Inferential Statistics - PDF
Document Details
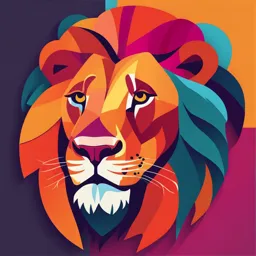
Uploaded by AvailableRoentgenium6942
Tags
Summary
This document covers inferential statistics, motivation, expectation algebra, sampling, hypothesis testing, and more. It discusses concepts like bias, variance, and sampling distributions. The document focuses on the theory and calculations related to statistical estimations.
Full Transcript
previous page next page Inferential statistics: Motivation inferential statistics: concern about statistics evaluated from sample and their quantitative relation to usually not known ‘true’ population parameters (e.g., mome...
previous page next page Inferential statistics: Motivation inferential statistics: concern about statistics evaluated from sample and their quantitative relation to usually not known ‘true’ population parameters (e.g., moments, quartiles) how to formulate statistical estimates; what are characteristics of good estimates? – bias (how close is estimate to population parameter?) and variance (how much scatter about population parameter is there in estimate?); ideal of zero bias (unbiased) and low variance – bias-variance tradeoff – unbiased estimator may have large variance, so an estimator with low bias and low variance may be preferred a sample statistic is a function of random variables and so must also be a random variable; what is the distribution of a sample statistic? not necessarily the same distribution as that from which the sample is drawn – sampling distribution (assumed theoretical or computationally obtained) used to relate sample statistic to population parameter BACK FORWARD 1 previous page next page Expectation again and expectation algebra recall: the expectation or the expected value of a (continuous) random variable, g(X), written as E(g(X)), assuming that X follows a pdf, f (x), is defined as Z E(X) = g(x) f (x) dx all x and may be interpreted as the ‘mean’ value of g(X) ‘basic’ expectations (for random variables, X and Y ) – mean, µx = E(X) – variance, σx2 = Var(X) = E[(X − µx)2] – covariance between two random variables, X and Y , σxy = Cov(X, Y ) = E[(X − µx)(Y − µy )]; if X and Y are statistically independent, i.e., the occurrence of X does not affect probability of Y occurring, then Cov(X, Y ) = 0; correlation coefficient, σxy /(σxσy ) expectation algebra (random variables: X, Y , Xi) P P – E(aX + bY ) = aE(X) + bE(Y ) =⇒ E( aiXi) = aiE(Xi) 2 2 – Var(aX Pn + bY ) = Pn 2a Var(X) + P Var(Y ) + 2ab Cov(X, Y ) =⇒ b Var( 1 aiXi) = i ai Var(Xi) + i6=j aiaj Cov(Xi, Xj ) BACK FORWARD 2 previous page next page Sampling: Multiple events and statistical independence sampling involves multiple random events (for sample size > 1) as each draw from a distribution is a random event; sample of size, n: n events leading to n random variables taking a value, X = (X1, X2,..., Xn) for two random ‘events’, A and B: the probability that A occurs given that B has occurred, P (A|B) = P (A and B)/P (B); two events are statistically independent if P (A and B) = P (A)P (B) =⇒ P (A|B) = P (A), i.e., B occurring has no influence on A occurring – two random variables, X and Y , are in general defined by a joint pdf, f (x, y), but if X and Y are statistically independent, then not only Cov(X, Y ) = 0, but also f (x, y) can be factored into f (x, y) = fx(x)fy (y) analysis of sample statistics considerably simplified if Xi’s are assumed drawn from the same distribution, i.e., identically distributed (id), and further that they are statistically independent (iid) some types of sampling are not necessarily statistical independent, e.g., time or spatial series, where sampling is done in ‘closely’ spaced time or spatial instants BACK FORWARD 3 previous page next page The estimation problem: how to develop good statistics assume a population with known parameters, e.g., normal distribution with known mean, µx, and standard deviation, σx, (or variance, Var(x) = σx2 , is it obvious that an effective means of estimating P µx and Var(x) is the usual formulae, e.g., for sample mean, X = i Xi/n, where n is the sample size? how are ‘good’ sample estimators (formulae) for population parameters developed, and what is a ‘good’ estimator anyway? criteria for the ‘goodness’ of an estimator, θ̂ in terms of population parameter, θ – estimation error denoted as dn(θ̂) = θ̂n − θ, for sample size, n – small mean squared (expected) error, E(d2n) = E[(θ − θ̂n)2]: low bias, B(θ̂n) = E(dn) = E(θ̂n) − θ, and low variance, Var(θ̂n) = E[{θ̂n − E(θ̂)}2]; squared error as squared Euclidean distance – consistency: as sample size (n) increases, estimator performance improves – robustness: reliable even in the presence of outliers BACK FORWARD 4 previous page next page (Sampling) distribution for the sample mean how to relate point estimate, X, to µx? as X is a random variable, relationship is probabilistic, i.e., must be formulated in probability terms and an interval estimate – need to determine (sampling) distribution of X X can be shown to be unbiased, i.e., E(X) = µx, and to have variance, σx2 /n, so its distribution has the same mean as the population from which sample is drawn, but the variance is much smaller (the distribution is much narrower) for large n √ – the standard deviation of X, i.e., σX = σx/ n is termed the standard error (s.e.); √ because σx is generally not known, this is usually estimated as sx/ n where sx is the sample standard deviation if the distribution from which sample is drawn is normal, then X is also normal if the distribution from which sample is drawn is not normal, then X is asymptotically normal for large samples (i.e., large n) due to the central limit theorem BACK FORWARD 5 previous page next page Confidence interval for sample mean probabilistic relationship between X and µx, due to distribution of X being either exactly or asymptotically normal: X − µx P √ < zp = p σx / n – interval estimate: the (1 − α)100% two-sided confidence interval for µx, where α is termed the significance level, is defined by X − µx P zα/2 < √ < z1−(α/2) = 1 − α σx / n ∗ alternatively, µx lies within the interval, [X + zα/2(s.e.), X − zα/2(s.e.)] with probability (1-α)100% (note zα/2 < 0, but because of symmetry, |zα/2| = z1−(α/2)) ∗ practical difficulty: σx is not known, instead sample standard deviation, sx, used for (s.e.) with the t-distribution with number of degrees of freedom, ν = n − 1 instead of the normal distribution, so in practice use interval, [X + tν,α/2(s.e.), X − tν,α/2(s.e.)] ∗ for n > 30, the t-distribution practically the same as the normal distribution ∗ in some applications, a one-sided interval might be appropriate BACK FORWARD 6 previous page next page Topics related to confidence intervals for means applications to mean-like statistics – issues of specifying standard error and number of degrees of freedom – two-sample problems: difference between means – single-sample and two-sample proportions – paired observations (two dependent samples) confidence interval vs prediction (or tolerance) limits – bounds on a single observation: use same results with (s.e.) = s, or n=1 implication for experiments and sample size for a given uncertainty, e0 √ – because uncertainty ∝ 1/ n, if a specified uncertainty, e0, is desired, required n ∝ 1/e20 hydrology practice: Yp = Y + KpSy , where Yp is a (flood) magnitude of an exceedance probability, p, and Kp is termed the frequency factor, depends on the model distribution, and traditionally tabulated BACK FORWARD 7 previous page next page Distribution and confidence interval for single variance the standard method for evaluating sample variance, Sx2 = P (Xi − X)2/(n − 1) for sample size, n, can be shown to be unbiased if sample is drawn from a normal distribution, with population variance, σx2 , then the statistic, X 2 = (n − 1)Sx2/σx2 , can be shown to follow the skewed chi-squared (χ2) distribution, defined by the number of degrees of freedom, k = n − 1, so that 2 2 (n − 1)Sx 2 P X = < χ p,k =p σx2 the (1 − α)100% two-sided confidence interval then defined by 2 2 (n − 1)Sx 2 P χα/2,k < < χ1−α/2,k =1−α σx2 alternatively, can express this as σx2 being within the interval, " # " # (n − 1)s2x (n − 1)s2x (n − 1) (n − 1) 2 2 , = , s x = K χ2 sx χ21−α/2,k χ2α/2,k χ21−α/2,k χ2α/2,k the confidence interval for the standard deviation, σx, is commonly obtained by taking the square root, but this is a biased estimate BACK FORWARD 8 previous page next page Distribution and confidence interval for two variances two sample variances, S12 and S22, of sample size, n1 and n2, can be compared using their ratio, S12/S22, and the relationship to the corresponding ratio of normal population variances, σ12/σ22 can be examined in terms of the statistic, F = (S12/S22)/(σ12/σ22) which is known to follow the skewed F -distribution, which is defined by two degrees of freedom, d1 = n1 − 1 and d2 = n2 − 1, for which 2 2 S1 /S2 2 P F = 2 2 < fp,d 1 ,d2 =p σ1 /σ2 the (1 − α)100% two-sided confidence interval then be defined by 2 2 S /S P fα/2,d1,d2 < 12 22 < f1−α/2,d1,d2 = 1 − α σ1 /σ2 alternatively, can express this as σ12/σ22 being within the interval, 2 2 1 1 S1 S1 , = K F f1−α/2,d1,d2 fα/2,d1,d2 S22 S22 whether two population variances are equal may be checked by determining whether the confidence interval brackets the unit value BACK FORWARD 9 previous page next page Hypothesis testing and the p-value statistical hypothesis testing: formal procedure involving – depending on what is desired, defining or choosing a test statistic, √ e.g., in analyzing mean-like quantities, choose T = (X − µx)/(sx/ n), – a null hypothesis, H0, in which a value of the population parameter is assumed or hypothesized, e.g., µx = k0, and – an alternative hypothesis, H1, e.g., µx 6= k0, or µx > k0, or µx < k0 – dictates the detailed form of the probability to be evaluated for testing the hypothesis ∗ if H1: √ µx 6= k0 =⇒ two-tailed test (P (T > |t0 = (x − µx)/(sx/ n)|) = p) ∗ if H1: µx > k0 or H1: µx < k0 =⇒ one-tailed test (P (T > t0) = p or P (T < t0) = p p-value: the probability of obtaining a value for test statistic (at least as extreme as that in the sample) assuming H0, with the evaluated probability consistent with H1 (one-tailed or two-tailed test) – the p-value for a two-tailed test taken to be twice that for a one-tailed test if this is meaningful (p ≤ 1) BACK FORWARD 10 previous page next page Interpretation of the p-value and acccepting/rejecting hypothesis the smaller the p-value, the less empirical support for H0; if the p-value is sufficiently small (perhaps smaller than a pre-selected significance level, α, with α traditionally chosen as 0.1, or 0.05, or even 0.01), then H0 is ‘rejected’, i.e., as being not supported by the sample – ‘accepting’ or non-rejecting H0 does not necessarily mean that H0 is true, only that there is insufficient experimental evidence for rejecting it; rejecting H0 due to small p does indicate that the experimental evidence does not support H0 – type I error (rejecting H0 when it is true, false positive), and type II error (accepting H0 when it is false, false negative) – Probability(type I error) = α, Probability (type II error) = β, statistical power = 1 − β – generally want small α and β and high power; can reduce α and β by increasing sample size, n; for fixed n, decreasing α increases β relationship to confidence intervals BACK FORWARD 11 previous page next page Goodness of Fit Tests for normal and other distributions Shapiro-Wilk test for normality (or lognormality): Pn [ i=1 aix(i)]2 test statistic: W = Pn 2 i=1 (xi − x) for sample, xi, where x(i) is the (ordered) ith smallest number in the sample, and ai coefficients related to theoretical normal distribution – null hypothesis: sample from a normal (or lognormal) distribution Anderson-Darling test between theoretical (or model, not only normal) cdf, F , e.g., a normal, and observed cdf, Fn: Z ∞ 2 [Fn(x) − F (x)]2 based on distance (metric): A = n dF (x) −∞ F (x)[1 − F (x)] – null hypothesis: Fn is same as F – issue of whether distribution parameters are known beforehand, or estimated from data effectiveness may depend on sample size and parameter ranges quantile-quantile plots still recommended BACK FORWARD 12 previous page next page Transformed variables and uncertainty propagation given statistics on a random variable, X (and later, an additional random variable, Y ), can we estimate statistics of a non-linearly transformed variable, g(X) (and later, g(X, Y )? – basic issue in propagation of uncertainty (or error): going from uncertainty in basic variables, X and Y (and perhaps others), to the uncertainty in final derived (usually nonlinear) variable, g consider a Taylor expansion about the (population) mean, e.g., for the univariate case, µx: g(X) = g(µx) + g 0(µx)(X − µx) + [g 00(µx)/2](X − µx)2 +... 2 How can the desired mean (µg(x), and variance (σg(x) ) of g(X) be related to the known mean (µx) and variance (σx2 ) of X? apply expectation algebra as appropriate to both sides to find desired statistic BACK FORWARD 13 previous page next page Statistics of transformed variable first-order estimates: include only linear term, and neglect quadratic (and higher) terms µg(x) ≈ g(µx), 2 σg(x) = [g 0(µx)]2σx2 first-order estimates can be substantially biased, depending on the strength of non-linearity (in range of interest), and the relative uncertainty, measured e.g., by a coefficient of variation, σx/µx second-order estimate for the mean (include up to quadratic term): µg(x) ≈ g(µx) + [g 00(µx)/2]σx2 second-order results available for variance, but rather more complicated and so relatively rarely used to obtain confidence intervals for µg(x), need distribution √ of g(X) which is given by the (first-order) Delta method: √ If n(X − µx) approaches a normal distribution (N (0, σx2 )), then n[g(X) − g(µx)] approaches a normal distribution (N (0, [g 0(µx)σx]2)) – apply previous confidence-interval√procedure for µx √ to µg(x) changing only the standard error from σx/ n to |g 0(µx)|σx/ n BACK FORWARD 14 previous page next page The multivariate case of transformed variable first-order estimates of the bivariate (two-variable) case: µg(x,y) ≈ g(µx, µy ), 2 σg(x,y) = [gx0 ]2σx2 + [gy0 ]2σy2 + 2gx0 gy0 σxy where gx0 = ∂g/∂x and gy0 = ∂g/∂y, evaluated at the sample-mean values, and σxy is the covariance of X and Y , defining the correlation between the two variables to obtain confidence intervals for µg(x,y), appealing to the (first-order) Delta method for multivariate case, apply previous confidence-interval procedure for µg(x,y) using as the standard error q {[gx0 ]2σx2 + [gy0 ]2σy2 + 2gx0 gy0 σxy }/n in the more general case, where there are k variables, similar first-order estimates would give µg(x) ≈ g(µx) and X k X 0 2 2 2 σg(x) = [gxi ] σxi + 2 gx0 gy0 σxy i=1 i>j and confidence intervals can be similarly constructed using a suitably defined standard error BACK FORWARD 15