Lecture 4 Data Error Analysis.pdf
Document Details
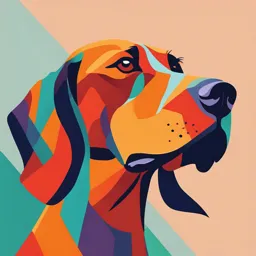
Uploaded by HandsomeIguana
Tags
Full Transcript
Data Error Analysis i-CHEP Selected Topics in Contemporary Issues ASU 114 Dr Ahmed Ragheb [email protected] Prof. Dr. Ahmed Ragheb Lec. 4 ASU 114 Selected Topi...
Data Error Analysis i-CHEP Selected Topics in Contemporary Issues ASU 114 Dr Ahmed Ragheb [email protected] Prof. Dr. Ahmed Ragheb Lec. 4 ASU 114 Selected Topics in Contemporary Issues Data Error Analysis What is the Problem? Any measurement process will include certain part of Errors due to various factors Accordingly, the true value of any variable is impossible but we get what is called: MOST PROBABLE VALUE (MPV) or BEST ESTIMATE To obtain better Precision for the MPV, we can 1- Measure the required variable several times 2- Use more accurate instruments 3- Utilize experienced observers Prof. Dr. Ahmed Ragheb Lec. 4 ASU 114 Selected Topics in Contemporary Issues 1 Data Error Analysis Source of Errors Natural Errors Due atmospheric conditions or surrounding environment e.g. effect of Earth’s curvature, temp., pressure, humidity, refraction, gravity, setting the instrument on a moist ground causing settlement Instrumental Errors Due to used instrument e.g. missing part of the used tape, Horizontal axis not perpendicular to VL axis Personal Errors Due to the observer e.g. imperfect pointing, pointing to a different point, reading error, inaccurate horizontality of measuring device Prof. Dr. Ahmed Ragheb Lec. 4 ASU 114 Selected Topics in Contemporary Issues 2 Data Error Analysis Kind of Errors Blunders Mistakes Extremely wrong unrealistic error, unmeant typing or hearing error or due to forgetness (can be easily spotted) Usually personal error e.g. writing 67 instead of 76, Pointing towards a total different point, forgetting to fix horizontality or operation of any instrument Systematic Errors appearing in an orderly fashion, with the same sign (always +ve or always –ve) following a certain criteria Mostly instrumental error e.g. writing a totally different number, wrong counting, Axes misalignment Prof. Dr. Ahmed Ragheb Lec. 4 ASU 114 Selected Topics in Contemporary Issues 3 Data Error Analysis Kind of Errors Random Errors appearing in a non organized manner, not following any criteria or habit: -Can not expect its value, as it can’t be removed but only minimized -Probability of –ve errors = Probability of +ve errors -Probability of large errors is small, while probability of small errors is large -Large in number but small in value -Exists in any observation operation -Tend to cancel each other as they are +ve and –ve errors -Follow Gauss Probability Normal Distribution function e.g. different values for the same variable, imprecise pointing at target Prof. Dr. Ahmed Ragheb Lec. 4 ASU 114 Selected Topics in Contemporary Issues 4 Data Error Analysis Treatment of Errors Blunders Delete, Remove, Exclude GET RID OF IT this error completely from observations Systematic Remove by following certain Observation procedure, using MODEL THE ERROR a certain mathematical model or Calibration if instrumental Random Decrease its effect through TRY TO MINIMIZE THEORY OF ERRORS Note that this is done after removing blunders and systematic errors Prof. Dr. Ahmed Ragheb Lec. 4 ASU 114 Selected Topics in Contemporary Issues 5 Data Error Analysis Behavior of Errors Consider a single variable (say length L) measured more than once (say n times): i=n L1+L2+L3+……..+Ln ∑Li MPV (L)= = i=1 Arithmetic Mean n n For each measured quantity (i) of the variable L, the difference between variable value at (i) and the mean value is called Residual (V): Where Vi = L – Li For all quantities from i=1,2,3,…..n i=n If we consider ∑V i=1 i =0 plotting batches of Histogram the residual with a of Errors Always check certain interval (∆) against the number of frequency of This is occurrence of each called Equal batch, we get Histogram of weight Errors: Univariate statistics As it gets more symmetric, it shows homogeneity of observations Prof. Dr. Ahmed Ragheb Lec. 4 ASU 114 Selected Topics in Contemporary Issues 6 Data Error Gauss Probability Normal Analysis Distribution Function (NDF) If n is very very large tending to be ∞ and ∆ is decreased due to large no. of observations, the MPV value becomes the true value (L*), residuals are called true error (ξ), and the histogram transforms into a Normal Distribution Function (NDF) with no. of occurrence of any batch interval turns into probability: Properties of Gauss NDF 1- Bell Shaped 2- Area under the curve = 1 (Probability of all errors =100%) 3- Symmetric around ξ =0 4- Maximum at ξ =0 5- Parallel to ξ-axis at ξ = ±∞ Prof. Dr. Ahmed Ragheb Lec. 4 ASU 114 Selected Topics in Contemporary Issues 7 Data Error Analysis Measure of Errors Precision = Accuracy Precision if there is no systematic error Degree of closeness of repeated measurements to each other Measure of Internal consistency Accuracy Degree of closeness of repeated (random error) measurements to the true value Measure of External Consistency Prof. Dr. Ahmed Ragheb Lec. 4 ASU 114 Selected Topics in Contemporary Issues 8