Lecture 1-merged.pdf
Document Details
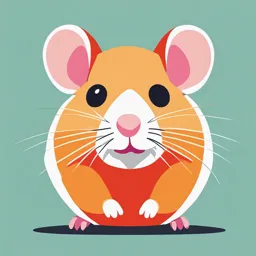
Uploaded by CleanlyRockCrystal4759
Vanier College
Tags
Full Transcript
1 Lecture 1: Quantitative Analysis in the Social Sciences & Measurement TOPICS: QUANTITATIVE DATA, RESEARCH METHODS, ETHICS, TYPES OF VARIABLES & SCALE OF MEASUREMENT, VALIDITY/RELIABILITY What we cover in 2 this chapter Brief history of...
1 Lecture 1: Quantitative Analysis in the Social Sciences & Measurement TOPICS: QUANTITATIVE DATA, RESEARCH METHODS, ETHICS, TYPES OF VARIABLES & SCALE OF MEASUREMENT, VALIDITY/RELIABILITY What we cover in 2 this chapter Brief history of QT data Current use of QT data Distinctions between QT and QL research Variables & Scales of Measurement Research Methods IVs, DVs, operational definitions Secondary Data Sources History of quantification in 3 Social Science In Western Europe, the usage of statistics for social studies began in the 17th century Data was collected about local populations The first study published was a demographic study (things like age, race, sex, employment, income, education etc) Later, in France, the minister ordered the first register of births, weddings and deaths Later, in the 18th and 19th century, statistics were used to understand social realities Court verdicts Election results Organization of hospitals Poverty Suicide Recognition of emergence of 4 quantification in the Social Sciences Next, surveys of the entire population became a governmental practice (the Census) In the US, the first census occurred in 1790 In Quebec (1867), laws were passed to collect data on education, agriculture, municipalities and civil status Example of first Quebec census below. Data was collected by door to door interviews of each household. Around this time, the Canadian government carried out its first Census – every 10 years at first and then every 5 years as of 1971. Census now: https://www12.statcan.gc.ca/census-recensement/index- eng.cfm Current use of statistics 5 Harm 6 Data collection and measurement is not without the potential of harm. Intentional: this would include deliberate manipulation of data and results Rigging of an experiment: sticking all the sickest people in the control group; giving too high a dose of drug to the control group etc Manipulating data and presenting it in a more favorable light (e.g. leaving out some participants), outright deliberate fraud (switching group labels on a graph/data set), making up data to skew results Unintentional: the study involves a sample of people who are not representative of the general population; the influence of confounds; media misrepresentation, experimenter influence/bias Example: Hormone replacement therapy & SES Unanticipated: IQ tests (now considered insufficient as a measure of the diversity and creativity of human intelligence) were used in Eugenics (selective mating of humans to improve the human species) which was associated with forced sterilization https://www.ted.com/talks/stefan_c_dombrowski_the_dark_history_of_iq_tests?su btitle=en Use of numbers and 7 measurement & related harms How do you know if you can trust the results of a study? Is it enough that a study is published in an academic journal after a peer review process? Under what conditions can you say that the results of some study provide strong enough evidence that you will change your own life habits? You need to be a critical consumer of scientific information Relying on the media is not a fail safe option – the media can/does misrepresent studies (e.g. going too far with the findings, according them too much importance) For example, do 3 glasses of milk really lead to early death?! (not according to the original article…) Is the peer review process flawless? Are all studies harmless and without error or manipulation? No! Bad studies do get published, results can be skewed (negative results left out) and this can cause harm. A famous example of this is explained in the next slide. The Lancet & The MMR- 8 Vaccine controversy In 1998 the Lancet published a study that claimed a causal link between the MMR vaccine (measles, mumps & rubella) and Autism. The study and the subsequent media firestorm that followed caused: a sharp drop in vaccination rates. an increase in the incidence of measles and mumps, resulting in deaths and serious permanent injuries. promotion of the claimed link, which continues in anti-vaccination propaganda to this day despite being refuted Following the initial claims in 1998, multiple large epidemiological studies (some with over 500 000 children) were undertaken: all found no link between the MMR vaccine and autism. It was later revealed that the studies’ author had engaged in data manipulation and data selection – the study was deemed fraudulent and retracted (in 2010)…. The fallout from the study has been characterized as “the most damaging medical hoax of the 20th Century” and it is still ongoing to this day….. Quantitative data 9 vs Qualitative data This course concerns itself with quantitative data/methods and not qualitative.. What is the difference between QT and QL research methods/data? Quantitative (QT) vs Qualitative (QL) research: more in depth 1. Words vs numbers: __________________ 2. Points of view: ___________________ 3. Distance: __________________ 4. Theory/concepts: _________________ 5. Generalizability: _________________ 6. Data: _________________________ 7. Macro vs micro: _______________________ 8. Artificial settings vs natural settings: __________________ Both QT and QL methods can be 13 used in a study Take a study done by researchers in Montreal whose objective was to verify reports of the increasing use of crack among drug users in downtown Montreal. Researchers used both QT and QL methods to fully describe the epidemic. The patterns, frequency and types of drugs being used were described using QT data methods via structured interviews of 387 addicts. Researchers also carried out unstructured interviews and observations of 64 addicts. The combination provided a full understanding of the drug use problem Which set of results represents QT 14 vs QL data? 15 QT & QL combined A team of teachers analyzing Vanier student files discovers that students identified as at risk during midterm assessment were more likely to drop out of college in the next year than those identified as failing or not at risk of failing. This is a surprising finding as one would expect that it is those flagged as “failing” that would be at greater risk. The information from the quantitative data is insufficient in explaining this result QL methods are needed to carry out in-depth interviews with students who were at risk of failing and later abandoned college to explain the surprising result. Terminology: Hypothesis,Variables Data, Individuals 16 Data (what we collect) is provided by ‘INDIVIDUALS’ Individuals can be people, animals or things. However, in the social sciences we stick to human subjects or the products of human life (documents, speeches etc) Can be referred to as ‘participants or subjects’ as well Terminology: Variables 17 When researchers decide to Examples of variables: conduct a study, they might start with a question of interest Age or a hypothesis that they will Intelligence test. They also need to select Reaction time and define the ‘variables’ Educational attainment that they will collect data on Academic achievement Variables are any Aggression characteristic of a human Self esteem that can be observed, Mental health manipulated and measured Buying habits In the social sciences, Attitudes variables tend to reflect Political affiliation characteristics of people: Gender Formulating Hypotheses 18 In QT research, investigators tend to test ’hypotheses’. A hypothesis is derived as It is hypothesized that the logical next step from the quality of ones the body of pre-existing romantic relationship will scientific knowledge be correlated with a A hypothesis is a longer life statement of what the Participants who eat a investigator expects to find in their study (i.e. the 100% vegan diet versus expected outcome of a diet with meat will rank the research) and reveals themselves higher on the key variables that the global measures of research will be mental health investigating: Can you find the variables? 19 Example 1 Example 2 It is hypothesized that Participants who eat a the quality rating of 100% vegan diet versus a one's romantic diet with meat will rank relationship will be themselves higher on correlated with a longer global measures of life mental health Variable 1: ________ Variable 1: ________ Variable 2: ________ Variable 2: ________ Activity: Identify the 20 variables 1. Researchers examined the amount of milk consumed by participants (high vs low) and the quality of their bone densities 2. It hypothesized that the more hours spent on social media per day, the higher the risk of depression and anxiety. 3. Researchers were interested in the the relationship between income levels and happiness. It was predicted that as income increased past a certain point, there would be no further improvements in happiness levels. Which of the above is not a ‘hypothesis’? Activity: Formulate your 21 own hypothesis 1. Depression & Quality of Friendships 2. Eating Habits of Children & Eating Habits of Parents 3. Self Esteem & Fitness level Operational Definitions 22 Once a ‘variable’ of interest has been selected, researchers decide on how to measure that variable. The detailed explanation of the way a variable will be measured is that variable’s ‘operational definition’ (OD). ODs should be clear, detailed, precise and reproducible explanations of how each variable will be measured. For many variables, there are a multitude of ODs; for some variables, the options are more restricted: For example, to measure ‘weight’, a researcher would use a well calibrated scale. The OD here is straightforward (a scale) However, a variable like ‘happiness’ poses a far greater challenge. In this case, researchers might rely on a previously validated ‘survey’ that has proven adept. Alternately, a study might simply ask participants to rate how happy they are on a scale of 1 to 5. Whatever the choice, an OD must be provided. Measuring intelligence with the IQ score is still controversial and complicated – whatever the method used is, it must be clearly explained Operational 23 Definition: Variable = Fitness VO2 max (respiratory fitness) Participants will be fitted with a face mask (which can directly measure the volume and gas concentrations of inspired and expired air). The test involves either exercising on a treadmill or a bike at an intensity that increases every few minutes until exhaustion. Endurance test: Participants will be expected to run as far as possible in 12 minutes (Cooper Test) BMI Participants will have their height and weights measured and their BMI will be calculated. Push up test Participants will complete as many push-ups as they can at a rhythmic pace (no long breaks between push ups) Activity: what is the OD in 24 each example? A researcher is studying the effect of sleep on aggression and believes that less sleep will lead to more aggression. After manipulating the amount of sleep different groups of participants receive, she monitors aggressive behavior of all participants during a basketball game. During the game, she counts the number of aggressive acts; defined as ‘pushing, gesturing or yelling at other players’. Researchers think that visualizing the sports activity you will engage in will improve performance in that sport. They collect a sample of 30 students and divide them into two groups. One group is instructed to imagine successfully throwing a basketball into a hoop over and over. The other group is told to think about their last year’s birthday party. Following the visualization sessions, both groups will engage in a ‘free throw’ session during which time the number of successful baskets will be recorded from the ‘3 point line’. Participants will each have 25 shots on the basket. Solution: what is the OD in 25 each example? A researcher is studying the effect of sleep on aggression and believes that less sleep will lead to more aggression. After manipulating the amount of sleep different groups of participants receive, she monitors aggressive behavior of all participants during a basketball game. During the game, she counts the number of aggressive acts; defined as ‘pushing, gesturing or yelling at other players’. Researchers think that visualizing the sports activity you will engage in will improve performance in that sport. They collect a sample of 30 students and divide them into two groups. One group is instructed to imagine successfully throwing a basketball into a hoop over and over. The other group is told to think about their last year’s birthday party. Following the visualization sessions, both groups will engage in a ‘free throw’ session during which time the number of successful baskets will be recorded from the ‘3 point line’. Participants will each have 25 shots on the basket. INDIVIDUALS, VARIABLES & 26 DATA Look at the table below. How many individuals are there? What is/are the variables? What is an example of ‘data’? Participant Gender MAJOR AGE GRADE 1 Male COMMERCE 18 A 2 Female HISTORY 18 C 3 Female PSYCHOLOGY 20 B Notice that each of these variables produced different kinds of responses that cannot be summarized in the same way. These variables have different scales of measurement. Variables and Scales of 27 Measurement Variables can differ according to the underlying properties of the responses they can produce We call this ‘scale of measurement’ The scale of a variable affects the kinds of mathematical operations, graphs, descriptive and inferential tests (statistical analyses) that should be used Therefore, identifying a variables scale of measurement is critical Qualitative (categorical) vs 28 Quantitative (numerical) With Qualitative variables (QL), the different responses possible on the variable represent distinct categories It is only possible to summarize a QL variable using counts (adding up the number of people in a particular category) or a percentage per category (adding up and dividing by the total people in the study) Example: Variable: Hair Color Response options: a) Brown b) Red c) blond d) black Generally, you would select one answer from the above options. Each answer differs from the others in kind – but not in ‘number’ (blond is not ‘2 units’ bigger than ‘black’). You cannot assign any meaningful numbers or values to these answer and therefore you cannot perform any and all mathematical operations on this variable (e.g. calculate a ‘mean’, add or subtract response option). The difference between categories is in kind – not number. Additional Examples 29 A survey or census will ask questions that may produce qualitative data. Would the questions below produce qualitative data? Why? 1) What is your living 2) How would you rate the arrangement? service at this restaurant? a. With roommates a. Excellent b. Alone b. Good c. Fair c. With parents d. Poor d. With family members e. Very poor e. Currently homeless Qualitative: Nominal vs 30 Ordinal With nominal variables, individual’s responses belong to one category or another and there is no sense of magnitude when comparing the categories You cannot make greater than or less than statements (they simply make no sense!) There is no inherent ranking of the data, even if arbitrary codes are given for the various values, one code may be numerically greater than another – but the characteristic they represent is not! (e.g. Male =1, Females = 2) With ordinal variables, responses still belong to one category or another, but there is a natural order or rank to the responses that would allow greater or lesser than statements. There is an ‘order of magnitude’ Qualitative: 31 Ordinal Example: Race standings, Competitors will finish 1st, 2nd, 3rd etc The difference between 1st and 2nd place may not be the exact same as between 3rd and 4th , but 1st and 2nd beat 3rd and 4th Presented in this way (without precise race times), this variable is ordinal. Example: Ordinal Data cont’d 32 1. Likert scales: Many surveys ask respondents to rate some emotion or feeling on a scale: This is an example of a Likert Scale The numbers on this type of scale represent ‘feelings’ or ‘states of being’ or qualities of a person. If the scale changes, (1- 30), the persons selection would also change (although their happiness level hasn’t! – the numbers are arbitrary). We cannot be sure the difference between a ‘2’ and ‘3’ rating is the same as a ‘4’ and ‘5’ rating, but we know 4 is less than 5. 2. Ranges of numbers are also ordinal. We don’t know the exact difference between choices (a vs b vs c etc), but we know one choice is bigger than another. For example: Q2: How many siblings do you have? If someone selects b and a) 0 someone else c, we b) 1-2 don’t know the exact c) 3-4 difference in number of d) 5-6 siblings between them (could be 1 to 3 siblings) e) 7 or more Remember these two examples? They both represent qualitative variables 33 (‘living arrangement’, ‘service opinion’) but reflect different sub-scales of measurement. 1) What is your living 2) How would you rate the arrangement? service at this restaurant? a. With roommates a. Excellent b. Good b. Alone c. Fair c. With parents d. Poor d. With family members e. Very poor Nominal Ordinal Quantitative 34 variables (vs Qualitative) Quantitative variables (QT) can be measured precisely (decimals are possible) and they take on precise numerical values (i.e. a number) that represent different amounts or quantities of a characteristic. As such, QT data can be subjected to mathematical procedures including: addition, subtraction, averages, multiplication etc We further divide QT data into Interval or Ratio scales (the distinction will not be made in this course, therefore, all QT data is “interval/ratio’ in its sub-scale). 35 Examples: The variable height is an example of a quantitative variable When asked to give your height, participants will report a numerical value (measured in inches, feet or meters) As such, the differences between response options can be precisely quantified: For example, a person that is 5 feet tall is 1 foot taller than a person who is 4 feet tall (5-4=1); similarly, a person who is 6 feet tall is 1 foot taller than someone who is 5 feet tall The numbers all follow a predictable sequence, with equal intervals between each Decimals are possible Multiplication and division is possible: 6 feet is 2 times greater than 3 feet etc Averages can be calculated: the average height of this sample is 5.5 feet Additional examples 36 How many hours How much does How old are you: do you spend your family make a Ans: 25 years old surfing on the year? Ans: 25 000$ internet? Ans: 14 hrs How many times do you drink How many cars do alcohol a week? you own? Ans: 4 Ans: 28.5 drinks a cars week In many cases, researchers can influence scale of measurement (and precision of data 37 obtained) Five (5) students Five (5) students Five (5) students provide information provide information provide information about grades: about grades: about grades: Student 1: A+ Student 1: 95-99+ Student 1: 99% Student 2: B Student 2: 80-84 Student 2: 84% Student 3: A Student 3: 90-94 Student 3: 90% Student 4: C Student 4: 75-79 Student 4: 75% Student 5: B Student 5: 80-84 Student 5: 80% Qualitative Qualitative Quantitative Ordinal Ordinal Interval/ratio Activity 38 For each of the following examples: 1) Identify the variable 2) Pick the scale of measurement (qualitative or quantitative) 3) Decide on the subscale: nominal or ordinal or interval-ratio For each of the following examples: 1) state the variable name 2) pick the scale of measurement 39 (qualitative or quantitative) & decide the subscale: nominal/ordinal/interval-ratio 1. A grocery store owner gives a code ranging from 1 to 1500 for each product in his cereal/crackers section. 2. The temperature in a small town in Europe has been recorded for 5 days. 3. Students are asked to rate their favorite animal: Student 1: cats; Student 2: turtle; Student 3: cat; Student 4: dog; Student 5: fish; Student 6: cat; Student 7: dog 4. Children in elementary school are evaluated and classified as ‘non-readers’, ‘beginning readers’, ‘grade level readers’, or ‘advanced readers’. The classification is done in order to place them in reading groups. 5. A college finds 80% of their students identify as ‘female’. 6. Researchers measure various characteristics in a new daycare and find that the most common number of children in each class is ‘16-20’ (response options included: 11-15, 16-20, 21-25, 26-30). 40 MEASUREMENT: ASSESSING THE RELIABILITY & VALIDITY OF VARIABLES MEASUREMENT 41 Reliability and validity are central to social science research and are factors to consider for researchers when they select instruments to make their measurements Examples of instruments or measurement tools are varied: A tape measure for length, an IQ test for intelligence, a blood pressure machine for blood pressure measurement, a scale for weight, a single question or series of questions measuring happiness, personality etc When using some device or test/survey to collect data, researchers must make sure that it is a good, consistent measure of the characteristic or variable in question. Measurement devices can be Valid or not valid Reliable or unreliable The validity or reliability of a test or measure can greatly affect the quality of your research – therefore it must be evaluated carefully! An example to illustrate 42 Validity and Reliability A school in the US has lost all of its applicants IQ tests! The principal needs to find a way to re-assess students intelligence and quickly. The admission results deadline is 6 hrs away! To fix the problem, the principal compiles a team of staff and teachers. Each applicant is then called in and the ‘circumference of their head’ is measured with a tape measure. Based on these tesults, the principal makes her final selection for entry into the school. The principals quick fix was ‘reliable’ but not ‘valid’. Explain. Validity & Reliability: 43 Defined Validity: validity is the extent to which the instruments used in a study measure exactly what they are intended to measure. In our example, we are interested in the variable/construct of ‘intelligence’, however, head size is not a good (not a valid) measure of intelligence. Reliability has to do with the consistency of a measurement tool or test when the measurement is repeated multiple times. A reliable measurement tool will always produce nearly the same result if you use it multiple times on the same person. In our example, the tape measure used is probably a reliable tool for head size. It will produce the same head size measurement over and over if used again and again on the same person. How to detect and fix issues 44 with Validity and reliability Reliability: We can check reliability by taking many independent measurements on the same person. A reliable tool will produce the same or nearly the same result when used multiple times on the same person. One way to improve reliability is to improve your instrument ****If a tool is not reliable, it cannot be valid**** Validity for some variables is harder to assess than others. Tape measures, scales and blood pressure machines are clearly valid ways of measuring weight, height and blood pressure (although a particular machine may need calibration/be broken). Finding a valid measure of human characteristics (personality, temperament, IQ, happiness, well-being) is more difficult and complicated. To make sure an instrument is valid, you could compare it to another instrument with known validity To fix issues with validity, you must make a better instrument Validity & 45 Reliability A researcher interested in assessing ‘happiness’ decides to count wrinkles around the eyes of his participants. He takes detailed pictures of their eyes, magnifies the pictures and, using a computer, quantifies the amount of ‘wrinkles’ around their eyes. 1. Is this reliable (what is the reliability aspect?)? How could you find out? 2. Is this valid (what is the validity aspect?)? Why or why not? Bias (or systematic error) & 46 random error We can further classify the error in measurement made by an instrument into 2 types : random error and bias (systematic error). Random error is measurement error that is sometimes an overestimate and sometimes an underestimate (unreliable) and is due to the precision limitations of your instrument Random error can be addressed by increasing the number of measurements per person and reduced by averaging across many measurements per person. A reliable measurement tool will produce very little random error Bias: a systematic over or underestimation of the true value of the variable Cannot be addressed by changing the number of measurements, one must change the instrument. This is difficult to catch but very important to watch for! A visual representation of validity, 47 reliability, bias/random error BIAS RANDOM ERROR BIAS 1 2 3 4 Imagine we weighed the same person 10 times (using 4 different scales). Each black dot represents that person’s weight after each measurement. The center dot is the ‘true weight’ of the person. Activity: Measurement Error 48 A scale is usually a valid way to measure weight. However, a particular scale may be old, rusty and badly calibrated and lead to measurement error. Imagine your true weight is 200lbs. In each example below, 3 measurements were taken using 3 different scales. Determine the kind of error that applies in each case. Is there bias, random error? Are the scales reliable/valid? Scale 1: 400, 401, 400 Scale 2: 200, 201, 199 Scale 3: 45, 50, 39, 79 Activity: Measurement Error 49 A scale is usually a valid way to measure weight. However, a particular scale may be old, rusty and badly calibrated lead to measurement error. Imagine your true weight is 200lbs. In each example below, 3 measurements were taken using 3 different scales. Determine the kind of error that applies in each case. Is there bias, random error? Are the scales reliable/valid? Scale 1: 400, 401, 400 Biased: Reliable, Not valid Scale 2: 200, 201, 199 No Bias/No or low Random Error: Reliable & Valid Scale 3: 45, 50, 39, 79 Biased: Not reliable, Not Valid Activity 50 1. Dr Roberta has developed her own version of a personality test. She gives it to her colleague over 5 consecutive days to test its reliability and validity. These are the scores: 175, 159, 180, 155, 190. On a validated test, her colleague scored 170. a. What is the measurement tool? b. What type of error is this test producing? How do you know? c. Does Dr Roberta have a problem with reliability or validity or both? Explain. 2. A researcher interested in the relationship between diet and health uses BMI to measure health. The tape measure he used to assess height (for the BMI measurement) was produced incorrectly and overestimates height by 3 centimeters, consistently. a. What is the measurement tool? b. Is this tool reliable? How do you know/not know? c. What type of measurement error is this tape measure producing? d. Is it valid? Why or why not? Research Methods SURVEYS, CENSUS, EXPERIMENTS, CONTENT ANALYSIS & NON-PARTICIPANT OBSERVATION 52 Research Methods Researchers use different methods to collect first hand data in the social sciences Various social science disciplines tend towards some methods over others The method chosen by the researcher is affected by the research question and what is possible/ethical Each method has its own strengths and weaknesses 1. Sample Surveys 53 Survey research collects information from a large, pre-defined group of individuals (a sample) by asking questions The main tool is a questionnaire or survey (mailed, emailed, or given in person) or a structured interview (with pre-set questions) Questions can be on many different measurable characteristics/variables of interest: Political opinions, health habits, attitudes, beliefs, well-being, height, weight, self esteem, happiness, attitudes, beliefs etc etc) Studies that use the survey method commonly assess multiple variables in the same study Surveys are very commonly used in the social science disciplines of Psychology or Sociology to assess thoughts, opinions, behaviors etc. Measure variables of Identify interest using a questionnaire or structured population and select a interview 54 large sample To conduct a survey a researcher first identifies a target population (i.e. the entire group of people they are interested in getting information about) and then, selects a sample from this population (more to come on this in a later chapter) No intervention is applied to the sample; rather participants simply complete a survey or questionnaire Survey research allows for the quick collection of data from a large group of people about a wide range of topics. Survey research is plagued by some issues: Making causal claims (i.e. one variables causes another) are not possible even if the samples are large (more to come on this later). Therefore, if researchers find a link between ‘drinking coffee and cancer’ – they cannot claim that drinking coffee actually CAUSES cancer, but only that there ‘might’ be some link (or not) and further research using a more robust research method is needed. Respondents can lie on questionnaires or be forgetful: the results are only as good as the memories or truthfulness of the respondents. Examples: CCHS 55 Study Canadian Community Health Survey is a Statistics Canada yearly survey that covers the population 12 years of age and over living in the ten provinces and the three territories of Canada Target population: All Canadians 12 years + Sample size: 65 000 Canadians, aged 12+ Method: telephone interviews The CCHS is a cross-sectional study: people are surveyed one time period, and not followed over time. All data collected is a snapshot of the individuals surveyed at one point in time. Example of questionnaire used by Statistics Canada for the Canadian Community Health Survey: https://www23.statcan.gc.ca/imdb/p3Instr.pl?Function=as sembleInstr&lang=en&Item_Id=1533125 List of variables: https://www23.statcan.gc.ca/imdb/p2SV.pl?Function=getSurv VariableList&Id=1531795 Operational Definitions: https://www23.statcan.gc.ca/imdb/p2SV.pl?Function=assembl eDESurv&DECId=433572&RepClass=591&Id=1531795&DFId=1535 560 Example: Harvard 56 Happiness Study Main objective: What life factors (social and psychosocial) promote health and well being in later life? Sample: 724 men followed annually since 1938 Method: In person interviews, surveys, access to medical records and brain scans at various time intervals (annual visits were common) The HHS study is a Longitudinal Study: the same people are followed over time and interviewed at various time points throughout the course of the study Conclusions: https://www.ted.com/talks/robert _waldinger_what_makes_a_good_ life_lessons_from_the_longest_stud y_on_happiness?subtitle=en Survey 57 Research: The Census A census involves the systematic collection of information on the population of a particular country. In Canada, the census is done every 5 years. https://www12.statcan.g c.ca/census- recensement/index- eng.cfm The census mainly gathers demographic information. This information includes where people live, as well as their age, sex, marital status and ethnic origin. 2. Experiments 58 Experiments are a type of research method designed to test predictions about cause-effect relationships. A well designed experiment will allow researchers to claim that one thing in fact causes another – this is not possible in even the best designed survey research study. Experiments are usually conducted in a controlled environment (laboratory or university setting). This allows researchers an element of control over many aspects of the environment. In an experiment, researchers determine a target population, select a sample and then divide that sample of individuals randomly into at least two groups (there can be more). The groups are referred to as: the control and the experimental groups. Identify Randomly allocate CONTROL GROUP population participants to control and of interest experimental and select groups a sample EXPERIMENTAL GROUP Next, researchers deliberately impose an 59 experience or treatment or event on the subjects in the experimental group a dose of drug a new drug a change in room temperature a new relaxation technique A new therapeutic approach Or anything that will not produce harm! The control group does not experience this treatment or event – however, they are treated the same as the experimental group in every other way We call the treatment or event the Independent Variable There are always at least 2 levels of the IV (there can be more) to reflect how it varies across the groups of the experiment! Following the treatment/exposure – both groups are compared to see if any differences are apparent. The outcome of interest is called the Both groups are compared Dependent Variable (DV). on the dependent variable Note that the terms IV and DV are used in the context of experiments and not in other research methods Example 60 Human Sleep Study Example: Researchers believe that “having less than a full nights sleep will result in impaired mathematical problem solving abilities”. Participants aged 18+ are brought into a sleep lab and allowed to sleep either a full nights sleep (8 hours) or are awakened after 4 hours, or 6 hours of sleep. Both groups are then given the same math test. Identify: 1. The Independent and Dependent variable 2. The different levels of the Independent Variable 3. The control vs experimental group Solutions: #1 & 2 61 The IV is the variable that is manipulated by the researchers. In this study, the IV is the ‘amount of sleep’. There are always at least 2 levels of an IV, to reflect the fact that there are always at least 2 groups in an experiment (there can be more, as in this case). In this experiment, the IV varies in 3 ways: 8hrs sleep, 4hrs sleep and 6 hrs sleep. Therefore, there are 3 levels to the IV. Dependent Variable: Math abilities The DV is the variable that is being ‘measured’ or the ‘outcome’ variable. In this example, the researchers are interested in measuring ‘Mathematical problem solving abilities’; this the Dependent Variable. Solutions: #3 62 In every experiment, there are control and experimental group: In this study, the control group would be made up of participants that are in a normal, untouched condition - in this case, the group that sleeps a full nights sleep: 8hrs of sleep Note: Were this a ‘drug’ study, the control group might be the group that gets ‘no drugs’ or ‘the standard drug’ Control Group Experimental Experimental (if a new drug is being tested). Control Group #1 Group #2 groups always get the basic, standard condition. Dependent Variable: The experimental groups are the Math abilities group that receive some manipulation or treatment or event that differs from normal. In this example, there are 2 experimental groups: Experimental Group 1: 4 hours of sleep Experimental group 2: 6 hours sleep Experiment (medical sciences): Randomized Controlled Trial of the 63 Pfizer Vaccine No Vaccine Control Group Experimental Group? IV? Levels of the IV? Vaccine Group DV? Experiment Examples 64 Social Eating Experiment: In a series of experiments, researchers evaluated the influence of a stranger’s snacking behavior on snack Can you identify: consumption of the participant. Participants were either exposed to another person that snacked heavily, The IV? very little or not at all (no other person was present); all The DV? while in a university lab waiting room. The Control Group? Facebook Mood Study (ethical grey zone). How will The the emotional tone of Facebook content impact the experimental emotional output of the user? Group? For one week in January 2012, data scientists skewed what almost 700,000 Facebook users saw when they logged into its service. Some people were shown content with a preponderance of happy and positive words; some were shown content analyzed as sadder than average. When the week was over, these manipulated users were more likely to post either especially positive or negative words themselves as compared to their pre-experiment baseline posts. Content Analysis 65 Content analysis is a research method used for studying and/or retrieving meaningful information from documents A content analysis is considered an ‘unobtrusive method’ as researchers do not interact with human participants – rather they only interact with the products of human activity. Generally, a content analysis is used to determine the presence of (and to quantify) certain aspects of documents including: words, themes, concepts, phrases, ideas, images, characters, or sentences within texts or sets of texts It uses any communication type materials: Magazines, books, newspaper headlines, email traffic Transcripts of interviews, speeches, conversations TV shows, commercials, internet sites, movies, videos, programs Documents of any sort (official, unofficial) 66 Examples of Content Analysis 67 Example of Content Analysis Non-participant Observation Non-participant observation: Researchers insert themselves into a public or private place(a natural setting) and observe people for academic and intellectual purposes. The goal of this kind of research is to permit the natural flow of life and for the researcher to record aspects of the behavior of the group they are observing without interfering with the group. Participants may be unaware they are being observed or may not ever interact with the researcher Usually a tally table is prepared in advance and each characteristic, behaviour and/or event of interest is recorded/tallied as it occurs. Examples: The nature of play by children in a playground. What age group, gender or ethnicity is most likely to jaywalk? How often do doctors in a hospital wash their hands between patients? Secondary data 69 sources and analysis In a secondary data analysis, researchers use and analyze data that was collected or created by someone else or an organization but to answer a different research question. This method is often cheaper than collecting one’s own primary data. The disadvantage of this method is that one is limited by the data provided in terms of what can be investigated (variables selected by the primary study; operational definitions decided by the primary study etc). Examples of Secondary 70 Data Sources In CANADA Statistics Canada https://www.statcan.gc.ca/en/start Institut de la statistique du Québec https://statistique.quebec.ca/fr In the USA PEW Research Centre https://www.pewresearch.org/our-methods/ In the WORLD UN data on many topics such as crime, education, employment, environment, health, human development, etc. http://data.un.org/ 71 end LECTURE 2: GRAPHS WHY DO WE NEED GRAPHS? A picture is worth a thousand words (or lines of data)! Graphs allow us to summarize large amounts of information into one image whereby trends and patterns can be seen almost immediately EXAMPLE: AVERAGE IQ’S OF 112 QM CLASSES ACROSS 8 REGIONAL CEGEPS GRAPH DEPICTING THE AVERAGE IQ OF QM CLASSES ACROSS 8 REGIONAL CEGEPS Average IQ GRAPHS THAT WE WILL COVER IN THIS MODULE 1. Bar graphs 2. Pie charts 3. Line graphs 4. Histograms HOW TO CHOOSE THE RIGHT GRAPH ❖Choosing the right graph starts with a decision about what you what to portray. It is a good idea to think about what the title of your graph would be – this will help you to narrow down the variables that will make up your graph! ❖Another important consideration is the scale of measurement of the variables that will make up your graph ❖In general: ❖When dealing with QUALITATIVE data, pie charts and bar graphs are the graph of choice ❖When dealing with QUANTITATIVE data, line graphs and histograms are appropriate. BAR GRAPH Bar graphs look like the way they sound, they are graphs made up of ‘bars’!! Bar graphs are used when we are plotting different categories of a QL variable. As such, in a bar graph, you will find that each bar represents one of the categories of the Q L variable in question and the height of each bar represents the magnitude of each category. In a bar graph, the Y axis is reserved for ʻquantitative data/variableʼ while the X axis is reserved for the ʻcategories of the qualitative variableʼ The y-axis can plot an average, a sum, a count or total number of observation or a percent Y axis (quantitative) Y-axis (number of births) = a count or total # of observations or X axis absolute frequency (categories/qualitative) BAR GRAPHS ❖Consider the data collected into the table below. How does one decide a bar graph is appropriate? Absolute Relative frequency frequency The first column depicts ‘Level of Education’ (a QL variable). The next column (Number of persons) represents the number of people within each level of education (a tally/count) or absolute frequencies (QT) The final column (Percent) depicts the percentage of people with each level of education (constructed by dividing the count within each category by the total number of people X 100) or relative frequencies (QT) The decision to use relative vs absolute frequencies is more or less arbitrary and up to the researcher. Relative frequencies are easier to work with and allow for quick and easy comparison. HOW TO COMPUTE A RELATIVE FREQUENCY To compute a relative frequency, we divide the individual ʻcountsʼ (number of persons in a category) by the total number of people in the sample. We then multiply by 100 to get the relative frequency (or percent) (i.e. amount per 100 people). Example: ‘Less than high school’: 27 896/191 884 X 100 = 14.5% Graph depicting the education level of people aged 25 years and older y-axis = a percent or relative frequency BAR GRAPHS: SUMMARY 1. Bar graphs plot categories of a Qualitative variable. The height of each bar represents the amount of each category. 2. The y axis can plot a sum, average, count or absolute frequency or percentage (relative frequency). 3. The QL variable always appears on the x axis 4. Bars should be ‘equally wide’ 5. Bars should NOT touch and be equally spaced 6. Each axis should have a clear label and a chart title should be present PIE CHARTS ❖Pie charts are an alternative to a bar graph and are used in certain cases Pie charts are a circular chart (like a pizza) divided into ‘slices’ that add up to 100% of the data Pie charts are reserved for QL variables; as such, each slice of a pie chart represents a category – the bigger the size of a slice, the bigger the % of the whole that category represents The slices of a pie chart must all add up to 100% (bar graphs do not have this same restriction as data can be plotted using ‘counts’ and not percentages). PIE CHART EXAMPLE How phones vs tablets are used Same data: Bar vs Pie Bar Graphs Pie Charts WHEN NOT TO USE A PIE CHARTS 1. When values are too similar bar graphs show trends better 2. When the number of categories is high: rule of thumb is if they exceed ʻ6ʼ use a bar graph 3. When the available categories do not total 100% of data for example, if participants can select more than one response option. HOMEWORK ASSIGNMENT QUESTION AREA CODE NUMBER OF The following table depicts the frequency of various telephone area codes for a STUDENTS randomly selected sample of students in a 514 14 first year CEGEP course. The area codes 418 25 represent regions in and around Montreal, QC and help administrators anticipate travel 581 8 times for students attending the CEGEP. 438 33 1. Draw a bar graph of this data using 873 10 relative frequencies. Label all axes. 613 6 819 1 LINE GRAPHS ❖A line graph is a type of graph that displays how one QUANTITATIVE variable varies over some time interval (also a QT variable) ❖The x-axis of a line graph is reserved for the time factor (which could be: seconds, minutes, hours, days (1, 2, 3 or Axis title April 10th, April 11th etc), years (1,2,3 or 2019, 2020, etc), decades etc) ❖The y-axis in a line graph usually indicates the quantity or percentage of the second variable at each time point Axis title ❖Paired data in line graphs are represented by a ‘dot’; dots are connected with a line. ❖Line graphs are great for showing trends in a variable LINE GRAPHS REVEAL TRENDS QUICKLY Percent (%) EXAMPLE: PLOTTING A LINE GRAPH Table 1: Number of People at a local music store ❖The table on the right during opening hours (24 presents data on the amount hr clock) of people present in a store Time of day Amount of (24 hour people in store during a typical work day. clock) ❖To determine whether a 10:00 2 particular data set is suited 11:00 5 to a line graph ask: Are both 12:00 12 variables quantitative and is 13:00 22 there a ‘time aspect’? 14:00 16 15:00 6 16:00 7 LINE GRAPH Non-time related variable on Y axis Line is joined across data Each dot points represents 2 scores Time factor always on X CAUTION! Always beware of the scale of the data you are looking at Playing with the the scale of an axis is a tactic used to convey data in a more or less favorable light. For example, changing the scale or stretching the axis can exaggerate or minimize certain differences and change the impact. Do the two graphs below depict the same data? Number of unmarried couples 24000 Number of unmarried couples unmarried couples (thousands) 22000 20000 18000 16000 14000 12000 10000 8000 6000 4000 2000 0 1980 1982 1984 1988 1990 1992 1996 1998 2000 2002 2004 year How do you decide how to portray data fairly? Be aware that using a small scale will focus on a small range and therefore exaggerate small differences. A large scale will minimize differences. HOMEWORK ASSIGNMENT QUESTION Jane is tracking her dog Spot's growth from 1 month old to 9 months old. She kept track of his weight gain and recorded it in the table below. 1. Make an appropriate graph of this data, describe the trend of the graph and anything that stands out. 2. Make a second graph with a y axis that ranges from 1 to 100lbs. Age Weight in pounds (months) (lbs) 1 8 2 13 3 16 4 19 5 22 6 30 7 27 8 22 9 27 HISTOGRAMS Histograms allow us to see how one quantitative variable is distributed in a group of people; it can quickly show us the major features of the distribution of one variable To know if a histogram is appropriate, look at the variable of interest (e.g. Weight, Height etc) and determine if it is measured on interval/ratio scale of measurement. If there is no ‘time’ aspect, and only one variable being graphed, a histogram is likely appropriate. Histograms look very much like bar graphs except that the bars of a histogram are glued together. This is to illustrate the ‘continuous nature’ of QT variables. BAR GRAPH VS HISTOGRAM HISTOGRAMS ❖In a histogram, the Y axis plots ʻfrequencyʼ data Frequency ❖Frequencies tell us ʻthe number of times a particular score or value on the X axis has occurredʼ ❖The X axis represents values of the quantitative variable in question (e.g. grades, weight etc). Most histograms plot ranges of values along the x axis. This means every bar represents a range of scores or values. In the graph on the bottom right, each bar represents a range of ’10’ points on the variable in question HOW TO MAKE A HISTOGRAM WITH CLASS INTERVALS Step 1: The first step is to decide on the width of each interval (5 or 10 is used often, this will be told to you on tests, exams and assignments) Step 2: Create a frequency table and begin by building your first class interval Look for the smallest score in the raw data. Is it a perfect multiple of your ‘interval width’ (if you divide the smallest score by your interval width, is the result a whole number)? If it is, this will be the first number of your first interval. Not a perfect multiple? Pick the very next SMALLER number that is a perfect multiple of your interval width. This will be the number you will use to start your very first interval. Step 3: Complete your frequency table by constructing all of your remaining class intervals until the largest score in your dataset has been contained in an interval. Now, tally how many scores fall into each of your intervals. Step 4: Plot everything in a histogram, placing the frequency counts on the y axis and the values of the QT variable on the x axis. Make sure bars are stuck together. Label all axes. BLACKBOARD DEMO Participant Hrs on Social Media Sites (per week) 1 11 A researcher collects data on 2 12 social media usage in her 3 19 Grade 6 class. Using a class 4 22 interval width of 5, make a 5 23 histogram using the following 6 24 tabled data. 7 25 8 27 9 29 10 35 11 36 12 37 13 45 14 49 STEP BY STEP EXPLANATION Remember to start by finding Number of hours Frequency the smallest number in the data on SM (x) set (11) 10-14 2 Is it a perfect multiple of the interval width? 15-19 1 11/5=2.2 (not a whole number)! 20-24 3 Go to next smallest (10)….10/5 = 2 (a 25-29 3 whole number). 10 becomes our starting point! 30-34 0 35-39 3 Build your frequency table! 40-44 0 45-49 2 HOMEWORK ASSIGNMENT QUESTION Student Grade 1 84 Task: Make a histogram using the tabled data 2 91 on the left which depicts student Math scores 3 75 (%) on a recent test. Use a class interval of 4 68 size of 4 (n=13). 5 78 1. What interval of grades represents the most common test score? How many students 6 77 are in this interval? 7 86 2. How many students obtained a grade in the 8 94 highest grade interval (best grade) and 9 64 lowest grade interval (worst grades)? 10 59 3. How many students passed (60% or 11 54 greater)? 12 89 13 76 IN-CLASS ACTIVITY: SOLUTIONS Frequency Table Smallest score: 54; interval width: 4; perfect Math Score Frequency multiple: 52 52-55 1 1. What interval of grades represents the most common test score? How many students are in this 56-59 1 interval? 60-63 0 Ans: 76-79, 3 students 64-67 1 2. How many students obtained a grade in the 68-71 1 highest grade interval (best grade) and the lowest 72-75 1 grade interval (worst grades)? 76-79 3 Highest (92-95): 1 student; lowest (52-55) 1 student 80-83 0 3. How many students passed (60% or greater)? 84-87 2 11 students passed. 88-91 2 4. 92-95 1 DESCRIBING DISTRIBUTIONS ❖Histograms can reveal key features of a variable. ❖We generally describe histograms in terms of their: 1. Shape: symmetric, left or right skew 2. Center 3. Spread 4. Presence or absence of outliers SHAPE: SYMMETRIC A symmetric histogram looks like this one. There is one peak, it is roughly in the middle. One half of the graph is approximately a mirror image of the other half. 50% of scores fall to the left and right of the center of the graph. SHAPE: HISTOGRAM WITH RIGHT VS LEFT SKEW The two graphs on the right represent a right and left skew, respectively. In a right skewed histogram (top graph), there are many low values and not very many high values (a very hard test might produce this kind of shape) In a left skewed histogram, there are many high values and not very many low values (a very easy test might produce a shape like this) ʻSPREADʼ AND ‘CENTER’ 1. The ʻSpreadʼ of a histogram is the span between the smallest and largest value (e.g. 0-100) The spread can be large (a big distance between the smallest and largest number) or small (not much distance between smallest and largest number) Spread (using intervals) = 45-49 to 95-99 or low score to high score (based on actual data): 46-97 2. The ʻcenterʼ is the middle of the histogram such that 50% of scores will be on either side. Sometimes the center is the middle of a histogram - but not always: In a perfectly symmetric or nearly symmetric distribution, the center is basically the score (or interval) that is at the middle of the histogram In skewed distributions, the center point moves in the direction of the highest bars. Symmetric: the center point is the middle of the histogram, this is also the most frequently occurring interval and would come close to the average. Right skewed:The center (50% split of scores) is towards the end with the most scores (left in this example/low scores) Left skewed:The center (50% split of scores) is towards the end with the most scores (right in this example/low scores) OUTLIERS An outlier is any score or individuals response that falls outside of the overall pattern of a histogram. An outlier may be caused by human error (researcher jotting down the wrong number), a false report by a participant or a true value that indicates a new, interesting phenomenon. Because outliers affect many descriptive statistics (negatively), we must evaluate them and possibly remove them if they are present. Note: Not every value that is ‘apart’ is an outlier. There are mathematical ways to determine if a value is a true outlier. These calculations will not be taught in this course - any outlier that you need to determine on a test will be obvious. LAST YEARS FINAL EXAM! SPREAD, SHAPE, OUTLIER, CENTER? COMPARING DISTRIBUTIONS What do these histograms reveal about height in men vs women? end 1 Lecture 3: Describing Distributions with Numbers What is a summary 2 statistic? Summary or descriptive statistics help us to understand large amounts of data at a glance A ‘descriptive statistic’ is a number that summarizes one variable. Imagine having thousands of data points on thousands of people. For example: How much 120 000 Canadians spend a year on ‘non essential goods’. The type of car owned by all 16-21 year olds in Montreal We collect data to answer questions – a descriptive statistic is a good first place to start: What is the average amount (mean) Canadians spend a year on non essential good? What is the most popular (mode) car owned by 16-21 yr olds? The answer to these questions is a descriptive statistic! Remember this? 3 Average (Mean) IQ’s of 112 QM classes across 8 regional CEGEPs An average value (or mean) was calculated for each CEGEP – this value was used to plot the bar graph. The mean is an example of a common descriptive statistics. 2 categories of descriptives: Measures of Central Tendency and Spread 4 Descriptive measures tend to fall into two categories : measures of central tendency (e.g. ‘an average’) and measures of spread (variability) 1. A measure of central tendency is a single value that describes a distribution by identifying the number that occupies or represents the central position within the data. Three common measures of CT include: Mode Mean Median 2. Measures of spread ask the question: How spread out are the individual scores in the raw data? Two common measures of spread include: Standard deviation (accompanies the mean) Quartiles (accompany the median) 3 Measures of central 5 tendency When describing a variable in a study with a measure of CT, we generally choose among the following: 1. Mode: the most frequently occurring score (s) or response (s) in a set of values/numbers. 2. Mean: the midpoint of a Which measure of CT is best with distribution of numbers that each variable in the table takes into account all the values of the individual scores above? That decision requires an 3. Median: the middle ‘score’ or understanding of the strength midpoint of a series of values and weaknesses of each when they are ordered from measure of CT. least to greatest. The median is the score that splits the distribution in two equal halves. Examples: when to use the Mode and how to calculate it 6 The mode is reserved for To calculate the mode, we simply nominal variables – but is locate the most frequently sometimes also used for occurring response or score: ordinal variables Previous Schooling: Mode = In this table, which is/are ‘Public’ (occurs the most) the nominal variable (s)? Biological Sex: Mode = ‘1’ or Previous Schooling ‘Male’ (occurs the most) Biological Sex It is possible to have 2 modes Examples: when to use the 7 Mean and how to calculate it The mean is reserved for To calculate the mean, we add up all interval/ratio variables the scores and divide by ‘n’ or the (with some limitations). ‘total # of values/participants’ In this table, which is/are the interval/ratio variable Formula for the (s)? mean: Exercise per week (hrs) Mean: 3 + 10 + 0 + 5 + 6 + 3 + 1/7 = 4hrs Examples: when to use the Median 8 and how to calculate it The median is reserved for To calculate the median, we line up ordinal variables but all the scores or values in order from sometimes is used in other least to greatest. circumstances. We then find the ‘middle number’ In this table, which is/are or the number that cuts the ordered the ordinal variable (s)? distribution in half. Well-being See next slide for demo Final Letter Grade in Math Examples: Median 9 Calculate the Median of the ‘Well-being’ variable: 1. Place all the responses in order from least to greatest 2, 3, 4, 4, 4, 4, 5 2. Find the ‘middle number’ that splits the series in two equal halves. That is the Median! 2, 3, 4, 4, 4, 4, 5 ANS: Median = 4 Examples: Median 10 Median (even number of values): Participants were asked to rate their appreciation of College life on a scale from 1 to 10.Calculate the median rating. 1. Place all the numbers in order from least to greatest Ratings: 8, 10, 10, 4, 5, 7, 7, 9 In order: 4, 5, 7, 7, 8, 9, 10, 10 2. Notice this time there are 2 middle scores. In the case of ‘two middle numbers’, add up the two numbers that split the list in two equal halves and divide by 2. 4, 5, 7, 7, 8, 9, 10, 10 Notice that the 7 + 8 = 15 median is not always an actual 15/2= 7.5 score in the original data set ANS: Median = 7.5 (7.5 was not a rating option)! Sometimes the data is 11 ordinal but the mode makes more sense 8 participants reported their level of reading ability: Responses: beginner, beginner, beginner, beginner, moderate, moderate, strong, strong, To calculate a median, we would need to add up the two middle responses (beginner + moderate) and divide by 2! In this case, it makes more sense to just report the mode (mode=beginner). Which measure of Central 12 Tendency is best? Knowing which measure of central tendency to use on a given data set depends on a few factors including: the scale of measurement of the variable in question characteristics of the data set (outliers or not?) as well as … the shape of the distribution when plotted (e.g. in a histogram) There are pros and cons to each measure of central tendency; this next activity highlights some of these. In-class Activity 13 Below are the incomes of 7 randomly chosen people in a coffee shop (in thousands of dollars). 25K 15k 25k 45k 25k 32k 400k 1. What is the variable? 2. What is the scale of measurement of this variable? 3. Which is the most appropriate measure of central tendency to use/report given the scale of measurement? 4. Calculate the mean, median and mode of this distribution of incomes. Do you notice anything about the 3 calculations? Can you explain the discrepancy? 5. What do you think is the better measure of central tendency given the actual data set? Median, Mean & Extreme 14 Scores/Outliers One thing you might have noticed from the previous demonstration is that the median is not influenced by ‘outliers’ (salary of 400k); the mean however is. It is not enough to simply know a variables scale of measurement when deciding on which measure of central tendency is best to use. Factors such as presence of outliers should be taken into account, In the previous example, the median was the better measure of CT despite salary being a quantitative variable! There are other reasons we might choose the median over the mean – a skewed histogram (left or right) is basically a situation with many outliers. Skewed distributions are generally best described by the median as well. In general, we tend to only use the mean when the data set contains no outliers and the variable we are trying to describe produces a symmetric histogram. The effect of ‘skew’ on median, mean and mode 15 The mean, in a skewed distribution, tends to be dragged out to the tails. The median is less affected by skew and therefore gives a better representation of the true ‘average’ of a skewed distribution Distributions of income report the median income rather than the mean income for this reason The mean would be heavily influenced by the few millionaires and not provide a good representation of the majority Outliers (another example) 16 If your data set has big outliers, the median is the better measure of central tendency. The mean is greatly affected by outliers. The median, on the other hand, is not! Data 1: 5, 8, 13, 19, 21 Data 2: 5, 8, 13, 19, 2000 Mean = 13.2 Median = 13 Median = 13 Mean = 409 The mean and median The mean and median converge here differ greatly here indicating symmetry in indicating the presence the data set of skew or outliers Measures of spread/variability: Using both a measure of ‘CT’ and ‘Spread’ for 17 a complete picture ❖ When summarizing a variable using CT, we usually also provide a measure of spread or variability. Both descriptives combined give a more complete understanding of the variable in question. ❖ Consider this example: Take two QM classes that historically are taught by two different teachers. Every year the final class average is the exact same in both classes, but there is a very different degree of ‘spread’ or ‘variability’ around that average value. Average: 75% Spread: 72%-78% Spread: 60%-99% Which class would you rather be in??? The 5 number summary 18 (Median + Quartiles) One of the simplest ways to describe the center and spread of a distribution of numbers is to identify it’s 5 number summary. The 5 number summary can be used for interval ratio or ordinal variables – and is the preferred combo when there are outliers in a data set (or it is skewed). The 5 number summary ‘summarizes’ a distribution of scores/responses using ‘5’ key numbers: Lowest score (Minimum) 1st Quartile: Q1 The Median or 2nd Quartile: Q2 3rd Quartile: Q3 Highest score (Maximum) Think of a chocolate bar :) 19 These 5 numbers ‘cut’ up an ordered Q2 (Median) distribution of 4 numbers into ‘4 equal 3 25% parts’, with each part 2 25% containing 25% of all 1 25% the (chocolate) or 25% Q3 data 50% of scores (or the Q1 chocolate bar!) are below Q2; 25% of scores below Q1 etc etc Working with a 5 number 20 summary Below are the IQs of students in a 1st year CEGEP QM class (n=112) (raw data) along with the 5 number summary. What percent of students have an IQ that is 116 or higher? What percent of students have an IQ at the median or lower?? Have an IQ greater than Q3? Min=50 Q1= 116 Q2=125 Q3=138 Max=158 Calculating a 5-number 21 summary Data set: Amount of hours spent a month on online dating sites by 7 Vanier students: Student data: 65 15 35 55 5 25 45 Step 1: place the data set in order from smallest to largest Step 1 : 5 15 25 35 45 55 65 Calculating a 5-number 22 summary Step 2: Locate the Median also known as Quartile 2 (Q2) Step 2 : 5 15 25 35 45 55 65 Q2 Step 3 & 4: Locate Q1. To do this, find the ‘median’ of the numbers to the left of Q2 – the true median (and excluding it). Same for Q3. Step 3 & 4 : 5 15 25 35 45 55 65 Q1 Q2 Q3 Calculating a 5-number summary 23 Select the smallest number (the Min) and the largest number (the Max), to complete the 5 Number Summary Min Max Step 3 & 4 : 5 15 25 35 45 55 65 Q1 Q2 Q3 Report the 5 number summary: Min=5 Q1=15 Q2=35 Q3=55 Max=65 Another example 24 Find the 5 number summary of the following 8 numbers. Data set: 7 13 15 24 26 29 32 35 Step 1: place the numbers in order from least to greatest. Step 2: Locate the Median also known as Quartile 2 (Q2) Step 2 : 7 13 15 24 26 29 32 35 Q2 Median= 24+26/2 Median (Q2) = 25 Another example 25 Step 3 & 4: Locate Q1 and Q3 (find the median of the numbers to the right and left of the true median for Q1 and Q3 calculations. 25 Step 2 : 7 13 15 24 26 29 32 35 Q2 Q1=(13+15)/2 Q3=(29+32)/2 Q1=14 Q3=30.5 26 Report the 5 number summary: 5 number summary Min=7 Q1= 14 Median = 25 Q3=30.5 Max=35 In-class activity 27 Find the 5 number summary of the following scores: Example 1: 13, 29, 36, 4, 9, 18, 99 Example 2: 10 , 13, 23, 16, 50, 14, 32, 6 Working with a 5 number 28 summary Below are the IQs of a 1st year CEGEP QM class (raw data) along with the 5 number summary. Using the 5 number summary, answer the following questions: What percent of students have an IQ that is 116 or higher? What percent of students have an IQ at the median or lower?? Have an IQ greater than Q3? Min=50 Q1= 116 Q2=125 Q3=138 Max=158 5 number summary 29 IQ=125 IQ=50 IQ=116 Median IQ=138 IQ=158 Q1 (Q2) Q3 Max Min 25% 25% 25% 25% (25th (50th (75th percentile) percentile) percentile) Percentiles 30 A percentile is a number/value below which a certain percent of observations fall Example: If you are in the 75th percentile, then 75 percent of ʻscoresʼ are equal to or lower than yours (alternately: 25% are higher). A score at Q3 would be in the 75th percentile ….50th percentile: 50% of observation/scores are equal to or lower than yours (50% are higher) and so on A score at the median is in the 50th percentile Working with 5 number summary 31 2500 Vanier College students were asked how often they access their 1. What percentage of students access their email accounts a day. Use the email between 6 and 14 times a day? following 5 number summary to answer questions regarding email 2. If you access your email 6 times a day or usage in students. less, what percentile are you in? Min=1 3. What percentage of students in this distribution access their email 19 times or Q1=6 less a day? Median=14 4. What percent of students access their email Q3=19 less than the ‘median’ of the distribution? Max=26 5. What percentage of students access their email more than Q3. 6. If you are at the 75th percentile, how many times a day do you access your email? 7. If you are in the top 25% of students who access their email, how often do you access your email? What percentile is this? Email usage per day 32 14 1 6 Median 19 26 Q1 (Q2) Q3 Max Min 25% 25% 25% 25% (25th (50th (75th percentile) percentile) percentile) BOXPLOTS 33 5 number summaries are easily displayed in a type of graph called a Boxplot The y axis represents the values of the 5 number summary – which are based on scores from the variable in question Max Boxplots are best used when Q3 we want a side by side Q2 comparison of 2 or more Q1 different categories The boxplots on the right are Min comparing the different scores obtained by students depending on the teaching method used The x axis represents the different categories of the variable being graphed Making a Boxplot: 34 Blackboard Demo Two groups of students (Science vs Social Science) provide information on the amount of time (in hours) they spend being physically active in a month (i.e. doing sports, exercise, biking, walking etc). Plot their 5 numbers summaries in two separate boxplots ( side-by-side). Group 1=Science Group 2=Social Science Min=0 Min=15 Q1=15 Q1=20 Median=25 Median=25 Q3=35 Q3=50 Max=40 Max=65 In a vertical boxplot, the Y axis contains the values representing the 5 number summaries of the variable in question. The X axis represents the groups or categories you are comparing on this 35 variable. Make sure your y axis contains equal intervals between ticks and will represent both groups 5 number summaries. 70 Hours of physical activity 60 50 40 30 What can we 20 conclude 10 from these boxplots? Science Social Science BOXPLOTS: Skew and Spread 36 A boxplot can quickly reveal the shape of an underlying distribution and the variability in that distribution Perfectly symmetrical box plot = a symmetrical histogram = few low scores, few high scores and many people in the middle. Box plot with a median being pulled to one side or another = skewed histogram = many low (right skewed) or many high scores (left skewed) Short vs long box (and length of whiskers) = small variability/spread vs large variability/spread = small vs big difference between smallest and biggest score (see next slide) 37 What’s the best teaching method and why? Method 1 and 2 have nearly identical medians, but Method 1 has somewhat more variability (symmetrical). Method 3 has the highest variability in scores (long whiskers!) and is potentially left-skewed. Method 4 has the highest median, low variability and seems to have best outcomes overall. Mean and Standard 38 Deviation (stdev) The mean and standard deviation are the two most common ways to describe values whose underlying distributions/histograms are symmetric or roughly symmetric and whose underlying scale of measurement is interval/ratio (height, speed, temperature etc) No skew & No Outliers = Mean & Stdev ****** When a distribution is not symmetric or there are outliers, the mean & standard deviation may not be the best choice***** Remember this data set? 39 IQs of a CEGEP class We can also get the mean and standard deviation of this data set: Min=50 Q1= 116 Mean=101 Q2=125 Stdev=18.8 Q3=138 Max=158 Notice the mean and median are different? Why? What does it tell us about this data set? What’s the better measure of CT to use? Standard Deviation (and variance) 40 Definition: a number that describes how spread out scores are from the mean, on average. Or, more simply: “the average distance of scores from the mean” Low standard deviation means scores in a data set are close to the mean (on both sides) High standard deviation means scores in a data set are spread out over a larger range of values, further from the mean (on both sides) No standard deviation (‘0’): all scores are the same and equal to the mean - there is no spread at all. Low and high variability 41 (stdev) Notice that the histograms on the right center on the same Same mean but different standard deviation value of ’50’, but the spread/stdev of values is notably different. The standard deviation determines how fat or narrow a histogram is (the mean just determines where the peak of the histogram is on the x- axis) The mean does not tell the entire story! At a glance, the Example Example difference is evident in the Mean=50% Mean=50% histograms. Stdev=10% Stdev=35% Units of Standard 42 Deviation A standard deviation is always in the same units as the original variable: Imagine 50 men provided their heights. The average height is 6ft and the standard deviation is ‘1.5’. The 1.5 has a unit of ‘feet’. It is telling us that the spread of all heights is about 1.5 feet (on average) around the mean. Similarly, your first QM Test will produce an average score in % terms. Lets imagine the average is 80% for the 1 st test, with stdev of 10. The unit of the ’10’ is %. This is telling us that students marks deviated, on average, about 10 percentage points from the mean (above and below). How to compute STDEV 43 To compute a standard deviation we will use the following formula: Formula Defined 44 s = symbol for ʻstandard deviationʼ X = raw score from data set X’bar’ = the Mean n = number of scores in data set = ʻSum Ofʼ The 3 Column Method 45 Compute the VARIANCE (s2) AND STDEV (s) of the following numbers using the 3 column method: Data values: 4, 7, 10, 11 Mean = 8 3 column method 46 x x-mean (x-mean) 2 4 4-8 = -4 -42= 16 7 7-8= -1 -12= 1 10 10-8= 2 22 = 4 11 11-8 = 3 32 = 9 Sum = 30 Step 2: s2= 30/3 = 10 (variance) Step 3: s = √10 = 3.16 (stdev) In class activity: Compute 47 the Mean, Variance and STDEV Data values: 5, 4, 10, 8 a) stdev=2.75 b) Variance=7.56 Understanding the mean