EEP/IAS 118 Introductory Applied Econometrics, Section 1 PDF
Document Details
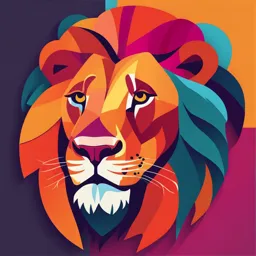
Uploaded by SwiftPrimrose2997
University of California, Berkeley
2024
Nicolas Polasek and Leila Njee Bugha
Tags
Related
- 1 Introduction Linear regression model.pdf
- 2 Regression diagnostics complications.pdf
- 3 Endogeneity Instrumental variables.pdf
- 11_Regression with a binary dependent variable.pptx (1).pdf
- EEP/IAS 118 - Introductory Applied Econometrics, Section 2 PDF
- EEP/IAS 118 Introductory Applied Econometrics, Section 3 PDF
Summary
These are lecture notes covering introductory applied econometrics. Topics include linear regression models, functional forms, and random variable reviews. The document includes relevant examples, including interpreting marginal effects and exploring relationships between variables.
Full Transcript
EEP/IAS 118 - Introductory Applied Econometrics, Section 1 Nicolas Polasek and Leila Njee Bugha Week of September 2, 2024 Intro Brief Intro Section In-person; notes and slides posted online beforehand (usually), solutions and vi...
EEP/IAS 118 - Introductory Applied Econometrics, Section 1 Nicolas Polasek and Leila Njee Bugha Week of September 2, 2024 Intro Brief Intro Section In-person; notes and slides posted online beforehand (usually), solutions and videos posted afterward Attendance not required Mainly review material from lectures; sometimes discuss assignments Try to come to your scheduled section - fine to swap here and there 2 / 20 Talk to us Office hours: in-person Nicolas: Wednesdays 3PM-5PM; 203 Giannini Leila: Fridays 1:30PM-3:30PM; 237 Giannini Email us if these times don’t work for you, or if you need remote accommodations Email policy: we’ll respond within 48 hours during the week Don’t be afraid to reach out - we can’t help you if we don’t know! Econometrics material can be hard to grasp initially - but that’s okay! Tell us if you are having technology problems (e.g., bad Internet connection, rolling power outages, issues with DataHub) 3 / 20 Class websites bCourses: course announcements, files, videos, assignments DataHub server: host for assignment Jupyter notebooks; do your work there and then save as PDF to submit Gradescope: website for submitting completed assignments 4 / 20 Topics for today Linear regression models Functional forms Random variable review Announcements: Small Assignment 1 due next Friday (Sept 13) 5 / 20 The linear regression model assumes that the relationship between Y and X is linear - economists we then try to find the line that most closely approximates the true relationship. The Linear Regression priate Models picture to have in mind is the following:2 Key components of figure: Any data: Actual data set x j , you y j - work with will observations of have some the two outcomes you are interested in (the Y term variables some explanatory variables (the X term). Plotting these data points will often produce somethi Line equation resembles a line. The ŷ = β 0 + β 1role for economist’s x - is to is this estimate the equation the predicted value of ofthat the line - notice how the eq outcome in the graph y, variable ŷ b0 + b1 x is the equation of a line, as you learned in calc 1. ŷ = Residuals (ûi ) - the difference between the predicted ŷi and the actual observed yi 2. Model Example 6 / 20 Relationships between variables A model is simply trying to describe a relationship between variables. However, we need to be careful. A news anchor shows a hospital in poor condition, and states: “As you can see, health services here are so bad that going to a hospital is actually worse than staying at home. The following statistics demonstrate that you are better off staying away from hospitals” 7 / 20 A news anchor states “The following statistics demonstrate that you are better off staying away from hospitals” What is the implied research question from this story? Do you agree with the news anchor’s conclusion? Why or why not? What are the components of the regression model you would use to analyze this question (if you had the data)? 8 / 20 What is the implied research question from this story? What is the effect of going to the hospital on full recovery from an illness? Do you agree with the news anchor’s conclusion? No, because the sample of people who go to the hospital is different from the sample that does not. What are the components of the regression model we would use to analyze this question (if you had the data)? Dependent variable (Y) = Fully Recover Explanatory variable of interest (X1 ) = Went to hospital Other explanatory variables (X1 , X2 ,...) = Age, Medical History, Severity of illness 8 / 20 Correlation vs. Causation In this example, we do see a negative correlation between recovery and visiting the hospital So, what does newspaper article get wrong? There is a correlation! The article falsely assigns causality to the relationship - this is the classic correlation ̸= causation The statistic is misleading (if improperly understood) because it omits other important variables associated with recovery from the model (age, medical history, severity of illness, etc.) The core of this class is to understand how and when we can assign causality to observed relationships. 9 / 20 Review on functional forms Preliminary concepts: x1 − x0 Proportional change: x0 = ∆x x0 x1 − x0 Percentage change: x0 × 100 = ∆x x0 × 100 ∆z/z ∂z x Elasticity : ∆x/x = ∂x z Note, percent change is just proportional change times 100. Elasticity is the “percent change in one variable in response to a given (one) percent change in another variable” 10 / 20 Functional forms and Marginal Effects This table (Table 2.3 in Wooldridge) is meant to practice and continue familiarizing ourselves with these functional forms. Model DepVar Ind. Var ∆y relates to ∆x? Interpretation Linear y x ∆y = β 1 ∆x ∆y = β 1 ∆x Logarithmic y log( x ) ∆y = β 1 ∆x x ∆y = ( β 1 /100)%∆x ∆y Exponential log(y) x y = β 1 ∆x %∆y = (100β 1 )∆x ∆y Log-Log log(y) log( x ) y = β 1 ∆x x %∆y = β 1 %∆x Ex: %∆y = (100β 1 )∆x - Read this as ”ŷ increases by 100 ∗ β 1 % for a one unit increase in x.” Derivations of these are in the notes! Tip: When you see logs, think percent changes! 11 / 20 Examples: Interpreting Marginal Effects Suppose you’ve collected data on household gasoline consumption (gallons) in the Bay Area and gas prices ($ per gallon), and you estimate the following model: log( gasoline) = 12 − 0.21price According to the model, how does gas consumption change when price increases by $1? 12 / 20 Examples: Interpreting Marginal Effects Suppose you’ve collected data on household gasoline consumption (gallons) in the Bay Area and gas prices ($ per gallon), and you estimate the following model: log( gasoline) = 12 − 0.21price According to the model, how does gas consumption change when price increases by $1? If price increases by $1, then predicted gasoline consumption will decrease by 21%. 12 / 20 Examples: Interpreting Marginal Effects Professor Magruder uses firm data from Kenya to investigate how basket sales were affected by straw prices. In this example, he looks at the share of basket purchases that were made while baskets were on sale. The following model can be estimated: log(basketshare) = 0.83 + 0.491 log(strawprice) How does basketshare change if straw prices rise by 2%? 12 / 20 Examples: Interpreting Marginal Effects Professor Magruder uses firm data from Kenya to investigate how basket sales were affected by straw prices. In this example, he looks at the share of basket purchases that were made while baskets were on sale. The following model was estimated: log(basketshare) = 0.83 + 0.491 log(strawprice) How does basketshare change if straw prices rise by 2%? This is a log-log model, so if the price of straw increases by 2%, then the predicted share of baskets sold on sale increases by 0.98%. %∆y = 0.491 ∗ 2% = 0.98% 12 / 20 Examples: Interpreting Marginal Effects Suppose you’ve collected data on CEO salaries (hundred thousand $) and annual firm sales (million $), and you estimate the following model: salary = 2.23 + 1.1 log(sales) According to the model, how does salary change if annual firm sales increase by 10%? 12 / 20 Examples: Interpreting Marginal Effects salary = 2.23 + 1.1 log(sales) According to the model, how does salary change if annual firm sales increase by 10%? Sol. If annual firm sales increase by 10%, the model predicts that CEO salary increases by $11,000. If annual firm sales increase by 10%, then we know %∆x = 10. ∆y = ( β 1 /100)%∆x We can plug this and our estimate of β 1 into the formula from the table to see that 1.1 ∆y = 100 ∗ 10 = 0.11. Since the units of CEO salaries is $100,000, an increase of 0.11 units is an increase of $11,000. 12 / 20 Why do we care about random variables? Let’s start with an example from physics Dx = v avg Dt. This is an example of a deterministic relationship, if we know the average velocity (v ) and the time Stat Review: Random Variables avg that has an object has traveled (t), we know the change in it’s position (Dx) with certainty. There are few (if any!) relationships like this in economics. If we know someone’s education level and gender, Random variables are numbers that are taken from a distribution of possible outcomes. we may have a good sense of their expected wages, but we don’t have a formula for their exact wages. Thus, we treat A fundamental wages, way to education, describeand gender as random a random variables, variable and explain is through itstheir relationships probability using distribution statistical techniques. function. Any discrete random variable can be completely described by detailing the possible values it takes, as well as the associated probability that it takes each value. The probability density function Discrete (pdf)random variable of X summarizes pdf: the information concerning the possible outcomes of X and the associated prob- abilities. f ( x j ) = P( X = x j ), j = {1, 2, 3, 4, 5,...k } f (0) = 0.20 ; f (1) = 0.44 ; f (2) = 0.36 13 / 20 Stat Review: Random Variables Continuous variable (pdf): Z b EEP/IAS 118 - Introductory Applied Econometrics Lane and Ramirez Ritchie Spring 2017 Pr ( a < X < b) = f ( x )dx Section Handout 1 a Cumulative Distribution Function: When computing probabilities for continuous random vari- 14 / 20 ables, it is easiest to work with the cumulative distribution function (cdc). The CDF of a random variable Stat Review: Random Variables The cumulative distribution function is another useful way to visualize a random variable: 15 / 20 Stat Review: Random Variables If we have two discrete random variables X and Y, we can define the joint probability density function of (X,Y): f X,Y = P( X = x, Y = y) Two variables are independent if the joint PDF is equal to the product of the individual variables’ pdf. P( X = x, Y = y) = P( X = x ) P(Y = y) The conditional distribution of Y given X, which is described by the conditional probability density function : f (Y | X ) ( y | x ) = P ( Y = y | X = x ) 16 / 20 distribution of Y given X, which is described by the conditional probability density function : Stat Review: Random Variables f ( y | x ) = P (Y = y | X = x ) (Y | X ) b. Example Take the following example of a survey of 652 women applying for a job at a factory. Two pieces of Let’s do information an example that were usingcollected surveyinclude whether data from a woman was the head of her household and how 652 women: much education she had completed. Look below at the following charts: Head of household Head of household Yes No Yes No Incomplete primary 30 124 Incomplete primary 0.05 0.19 Primary only 44 192 Primary only 0.07 0.29 Secondary 123 139 Secondary 0.19 0.21 Note that the chart on the left gives the total number of women who fit in each cell of the chart. The sum of these cells is 652. From this chart, we could then calculate the chart on the right which tells us What whatisproportion joint probability of women fall that a category. into each randomEach person is chart cell of the a secondary schoolusgraduate on the right provides with and the joint probability of two events happening. not a head of household? 4 17 / 20 f (Y | X ) ( y | x ) = P ( Y = y | X = x ) Stat Review: Random Variables b. Example Take the following example of a survey of 652 women applying for a job at a factory. Two pieces of Let’s do information an example that were usingcollected surveyinclude whether a woman was the head of her household and how data: much education she had completed. Look below at the following charts: Head of household Head of household Yes No Yes No Incomplete primary 30 124 Incomplete primary 0.05 0.19 Primary only 44 192 Primary only 0.07 0.29 Secondary 123 139 Secondary 0.19 0.21 Note that the chart on the left gives the total number of women who fit in each cell of the chart. The sum of these cells is 652. From this chart, we could then calculate the chart on the right which tells us What whatisproportion joint probability of women fall that a category. into each randomEach person is chart cell of the a secondary schoolusgraduate on the right provides with and the joint probability of two events happening. not a head of household? 4 f (Secondary, no ) = 0.21 17 / 20 distribution of Y given X, which is described by the conditional probability density function : Stat Review: Random Variables f ( y | x ) = P (Y = y | X = x ) (Y | X ) b. Example Take the following example of a survey of 652 women applying for a job at a factory. Two pieces of Let’s do information an example that were usingcollected surveyinclude whether a woman was the head of her household and how data: much education she had completed. Look below at the following charts: Head of household Head of household Yes No Yes No Incomplete primary 30 124 Incomplete primary 0.05 0.19 Primary only 44 192 Primary only 0.07 0.29 Secondary 123 139 Secondary 0.19 0.21 Note that the chart on the left gives the total number of women who fit in each cell of the chart. The sum of these cells is 652. From this chart, we could then calculate the chart on the right which tells us What whatisproportion the conditional of women fallprobability thatEach into each category. a cell randomly of the chartdrawn head on the right of household provides us with did the joint probability of two events happening. NOT complete primary school? 4 17 / 20 f (Y | X ) ( y | x ) = P ( Y = y | X = x ) Stat Review: Random Variables b. Example Take the following example of a survey of 652 women applying for a job at a factory. Two pieces of Let’s do information an example that were usingcollected surveyinclude whether a woman was the head of her household and how data: much education she had completed. Look below at the following charts: Head of household Head of household Yes No Yes No Incomplete primary 30 124 Incomplete primary 0.05 0.19 Primary only 44 192 Primary only 0.07 0.29 Secondary 123 139 Secondary 0.19 0.21 Note that the chart on the left gives the total number of women who fit in each cell of the chart. The sum of these cells is 652. From this chart, we could then calculate the chart on the right which tells us What whatisproportion the conditional of women fallprobability thatEach into each category. a cell randomly of the chartdrawn head on the right of household provides us with did the joint probability of two events happening. NOT complete primary school? 4 f ( Incomplete|yes) = 30/197 = 0.15 17 / 20 Features of Probability Distributions The expected value of X: k E ( X ) = x1 f ( x1 ) + x2 f ( x2 ) + · · · + x k f ( x k ) = ∑ xj f (xj ) j =1 If X is continuous Z +∞ E( X ) = x f ( x )d( x ) −∞ The variance of X: Var ( X ) = E[( X − E( X ))2 ] The standard deviation of X q sd( X ) = Var ( X ) 18 / 20 Sample Properties An important distinction in this class is the difference between populations and samples. We deal with samples, so we can never know the real pdf or cdf of the population at large. However, we can calculate the statistical properties of these samples: Sample Mean: 1 n n i∑ X̄n = Xi =1 Sample Variance: n 1 n − 1 i∑ S2 = ( Xi − X̄n )2 =1 Note: it seems like we should divide by n, but instead we divide by n − 1. We do this to ensure that the sample variance estimator is an unbiased estimator of population variance 19 / 20 Sample Properties: Law of Large Numbers In small samples, the sample mean can be quite different from the true population mean For example, if I roll a five and a six on a die the sample mean will be 1 2 (6 + 5) = 5.5, even when we know the true population expected value of a die roll is 3.5: E( X ) = 1 61 + 2 16 + 3 16 + 4 16 + 5 16 + 6 16 = 3.5 Usefully, the law of large numbers says that if we draw a sample consisting of n realizations of our random variable, and take the average, this sample mean will approach the population mean as n approaches infinity. This means that if I roll a die more and more, my sample mean will approach the true population mean of 3.5 20 / 20