Lecture 4 Modeling Volatility PDF
Document Details
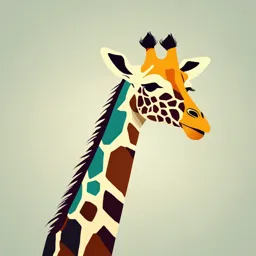
Uploaded by ReceptiveSugilite4494
Tags
Summary
This lecture covers modeling volatility in economic time series, examining stylized facts, and formalizing models of heteroskedasticity. The lecture discusses how asset holders are interested in the volatility of returns, not historical periods, and why the understanding of volatility is important for risk management. Also, the lecture explores the Black-Scholes option pricing model and the VIX volatility index.
Full Transcript
Modeling Volatility Lecture 4 Lecture Objectives ❖Examine the stylized facts concerning the properties of economic time- series data. Casual inspection of GDP, interest rates, exchange rates suggests they don’t have a constant mean and variance. A stochastic variab...
Modeling Volatility Lecture 4 Lecture Objectives ❖Examine the stylized facts concerning the properties of economic time- series data. Casual inspection of GDP, interest rates, exchange rates suggests they don’t have a constant mean and variance. A stochastic variable with a constant variance is called homoscedastic (as opposed to heteroskedastic). For series exhibiting volatility, the unconditional variance may be constant even though the variance during some periods is unusually large. Lecture Objectives ❖Formalize simple models of variables exhibiting heteroskedasticity. Asset holders are interested in the volatility of returns over the holding period, not over some historical period. This forward-looking view of risk means that it is important to be able to estimate and forecast the risk associated with holding a particular asset. Why do we care ❖Many derivative prices depend on volatility. E.g. A European call option A contract giving its holder the right but not the obligation to buy a fixed number of shares of a specified common stock at a fixed price on a given date. The fixed price is called the strike price and is denoted by K The given date is called the expiration date. The important time span here is the time to expiration and is denoted l. Why do we care Black-Scholes call option price: Where: 𝑐𝑡 =𝑃𝑡 Φ(x)-K𝑟 −𝑙 Φ(x-σ𝑡 𝑙) 𝑃𝑡 is the current stock price. ln( 𝑃𝑡 ) r is the risk-free interest rate x= 𝐾𝑟−𝑙 1 + σ𝑡 𝑙 σ𝑡 𝑙 2 𝑙 is time to maturity σ𝑡 is the conditional standard deviation of the log return of the specified stock. Φ(x) is the cumulative distribution function of the standard normal random variable evaluated at x. Why do we care ❖Risk Management Examine the relationship between long and short-term interest rates. Volatility modeling provides a simple approach to calculating value at risk of a financial position. The volatility index of a market became a financial instrument. The VIX volatility index compiled by the Chicago Board of Option Exchange (CBOE). Stylized Facts ❖Most of the series contain a clear GDP 8 trend. 7 GDP and consumption exhibit an 6 upward trend. 5 4 Government expenditure has an 3 upward trend but more volatile 2 1 than GDP. 0 5 10 15 20 25 30 35 0 1961 1962 1963 1964 1965 1966 1967 1968 1969 1970 1971 1972 1973 1974 1975 1976 1977 1978 1979 1980 1981 1982 1983 1984 1985 1986 1987 1988 1989 1990 1991 1992 1993 1994 Gvt expenditure 1995 1996 1997 1998 1999 2000 2001 Stylized Facts 2002 2003 2004 2005 2006 2007 2008 2009 2010 2011 2012 2013 2014 2015 2016 2017 2018 2019 2020 2021 2022 2023 Stylized Facts ❖Shocks to a series can display a 20 18 high degree of persistence. 16 14 Interest rates exhibit no clear 12 upward or downward trend. 10 8 The variables show a high degree 6 4 of persistence. 2 0 short-term interest rate long-term interest rate Stylized Facts ❖The volatility of many series is not constant over time. Daily changes in the NYSE index shows ▪ The periods where the stock market seems tranquil. ▪ Alongside periods with large increase and decreases in the market. Stylized Facts ❖Such series are called conditionally heteroskedastic if the unconditional (or long-run) variance is constant, but there are periods in which the variance is relatively high. Stylized Facts ❖Although volatility is not directly observable, it has some characteristics that are commonly seen in asset returns There exist volatility clusters Volatility evolves over time in a continuous manner. Volatility does not diverge to infinity Volatility seems to react differently to a big price increase or a big price drop. Stylized Facts ❖Some series seem to meander. Real effective exchange rate 180 The REER show no particular 160 tendency to increase or decrease. 140 Seem to go through sustained periods 120 100 of appreciation and depreciation with 80 no tendency to revert to a long-run 60 mean. 40 This type of random walk behaviour is 20 0 typical of nonstationary series. 1972 1974 1970 1976 1978 1980 1982 1984 1986 1988 1990 1992 1994 1996 1998 2000 2002 2004 2006 2008 2010 2012 2014 2016 2018 2020 2022 Stylized Facts ❖Eyeballing the data is not a substitute for formally testing for the presence of conditional heteroskedasticity or nonstationary behaviour. ❖Casual inspection does have its perils. Formal testing is necessary to substantiate any first impressions. The strong visual pattern is that these series (graphs in previous slides) are not stationary. ▪ The sample means don’t appear to be constant. ▪ There is the strong appearance of heteroskedasticity (variance not constant). ARCH Processes ❖In conventional econometric models The variance of the disturbance term is assumed to be constant. However, many economic time series data exhibit periods of unusually large volatility, followed by periods of relative tranquility. In such circumstance, the assumption of a constant variance (homoskedasticity) is inappropriate. ARCH Processes ❖It’s easy to imagine instances in which you might want to forecast the conditional variance of a series. As an asset holder, you would be interested in forecasts of the rate of return and its variance over the holding period. The unconditional variance (i.e. the long-run forecast of the variance) would be unimportant if you plan to buy the asset at t and sell at t+1. ARCH Processes ❖One approach to forecasting the variance is to explicitly introduce an independent variable that helps to predict the volatility. ❖Consider the simplest case in which: ❖𝑦𝑡+1 =𝜀𝑡+1 𝑥𝑡 𝑦𝑡+1 is the variable of interest. 𝜀𝑡+1 is a white-noise disturbance term with variance 𝜎 2 𝑥𝑡 is an independent variable that can be observed at period t. ARCH Processes ❖If 𝑥𝑡 =𝑥𝑡−1 = 𝑥𝑡−2 = ⋯ = constant The 𝑦𝑡 sequence is the familiar white-noise process with a constant variance. However, when the realizations of the 𝑥𝑡 sequence are not all equal, the variance of 𝑦𝑡+1 conditional on the observable value of 𝑥𝑡 is Var(𝑦𝑡+1 |𝑥𝑡 )=𝑥𝑡2 𝜎 2 The conditional variance of 𝑦𝑡+1 is dependent on the realized value of 𝑥𝑡 ARCH Processes ❖Since you can observe 𝑥𝑡 at time period t ❖You can form the variance of 𝑦𝑡+1 conditionally on the realized value of 𝑥𝑡 ❖If the magnitude (𝑥𝑡 )2 is large (small) the variance of 𝑦𝑡+1 will be large (small) as well. ❖If the successive values of 𝑥𝑡 exhibit positive serial correlation (a large value of 𝑥𝑡 tends to be followed by a large value of 𝑥𝑡+1 ) the conditional variance of the 𝑦𝑡 sequence will exhibit positive serial correlation as well. ARCH Processes The intro of the 𝑥𝑡 sequence can explain periods of volatility in the 𝑦𝑡 sequence. In practice, might want to estimate the regression equation in logarithmic form as ln 𝑦𝑡 =𝑎0 + 𝑎1 ln 𝑥𝑡−1 +𝑒𝑡 where: 𝑒𝑡 =ln 𝜀𝑡 ARCH Processes ❖Log transformation results in a linear regression equation ❖OLS can be used to estimate 𝑎0 and 𝑎1 directly. ❖A major difficulty with this strategy is that it assumes a specific cause for the changing variance. ❖The methodology also forces 𝑥𝑡 to affect the mean of ln 𝑦𝑡. ❖Oftentimes, one might not have a firm theoretical reason for selecting one candidate for the 𝑥𝑡 sequence over other reasonable choices. ARCH Processes ❖E.g. was it the oil price shocks, a change in the conduct of monetary policy and/or the breakdown of the Bretton Woods system that was responsible for the volatility of real investment during the 1970s? The technique necessitates a transformation of the data such that the resulting series has a constant variance. In the example at hand, the 𝑒𝑡 sequence is assumed to have a constant variance. If this assumption is violated, some other transformation of the data is necessary. ARCH Processes Conditional moments ❖Instead of using ad hoc variable choices for 𝑥𝑡 and/or data transformation. ❖Engle (1982) shows that its possible to simultaneously model the mean and the variance of a series. ❖Suppose you estimate the stationary ARMA model ❖𝑦𝑡 = 𝑎0 + 𝑎1 𝑦𝑡−1 + 𝜀𝑡 and want to forecast 𝑦𝑡+1 ❖The conditional mean of 𝑦𝑡+1 is: 𝐸𝑡 𝑦𝑡+1 = 𝑎0 + 𝑎1 𝑦𝑡 ❖The forecast error variance is: 𝐸𝑡 [(𝑦𝑡+1 − 𝑎0 − 𝑎1 𝑦𝑡 )2 ]=𝐸𝑡 𝜀𝑡+1 2 =𝜎 2 ARCH Processes unconditional moments ❖The unconditional forecast is always the long-run mean of the 𝑦𝑡 sequence: ❖𝐸(𝑦𝑡+𝑛 ) =𝑦𝑡 =1−𝑎 𝑎0 1 ❖The unconditional forecast error variance is ❖Eሼ[𝑦𝑡+1 − 𝑎0 /(1 − 𝑎1 )]^2ሽ=E[(𝜀𝑡+1 + 𝑎1 𝜀𝑡 + 𝑎12 𝜀𝑡−1 + 𝑎13 𝜀𝑡−2 + ⋯)2] 𝜎2 = 1−𝑎21 ARCH Processes unconditional moments ❖Since 1/(1-𝑎12 )>1, the unconditional forecast has a greater variance than the conditional forecast. Thus, conditional forecasts are preferable (since they take into account the known current and past realizations of series). ARCH Processes ❖If the variance of 𝜀𝑡 is not constant One can estimate any tendency for sustained movements in the variance using an ARMA model. Let 𝜀ෝ𝑡 denote the estimated residuals from the model 𝑦𝑡 =𝑎0 + 𝑎1 𝑦𝑡−1 + 𝜀𝑡 So that the conditional variance of 𝑦𝑡+1 is: ARCH Processes ❖Var(𝑦𝑡+1 |𝑦𝑡 )= 𝐸𝑡 [(𝑦𝑡+1 − 𝑎0 − 𝑎1 𝑦𝑡 )2 ]=𝐸𝑡 𝜀𝑡+12 ❖Until now, we have set 𝐸𝑡 𝜀𝑡+1 2 =𝜎 2 Suppose that the conditional variance is not constant. One simple strategy is to forecast the conditional variance as an AR(q) process using the squares of the estimated residuals ARCH Processes 𝜀𝑡Ƹ2 =𝛼0 + 𝛼1 𝜀𝑡−1 Ƹ2 +…+𝛼𝑞 𝜀𝑡−𝑞 Ƹ2 +𝛼2 𝜀𝑡−2 Ƹ2 +𝑣𝑡 ….(1) Where 𝑣𝑡 is a white-noise process. If the values of 𝛼1 , 𝛼2 , … , 𝛼𝑛 = 0 implies the estimated variance is simply the constant 𝛼0 Otherwise, the conditional variance of 𝑦𝑡 evolves according to the autoregressive process given by equation (1). One can use equation (1) to forecast the conditional variance at t+1. For this reason, an equation like (1) is called an autoregressive conditional heteroskedastic (ARCH) model. ARCH Processes ❖Autoregressive Conditional Heteroskedastic (ARCH) Model has constant unconditional variance but non-constant conditional variance. ❖We often use Maximum Likelihood (ML) techniques to estimate the values for the coefficients a and α simultaneously. ❖It is more tractable to specify 𝑣𝑡 as a multiplicative disturbance. ARCH Processes ❖Engle (1982) proposed a multiplicative conditionally heteroskedastic model as follows: ❖𝜀𝑡 =𝑣𝑡 𝛼0 + 𝛼1 𝜀𝑡−12 …(2) ❖Where ❖ 𝑣𝑡 is white-noise process such that 𝜎𝑣2 =1 ❖𝑣𝑡 and 𝜀𝑡−1 are independent of each other. ❖𝛼0 and 𝛼1 are constants such that 𝛼0 >0 and 0< 𝛼1