Data Analysis SS L1 PDF
Document Details
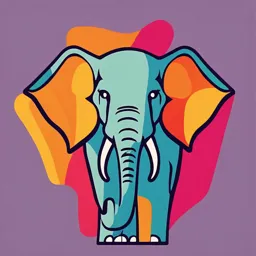
Uploaded by GenuineJaguar3107
Tags
Summary
These lecture notes cover data analysis, including learning outcomes, introductions, types of data, data analysis processes, tools, importance, applications, and graphical representations. The material is focused on practical application for financial analysis.
Full Transcript
Data Analysis Learning outcomes Explain what data analysis entails. Calculate and interpret the following descriptive statistics Discuss what graphical representation of data refers to and what its purpose is. Critically evaluate the inputs used in preparing the graphs and visuals. Discu...
Data Analysis Learning outcomes Explain what data analysis entails. Calculate and interpret the following descriptive statistics Discuss what graphical representation of data refers to and what its purpose is. Critically evaluate the inputs used in preparing the graphs and visuals. Discuss how graphs can be manipulated and misinterpreted. Discuss what the term “risk” refers to. Calculate and discuss the expected value of a decision. Discuss what decision tree analysis is and what its purpose is. Draw and interpret a decision tree. Briefly discuss the various data analytics tools available. Discuss how data analysis links with Financial statement analysis and Budgeting. Refer to Click Up material Introduction to data analysis Data analysis is the process of inspecting, cleaning, and modelling data to discover useful information. Data analysis supports decision-making and enhances understanding of financial performance. Types of data Qualitative data: Non numerical information (e.g., customer satisfaction). Quantitative data: Numerical data (e.g., revenue, expenses.) Structured: Organised data (e.g., spreadsheets). Unstructured: Unorganised data (e.g., emails, reports) Data analysis process Steps Involved: 1.Data Collection: Gather relevant financial data from various sources. 2.Data Cleaning: Remove errors and inconsistencies. 3.Data Modelling: Use statistical methods to analyse data. 4.Data Interpretation: Draw conclusions and insights. Tools used in data analysis Software Applications: Excel: Basic data analysis and visualization. R and Python: Advanced statistical analysis and modelling. BI Tools: Tableau, Power BI for data visualization. Importance of data analysis Informed Decision-Making: Helps managers make strategic decisions based on data insights. Risk Assessment: Identifies potential financial risks. Performance Evaluation: Measures financial performance against benchmarks. Applications of Data Analysis Budgeting: Forecasting revenues and expenses. Investment Analysis: Evaluating investment opportunities and risks. Cost Management: Identifying areas for cost reduction and efficiency improvement. Graphical presentation of data Graphical representation of data refers to the visual depiction of data sets using charts, graphs, and other visual tools. This method transforms numerical and categorical information into a format that is easier to understand and interpret. Purpose: 1. Simplification of Complex Data: Graphs help distil large amounts of data into a more digestible format, making it easier to identify trends, patterns, and anomalies. 2. Enhanced Understanding: Visual aids facilitate comprehension, allowing viewers to grasp relationships and distributions within the data more readily than raw numbers alone. 3. Effective Communication: Graphical representations provide a clear way to present findings to stakeholders, making it simpler to convey insights and conclusions. Graphical presentation of data 4. Identification of Trends: Charts and graphs enable users to visualize trends over time, making it easier to analyse changes and predict future performance. 5. Comparison of Data Sets: Visual tools allow for straightforward comparisons between different data sets, highlighting differences and similarities that may not be obvious in tabular form. 6. Engagement: Well-designed visuals can engage the audience more effectively than textual data, leading to greater interest and retention of information. By utilizing graphical representations, data analysts and financial managers can communicate insights more effectively and support better decision-making processes. Inputs Used in Preparing Graphs and Visuals: Data Quality Accuracy: The data must be correct and reliable. Inaccurate data can lead to misleading visuals and erroneous conclusions. Completeness: Missing data can skew results. It’s essential to ensure that the dataset is comprehensive to represent the intended insights accurately. Relevance: Data should be pertinent to the questions being addressed. Irrelevant information can clutter visuals and obscure key messages. Inputs Used in Preparing Graphs and Visuals: Data Selection Purpose-Driven Selection: Choosing the right data subset for the specific analysis is crucial. Including extraneous data can dilute focus and mislead the audience. Granularity: The level of detail in the data should match the objectives of the visualization. Too much detail can overwhelm, while too little can oversimplify. Inputs Used in Preparing Graphs and Visuals: Graph Type Choice Appropriate Visualization: Selecting the right type of graph (e.g., bar chart, line graph, pie chart) is critical for effectively conveying the message. The choice should align with the data characteristics and the story being told. Clarity of Representation: Some graph types may distort the data (e.g., 3D graphs can mislead), so it’s vital to prioritize clarity and honesty in representation. Inputs Used in Preparing Graphs and Visuals: Design Elements Colour and Contrast: Colour choices should enhance readability and accessibility. Poor colour combinations can hinder understanding, especially for colour-blind individuals. Labels and Legends: Clear labelling of axes, data points, and legends is necessary for interpretation. Ambiguous labels can confuse the audience. Simplicity vs. Complexity: A balance must be struck between a clean design and the inclusion of necessary details. Overly complex visuals can detract from the key message. Inputs Used in Preparing Graphs and Visuals: Statistical Techniques Methodology: The statistical methods used to process the data (e.g., averages, trends) should be sound. Misapplication of statistical techniques can lead to incorrect conclusions. Handling Outliers: Decisions on how to deal with outliers (include, exclude, or adjust) can significantly impact the visual’s accuracy and interpretation. Inputs Used in Preparing Graphs and Visuals: Contextual Information Background Data: Providing context around the data, such as historical trends or relevant benchmarks, enhances interpretation and understanding. Audience Awareness: Understanding the audience’s level of expertise is vital for tailoring visuals. What may be clear to an expert could be confusing to a layperson. The inputs used in preparing graphs and visuals are critical to their effectiveness. Ensuring data quality, appropriate selection, thoughtful design, and sound statistical techniques enhances the clarity and impact of the visualizations. A critical evaluation of these inputs can help prevent misinterpretation and support more informed decision-making. Manipulation and Misinterpretation of Graphs: Scale manipulation Graphs can be powerful tools for visual communication, but they can also be manipulated or misinterpreted in ways that distort the underlying data. Changing axes scales: By altering the scale on the axes (e.g., starting from a non-zero point or using logarithmic scales), the visual impact of trends can be exaggerated or minimized. For instance, a small change in a large value can appear dramatic if the scale is not properly set. Inconsistent Intervals: Using uneven intervals on the axes can mislead viewers about the true nature of the data changes. Manipulation and Misinterpretation of Graphs: Selective Data Presentation Cherry-Picking Data: Presenting only certain time periods or data points that support a specific narrative while omitting others can create a biased view. This selective reporting can lead to misconceptions about trends or relationships. Ignoring Context: Failing to provide background information or context (e.g., economic conditions) can mislead the audience about the significance of the data. Manipulation and Misinterpretation of Graphs: Misleading Graph Types Inappropriate Graph Selection: Using a graph type that does not suit the data can obscure meaning. For example, a pie chart may not effectively represent changes over time, while a line graph would be more appropriate. 3D Graphs: Adding unnecessary dimensions can distort perceptions of size and proportion, leading to confusion. Manipulation and Misinterpretation of Graphs: Data Manipulation Techniques Exaggerating Differences: By manipulating the visual elements (e.g., using different sizes or colours), significant differences between data points can be overstated or understated. Inconsistent Visual Elements: Using different styles (e.g., colour gradients or patterns) can create a false sense of variance, even when the actual data differences are minimal. Manipulation and Misinterpretation of Graphs: Ambiguous Labels and Legends Vague Labels: Using unclear or misleading labels can confuse the audience. For example, vague titles can fail to convey what the data represents, leading to misinterpretation. Omitting Legends: Not providing a legend or key can make it difficult for viewers to understand what different colours or symbols represent. Manipulation and Misinterpretation of Graphs: Visual Overload Cluttered Designs: Overloading a graph with too much information (multiple datasets, excessive colours, or unnecessary embellishments) can distract from the main message and lead to misinterpretation. Complexity: Using overly complex visuals may confuse the audience rather than clarify the data. Manipulation and Misinterpretation of Graphs: Confirmation Bias Preconceived Notions: Viewers may interpret graphs through the lens of their own biases or beliefs, leading to misinterpretations that reinforce existing viewpoints rather than objective analysis. Graphs can be powerful communicative tools, but they are susceptible to manipulation and misinterpretation. Awareness of these techniques and pitfalls is essential for both creators and consumers of data visuals. A critical approach to graph interpretation can help ensure that the insights drawn from data are accurate and meaningful. Data analysis Class example: Profit vs Debt R (Correlation Coefficient): The R value of 0.254 indicates a weak positive correlation between the dependent variable and the predictor (DebtEquity1). This means that as DebtEquity1 increases, the dependent variable tends to increase slightly, but the relationship is not strong. The R Square value of 0.064 indicates that approximately 6.4% of the variance in the dependent variable can be explained by DebtEquity1. This suggests that the model does not explain much of the variability in the dependent variable, implying that other factors might also play significant roles. Data analysis ANOVA Significance (Sig.):The p-value (Sig.) of 0.035 indicates the probability that the observed relationship is due to chance. Since this value is less than the common alpha level of 0.05, it suggests that the relationship between DebtEquity1 and ROAE1 is statistically significant. Data analysis Coefficients Constant (Intercept): 24.024. This is the expected value of ROAE1 when DebtEquity1 is zero. It represents the baseline level of the dependent variable. DebtEquity1 (B): 0.261. This coefficient indicates that for every one-unit increase in DebtEquity1, ROAE1 is expected to increase by 0.261 units, assuming all other variables are held constant. Beta: 0.254. This standardized coefficient indicates the strength and direction of the relationship between DebtEquity1 and ROAE1 in standard deviation units. It allows for comparison between different predictors if there were more in the model. Data analysis Multiple variables DebtEquity1 and InternGrowRateAT1:Pearson Correlation: 0.058. This indicates a very weak positive correlation, suggesting that changes in DebtEquity1 have little to no relationship with InternGrowRateAT1. Significance (Sig.): 0.637. This value is much greater than 0.05, indicating that the correlation is not statistically significant Data analysis Multiple variables: Regression R Square: The R Square value of 0.086 means that approximately 8.6% of the variance in the dependent variable can be explained by the model with these two predictors. While this is an improvement over the previous model (which had an R Square of 0.064), it still suggests that a large portion of the variance remains unexplained. The regression model including both InternGrowRateAT1 and DebtEquity1 shows a moderate correlation with the dependent variable, but the explanatory power remains low. Data analysis Multiple variables Regression Significance (Sig.): The p-value (Sig.) of 0.052 indicates the probability that the observed relationship is due to chance. Since this value is slightly above the common alpha level of 0.05, it suggests that the overall model is not statistically significant at the 5% level, but it is close to being significant. The ANOVA results indicate that the regression model with InternGrowRateAT1 and DebtEquity1 does not achieve conventional statistical significance at the 0.05 level (p = 0.052), suggesting that these predictors may not explain a meaningful portion of the variance in ROAE1 Data analysis Multiple variables Regression Unstandardized Coefficients: Constant (Intercept): 28.141. This is the expected value of ROAE1 when both predictors are zero. It represents the baseline level of ROAE1. DebtEquity1 (B): 0.270. This indicates that for every one-unit increase in DebtEquity1, ROAE1 is expected to increase by 0.270 units, holding InternGrowRateAT1 constant. InternGrowRateAT1 (B): -0.150. This indicates that for every one-unit increase in InternGrowRateAT1, ROAE1 is expected to decrease by 0.150 units, holding DebtEquity1 constant. Data analysis Multiple variables Regression Standardized Coefficients (Beta): DebtEquity1 (Beta): 0.262. This suggests that DebtEquity1 has a moderate positive influence on ROAE1 relative to other variables if there were more in the model. InternGrowRateAT1 (Beta): -0.146. This indicates a negative influence of InternGrowRateAT1 on ROAE1, but it is weaker compared to the effect of DebtEquity1.