Index of Applications LA Larson PDF
Document Details
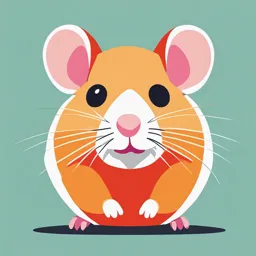
Uploaded by MeritoriousNitrogen
COEP Technological University
LA Larson
Tags
Related
- Rosen Discrete Mathematics and Its Applications 7th Edition PDF
- Kenneth Rosen - Discrete Mathematics and Its Applications - 8th edition.pdf
- The Nature of Mathematics PDF
- ken-rosen-discrete-mathematics-and-its-applications.pdf
- AVO_Module1-GE4-MMW Mathematics in Modern World PDF
- Discrete Mathematics with Applications (5th Edition) PDF
Summary
This document is an index of applications, encompassing various fields like biology, life sciences, mathematics, engineering, and business economics. It outlines the diverse range of subjects and real-world contexts where mathematical models and concepts are used.
Full Transcript
INDEX OF APPLICATIONS BIOLOGY AND LIFE SCIENCES Petroleum production, 292 MATHEMATICS AND GEOMETRY Age distribution vector, 378, 391, 392, 395 Profit,...
INDEX OF APPLICATIONS BIOLOGY AND LIFE SCIENCES Petroleum production, 292 MATHEMATICS AND GEOMETRY Age distribution vector, 378, 391, 392, 395 Profit, from crops, 50 Adjoint of a matrix, 134, 135, 142, 146, 150 Age progression software, 180 Purchase of a product, 91 Collinear points in the xy-plane, 139, 143 Age transition matrix, 378, 391, 392, 395 Revenue Conic section(s), 226, 229 Agriculture, 37, 50 fast-food stand, 242 general equation, 141 Cosmetic surgery results simulation, 180 General Dynamics Corporation, 266, 276 rotation of axes, 221–224, 226, 229, Duchenne muscular dystrophy, 365 Google, Inc., 291 383–385, 392, 395 Galloping speeds of animals, 276 telecommunications company, 242 Constrained optimization, 389, 390, 392, Genetics, 365 software publishers, 143 395 Health care expenditures, 146 Sales, 37 Contraction in R2, 337, 341, 342 Heart rhythm analysis, 255 concession area, 42 Coplanar points in space, 140, 143 Hemophilia A, 365 stocks, 92 Cramer’s Rule, 130, 136, 137, 142, 143, 146 Hereditary baldness, 365 Wal-Mart, 32 Cross product of two vectors, 277–280, Nutrition, 11 Sales promotion, 106 288, 289, 294 Population Satellite television service, 85, 86, 147 Differential equation(s) Software publishing, 143 linear, 218, 225, 226, 229 of deer, 37 second order, 164 of laboratory mice, 91 system of first order, 354, 380, 381, of rabbits, 379 ENGINEERING AND TECHNOLOGY 391, 392, 395, 396, 398 of sharks, 396 Aircraft design, 79 Expansion in R2, 337, 341, 342, 345 of small fish, 396 Circuit design, 322 Fibonacci sequence, 396 Population age and growth over time, 331 Computer graphics, 338 Fourier approximation(s), 285–287, 289, 292 Population genetics, 365 Computer monitors, 190 Geometry of linear transformations in R2, Population growth, 378, 379, 391, 392, 336–338, 341, 342, 345 Control system, 314 395, 396, 398 Hessian matrix, 375 Controllability matrix, 314 Predator-prey relationship, 396 Jacobian, 145 Cryptography, 94–96, 102, 107 Red-green color blindness, 365 Lagrange multiplier, 34 Data encryption, 94 Reproduction rates of deer, 103 Laplace transform, 130 Decoding a message, 96, 102, 107 Sex-linked inheritance, 365 Least squares approximation(s), 281–284, 289 Digital signal processing, 172 Spread of a virus, 91, 93 linear, 282, 289, 292 Electrical network analysis, 30, 31, 34, 37, Vitamin C content, 11 quadratic, 283, 289, 292 150 Wound healing simulation, 180 Linear programming, 47 Electronic equipment, 190 X-linked inheritance, 365 Magnification in R2, 341, 342 Encoding a message, 95, 102, 107 Encryption key, 94 Mathematical modeling, 273, 274, 276 BUSINESS AND ECONOMICS Parabola passing through three points, 150 Engineering and control, 130 Partial fraction decomposition, 34, 37 Airplane allocation, 91 Error checking Polynomial curve fitting, 25–28, 32, 34, 37 Borrowing money, 23 digit, 200 Quadratic form(s), 382–388, 392, 395, 398 Demand, for a rechargeable power drill, 103 matrix, 200 Quadric surface, rotation of, 388, 392 Demand matrix, external, 98 Feed horn, 223 Reflection in R2, 336, 341, 342, 345, 346 Economic system, 97, 98 Global Positioning System, 16 Relative maxima and minima, 375 of a small community, 103 Google’s Page Rank algorithm, 86 Rotation Finance, 23 Image morphing and warping, 180 in R2, 303, 343, 393, 397 Fundraising, 92 Information retrieval, 58 in R3, 339, 340, 342, 345 Gasoline sales, 105 Internet search engine, 58 Second Partials Test for relative extrema, 375 Industrial system, 102, 107 Ladder network, 322 Shear in R2, 337, 338, 341, 342, 345 Input-output matrix, 97 Locating lost vessels at sea, 16 Taylor polynomial of degree 1, 282 Leontief input-output model(s), 97, 98, 103 Movie special effects, 180 Three-point form of the equation of a plane, Major League Baseball salaries, 107 Network analysis, 29–34, 37 141, 143, 146 Manufacturing Radar, 172 Translation in R2, 308, 343 labor and material costs, 105 Sampling, 172 Triple scalar product, 288 models and prices, 150 Satellite dish, 223 Two-point form of the equation of a line, production levels, 51, 105 Smart phones, 190 139, 143, 146, 150 Net profit, Microsoft, 32 Televisions, 190 Unit circle, 253 Output matrix, 98 Wireless communications, 172 Wronskian, 219, 225, 226, 229 Copyright 2017 Cengage Learning. All Rights Reserved. May not be copied, scanned, or duplicated, in whole or in part. Due to electronic rights, some third party content may be suppressed from the eBook and/or eChapter(s). Editorial review has deemed that any suppressed content does not materially affect the overall learning experience. Cengage Learning reserves the right to remove additional content at any time if subsequent rights restrictions require it. PHYSICAL SCIENCES Newton’s Second Law of Motion, 164 Smokers and nonsmokers, 91 Acoustical noise levels, 28 Ohm’s Law, 322 Sports Airplane speed, 11 Pendulum, 225 activities, 91 Area Planetary periods, 27, 274 Super Bowl I, 36 of a parallelogram using cross product, Primary additive colors, 190 Television watching, 91 279, 280, 288, 294 RGB color model, 190 Test scores, 108 of a triangle Stiffness matrix, 64, 72 using cross product, 289 Temperature, 34 STATISTICS AND PROBABILITY using determinants, 138, 142, 146, Torque, 277 Traffic flow, 28, 33 Canonical regression analysis, 304 150 Least squares regression Astronomy, 27, 274 Undamped system, 164 Unit cell, 213 analysis, 99–101, 103, 107, 265, 271–276 Balancing a chemical equation, 4 cubic polynomial, 276 Beam deflection, 64, 72 end-centered monoclinic, 213 Vertical motion, 37 line, 100, 103, 107, 271, 274, 276, 296 Chemical quadratic polynomial, 273, 276 changing state, 91 Volume of a parallelepiped, 288, 289, 292 Leslie matrix, 331, 378 mixture, 37 Markov chain, 85, 86, 92, 93, 106 reaction, 4 of a tetrahedron, 114, 140, 143 Water flow, 33 absorbing, 89, 90, 92, 93, 106 Comet landing, 141 Multiple regression analysis, 304 Computational fluid dynamics, 79 Wind energy consumption, 103 Work, 248 Multivariate statistics, 304 Crystallography, 213 State matrix, 85, 106, 147, 331 Degree of freedom, 164 Steady state probability vector, 386 Diffusion, 354 Stochastic matrices, 84–86, 91–93, 106, 331 SOCIAL SCIENCES AND Dynamical systems, 396 Earthquake monitoring, 16 DEMOGRAPHICS Electric and magnetic flux, 240 Caribbean Cruise, 106 MISCELLANEOUS Flexibility matrix, 64, 72 Cellular phone subscribers, 107 Architecture, 388 Force Consumer preference model, 85, 86, 92, 147 Catedral Metropolitana Nossa Senhora matrix, 72 Final grades, 105 Aparecida, 388 to pull an object up a ramp, 157 Grade distribution, 92 Chess tournament, 93 Geophysics, 172 Master’s degrees awarded, 276 Classified documents, 106 Grayscale, 190 Politics, voting apportionment, 51 Determining directions, 16 Hooke’s Law, 64 Population Dominoes, A2 Kepler’s First Law of Planetary Motion, 141 of consumers, 91 Flight crew scheduling, 47 Kirchhoff’s Laws, 30, 322 regions of the United States, 51 Sudoku, 120 Lattice of a crystal, 213 of smokers and nonsmokers, 91 Tips, 23 Mass-spring system, 164, 167 United States, 32 U.S. Postal Service, 200 Mean distance from the sun, 27, 274 world, 273 ZIP + 4 barcode, 200 Natural frequency, 164 Population migration, 106 Copyright 2017 Cengage Learning. All Rights Reserved. May not be copied, scanned, or duplicated, in whole or in part. Due to electronic rights, some third party content may be suppressed from the eBook and/or eChapter(s). Editorial review has deemed that any suppressed content does not materially affect the overall learning experience. Cengage Learning reserves the right to remove additional content at any time if subsequent rights restrictions require it. Copyright 2017 Cengage Learning. All Rights Reserved. May not be copied, scanned, or duplicated, in whole or in part. Due to electronic rights, some third party content may be suppressed from the eBook and/or eChapter(s). Editorial review has deemed that any suppressed content does not materially affect the overall learning experience. Cengage Learning reserves the right to remove additional content at any time if subsequent rights restrictions require it. Elementary Linear Algebra Copyright 2017 Cengage Learning. All Rights Reserved. May not be copied, scanned, or duplicated, in whole or in part. Due to electronic rights, some third party content may be suppressed from the eBook and/or eChapter(s). Editorial review has deemed that any suppressed content does not materially affect the overall learning experience. Cengage Learning reserves the right to remove additional content at any time if subsequent rights restrictions require it. Copyright 2017 Cengage Learning. All Rights Reserved. May not be copied, scanned, or duplicated, in whole or in part. Due to electronic rights, some third party content may be suppressed from the eBook and/or eChapter(s). Editorial review has deemed that any suppressed content does not materially affect the overall learning experience. Cengage Learning reserves the right to remove additional content at any time if subsequent rights restrictions require it. Elementary Linear Algebra 8e Ron Larson The Pennsylvania State University The Behrend College Australia Brazil Mexico Singapore United Kingdom United States Copyright 2017 Cengage Learning. All Rights Reserved. May not be copied, scanned, or duplicated, in whole or in part. Due to electronic rights, some third party content may be suppressed from the eBook and/or eChapter(s). Editorial review has deemed that any suppressed content does not materially affect the overall learning experience. Cengage Learning reserves the right to remove additional content at any time if subsequent rights restrictions require it. This is an electronic version of the print textbook. Due to electronic rights restrictions, some third party content may be suppressed. Editorial review has deemed that any suppressed content does not materially affect the overall learning experience. The publisher reserves the right to remove content from this title at any time if subsequent rights restrictions require it. For valuable information on pricing, previous editions, changes to current editions, and alternate formats, please visit www.cengage.com/highered to search by ISBN#, author, title, or keyword for materials in your areas of interest. Important Notice: Media content referenced within the product description or the product text may not be available in the eBook version. Copyright 2017 Cengage Learning. All Rights Reserved. May not be copied, scanned, or duplicated, in whole or in part. Due to electronic rights, some third party content may be suppressed from the eBook and/or eChapter(s). Editorial review has deemed that any suppressed content does not materially affect the overall learning experience. Cengage Learning reserves the right to remove additional content at any time if subsequent rights restrictions require it. Elementary Linear Algebra © 2017, 2013, 2009 Cengage Learning Eighth Edition WCN: 02-200-203 Ron Larson Product Director: Terry Boyle ALL RIGHTS RESERVED. No part of this work covered by the copyright herein may be reproduced, transmitted, stored, or used in any form or by Product Manager: Richard Stratton any means graphic, electronic, or mechanical, including but not limited Content Developer: Spencer Arritt to photocopying, recording, scanning, digitizing, taping, Web distribution, Product Assistant: Kathryn Schrumpf information networks, or information storage and retrieval systems, Marketing Manager: Ana Albinson except as permitted under Section 107 or 108 of the 1976 United States Content Project Manager: Jennifer Risden Copyright Act, without the prior written permission of the publisher. Manufacturing Planner: Doug Bertke Production Service: Larson Texts, Inc. For product information and technology assistance, contact us at Cengage Learning Customer & Sales Support, 1-800-354-9706. Photo Researcher: Lumina Datamatics For permission to use material from this text or product, Text Researcher: Lumina Datamatics submit all requests online at www.cengage.com/permissions. Text Designer: Larson Texts, Inc. Further permissions questions can be e-mailed to Cover Designer: Larson Texts, Inc. [email protected]. Cover Image: Keo/Shutterstock.com Compositor: Larson Texts, Inc. Library of Congress Control Number: 2015944033 Student Edition ISBN: 978-1-305-65800-4 Loose-leaf Edition ISBN: 978-1-305-95320-8 Cengage Learning 20 Channel Center Street Boston, MA 02210 USA Cengage Learning is a leading provider of customized learning solutions with employees residing in nearly 40 different countries and sales in more than 125 countries around the world. Find your local representative at www.cengage.com. Cengage Learning products are represented in Canada by Nelson Education, Ltd. To learn more about Cengage Learning Solutions, visit www.cengage.com. Purchase any of our products at your local college store or at our preferred online store www.cengagebrain.com. Printed in the United States of America Print Number: 01 Print Year: 2015 Copyright 2017 Cengage Learning. All Rights Reserved. May not be copied, scanned, or duplicated, in whole or in part. Due to electronic rights, some third party content may be suppressed from the eBook and/or eChapter(s). Editorial review has deemed that any suppressed content does not materially affect the overall learning experience. Cengage Learning reserves the right to remove additional content at any time if subsequent rights restrictions require it. Contents 1 Systems of Linear Equations 1 1.1 Introduction to Systems of Linear Equations 2 1.2 Gaussian Elimination and Gauss-Jordan Elimination 13 1.3 Applications of Systems of Linear Equations 25 Review Exercises 35 Project 1 Graphing Linear Equations 38 Project 2 Underdetermined and Overdetermined Systems 38 2 Matrices 39 2.1 Operations with Matrices 40 2.2 Properties of Matrix Operations 52 2.3 The Inverse of a Matrix 62 2.4 Elementary Matrices 74 2.5 Markov Chains 84 2.6 More Applications of Matrix Operations 94 Review Exercises 104 Project 1 Exploring Matrix Multiplication 108 Project 2 Nilpotent Matrices 108 3 Determinants 109 3.1 The Determinant of a Matrix 110 3.2 Determinants and Elementary Operations 118 3.3 Properties of Determinants 126 3.4 Applications of Determinants 134 Review Exercises 144 Project 1 Stochastic Matrices 147 Project 2 The Cayley-Hamilton Theorem 147 Cumulative Test for Chapters 1–3 149 4 Vector Spaces 151 4.1 Vectors in Rn 152 4.2 Vector Spaces 161 4.3 Subspaces of Vector Spaces 168 4.4 Spanning Sets and Linear Independence 175 4.5 Basis and Dimension 186 4.6 Rank of a Matrix and Systems of Linear Equations 195 4.7 Coordinates and Change of Basis 208 4.8 Applications of Vector Spaces 218 Review Exercises 227 Project 1 Solutions of Linear Systems 230 Project 2 Direct Sum 230 v Copyright 2017 Cengage Learning. All Rights Reserved. May not be copied, scanned, or duplicated, in whole or in part. Due to electronic rights, some third party content may be suppressed from the eBook and/or eChapter(s). Editorial review has deemed that any suppressed content does not materially affect the overall learning experience. Cengage Learning reserves the right to remove additional content at any time if subsequent rights restrictions require it. vi Contents 5 Inner Product Spaces 231 5.1 Length and Dot Product in R n 232 5.2 Inner Product Spaces 243 5.3 Orthonormal Bases: Gram-Schmidt Process 254 5.4 Mathematical Models and Least Squares Analysis 265 5.5 Applications of Inner Product Spaces 277 Review Exercises 290 Project 1 The QR-Factorization 293 Project 2 Orthogonal Matrices and Change of Basis 294 Cumulative Test for Chapters 4 and 5 295 6 Linear Transformations 297 6.1 Introduction to Linear Transformations 298 6.2 The Kernel and Range of a Linear Transformation 309 6.3 Matrices for Linear Transformations 320 6.4 Transition Matrices and Similarity 330 6.5 Applications of Linear Transformations 336 Review Exercises 343 Project 1 Reflections in R 2 (I) 346 Project 2 Reflections in R 2 (II) 346 7 Eigenvalues and Eigenvectors 347 7.1 Eigenvalues and Eigenvectors 348 7.2 Diagonalization 359 7.3 Symmetric Matrices and Orthogonal Diagonalization 368 7.4 Applications of Eigenvalues and Eigenvectors 378 Review Exercises 393 Project 1 Population Growth and Dynamical Systems (I) 396 Project 2 The Fibonacci Sequence 396 Cumulative Test for Chapters 6 and 7 397 8 Complex Vector Spaces (online)* 8.1 Complex Numbers 8.2 Conjugates and Division of Complex Numbers 8.3 Polar Form and DeMoivre’s Theorem 8.4 Complex Vector Spaces and Inner Products 8.5 Unitary and Hermitian Matrices Review Exercises Project 1 The Mandelbrot Set Project 2 Population Growth and Dynamical Systems (II) Copyright 2017 Cengage Learning. All Rights Reserved. May not be copied, scanned, or duplicated, in whole or in part. Due to electronic rights, some third party content may be suppressed from the eBook and/or eChapter(s). Editorial review has deemed that any suppressed content does not materially affect the overall learning experience. Cengage Learning reserves the right to remove additional content at any time if subsequent rights restrictions require it. Contents vii 9 Linear Programming (online)* 9.1 Systems of Linear Inequalities 9.2 Linear Programming Involving Two Variables 9.3 The Simplex Method: Maximization 9.4 The Simplex Method: Minimization 9.5 The Simplex Method: Mixed Constraints Review Exercises Project 1 Beach Sand Replenishment (I) Project 2 Beach Sand Replenishment (II) 10 Numerical Methods (online)* 10.1 Gaussian Elimination with Partial Pivoting 10.2 Iterative Methods for Solving Linear Systems 10.3 Power Method for Approximating Eigenvalues 10.4 Applications of Numerical Methods Review Exercises Project 1 The Successive Over-Relaxation (SOR) Method Project 2 United States Population Appendix A1 Mathematical Induction and Other Forms of Proofs Answers to Odd-Numbered Exercises and Tests A7 Index A41 Technology Guide* *Available online at CengageBrain.com. Copyright 2017 Cengage Learning. All Rights Reserved. May not be copied, scanned, or duplicated, in whole or in part. Due to electronic rights, some third party content may be suppressed from the eBook and/or eChapter(s). Editorial review has deemed that any suppressed content does not materially affect the overall learning experience. Cengage Learning reserves the right to remove additional content at any time if subsequent rights restrictions require it. Copyright 2017 Cengage Learning. All Rights Reserved. May not be copied, scanned, or duplicated, in whole or in part. Due to electronic rights, some third party content may be suppressed from the eBook and/or eChapter(s). Editorial review has deemed that any suppressed content does not materially affect the overall learning experience. Cengage Learning reserves the right to remove additional content at any time if subsequent rights restrictions require it. Preface Welcome to Elementary Linear Algebra, Eighth Edition. I am proud to present to you this new edition. As with all editions, I have been able to incorporate many useful comments from you, our user. And while much has changed in this revision, you will still find what you expect—a pedagogically sound, mathematically precise, and comprehensive textbook. Additionally, I am pleased and excited to offer you something brand new— a companion website at LarsonLinearAlgebra.com. My goal for every edition of this textbook is to provide students with the tools that they need to master linear algebra. I hope you find that the changes in this edition, together with LarsonLinearAlgebra.com, will help accomplish just that. New To This Edition NEW LarsonLinearAlgebra.com This companion website offers multiple tools and resources to supplement your learning. Access to these features is free. Watch videos explaining concepts from the book, explore examples, download data sets and much more. 5.2 Exercises 253 True or False? In Exercises 85 and 86, determine 94. Use the result of Exercise 93 to find W⊥ when W is the whether each statement is true or false. If a statement span of (1, 2, 3) in V = R3. is true, give a reason or cite an appropriate statement 95. Guided Proof Let 〈u, v〉 be the Euclidean inner from the text. If a statement is false, provide an example product on Rn. Use the fact that 〈u, v〉 = uTv to prove that shows the statement is not true in all cases or cite an that for any n × n matrix A, appropriate statement from the text. (a) 〈ATAu, v〉 = 〈u, Av〉 85. (a) The dot product is the only inner product that can be and defined in Rn. (b) 〈ATAu, u〉 = Au2. (b) A nonzero vector in an inner product can have a norm of zero. Getting Started: To prove (a) and (b), make use of both 86. (a) The norm of the vector u is the angle between u and the positive x-axis. the properties of transposes (Theorem 2.6) and the properties of the dot product (Theorem 5.3). REVISED Exercise Sets (i) To prove part (a), make repeated use of the property (b) The angle θ between a vector v and the projection of u onto v is obtuse when the scalar a < 0 and 〈u, v〉 = uTv and Property 4 of Theorem 2.6. The exercise sets have been carefully and extensively acute when a > 0, where av = projvu. (ii) To prove part (b), make use of the property 〈u, v〉 = uTv, Property 4 of Theorem 2.6, and examined to ensure they are rigorous, relevant, and 87. Let u = (4, 2) and v = (2, −2) be vectors in R2 with Property 4 of Theorem 5.3. the inner product 〈u, v〉 = u1v1 + 2u2v2. cover all the topics necessary to understand the (a) Show that u and v are orthogonal. (b) Sketch u and v. Are they orthogonal in the Euclidean 96. CAPSTONE (a) Explain how to determine whether a function fundamentals of linear algebra. The exercises are sense? 88. Proof Prove that defines an inner product. (b) Let u and v be vectors in an inner product space V, ordered and titled so you can see the connections u + v2 + u − v2 = 2u2 + 2v2 for any vectors u and v in an inner product space V. such that v ≠ 0. Explain how to find the orthogonal projection of u onto v. between examples and exercises. Many new skill- 89. Proof Prove that the function is an inner product on Rn. 〈u, v〉 = c1u1v1 + c2u2v2 +... + cnunvn, ci > 0 building, challenging, and application exercises have Finding Inner Product Weights In Exercises 97–100, 90. Proof Let u and v be nonzero vectors in an inner product space V. Prove that u − projvu is orthogonal find c1 and c2 for the inner product of R2, been added. As in earlier editions, the following 〈u, v〉 = c1u1v1 + c2u2v2 to v. 91. Proof Prove Property 2 of Theorem 5.7: If u, v, such that the graph represents a unit circle as shown. pedagogically-proven types of exercises are included. 97. y 98. y and w are vectors in an inner product space V, then 3 4 〈u + v, w〉 = 〈u, w〉 + 〈v, w〉. 92. Proof Prove Property 3 of Theorem 5.7: If u and v 2 ||u|| = 1 ||u|| = 1 True or False Exercises 1 are vectors in an inner product space V and c is any real x x number, then 〈u, cv〉 = c〈u, v〉. −3 −2 2 3 −3 −1 1 3 Proofs 93. Guided Proof Let W be a subspace of the inner −2 product space V. Prove that the set W⊥ = { v ∈ V: 〈v, w〉 = 0 for all w ∈ W } 99. −3 y 100. −4 y Guided Proofs 5 6 is a subspace of V. Getting Started: To prove that W⊥ is a subspace of ||u|| = 1 4 ||u|| = 1 Writing Exercises V, you must show that W⊥ is nonempty and that the 1 x x closure conditions for a subspace hold (Theorem 4.5). −5 −3 1 3 5 −6 6 Technology Exercises (indicated throughout the (i) Find a vector in W⊥ to conclude that it is nonempty. −4 (ii) To show the closure of W⊥ under addition, you −5 −6 text with ) need to show that 〈v1 + v2, w〉 = 0 for all w ∈ W 101. Consider the vectors and for any v1, v2 ∈ W⊥. Use the properties of inner products and the fact that 〈v1, w〉 and 〈v2, w〉 are both zero to show this. u = (6, 2, 4) and v = (1, 2, 0) from Example 10. Without using Theorem 5.9, show Exercises utilizing electronic data sets are indicated (iii) To show closure under multiplication by a scalar, proceed as in part (ii). Use the properties of inner that among all the scalar multiples cv of the vector v, the projection of u onto v is the vector closest to by and found at CengageBrain.com. products and the condition of belonging to W⊥. u—that is, show that d(u, projvu) is a minimum. ix Copyright 2017 Cengage Learning. All Rights Reserved. May not be copied, scanned, or duplicated, in whole or in part. Due to electronic rights, some third party content may be suppressed from the eBook and/or eChapter(s). Editorial review has deemed that any suppressed content does not materially affect the overall learning experience. Cengage Learning reserves the right to remove additional content at any time if subsequent rights restrictions require it. x Preface Table of Contents Changes Based on market research and feedback from users, Section 2.5 in the previous edition (Applications of 2 Matrices Matrix Operations) has been expanded from one section 2.1 Operations with Matrices to two sections to include content on Markov chains. 2.2 Properties of Matrix Operations 2.3 The Inverse of a Matrix So now, Chapter 2 has two application sections: 2.4 Elementary Matrices Section 2.5 (Markov Chains) and Section 2.6 (More 2.5 Markov Chains 2.6 More Applications of Matrix Operations Applications of Matrix Operations). In addition, Section 7.4 (Applications of Eigenvalues and Eigenvectors) has been expanded to include content on constrained optimization. Trusted Features Data Encryption (p. 94) Computational Fluid Dynamics (p. 79) ® For the past several years, an independent website— CalcChat.com—has provided free solutions to all odd-numbered problems in the text. Thousands of students have visited the site for practice and help Beam Deflection (p. 64) with their homework from live tutors. You can also use your smartphone’s QR Code® reader to scan the icon at the beginning of each exercise set to access the solutions. Information Retrieval (p. 58) Flight Crew Scheduling (p. 47) Clockwise from top left, Cousin_Avi/Shutterstock.com; Goncharuk/Shutterstock.com; 39 Gunnar Pippel/Shutterstock.com; Andresr/Shutterstock.com; nostal6ie/Shutterstock.com 62 Chapter 2 Matrices 2.3 The Inverse of a Matrix Find the inverse of a matrix (if it exists). Chapter Openers Use properties of inverse matrices. Use an inverse matrix to solve a system of linear equations. Each Chapter Opener highlights five real-life MATRICES AND THEIR INVERSES applications of linear algebra found throughout the Section 2.2 discussed some of the similarities between the algebra of real numbers and chapter. Many of the applications reference the the algebra of matrices. This section further develops the algebra of matrices to include the solutions of matrix equations involving matrix multiplication. To begin, consider the real number equation ax = b. To solve this equation for x, multiply both sides of Linear Algebra Applied feature (discussed on the the equation by a−1 (provided a ≠ 0). next page). You can find a full list of the ax = b (a−1a)x = a−1b applications in the Index of Applications on the (1)x = a−1b x = a−1b inside front cover. The number a−1 is the multiplicative inverse of a because a−1a = 1 (the identity element for multiplication). The definition of the multiplicative inverse of a matrix is similar. Section Objectives Definition of the Inverse of a Matrix An n × n matrix A is invertible (or nonsingular) when there exists an n × n A bulleted list of learning objectives, located at matrix B such that the beginning of each section, provides you the AB = BA = In where In is the identity matrix of order n. The matrix B is the (multiplicative) opportunity to preview what will be presented inverse of A. A matrix that does not have an inverse is noninvertible (or singular). in the upcoming section. Nonsquare matrices do not have inverses. To see this, note that if A is of size m × n and B is of size n × m (where m ≠ n), then the products AB and BA are of different sizes and cannot be equal to each other. Not all square matrices have inverses. (See Example 4.) The next theorem, however, states that if a matrix does have an Theorems, Definitions, and inverse, then that inverse is unique. Properties THEOREM 2.7 Uniqueness of an Inverse Matrix Presented in clear and mathematically precise If A is an invertible matrix, then its inverse is unique. The inverse of A is denoted by A−1. language, all theorems, definitions, and properties PROOF are highlighted for emphasis and easy reference. If A is invertible, then it has at least one inverse B such that AB = I = BA. Assume that A has another inverse C such that Proofs in Outline Form AC = I = CA. In addition to proofs in the exercises, some Demonstrate that B and C are equal, as shown on the next page. proofs are presented in outline form. This omits the need for burdensome calculations. QR Code is a registered trademark of Denso Wave Incorporated Copyright 2017 Cengage Learning. All Rights Reserved. May not be copied, scanned, or duplicated, in whole or in part. Due to electronic rights, some third party content may be suppressed from the eBook and/or eChapter(s). Editorial review has deemed that any suppressed content does not materially affect the overall learning experience. Cengage Learning reserves the right to remove additional content at any time if subsequent rights restrictions require it. Preface xi Discovery Using the Discovery feature helps you develop Finding a Transition Matrix an intuitive understanding of mathematical See LarsonLinearAlgebra.com for an interactive version of this type of example. concepts and relationships. Find the transition matrix from B to B′ for the bases for R3 below. B = {(1, 0, 0), (0, 1, 0), (0, 0, 1)} and B′ = {(1, 0, 1), (0, −1, 2), (2, 3, −5)} Technology Notes SOLUTION Technology notes show how you can use First use the vectors in the two bases to form the matrices B and B′. D I S CO V E RY graphing utilities and software programs [ ] [ ] 1 0 0 1 0 2 B= 0 1 0 and B′ = 0 −1 3 1. Let B = {(1, 0), (1, 2)} appropriately in the problem-solving process. and B′ = {(1, 0), (0, 1)}. 0 0 1 1 2 −5 Form the matrix Then form the matrix [B′ B] and use Gauss-Jordan elimination to rewrite [B′ B] as Many of the Technology notes reference the [B′ B]. [I3 P−1]. Technology Guide at CengageBrain.com. 2. Make a conjecture −1 [ ] [ ] 1 0 2 1 0 0 1 0 0 4 2 about the necessity of 0 −1 3 0 1 0 0 1 0 3 −7 −3 using Gauss-Jordan [ ] 1 2 −5 0 0 1 0 0 1 1 −2 −1 elimination to obtain the transition matrix From this, you can conclude that the transition matrix from B to B′ is P −1 when the change −1 [ ] 4 2 of basis is from a nonstandard basis to P−1 = 3 −7 −3. SOLUTION a standard basis. 1 −2 −1 Notice that three of the entries in the third column are zeros. So, to eliminate some of the work in the expansion, use the third column. Multiply P−1 by the coordinate matrix of x = [1 2 −1]T to see that the result is the same as that obtained in Example 3. ∣A∣ = 3(C13) + 0(C23) + 0(C33) + 0(C43) The cofactors C23, C33, and C43 have zero coefficients, so you need only find the TECHNOLOGY cofactor C13. To do this, delete the first row and third column of A and evaluate the Many graphing utilities and determinant of the resulting matrix. ∣ ∣ software programs can find the determinant of −1 1 2 R3 a square matrix. If you use C13 = (−1)1+3 0 2 3 Delete 1st row and 3rd column. a graphing utility, then you may 3 4 −2 ∣ ∣ see something similar to the screen below for Example 4. −1 1 2 The Technology Guide at = 0 2 3 Simplify. CengageBrain.com can help 3 4 −2 you use technology to find a determinant. Expanding by cofactors in the second row yields ∣ ∣ ∣ ∣ ∣ ∣ A 1 2 −1 2 −1 1 [[1 -2 3 0 ] C13 = (0)(−1)2+1 + (2)(−1)2+2 + (3)(−1)2+3 LINEAR Time-frequency analysisT of irregular physiological signals, [-1 1 0 2 ] 4 −2 3 −2 3 4 ALGEBRA such as beat-to-beat cardiac rhythm variations (also known [0 2 0 3 ] as heart rate variability or HRV), can be difficult. This is [3 4 0 -2]] = 0 + 2(1)(−4) + 3(−1)(−7) APPLIED because the structure of a signal can include multiple det A periodic, nonperiodic, and pseudo-periodic components. = 13. Researchers have proposed and validated a simplified HRV 39 analysis method called orthonormal-basis partitioning and You obtain time-frequency representation (OPTR). This method can detect both abrupt and slow changes in the HRV signal’s ∣A∣ = 3(13) structure, divide a nonstationary HRV signal into segments = 39. that are “less nonstationary,” and determine patterns in the HRV. The researchers found that although it had poor time resolution with signals that changed gradually, the OPTR method accurately represented multicomponent and abrupt changes in both real-life and simulated HRV signals. (Source: Orthonormal-Basis Partitioning and Time-Frequency Representation of Cardiac Rhythm Dynamics, Aysin, Benhur, et al, IEEE Transactions on Biomedical Engineering, 52, no. 5) 108 Chapter 2 Matrices Sebastian Kaulitzki/Shutterstock.com 2 Projects 1 Exploring Matrix Multiplication The table shows the first two test scores for Anna, Bruce, Chris, and David. Use the Test 1 Test 2 Anna 84 96 table to create a matrix M to represent the data. Input M into a software program or a graphing utility and use it to answer the questions below. 1. Which test was more difficult? Which was easier? Explain. Linear Algebra Applied Bruce Chris 56 78 72 83 2. How would you rank the performances of the four students? The Linear Algebra Applied feature describes a real-life [] [] 1 0 David 82 91 3. Describe the meanings of the matrix products M 0 and M 1. 4. Describe the meanings of the matrix products [1 0 0 0]M and [0 0 1 0]M. application of concepts discussed in a section. These 5. Describe the meanings of the matrix products M 1 1 and 21M 1 1[]. [] applications include biology and life sciences, business 6. Describe the meanings of the matrix products [1 1 1 1]M and 14 [1 1 1 1]M. and economics, engineering and technology, physical