DAT320 Basic Forecasting PDF
Document Details
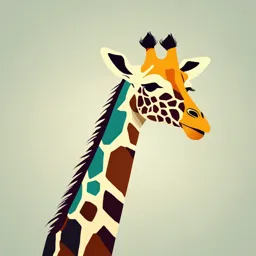
Uploaded by WittyAloe
Norwegian University of Life Sciences
2024
Norwegian University of Life Sciences
Kristian Hovde Liland
Tags
Summary
This document from the Norwegian University of Life Sciences, Autumn 2024, discusses various forecasting methods in a lecture format, including basics and more complex models. Topics covered include baseline models and time series analysis, and an overview of statistical forecasting models.
Full Transcript
DAT320: Forecasting Basic concepts Kristian Hovde Liland [email protected] Autumn 2024 Norwegian University of Life Sciences Forecasting: problem setup Baseline models Experimental setup 1 Norwegian University of Life Sciences Forec...
DAT320: Forecasting Basic concepts Kristian Hovde Liland [email protected] Autumn 2024 Norwegian University of Life Sciences Forecasting: problem setup Baseline models Experimental setup 1 Norwegian University of Life Sciences Forecasting I prediction model I target variable: future values xt+1 , xt+2 ,... I predictors: present and past values (history) xt , xt−1 ,... 2 Norwegian University of Life Sciences Forecasting I prediction horizon = maximum number of time steps h to predict ahead I in general: the more steps ahead, the more uncertain Single-step ahead Multi-step ahead I target variable xt+1 I target variable xt+h , h > 1 3 Norwegian University of Life Sciences Uni- and multivariate time series I which information should be used for predictors? I history / present state of (xt )t∈T I history / present state of other variables (exogeneous variables, covariates) (zt )t∈T Univariate forecasting Univariate forecasting with covariates Multivariate forecasting I predictors xs , s ≤ t I predictor xs and zs , I predictors xs , s ≤ t I target variable s≤t I target variable xt+1 ,... , xt+h I target variable xt+1 ,... , xt+h xt+1 ,... , xt+h 4 Norwegian University of Life Sciences Baseline models I 4 baseline models should be evaluated as minimum benchmarks for any more complex forecasting models I average method I drift method I naïve method I seasonal naïve method I Any forecasting model has to beat these 4! I [Hyndman and Athanasopoulos, 2021, ch. 5.2] 5 Norwegian University of Life Sciences Average method I given x1 ,... , xt , estimate future time point t + h, h > 0, by average over history, t 1X x̂t+h = xs t s=1 I → same as global missing value replacement xt x̂t+2 x̂t+1 6 Norwegian University of Life Sciences Drift method I given x1 ,... , xt , estimate future time point t + h, h > 0, by the last observed value plus average drift (trend), xt − x1 x̂t+h = xt + h t−1 xt x̂ x̂t+2 t+1 7 Norwegian University of Life Sciences Average and drift method (a) Average method (b) Drift method Figure 1: Baseline forecasts – passenger data 8 Norwegian University of Life Sciences Naïve method I given x1 ,... , xt , estimate future time point t + h, h > 0, by the last observed value, x̂t+h = xt I → last observation carried forward (LOCF) xt x̂t+2 x̂t+1 9 Norwegian University of Life Sciences Seasonal naïve method I given x1 ,... , xt and a period of p, estimate future time point t + h, p ≥ h > 0, by the same value from the last period, x̂t+h = xt+h−p(k+1) , where p is the seasonal period and k = b h−1 p c x̂t+1 xt x̂t+2 10 Norwegian University of Life Sciences Naïve and seasonal naïve method (a) Naïve method (b) Seasonal naïve method Figure 2: Baseline forecasts 11 Norwegian University of Life Sciences Baseline models library ( forecast ) library ( datasets ) data ( " AirPassengers " ) # average method ( mean f o r e c a s t ) mod _ avg