Xi, ui) ≠ 0, OLS estimates correlations but not causality. The independent variable x is not entirely "independent" because its correlated with (things) in the error term. Estimated beta is the causal effect of Xi on yi if the following assumption holds: 1. Conditional mean zero or exogeneity: E(ui | Xi) = 0 → E(Xi, ui) = 0. The mean of ui should always be zero whatever the value of Xi is. Notice that ui is the error term, which is not observable (not the residual from a regression) 2. Random sample: i.i.d draws Xi, yi 3. No outliers If X is endogenous (correlated with u) the OLS estimator is inconsistent. Two major sources of endogeneity Omitted variable Reverse causality Possible omitted variables that cause the counterintuitive result in our case could be: Excessive trying that lead to physical and mental fatigue (indicator variables: recovery time, sleep quality) Training quality might be more critical than quantity (indicator variables: coach rating) Players with lower initial skill might train more to catch up (indicator variables: baseline skill level1 player rating before training) Nutrition (indicator variables: diet, hydration) External stressors (indicator variables: family and personal life disruptors) Match specific factors (indicator variables: weather, opponent strength) Poor coaching, lack of motivation (indicator variables: player feedback, team morale) The direction of the bias depends on the sign of γ / π We can calculate the bias using formula: 3 OLS 3 cov Xi Ui varixil Reverse causality: Instead of hours trained causing scores it might run the other way. This would means that poor performance lead to more hours. This means that hours trained depends on seasonal score: Hours trained t score the Bo β Reverse causality will cause bias of: Cov(Hours trained, ui) / Var(Hours trained) = γ1 Var(ui) / (1-γ1β1) Var(Hours trained) The sign of the bias depends on: (1-γ1β1) / γ1 This can be explained by for example that players who perform poorly may feel the need to compensated by training more, coaches might assign additional training sessions. You have to practice this c 7. You realize that the data does not only keep records of the time spent in training, but also an estimate of the players’ physical state over the season. Here a “0” could indicate both an injury and lacking in stamina. Integrate these into your estimate from question 3) and interpret carefully. Make sure to write out any equation you are estimating. For every additional hour of training, the seasonal score decrease by approximately 0,1526 points. 8. Are potential issues of endogeneity now solved? Why / why not? If we compare with the previous regression we can see that the coefficient is less negative now when we have included physical training (-0,1526 compared to -0,357) this suggest that we overestimated the negative effect from additional training hours and that physical training was a potential omitted variable. It seems like we got rid of parts of the endogeneity issue but there can still be issues with endogeneity do to other factors that we mentioned above. How can we be sure that the endogeneity issue is solved, should we calculate if β1 is consistent, can we assume this because of sample size or randomized test and are we biased if we continue to include possible omitted variables to get a result that is less counterintuitive? mm 9. To your surprise, payers were randomized into being subject to a new training method that is supposed to boost player performance (new_method). Estimate the ATE with the regress command and without. From this analysis, would you advise the Gothenburg team to use this new method? ATE = average treatment effect How much on average any individual would be affected by receiving treatment / the causal effect of a treatment. Treatment is a binary variable Xi (1 if treated, 0 otherwise) ATE = E [Y(1)-Y(0)] Where: Y(1): outcome if the individual receives treatment Y(0): outcome if the individual does not receive treatment The counterfactual problem: Challenge: for any individual, we observe only one of the two potential outcomes either Y(1) or Y(0). The unobserved outcome is the counterfactual. As a result cannot directly compute Y(1)-Y(0) for any individual, so we rely on statistical methods and assumptions to estimate the ATE by comparing groups of treated and untreated individuals Randomization ensures that treated and underrated groups are comparable (on average) Do to randomization we can calculate the difference in means between groups treated and those not treated. When compare average score for newmethod and (not new) method we see a very small difference. Therefore we cannot recommend the newmethod. Average treatment effect on the treated (ATET) The average treatment effect on the treated describes how much on average the individuals who actually received the treatment are affected by the treatment. ATET: E [Y1i - Y0i| Xi =1] = E [Y1i | Xi =1] - E [Y0i| Xi =1] Estimating ATET still involves one unobserved counterfactual: E [Yi (0)| Xi =1] , i.e. the potential outcome if untreated for those who actually revived treatment. Problems with self selection: Positive selection, E(Yi(0)|Xi=1) - E(Yi(0)|Xi=0) > 0 Negative selection, E(Yi(0)|Xi=1) - E(Yi(0)|Xi=0) < 0 Solutions to the counterfactual problem: Randomized experiments (randomized control trails) Observational data (units are as if randomly assigned given some additional assumptions: IV, panel data and diff-in-diff, regression discontinuity) t These methods exploit what can sometimes be seen as natural or quasi-experimental settings Internal validity: does the experiment provide an estimate of the causal effect in the population under study External validity: the extent to which the estimated causal effect can be generalized to other populations, economic settings and related treatments Threats to internal validity Failure to randomize Partial compliance (do not comply, substitution) Attrition (drop out that is not random) Experimental Hawthorne effects (treaded and controls behave differently because they know they are treated) Spillover Threats to external validity Non-representative sample Non-representative policy General equilibrium effects 10. First estimate the naïve OLS model: = 0+ 1 + ’ + Where ’ is a vector of the control variables we defined above. Interpret 1. For each additional colonial medical visit, the vaccination index decrease by 0.068 units holding all other factors constant. 11. Explain why the OLS model is likely to not show the causal effect of colonial wrongdoings on modern day medical interventions. Because of issues with endogencity, omitted variable or reverse causality Possible OV bias: pre-colonial conditions, cultural practices Reverse causality (vaccination index influence times visited): colonial administrators may have directed more medical campaigns increasing times visited) to areas with initially poor vaccination outcomes or higher disease burden. This would create a scenario where vaccination rates determined the campaign intensity The authors propose an IV strategy using suitability for cassava (a new world staple food also known as manioc) as their instrument. Specifically, they use the log soil suitability for cassava relative to the log soil suitability for millet (relative_suitability in the dataset). Due to the way cassava is farmed (processing is done near water and less land must be cleared) there is more interaction with the Tsetse fly – the transmitter of sleeping sickness. The IV method provide a solution to identification when randomized experiments are not feasible We need to think of the variation in Xi as having two parts: 1. One endogenous part that is correlated with ui 2. One exogenous part that is uncorrected with ui It we can isolate the variation that captures only the exogenous part we can use this to get an unbiased estimate of ß1. The instrument variable Z can give us an consistent estimate of ß1 if we have a variable that affect y that we cannot observe. The instrument variable Z must be: Relevant: correlate with X cov(zi, X) ≠ 0 error term Exogenous: uncorrelated with any other determinants of y Cov(Zi, unobserved variable) = 0 and Cov(Zi, vi) = 0 Independence: Z is uncorrected with other omitted variables and the error term Exclusion restriction: Z only affects y through its effect on X In our case the IV is relevant (must strongly correlate with times visited) because it captures soil conditions that made cassava farming more likely, which, due to cassava's link to tsetse fly habitats, influence the intensity of medical campaigns. Relevance can be tested through first - stage repression. For the exogeneity condition the instrument test affect the dependent variable only through its effect on X. It is plausible that soil suitability directly impacts times visited via tsetse fly exposure. Is the exogeneity condition violated since fly density could be an omitted variable that directly impacts vaccination rates 12. Draw a directed acyclic graph (fancy name for the graph with arrows on lecture slide 6) of this set-up and discuss briefly. You can draw this for instance using PowerPoint or Excel and just take a screenshot. Soil Suitability for Cassava Control Variables Times Visited Vaccination Index 13. What are the crucial assumptions for IVs in general and what do each mean in this specific case? See answer question 11 14. Specify the first stage equation and reduced form and estimate both using. The first stage: estimating the impact of our instrument on the endogenous variable and obtaining the fitted values x̂ (regress X on Z) i do ta Zi t ni The second stage: using the fitted values from the first stage Instrument relevance β it ei yi po Reduced form: regress Y on Z i no to zit ei Estimated ß = reduced form / first stage Different notation from teachers slides: Two stage least square 4 by cx ay ou 3 2 SLS 2 a c z j u e z O R F B FS 24 cy a x I it R F F S 2 c z c Z The simplest IV estimator uses a single binary instrument Z and one endogenous variable X, this is called the Wald estimator. 2ˢᵗˢ I 3 wald RF Fs g Y z 1 Y z 0 T 2 1 x̅ 1 2 01 15. Use a 2SLS set-up to run the instrumental variable regression. You can use the Stata command ivreg2 or ivregress. 16. Carefully interpret the IV coefficients. We observe that the coefficient for times visited is -0.3345. This indicates that for each additional medical visit, the vaccination index decreases by approximately 0.3345 units. In the previous regression, which did not use an instrumental variable, the vaccination index decreased by only 0.068 units per additional visit. The larger negative effect observed with the instrumental variable suggests that the earlier model underestimated the true negative effect of visits. The bias in the initial model was positive, as the unadjusted coefficient (-0.068) was closer to zero than the instrumented estimate (-0.3345). This likely occurred because unaccounted factors, such as reverse causality (e.g., more visits targeting regions with initially lower vaccination rates), or omitted variable bias (e.g.,). 17. You come up with another potential IV idea by using accessibility during colonial times (captured in the variable hist_road_access) discuss the assumptions for this idea and calculate the first stage. Relevance: The instrument Z (historical road access) must predict X (times visited). This condition appears plausible, as better road access likely facilitates more frequent visits to a location. Exogeneity: The chosen instrument for IV must not be correlated with the error term in the regression. This means that road access should only affect vaccination rates through visits, and not through other channels. If, for instance, better road access is also associated with higher-quality health services, this assumption could be violated. v Exclusion Restriction: The instrument Z (road access) must not have a direct effect on Y (vaccination index) but should influence it only through X (times visited). This seems reasonable, as improved road access would primarily affect the number of visits, which in turn impacts vaccination rates. 18. Use hist_road_access, disregarding any issues about the assumptions, and identify whether it this a “weak instrument”? Is/would this be an issue? Weak instrument means that the relevance condition fails. IV can cause substantial bias toward OLS when first stage F-stat is small. Rule ot thumb is that an instrument is strong if first-stage F-stat is > 10. A weak instrument can lead to biased IV estimates, unreliable standard errors, and an increased likelihood of incorrectly rejecting or failing to reject the null hypothesis. In our case, we assess the strength of the instrument using Wald’s weak identification test, which returns an F-statistic of 8.375. Since this is below 10, it suggests that the instrument is weak. As a result, the IV estimates may be unreliable, and their interpretation should be approached with caution. Run this regression so yo can see for yourself