Data Analysis for Marketing Decisions (PDF)
Document Details
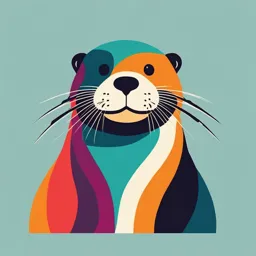
Uploaded by AwesomeCarnelian4810
Copenhagen Business School, Vienna University of Economics and Business
Georgios Halkias
Tags
Summary
This document presents a lecture or presentation on statistical inference in marketing decisions, using practical examples to illustrate parameter estimation and hypothesis formulation. The document illustrates how to use probability distribution, confidence intervals, and different types of hypothesis to analyze sample data for marketing decisions. This document is intended for a postgraduate audience and is aligned with marketing and business contexts.
Full Transcript
Data Analysis for Marketing Decisions Session 2: Statistical inference I: Parameter estimation and hypothesis formulation Priv.-Doz. Dr. Georgios Halkias Associate Professor, Department of Marketing | Cop...
Data Analysis for Marketing Decisions Session 2: Statistical inference I: Parameter estimation and hypothesis formulation Priv.-Doz. Dr. Georgios Halkias Associate Professor, Department of Marketing | Copenhagen Business School Adjunct Professor, Department of Marketing and International Business | University of Vienna E: [email protected] Priv.-Doz. Dr. Georgios Halkias © 1/26 Statistical inference …we analyze sample data to make inferences about the population. derive estimates test hypotheses Specific population characteristics (parameters) Contrasts & comparisons Associations & relationships Fit the model (statistical testing) Statistical model ► Statistically model the hypothesis using a certain test statistic ► Get a random/representative sample ► Summarize sample data with your test statistic ► Use probability distribution of test statistics to make inferences about the population Priv.-Doz. Dr. Georgios Halkias © 2 2/26 1 Probability distribution 2 Estimating population parameters 3 Hypothesis formulation Priv.-Doz. Dr. Georgios Halkias © 3 3/26 Probability (frequency) distribution …a function that describes how likely different values of a random variable are. The possible values of this variable are based on the underlying probability distribution. No mask Mask 0.95 * Refers to discrete probabilities – 0.59 continuous probability distributions follow the same reasoning 0.41 0.05 Number of times got sick since COVID19 outbreak (n = 100)* Priv.-Doz. Dr. Georgios Halkias © 4 4/26 Probability distribution Normal and standard normal distribution Every normal distribution (regardless of what the variable represents…) has these properties! 68–95–99 empirical rule A normal distribution can be standardized: population sample mean = 0 mean = median = mode SD = 1 symmetric Priv.-Doz. Dr. Georgios Halkias © 5 5/26 Example… How likely is it that students score less than 58? 58 points Mean = 55pts SD = 5pts n = 60 40 45 50 55 60 65 70 points 50.00% (half below the mean) + 22.57% 72.57% ► ~ a 73% probability that any observed grade is less than 58pts (or z < 0.6). ► ~ 73% of the grades will be below 58pts. ► ~ 27% of grades is 58pts or higher. 0.6 Priv.-Doz. Dr. Georgios Halkias © 7 7/26 Statistical inference Wait a minute! I operate on a sample, not the population. How confident can I be that what I find applies to the whole population??? Sample statistic = known (based on the empirical sample) Population parameter = unknown Using a sample always implies sampling error. Given a level of confidence, this error can be calculated and allow us to draw inferences. Priv.-Doz. Dr. Georgios Halkias © 8 8/26 1 Probability distribution 2 Estimating population parameters 3 Hypothesis formulation Priv.-Doz. Dr. Georgios Halkias © 9 9/26 Parameter estimation We collect data to get a sample statistic (e.g., mean, proportion, etc.) with which we estimate the corresponding population parameter. Population (N = 10): = 21.20 (nobody knows this) Standard deviation of each sample: SA = 2.309 19 SB = 1.528 SC = 1.528 18 20 19 22 18 21 18 Sample size: n = 3 22 19 Priv.-Doz. Dr. Georgios Halkias © 1010/26 Sampling Error (Margin of Error) Population parameter = Sample statistic ± Sampling error (b) known unknown (a) ► Population parameter fixed, but variation of sample statistic(s) ► Sampling error may overestimate/underestimate parameters Range of values due to sampling error can be theoretically estimated using: (a) the variability of the sample statistic (e.g., mean) in the population, i.e., the Standard Error (SE) (b) the critical value in the probability distribution that corresponds to our confidence level/error rate Priv.-Doz. Dr. Georgios Halkias © 11 11/26 Parameter estimation – Sampling distribution & Standard Error Standard deviation (S): variability of observations from the sample mean. Standard error (σx̅): variability of means across samples drawn from the same population = standard deviation of the sampling distribution. If the population is normally distributed, the sampling distribution of the mean is also normally distributed with a mean equal to the population mean (e.g., μ =3) For large enough sample sizes (>30; see, CLT), regardless of the population distribution, the sampling distribution of the statistic will be approximately normally distributed. So, the standard deviation of the sampling distribution (Std. Error) can also be approximated: 𝑠 𝜎𝑋ሜ = 𝑛 Priv.-Doz. Dr. Georgios Halkias © 12 12/26 Parameter estimation – Confidence Level Confidence level: How many times are estimations expected to capture the true parameter? → frequency (%) of all possible sample estimations that are expected to include the true population parameter. Specifying a confidence level, also determines how much “risk” (α=alpha) you are willing to take (“likelihood that your estimation is wrong”) → Confidence level = 1-α Typical “risk” levels, α = 5%, 1%, 0.1% The risk level α or Type I error or error rate or significance level is the opposite of confidence level. If we want to estimate with a 95% confidence level, we also allow 5% of “wrong estimations.” Confidence and Significance level (α=alpha) indicate how “strict” we are and specify critical (cut-off) values on the probability distribution Priv.-Doz. Dr. Georgios Halkias © 15 15/26 Parameter estimation Critical values and Conf./Sig. level 2.5% 95% 2.5% No expectations of directionality → 2-tails Z-score = -1.96 Z-score = +1.96 Priv.-Doz. Dr. Georgios Halkias © 16 16/26 Parameter estimation – Confidence Interval Range of values due to sampling error can be theoretically estimated using: (a) the variability of our sample statistic (e.g., mean), that is, the Standard Error (SE) (b) the critical value in the prob. distribution that corresponds to our confidence level Critical value for 95% confidence (α/2 = 5% ) Standard error of statistic We end up with a lower and upper limit for our statistic → the Confidence Interval 95% confidence interval: If you get repeated samples, for 95% of them the confidence intervals will contain the true value of the population mean → we can be 95% confident that this range of values contains the true population parameter Priv.-Doz. Dr. Georgios Halkias © 18 18/26 Parameter estimation – Confidence Interval True population parameter Priv.-Doz. Dr. Georgios Halkias © 19 19/26 Parameter estimation Critical values and Confidence Intervals Sample A μ=20.67 ± 1.96×(2.309/1.732) The CIs around the sample mean account for 18.06 < μ < 23.28 the “uncertainty” of our estimation Sample B μ=19.33 ± 1.96×(1.528/1.732) Q1. Does the confidence interval include 17.60 < μ < 21.06 the population value (i.e., 21.20)? Sample C μ=19.66 ± 1.96×(1.528/1.732) I’m using the 2-tail critical value (Za/2) because the true value may be “higher or lower” (two-tailed test) 17.93 < μ < 21.39 Priv.-Doz. Dr. Georgios Halkias © 20 20/26 1 Probability distribution 2 Estimating population parameters 3 Hypothesis formulation Priv.-Doz. Dr. Georgios Halkias © 22 22/26 What is a “hypothesis”? Hypothesis is a prediction about the state of the world. It is a scientific statement that must be able to be empirically disproved, i.e., be falsifiable –testable and able to be disconfirmed based on evidence. …translated into relationships between variables that can be empirically measured (in a valid and reliable manner). Hypothesis: Being in a bad mood makes people spend more money. Independent variable (predictor variable) → mood (good/bad) Dependent variable (outcome/criterion variable) → money spending Which of the following statements represents a hypothesis and which one doesn’t? ► Small and large companies evoke different levels of consumer trust. ► Psychotherapy leads to improved well-being. ► Most people who commit suicide they regret doing so. ► If one had studied medicine, they would make more money. ► Dreaming duration for males is longer than that for females. Priv.-Doz. Dr. Georgios Halkias © 23/26 Types of hypotheses Directional hypotheses relates to 1-tail testing The researcher indicates a priori the direction (either positive or negative) of the expected relationship. e.g., Global brands evoke higher perception of quality than local brands. Advertising creativity increases consumer attitudes. Non-directional (exploratory) hypotheses relates to 2-tail testing The researcher expects an effect but has no a priori expectation about the direction of the effect. e.g., Global and local brands evoke different perception of quality. Advertising creativity influences consumer attitudes. Perceived ≠ Perceived quality of global quality of local brands < brands > Perceive H1 (+) H2 (+) Quality Willingness brand perception to pay globalness Priv.-Doz. Dr. Georgios Halkias © 24/26 Types of hypotheses (…they come in pairs) Alternative hypothesis (H1): Our predictions/expectations of how things in the real world are. Usually, that there is an effect (e.g., a difference or a relationship) in the population. …each alternative hypothesis has a corresponding Null hypothesis (H0) which is the opposite of (it “nullifies”) H1 and usually states that no effect exists. The null (H0) together with the alternative hypothesis (H1) account for all potential outcomes regarding the relationship being studied. Example: H1: Heavy metal fans have above average IQ. H0: Heavy metal fans do not have above average IQ. Priv.-Doz. Dr. Georgios Halkias © 25/26 Types of hypotheses (…they come in pairs, but why?) We NEVER prove the alternative hypothesis using statistical testing, we ONLY collect evidence against the H0! Rejecting H0, doesn’t prove H1 (it merely maintains it). Failing to reject H0, doesn’t prove H0 (it merely maintains it). NHST considers the chances of observing our sample data (results), assuming that the null hypothesis is true. ► How likely is it to find 20 (out of 100) metalheads with IQ above average, if…? ► How likely is it to find 95 (out of 100) metalheads with IQ above average, if…? Hypothesis testing is based on the probability (p-value) of obtaining such sample data or more extreme if, hypothetically speaking, the null is true. Priv.-Doz. Dr. Georgios Halkias © 26 26/26 ANY QUESTIONS?