Advanced Statistical Analysis: Event History Analysis 1
Document Details
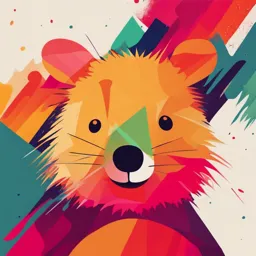
Uploaded by ClearerKoala
University of Groningen
Clara Mulder
Tags
Related
- Advanced Statistical Analysis: Event History Analysis 1
- ASA 6 Answers - Event History Analysis
- Advanced Statistical Analysis: Event History Analysis 2
- Advanced Statistical Analysis: Event History Analysis 3
- Analysis of Padre Faura Witnesses the Execution of Rizal PDF
- Palmer Raids Student Materials PDF
Summary
These lecture notes provide an introduction to event history analysis, a statistical approach for analyzing time-to-event data. The presentation covers key concepts, techniques, and examples, suitable for an undergraduate-level course in statistical modeling.
Full Transcript
F a c u l t y o f Sp a t i a l Sc i e n c e sF a c u l t y o f Sp a t i a l Sc i e n c e sF a c u l t y o f Sp a t i a l Sc i e n c e sF a c u l t y o f Sp a t i a l Sc i e n c e sAdvanced Statistical Analysis: Event History Analysis 1 Clara Mulder (thanks to colleagues) F a c u l t...
F a c u l t y o f Sp a t i a l Sc i e n c e sF a c u l t y o f Sp a t i a l Sc i e n c e sF a c u l t y o f Sp a t i a l Sc i e n c e sF a c u l t y o f Sp a t i a l Sc i e n c e sAdvanced Statistical Analysis: Event History Analysis 1 Clara Mulder (thanks to colleagues) F a c u l t y o f Sp a t i a l Sc i e n c e sF a c u l t y o f Sp a t i a l Sc i e n c e sF a c u l t y o f Sp a t i a l Sc i e n c e sF a c u l t y o f Sp a t i a l Sc i e n c e s | 2 Today: › Event history analysis 1: • Introduction • Cox regression › Literature: • Handbook Chapter 9 (Survival Analysis) F a c u l t y o f Sp a t i a l Sc i e n c e sF a c u l t y o f Sp a t i a l Sc i e n c e sF a c u l t y o f Sp a t i a l Sc i e n c e sF a c u l t y o f Sp a t i a l Sc i e n c e s | 3 Some terms › Survival analysis (general; also used specifically for univariate analysis!) › Event history analysis › Analysis of duration data › Hazard analysis / hazard regression › Intensity regression › Dutch: Gebeurtenissenanalyse › German: Ereignisanalyse F a c u l t y o f Sp a t i a l Sc i e n c e sF a c u l t y o f Sp a t i a l Sc i e n c e sF a c u l t y o f Sp a t i a l Sc i e n c e sF a c u l t y o f Sp a t i a l Sc i e n c e s | 4 How does this method link to univariate survival analysis / life table? › Survival analysis using Kaplan Meier: descriptive method to look at the distribution of time to event (for different groups in a sample) F a c u l t y o f Sp a t i a l Sc i e n c e sF a c u l t y o f Sp a t i a l Sc i e n c e sF a c u l t y o f Sp a t i a l Sc i e n c e sF a c u l t y o f Sp a t i a l Sc i e n c e s | 5 Question: How does the age of marriage differ between low, middle and highly educated women in India? Survival Function AGEMAR 500 400 300 200 100 0 C u m S u rviva l 1,2 1,0 ,8 ,6 ,4 ,2 0,0 -,2 Highest educational College/univers ity Secondary Primary Survival Function AGEMAR 500 400 300 200 100 0 C u m S u rviva l 1,2 1,0 ,8 ,6 ,4 ,2 0,0 -,2 Highest educational College/univers ity Secondary Primary Advanced Statistical AnalysisEHA: The hazard rate & the (Cohort) Life Table In a life table the hazard rate is estimated as (notation of the book): hazard rate (note: you may know r(t) as l x , n(t) as d x , h(t) as m x , with h(t) calculated over mid- year population) t r(t) n(t) h(t) S(t) 16 500 9 0.02 0.98 17 491 20 0.04 0.94 18 471 32 0.07 0.88 … … … … … … … … … … 32 39 3 0.08 0.08 33 36 1 0.03 0.07 Event: first partnership Survival: remaining single Interpretation: h(16) =0.02: 2% became partnered between age 16 and 17; h(32)= 0.08: of those who were unpartenered at their 32 nd birthday, 8% entered in their first relationship before 33 rd birthday. S(32)= .08: 8% are still single, 1-S(32) = 0.92 or 92% are partnered before age 33 F a c u l t y o f Sp a t i a l Sc i e n c e sF a c u l t y o f Sp a t i a l Sc i e n c e sF a c u l t y o f Sp a t i a l Sc i e n c e sF a c u l t y o f Sp a t i a l Sc i e n c e s | 7 From description to explanation: › What is the effect of educational level on the hazard (~probability) of marrying at age T? (quantifying the difference) › this is a question to be answered using event history analysis F a c u l t y o f Sp a t i a l Sc i e n c e sF a c u l t y o f Sp a t i a l Sc i e n c e sF a c u l t y o f Sp a t i a l Sc i e n c e sF a c u l t y o f Sp a t i a l Sc i e n c e s | 8 When applicable? › Assessing the influence of independent variables (covariates) on: (a) Duration from time zero until occurrence of an event, or (b) Hazard (~probability/rate) of occurrence of an event at a certain time point, given that it has not occurred before › Note: (a) and (b) are the same, only the effects would be opposite. Effects are expressed as effects on hazard. F a c u l t y o f Sp a t i a l Sc i e n c e sF a c u l t y o f Sp a t i a l Sc i e n c e sF a c u l t y o f Sp a t i a l Sc i e n c e sF a c u l t y o f Sp a t i a l Sc i e n c e s | 9 What is an event? › A change in status (e.g. from alive to dead, renter to homeowner, unmarried to married, married to divorced, employed to unemployed or the other way around): subject leaves study population = population at risk ( risk set ) upon event or › Something that happens at a certain time point without a change in status (e.g. change of residence): subject remains in risk set , new episode, duration clock back to 0 F a c u l t y o f Sp a t i a l Sc i e n c e sF a c u l t y o f Sp a t i a l Sc i e n c e sF a c u l t y o f Sp a t i a l Sc i e n c e sF a c u l t y o f Sp a t i a l Sc i e n c e sWhy not ‘ordinary’ linear regression of duration until event? | 10 F a c u l t y o f Sp a t i a l Sc i e n c e sF a c u l t y o f Sp a t i a l Sc i e n c e sF a c u l t y o f Sp a t i a l Sc i e n c e sF a c u l t y o f Sp a t i a l Sc i e n c e s | 11 Why not ‘ordinary’ linear regression of duration until event? › Cannot handle ‘censored data’ (what would be the value of the dependent variable if the event has not taken place?) › Impossible to include time-varying covariates (what is the ‘right’ value of an independent variable if the value changes between time 0 and the event?) F a c u l t y o f Sp a t i a l Sc i e n c e sF a c u l t y o f Sp a t i a l Sc i e n c e sF a c u l t y o f Sp a t i a l Sc i e n c e sF a c u l t y o f Sp a t i a l Sc i e n c e s | 12 Event history analysis › The crucial variable is the hazard h(t) › We first introduce the concept duration T = time until event F a c u l t y o f Sp a t i a l Sc i e n c e sF a c u l t y o f Sp a t i a l Sc i e n c e sF a c u l t y o f Sp a t i a l Sc i e n c e sF a c u l t y o f Sp a t i a l Sc i e n c e s | 13 What is a hazard? › a hazard is like a probability, BUT defined over a tiny (infinitely small) time interval between t and t+Δt › remember: a probability is always defined with reference to a certain time period: • e.g. in the life table: what is the probability of dying between ages x and x+n? Or in a given year F a c u l t y o f Sp a t i a l Sc i e n c e sF a c u l t y o f Sp a t i a l Sc i e n c e sF a c u l t y o f Sp a t i a l Sc i e n c e sF a c u l t y o f Sp a t i a l Sc i e n c e s | 14 Starting from a probability : › is the probability that an event occurs between time t and time t’ (where t < t’ ) › We want to know the probability that the event occurs between t and t’ , given that no event has occurred yet at time t: › That is the conditional probability: )' Pr( t T t )( tT ) | )' Pr( t T t T t )' Pr( t T t ) ( t T ) | )' Pr( t T t T t F a c u l t y o f Sp a t i a l Sc i e n c e sF a c u l t y o f Sp a t i a l Sc i e n c e sF a c u l t y o f Sp a t i a l Sc i e n c e sF a c u l t y o f Sp a t i a l Sc i e n c e s | 15 Hazard versus ‘ordinary’ probability › Probability, e.g. in the life table: the probability of dying between age x and x+1 conditional on having reached age x › The probability depends on the length of time: the larger the interval t’-t (= Δt) , the higher the probability › The hazard is not related to a particular interval of time: › = the propensity or intensity to have the event at time t › Is conditional, or: defined in relation to the population at risk , at time t, of having the event ' ' ) | )' Pr( lim ) ( ' t t t t t T t T t t h tt ' ' ) | )' Pr( lim ) ( ' t t t t t T t T t t h t t F a c u l t y o f Sp a t i a l Sc i e n c e sF a c u l t y o f Sp a t i a l Sc i e n c e sF a c u l t y o f Sp a t i a l Sc i e n c e sF a c u l t y o f Sp a t i a l Sc i e n c e s | 16 The hazard is the dependent variable in event history analysis › ‘ Application’ of the hazard leads to a survival function: the probability that the event has not occurred at time t, or equivalenty that the episode’s duration is at least t long (assuming it started at 0): › This is: the probability of (= proportion in the sample / population) surviving until time t ) Pr( ) ( t T t S ) Pr( ) ( t T t S F a c u l t y o f Sp a t i a l Sc i e n c e sF a c u l t y o f Sp a t i a l Sc i e n c e sF a c u l t y o f Sp a t i a l Sc i e n c e sF a c u l t y o f Sp a t i a l Sc i e n c e s | 17 for example: › the survival function in migration is the probability that no move has occurred at time t since last move, = the probability that the person has lived in her current place at least t time units (e.g. years) › the probability that a child lives at least t years (event = death) › the probability that no first child has been born (yet???) at least t years after partnership formation (event = first childbirth) F a c u l t y o f Sp a t i a l Sc i e n c e sF a c u l t y o f Sp a t i a l Sc i e n c e sF a c u l t y o f Sp a t i a l Sc i e n c e sF a c u l t y o f Sp a t i a l Sc i e n c e s | 18Censoring time a b Left censoring Right censoring Observation window Survey attrition a b F a c u l t y o f Sp a t i a l Sc i e n c e sF a c u l t y o f Sp a t i a l Sc i e n c e sF a c u l t y o f Sp a t i a l Sc i e n c e sF a c u l t y o f Sp a t i a l Sc i e n c e s | 19 Left censoring › Problematic in principle: duration unknown › Cox regression (this week): No proper way of handling left censoring › Discrete-time logit (next week): left censoring o.k. as long as there is no reason to suspect duration dependence of effects (= effects change through time) F a c u l t y o f Sp a t i a l Sc i e n c e sF a c u l t y o f Sp a t i a l Sc i e n c e sF a c u l t y o f Sp a t i a l Sc i e n c e sF a c u l t y o f Sp a t i a l Sc i e n c e s | 20 Right censoring › No problem as long as censoring can be assumed to be random F a c u l t y o f Sp a t i a l Sc i e n c e sF a c u l t y o f Sp a t i a l Sc i e n c e sF a c u l t y o f Sp a t i a l Sc i e n c e sF a c u l t y o f Sp a t i a l Sc i e n c e s | 21 Compare descriptive methods for duration data › Kaplan Meier (univariate survival analysis) › Example: job duration F a c u l t y o f Sp a t i a l Sc i e n c e sF a c u l t y o f Sp a t i a l Sc i e n c e sF a c u l t y o f Sp a t i a l Sc i e n c e sF a c u l t y o f Sp a t i a l Sc i e n c e s | 22 Kaplan Meier (estimates survival function): Job duration by sex F a c u l t y o f Sp a t i a l Sc i e n c e sF a c u l t y o f Sp a t i a l Sc i e n c e sF a c u l t y o f Sp a t i a l Sc i e n c e sF a c u l t y o f Sp a t i a l Sc i e n c e s | 23 › Graph describes the different survival of males and females in current job › But stated differently: what size is the effect of gender on job duration – or as it is usually expressed: on the hazard of ending a job? F a c u l t y o f Sp a t i a l Sc i e n c e sF a c u l t y o f Sp a t i a l Sc i e n c e sF a c u l t y o f Sp a t i a l Sc i e n c e sF a c u l t y o f Sp a t i a l Sc i e n c e s | 24 Event history model › We are going to model the hazard rate h(t) of quitting a job › The rate h(t) states how likely it is someone quits the current job (= event ) at time t (infinitely small time interval) › Whereas a probability p(t,t+n) states how likely it is that someone quits the current job between t and t+n. F a c u l t y o f Sp a t i a l Sc i e n c e sF a c u l t y o f Sp a t i a l Sc i e n c e sF a c u l t y o f Sp a t i a l Sc i e n c e sF a c u l t y o f Sp a t i a l Sc i e n c e s | 25 General form of event history models : estimator!Meier -Kaplan theis this model regressionCox In the )()( :y varsexplanator no have weif )exp()()( 0 0 thth Xthth estimator! Meier - Kaplan the is this model regression Cox In the ) ( ) ( : y vars explanator no have we if ) exp( ) ( ) ( 0 0 t h t h X t h t h F a c u l t y o f Sp a t i a l Sc i e n c e sF a c u l t y o f Sp a t i a l Sc i e n c e sF a c u l t y o f Sp a t i a l Sc i e n c e sF a c u l t y o f Sp a t i a l Sc i e n c e s | 26 The Cox model , introduced by D.R. Cox in 1972: ) exp( ) ( ) ( 0 X t h t h Baseline hazard = unspecified, can have any form Multiplied by exponentiated values of the explanatory vars ) exp( ) ( ) ( 0 X t h t h F a c u l t y o f Sp a t i a l Sc i e n c e sF a c u l t y o f Sp a t i a l Sc i e n c e sF a c u l t y o f Sp a t i a l Sc i e n c e sF a c u l t y o f Sp a t i a l Sc i e n c e s | 27 Cox model is also called the proportional hazards model : the hazard is assumed to be a time- constant ratio of a baseline hazard and Exp( X β ). Exp( β ) is the multiplicative effect of independent variable(s) X on the hazard (hazard ratio), β = additive effect on the log of the hazard. The plotted hazards should look like they have the same ratio through time, the log-hazards should look like they are parallel when plotted over time. F a c u l t y o f Sp a t i a l Sc i e n c e sF a c u l t y o f Sp a t i a l Sc i e n c e sF a c u l t y o f Sp a t i a l Sc i e n c e sF a c u l t y o f Sp a t i a l Sc i e n c e s | 28 Introduce the variable X: gender › Males: X=1, Females: X=0 › Male hazard function: › Female hazard function: › The hazard rates of males and females are assumed to be (and forced by the model into being) proportional by a constant ratio exp β exp)()( 0 thth males )()( 0 thth females exp) ( ) ( 0 t h t h males ) ( ) ( 0 t h t h femal es Advanced Statistical AnalysisThe Cox Model (or: Cox Regression) The general form of the model is: or is the hazard for individual i at time t is a vector of covariates with coefficients is the baseline hazard i.e. the hazard when =0. It can have any form! Note: an individual’s hazard depends on t through Interpretation: Covariates have a multiplicative effect on the hazard. i.e. for each unit increase in x the hazard is multiplied by exp(). if =0 if =1 is the ratio of the hazard for x=1 to x=0 also called relative risk or hazard ratio Advanced Statistical AnalysisThe Cox Model (continued) Interpretation: , = 0 no effect of x on the hazard found. In our example, no effect of sex on job duration. , > 0 positive effect of x on the hazard. Higher values of x are associated with shorter durations , < 0 negative effect of x on the hazard. Lower values of x are associated with longer durations Example: All else equal … The hazard of quitting a job is exp (0.4)= 1.49 times higher for women than for men The log hazard of quitting a job is 0.4 points higher for women than for men Women’s hazard of quitting a job is 49% higher compared to men Advanced Statistical AnalysisThe Cox Model (continued) In plot of log-hazard against time we should see parallel lines if proportional hazard assumption is met (left side). (what about hazard itself?). Proportional Non-Proportional F a c u l t y o f Sp a t i a l Sc i e n c e sF a c u l t y o f Sp a t i a l Sc i e n c e sF a c u l t y o f Sp a t i a l Sc i e n c e sF a c u l t y o f Sp a t i a l Sc i e n c e s | 32 The proportionality assumption is restrictive: › In many applications the hazard rates may not be proportional • E.g. marriage: women marry faster than men at young ages, but men have a higher marriage rate at older ages › Therefore you have to test if this assumption is valid › For example, use a graphical test: plot empirical survival or hazard functions using Kaplan Meier. There are also formal tests F a c u l t y o f Sp a t i a l Sc i e n c e sF a c u l t y o f Sp a t i a l Sc i e n c e sF a c u l t y o f Sp a t i a l Sc i e n c e sF a c u l t y o f Sp a t i a l Sc i e n c e s | 33 What if the test fails? › You may want to specify different baseline hazards for each category: ) exp( ) ( ) ( 0 X t h t h SEX Separate base line hazard rate for each sex The explanatory part with other vars may be similar or sex- specific ) exp( ) ( ) ( 0 X t h t h SEX F a c u l t y o f Sp a t i a l Sc i e n c e sF a c u l t y o f Sp a t i a l Sc i e n c e sF a c u l t y o f Sp a t i a l Sc i e n c e sF a c u l t y o f Sp a t i a l Sc i e n c e s | 34 Information in data: › Duration until event or censoring (number of time units) › Event or censoring? › Alternatively, for discrete-time models (next week!): Whether event takes place (yes or no) in time unit under study › Values of the independent variables Advanced Statistical AnalysisEvent History in Stata: stset command We need to tell STATA we want to perform survival analysis. The command is stset . stset: Tells Stata the structure of your survival data (so you do not have to repeat); Checks that the data structure makes sense; Allows us to describe complicated rules for when observations are included and excluded (advanced) General syntax: stset duration variable [if], failure( one_if_failure_var ) optionsNote: stset is useful in continuous-time models (Cox and parametric). Advanced Statistical Analysisstset command (continued) Before After Data in Event history format • Time variable • Censoring variable • Covariates Data in Event history format • Time variable • Censoring variable • Covariates stset information: • _t0, _t, _d, _st • Structure information • Data checkS T S E T Advanced Statistical Analysisstset command (continued) stset creates new variables: _ t0 and _t: they record the time span in analysis time units(t) for each record. _t0 is the starting and _t is the ending time; _d: it is equal to 1 if the event occurs and 0 if it does not. It helps us identifying failures and censoring; _st: for each observation, the variable = 1 if Stata uses the observation and 0 otherwise. stset has several options depending on which kind of data we have. Examples: a. Single episode (spell) data b. Multiple episode per person With time constant covariates (e.g. sex) c. Person-year data d. Time-varying covariates Advanced Statistical AnalysisEvent history analysis: Preparation After Stset: stsum to have an idea of your data distribution stdes for more advanced data structure Advanced Statistical AnalysisEvent history analysis: Preparation After Stset: Plotting the survival curve using Kaplan Meier Plotting the (smoothed) hazard sts graph, ci sts graph, by( sex ) sts graph, hazard by( sex ) sex .4121536 .0977979 4.21 0.000 .2204733 .6038338 _t Coef. Std. Err. z P>|z| [95% Conf. Interval] Log likelihood = -2388.8621 Prob > chi2 = 0.0000 LR chi2(1) = 17.53 Time at risk = 38153 No. of failures = 430 No. of subjects = 563 Number of obs = 563 Cox regression -- Breslow method for ties Iteration 0: log likelihood = -2388.8621 Refining estimates: Iteration 2: log likelihood = -2388.8621 Iteration 1: log likelihood = -2388.8741 Iteration 0: log likelihood = -2397.6278 analysis time _t: jobdur failure _d: event1Advanced Statistical AnalysisThe Cox Model in Stata Example: Cox regression model of job duration First step is to stset the data. stset jobdur , failure( event1 ) stcox i. sex , nohr =no hazard ratio. Stata shows coefficients, i.e. effects on logCategorical variable sex .4121536 .0977979 4.21 0.000 .2204733 .6038338 _t Coef. Std. Err. z P>|z| [95% Conf. Interval] Log likelihood = -2388.8621 Prob > chi2 = 0.0000 LR chi2(1) = 17.53 Time at risk = 38153 No. of failures = 430 No. of subjects = 563 Number of obs = 563 Cox regression -- Breslow method for ties Iteration 0: log likelihood = -2388.8621 Refining estimates: Iteration 2: log likelihood = -2388.8621 Iteration 1: log likelihood = -2388.8741 Iteration 0: log likelihood = -2397.6278 analysis time _t: jobdur failure _d: event1Advanced Statistical AnalysisThe Cox Model in Stata (continued) Example: Cox regression model of job duration First step is to stset the data. stset jobdur , failure( event1 ) stcox i. sex , nohr Note: If multiple records per individual, add the option vce(cluster id ) here. In this way standard errors will be robust. sex .4121536 .0977979 4.21 0.000 .2204733 .6038338 _t Coef. Std. Err. z P>|z| [95% Conf. Interval] Log likelihood = -2388.8621 Prob > chi2 = 0.0000 LR chi2(1) = 17.53 Time at risk = 38153 No. of failures = 430 No. of subjects = 563 Number of obs = 563 Cox regression -- Breslow method for ties Iteration 0: log likelihood = -2388.8621 Refining estimates: Iteration 2: log likelihood = -2388.8621 Iteration 1: log likelihood = -2388.8741 Iteration 0: log likelihood = -2397.6278 analysis time _t: jobdur failure _d: event1Advanced Statistical AnalysisThe Cox Model in Stata (continued) Example: Cox regression model of job duration stset jobdur , failure( event1 ) stcox i. sex , nohr test for null hypothesis (H0): If we remove the option nohr, the test will be for Advanced Statistical AnalysisThe Cox Model in STATA: Tip If more than one episode per person (person number = id): Use option vce or robust cluster(id) to obtain robust standard errors stcox i. sex , vce(cluster id ) [nohr] or stcox i. sex , robust cluster( id ) [nohr]