Graphs Chapter 5 PDF
Document Details
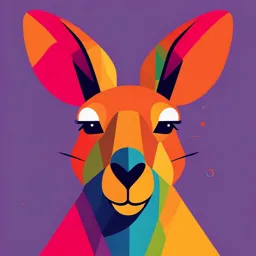
Uploaded by SharpestSerpentine2147
Tags
Summary
This document provides an overview of graphs, including terminologies, representations, and algorithms like Depth-First Search (DFS) and Breadth-First Search (BFS). It also touches upon concepts like weighted graphs, digraphs, and topological sorting.
Full Transcript
Chapter 5 Graphs Syllabus Graphs 32 Introduction, Graph Terminologies, 33 Representation of Graph 34 Graph Traversals-Depth First Search (DFS) and Breadth First Search (BFS), 35 Graph Application -Topological Sorting Graphs A...
Chapter 5 Graphs Syllabus Graphs 32 Introduction, Graph Terminologies, 33 Representation of Graph 34 Graph Traversals-Depth First Search (DFS) and Breadth First Search (BFS), 35 Graph Application -Topological Sorting Graphs A graph can be defined as group of vertices and edges that are used to connect these vertices. A graph can be seen as a cyclic tree, where the vertices (Nodes) maintain any complex relationship among them instead of having parent child relationship. A graph G can be defined as an ordered set G(V, E) where V(G) represents the set of vertices and E(G) represents the set of edges which are used to connect these vertices. A Graph G(V, E) with 5 vertices (A, B, C, D, E) and six edges ((A,B), (B,C), (C,E), (E,D), (D,B), (D,A)) is shown in the following figure. Directed and Undirected Graph A graph can be directed or undirected. However, in an undirected graph, edges are not associated with the directions with them. An undirected graph is shown in the earlier figure since its edges are not attached with any of the directions. If an edge exists between vertex A and B then the vertices can be traversed from B to A as well as A to B. In a directed graph, edges form an ordered pair. Edges represent a specific path from some vertex A to another vertex B. Node A is called initial node while node B is called terminal node. A directed graph is shown in the following figure. Graph Terminology Path A path can be defined as the sequence of nodes that are followed in order to reach some terminal node V from the initial node U. Closed Path A path will be called as closed path if the initial node is same as terminal node. A path will be closed path if V0=VN. Simple Path If all the nodes of the graph are distinct with an exception V0=VN, then such path P is called as closed simple path. Cycle A cycle can be defined as the path which has no repeated edges or vertices except the first and last vertices. Connected Graph A connected graph is the one in which some path exists between every two vertices (u, v) in V. There are no isolated nodes in connected graph. Complete Graph A complete graph is the one in which every node is connected with all other nodes. A complete graph contain n(n-1)/2 edges where n is the number of nodes in the graph. Weighted Graph In a weighted graph, each edge is assigned with some data such as length or weight. The weight of an edge e can be given as w(e) which must be a positive (+) value indicating the cost of traversing the edge. Digraph A digraph is a directed graph in which each edge of the graph is associated with some direction and the traversing can be done only in the specified direction. Loop An edge that is associated with the similar end points can be called as Loop. Adjacent Nodes If two nodes u and v are connected via an edge e, then the nodes u and v are called as neighbours or adjacent nodes. Degree of the Node A degree of a node is the number of edges that are connected with that node. A node with degree 0 is called as isolated node. Graph Representation There are two ways to store Graph into the computer's memory. 1. Sequential Representation In sequential representation, we use adjacency matrix to store the mapping represented by vertices and edges. In adjacency matrix, the rows and columns are represented by the graph vertices. A graph having n vertices, will have a dimension n x n. An entry Mij in the adjacency matrix representation of an undirected graph G will be 1 if there exists an edge between Vi and Vj. 2. Linked Representation: In the linked representation, an adjacency list is used to store the Graph into the computer's memory. Sequential Representation in the above figure, we can see the mapping among the vertices (A, B, C, D, E) is represented by using the adjacency matrix which is also shown in the figure. There exists different adjacency matrices for the directed and undirected graph. In directed graph, an entry Aij will be 1 only when there is an edge directed from Vi to Vj. A directed graph and its adjacency matrix representation is shown in the following figure. Representation of weighted directed graph is different. Instead of filling the entry by 1, the Non- zero entries of the adjacency matrix are represented by the weight of respective edges. The weighted directed graph along with the adjacency matrix representation is shown in the following figure. Linked Repesentation Linked Representation In the linked representation, an adjacency list is used to store the Graph into the computer's memory. Consider the undirected graph shown in the following figure and check the adjacency list representation. An adjacency list is maintained for each node present in the graph which stores the node value and a pointer to the next adjacent node to the respective node. If all the adjacent nodes are traversed then store the NULL in the pointer field of last node of the list. The sum of the lengths of adjacency lists is equal to the twice of the number of edges present in an undirected graph. Consider the directed graph shown in the following figure and check the adjacency list representation of the graph. In a directed graph, the sum of lengths of all the adjacency lists is equal to the number of edges present in the graph. In the case of weighted directed graph, each node contains an extra field that is called the weight of the node. The adjacency list representation of a directed graph is shown in the following figure. Graph Traversal Algorithm Traversing the graph means examining all the nodes and vertices of the graph. There are two standard methods by using which, we can traverse the graphs. Breadth First Search Depth First Search DFS and BFS Breadth First Search (BFS) Algorithm Breadth first search is a graph traversal algorithm that starts traversing the graph from root node and explores all the neighboring nodes. Then, it selects the nearest node and explore all the unexplored nodes. The algorithm follows the same process for each of the nearest node until it finds the goal. The algorithm of breadth first search is given below. The algorithm starts with examining the node A and all of its neighbors. In the next step, the neighbors of the nearest node of A are explored and process continues in the further steps. The algorithm explores all neighbors of all the nodes and ensures that each node is visited exactly once and no node is visited twice. BFS Algorithm A standard BFS implementation puts each vertex of the graph into one of two categories: Visited Not Visited The purpose of the algorithm is to mark each vertex as visited while avoiding cycles. The algorithm works as follows: 1. Start by putting any one of the graph's vertices at the back of a queue. 2. Take the front item of the queue and add it to the visited list. 3. Create a list of that vertex's adjacent nodes. Add the ones which aren't in the visited list to the back of the queue. 4. Keep repeating steps 2 and 3 until the queue is empty. Depth First Search (DFS) Algorithm Depth first search (DFS) algorithm starts with the initial node of the graph G, and then goes to deeper and deeper until we find the goal node or the node which has no children. The algorithm, then backtracks from the dead end towards the most recent node that is yet to be completely unexplored. The data structure which is being used in DFS is stack. The process is similar to BFS algorithm. In DFS, the edges that leads to an unvisited node are called discovery edges while the edges that leads to an already visited node are called block edges. Algorithm-DFS A standard DFS implementation puts each vertex of the graph into one of two categories: Visited Not Visited The purpose of the algorithm is to mark each vertex as visited while avoiding cycles. The DFS algorithm works as follows: 1. Start by putting any one of the graph's vertices on top of a stack. 2. Take the top item of the stack and add it to the visited list. 3. Create a list of that vertex's adjacent nodes. Add the ones which aren't in the visited list to the top of the stack. 4. Keep repeating steps 2 and 3 until the stack is empty. Depth first search (DFS) algorithm After inserting element 6 in the stack, we will look at the unvisited adjacent vertices of node 6. As there is no unvisited adjacent vertices of node 6, so we cannot move beyond node 6. In this case, we will perform backtracking. The topmost element, i.e., 6 would be popped out from the stack as shown below: BFS vs DFS Parameter BFS DFS Structure of Data It uses the Queue Data Structure for It uses the Stack Data Structure for finding the finding the shortest path. shortest path. Speed It is comparatively slower than the It is comparatively faster than the BFS method. DFS method. Distance from BFS acts better when the target stays DFS acts better when the target stays farther Source closer to the source. from the source. Suitability for Since BFS considers all of its DFS is comparatively much more suitable for Decision Tree neighbors, it is not very suitable for the decision trees. Within a decision, it allows a the decision trees used in puzzle user to traverse further to augment that decision. games. If you reach a conclusion, you reach the win situation, and you stop. Type of List BFS operates using the FIFO list. DFS operates using the LIFO list. Tracking Method BFS uses the queue to keep track of DFS uses the stack to keep track of the next the next location that it should visit. location that it should visit. Type of Solution It ensures a shallow path solution. BFS It does not ensure a shallow path solution. DFS directly finds the shortest path that first heads to the bottom of any subtree. Then, it leads to a solution. backtracks. Tree Path It traverses a path according to the tree It traverses a path according to the tree depth. level. Backtracking You don’t need to backtrack in BFS. You need to follow a backtrack in DFS. Applications of BFS and DFS Examples of applications of BFS: Check if a graph is connected Generating spanning tree Finding the shortest path in a graph GPS navigation Examples of applications of DFS: Testing connectivity Finding a path between two nodes Solving puzzles Topological Sort The topological sorting for a directed acyclic graph is the linear ordering of vertices. For every edge U-V of a directed graph, the vertex u will come before vertex v in the ordering. Nodes after topological sorted order: 5 4 2 3 1 0 Applications Few important applications of topological sort are- Scheduling jobs from the given dependencies among jobs Instruction Scheduling Determining the order of compilation tasks Data Serialization Example Find the number of different topological orderings possible for the given graph- Write in-degree of each vertex- Vertex-A has the least in-degree. So, remove vertex-A and its associated edges. Now, update the in-degree of other vertices. Vertex-B has the least in-degree. So, remove vertex-B and its associated edges. Now, update the in-degree of other vertices. There are two vertices with the least in-degree. So, following 2 cases are possible- In case-01, Remove vertex-C and its associated edges. Then, update the in-degree of other vertices. In case-02, Remove vertex-D and its associated edges. Then, update the in-degree of other vertices. In case-01, Remove vertex-D since it has the least in-degree. Then, remove the remaining vertex-E. In case-02, Remove vertex-C since it has the least in-degree. Then, remove the remaining vertex-E. For the given graph, following 2 different topological orderings are possible- ABC DE ABD CE