Lec08 Analysis of Variance (ANOVA) & Tukey-Kramer Procedure PDF
Document Details
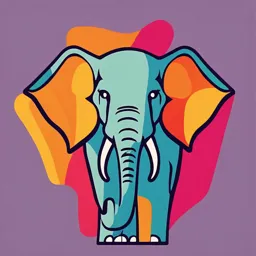
Uploaded by HandsomeRomanesque5388
Naveen Jindal School of Management, The University of Texas at Dallas
Rasoul Ramezani
Tags
Summary
This document is a lecture on advanced statistics for data science covering analysis of variance (ANOVA) and Tukey-Kramer procedures. It explores statistical techniques for comparing means across multiple groups and includes practical examples.
Full Transcript
Naveen Jindal School of Management The University of Texas at Dallas BUAN/ OPRE 6359 Advanced Statistics for Data Science Analysis of Variance (ANOVA) & Tukey-Kramer...
Naveen Jindal School of Management The University of Texas at Dallas BUAN/ OPRE 6359 Advanced Statistics for Data Science Analysis of Variance (ANOVA) & Tukey-Kramer Procedure Rasoul Ramezani The University of Texas at Dallas Jindal School of Management Naveen Jindal School of Management The University of Texas at Dallas Lecture Outline Analysis of Variance (ANOVA) – Testing the equality of several means using the F-Test Simultaneous pairwise comparisons of population means – Tukey-Kramer Procedure – Dunnet’s Procedure – Bonferroni Adjustment to LSD Method (FYI) Analysis of Variance (ANOVA) & Tukey-Kramer Procedure Rasoul Ramezani BUAN/OPRE 6359 Naveen Jindal School of Management The University of Texas at Dallas An Example: Investment in Stocks Does the investment in the stock market differ among different age groups? A financial analyst randomly sampled 366 American households and asked each about: 1. The age of the head of the household 2. The proportion of their financial assets invested in stocks. Analysis of Variance (ANOVA) & Tukey-Kramer Procedure Rasoul Ramezani BUAN/OPRE 6359 Naveen Jindal School of Management The University of Texas at Dallas Analysis of Variance (ANOVA) ANOVA is used to test the equality of more than two samples’ mean. Let: 𝐾 = The number of samples (groups) 𝑌 = % of financial assets invested in stocks The null and alternative hypotheses are: 𝐻0 : 𝜇1 = 𝜇2 = ⋯ = 𝜇𝐾 𝐻1 : At least one mean is different ANOVA assesses the mean differences by comparing the variation in 𝑌 from two sources: 1. Between-Group Variation: Differences in 𝑌 because of differences in ages. 2. Within-Group Variation: Differences in 𝑌 because of other relevant factors (such as household income, household size, marital status, race, education, etc.). Analysis of Variance (ANOVA) & Tukey-Kramer Procedure Rasoul Ramezani BUAN/OPRE 6359 Naveen Jindal School of Management The University of Texas at Dallas Steps of Conducting ANOVA Step 1: Calculate SSB & MSB 𝑆𝑆𝐵 = Sum of squares due to between-group variation 𝑀𝑆𝐵 = Mean square due to between-group variation Step 2: Calculate SSW & MSW 𝑆𝑆𝑊 = Sum of squares due to within-group variation 𝑀𝑆𝑊 = Mean square due to within-group variation Step 3: Conclude the Test Calculate F-ratio Calculate p-value Analysis of Variance (ANOVA) & Tukey-Kramer Procedure Rasoul Ramezani BUAN/OPRE 6359 Naveen Jindal School of Management The University of Texas at Dallas Step 1: SSB & MSB Identify the number of groups and Calculate the SSB: the size of each group: 2 𝑆𝑆𝐵 = ∑𝑛𝑖 𝑌ത𝑖 − 𝑌ത 𝐾 = 4, 𝑛1 = 84, 𝑛2 = 131, 𝑛3 = 93, 𝑛4 = 58 𝑆𝑆𝐵 = 84 44.3983 − 50.18 2 + ⋯ + 58 51.8381 − 50.18 2 = 3741.3636 Calculate the sample average for each group: Calculate MSB: 𝑌ത1 = 44.3983, 𝑌ത2 = 52.4724, 𝑀𝑆𝐵 = 𝑆𝑆𝐵 𝐾−1 𝑌ത3 = 51.1390, 𝑌ത4 = 51.8381 3741.3636 𝑀𝑆𝐵 = = 1247.1212 4−1 Calculate the overall sample ത average (called grand average), 𝑌: 𝑌ത = 50.18 Analysis of Variance (ANOVA) & Tukey-Kramer Procedure Rasoul Ramezani BUAN/OPRE 6359 Naveen Jindal School of Management The University of Texas at Dallas Step 2: SSW & MSW Calculate the sample variance (𝑠 2 ) for each group: 𝑠12 = 386.5464, 𝑠22 = 469.4371, 𝑠32 = 471.8239, 𝑠42 = 444.7895 Calculate SSW: 𝑆𝑆𝑊 = ∑ 𝑛𝑖 − 1 𝑠𝑖2 𝑆𝑆𝑊 = 84 − 1 386.5464 + ⋯ + 58 − 1 444.7895 = 161870.98 Note: Also, 𝑆𝑆𝑊 = 𝑛 − 𝐾 𝑆𝑝2 – Where: 2 𝑛1 −1 𝑠12 +⋯+ 𝑛𝐾 −1 𝑠𝐾 𝑆𝑝 = & 𝑛 = ∑ 𝑛𝑖 𝑛−𝐾 Calculate MSW: 𝑆𝑆𝑊 161870.98 𝑀𝑆𝑊 = = = 447.1574 𝑛−𝐾 366−4 Analysis of Variance (ANOVA) & Tukey-Kramer Procedure Rasoul Ramezani BUAN/OPRE 6359 Naveen Jindal School of Management The University of Texas at Dallas Step 3: Conclude the Test Calculate F-ratio: 𝑀𝑆𝐵 1247.1212 𝐹-ratio = = = 2.7890 𝑀𝑆𝑊 447.1574 Calculate p-value: 𝑝-value = 𝑝𝑓(F-ratio, 𝐾 − 1, 𝑛 − 𝐾, 𝑙𝑜𝑤𝑒𝑟. 𝑡𝑎𝑖𝑙 = 𝐹) 𝑝-value = 𝑝𝑓(2.789, 3,362) = 0.0405 Reject 𝐻0 if 𝑝-value ≤ 𝛼. Otherwise, do not reject 𝐻0. – Since p-value <.05, 𝐻0 is rejected, implying that the percentage of financial assets invested in stocks Command Approach for at least one age group is significantly different from other groups. Analysis of Variance (ANOVA) & Tukey-Kramer Procedure Rasoul Ramezani BUAN/OPRE 6359 Naveen Jindal School of Management The University of Texas at Dallas ANOVA Table Sum of Degrees of Mean Source of Variation Squares Freedom Squares F-ratio p-value 𝑆𝑆𝐵 𝑀𝑆𝐵 Between Group 𝑆𝑆𝐵 𝐾−1 𝑀𝑆𝐵 = 𝑃(𝐹 > 𝐹 -ratio) 𝐾 − 1 𝑀𝑆𝑊 𝑆𝑆𝑊 Within Group 𝑆𝑆𝑊 𝑛−𝐾 𝑀𝑆𝑊 = 𝑛−𝐾 Total 𝑆𝑆𝐵 + 𝑆𝑆𝑊 𝑛−1 Source of Variation SS df MS F-ratio p-value Between Group 3741.36 3 1247.12 2.79 0.0405 Within Group 161870.98 362 447.16 Total 165612.3 365 Analysis of Variance (ANOVA) & Tukey-Kramer Procedure Rasoul Ramezani BUAN/OPRE 6359 Naveen Jindal School of Management The University of Texas at Dallas Simultaneous Inferences Individual confidence level: The probability that a single CI covers the true parameter. – E.g., In a 95% CI, the individual success rate = 95% Familywise confidence level: The probability that all confidence intervals simultaneously cover the true parameter. – It could be shown that if the family consists of 𝑘 CIs, then: 100 1 − 0.05𝑘 % < success rate < 95% – E.g., with 𝑘 = 5, 75% < success rate < 95% Analysis of Variance (ANOVA) & Tukey-Kramer Procedure Rasoul Ramezani BUAN/OPRE 6359 Naveen Jindal School of Management The University of Texas at Dallas Multiple-comparison Procedures Multiple-comparison procedures have been developed to control the familywise confidence level (at, for example, 95%). The CI for 𝜇𝑖 − 𝜇𝑗 : (𝑌ത𝑖 −𝑌ത𝑗 ) ± 𝑀𝑢𝑙𝑡𝑖𝑝𝑙𝑖𝑒𝑟 × 𝑆𝐸(𝑌ത𝑖 −𝑌ത𝑗 ) for all 𝑖 ≠ 𝑗 Interval half-width (margin of error) Two procedures in estimating the multiplier: – Tukey–Kramer Test – Dunnett’s Procedure Analysis of Variance (ANOVA) & Tukey-Kramer Procedure Rasoul Ramezani BUAN/OPRE 6359 Naveen Jindal School of Management The University of Texas at Dallas Tukey-Kramer Test Testing ALL pairwise comparisons Steps 2: Calculate 𝑆𝐸 𝑌ത𝑖 − 𝑌ത𝑗 : simultaneously. 𝑠𝑒𝑖𝑗 = 𝑆𝑝 1/𝑛𝑖 + 1/𝑛𝑗 𝐻0 : 𝜇1 = 𝜇2 vs. 𝐻1 : 𝜇1 ≠ 𝜇2 𝐻0 : 𝜇1 = 𝜇3 vs. 𝐻1 : 𝜇1 ≠ 𝜇3 Step 3: Using R, calculate the critical value: ⋮ 𝑞𝑐 = 𝑞𝑡𝑢𝑘𝑒𝑦(1 − 𝛼, 𝐾, 𝑛 − 𝐾) 𝐻0 : 𝜇𝐾−1 = 𝜇𝐾 vs. 𝐻1 : 𝜇𝐾−1 ≠ 𝜇𝐾 Step 4: Calculate the margin of error: This procedure uses studentized range 𝑞𝑐 distribution (q). 𝑚𝑒 = ⋅ 𝑠𝑒𝑖𝑗 2 Assuming normality and equal 𝜎’s, steps Step 5: Construct the CI for (𝜇𝑖 − 𝜇𝑗 ): for testing 𝐻0 : 𝜇𝑖 = 𝜇𝑗 vs. 𝐻1 : 𝜇𝑖 ≠ 𝜇𝑗 : (𝑌ത𝑖 −𝑌ത𝑗 ) ± 𝑚𝑒 Step 1: Calculate pooled SD: Step 6: Conclude the test. If the CI includes ∑𝑖 𝑛𝑖 −1 𝑆𝑖2 zero, 𝐻0 is not rejected at 𝛼 level and thus, 𝑆𝑝 = 𝑛−𝐾 𝜇𝑖 = 𝜇𝑗. Otherwise 𝜇𝑖 ≠ 𝜇𝑗. Analysis of Variance (ANOVA) & Tukey-Kramer Procedure Rasoul Ramezani BUAN/OPRE 6359 Naveen Jindal School of Management * The University of Texas at Dallas Tukey-Kramer Test For example, comparing Young (1) vs. Senior (4) at 𝛼 = 0.05: 𝐻0 : 𝜇1 = 𝜇4 vs. 𝐻1 : 𝜇1 ≠ 𝜇4 84−1 19.662 + 131−1 21.672 + 93−1 21.722 + 58−1 21.092 1. 𝑆𝑝 = 366−4 = 21.1461 1 1 2. 𝑠𝑒14 = 21.1462 84 + 58 = 3.6101 3. 𝑞𝑐 = qtukey.95,4,362 = 3.6501 3.6501 4. 𝑚𝑒 = 2 (3.6101) = 9.3177 5. The 95% CI for 𝜇1 − 𝜇4 : 44.3983 − 51.8381 ± 9.3177 = (−16.76,1.88) 6. Since the 95% CI for 𝜇1 − 𝜇4 includes 0, thus 𝐻0 : 𝜇1 = 𝜇4 cannot be rejected. This process should be repeated for other five pairwise comparisons. Analysis of Variance (ANOVA) & Tukey-Kramer Procedure Rasoul Ramezani BUAN/OPRE 6359 Naveen Jindal School of Management The University of Texas at Dallas Tukey-Kramer Test: Command Approach Recall: ANOVA test for equality of all means: 𝐻0 : 𝜇1 = 𝜇2 = 𝜇3 = 𝜇4 𝐻0 rejected ⇒ At least Family pairwise comparisons: one 𝜇 is different. 𝐻0 : 𝜇1 = 𝜇2 , 𝜇1 = 𝜇3 , 𝜇2 = 𝜇3 , … p-adj = 𝑃 𝑞𝐼,𝑛−𝐼 > 𝑞𝑠𝑡𝑎𝑡 = 𝑝𝑡𝑢𝑘𝑒𝑦(𝑞𝑠𝑡𝑎𝑡 , 𝐾, 𝑛 − 𝐾, 𝑙𝑜𝑤𝑒𝑟. 𝑡𝑎𝑖𝑙 = 𝐹) 𝑌ത 𝑖 −𝑌ത 𝑗 Where 𝑞𝑠𝑡𝑎𝑡 = 𝑆𝐸(𝑌ത 𝑖 −𝑌ത 𝑗 )/√2 Analysis of Variance (ANOVA) & Tukey-Kramer Procedure Rasoul Ramezani BUAN/OPRE 6359 Naveen Jindal School of Management The University of Texas at Dallas Dunnet’s Procedure This multiple-comparison procedure compares every other group to a reference group. This test incorporates correlation between observations in the analysis, using a multivariate t- distribution. – CIs are narrower under Dunnet’s procedure than those under the Tukey-Kramer procedure. Analysis of Variance (ANOVA) & Tukey-Kramer Procedure Rasoul Ramezani BUAN/OPRE 6359 Naveen Jindal School of Management The University of Texas at Dallas Bonferroni Adjustment to LSD Method (FYI) In a multiple comparison problem with 𝐾 E.g., with 𝐾 = 4, if we want the probability of groups, there are 𝐶 = 𝐾(𝐾 − 1)/ making at least one type-I error to be no 2 pairwise tests. more than 0.05, we should set 𝛼 =.05/6 = The probability of at least one type-I error 0.0083. (i.e., rejecting a TRUE 𝐻0 ) is: This is called the modified significance level, 𝛼𝐸 = 1 − 1 − 𝛼 𝐶 and the resulting procedure called Bonferroni Adjustment. – Hence, it is very likely to reject at least one TRUE 𝐻0. It could be shown that 𝛼𝐸 ≤ 𝛼𝐶. Thus, if we want the probability of making at least one type-I error to be no more than 𝛼𝐸 , we should select 𝛼 such that: 𝛼 = 𝐶 Analysis of Variance (ANOVA) & Tukey-Kramer Procedure Rasoul Ramezani BUAN/OPRE 6359 Naveen Jindal School of Management The University of Texas at Dallas This is the last slide End of Lecture 8 Analysis of Variance (ANOVA) & Tukey-Kramer Procedure Rasoul Ramezani BUAN/OPRE 6359