Thermal facial image analyses reveal quantitative hallmarks of aging and metabolic diseases (Cell Metabolism PDF)
Document Details
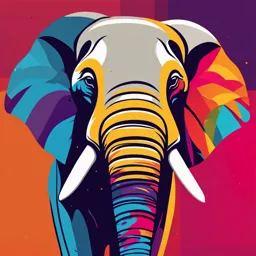
Uploaded by MagnanimousCosmos
Zhengqing Yu, Yong Zhou, Kehang Mao, etc.
Tags
Summary
This article from Cell Metabolism describes a new method for analyzing thermal facial images to predict age and detect metabolic diseases. Researchers collected and analyzed over 2,800 thermal facial images to develop a model called "ThermoFace".
Full Transcript
7/11/24, 2:47 PM Thermal facial image analyses reveal quantitative hallmarks of aging and metabolic diseases: Cell Metabolism Log in...
7/11/24, 2:47 PM Thermal facial image analyses reveal quantitative hallmarks of aging and metabolic diseases: Cell Metabolism Log in Search for... CLINICAL AND TRANSLATIONAL REPORT | VOLUME 36, ISSUE 7, P1482-1493.E7, JULY 02, 2024 Download Full Issue Thermal facial image analyses reveal quantitative hallmarks of aging and metabolic diseases Zhengqing Yu Yong Zhou 8 Kehang Mao 8... Hongxiao Liu Yi Wang Jing-Dong J. Han 8 9 Show all authors Show footnotesDOI: https://doi.org/10.1016/j.cmet.2024.05.012 PlumX Metrics Highlights Collected >2,800 thermal facial images and developed ThermoFace aging clock models ThermoFace is related to metabolic indicators, DNA repair, ATPase, and lipolysis ThermoFace aging rate increases with metabolic diseases and decreases with adequate sleep ThermoFace can rapidly assess aging and metabolic diseases and intervention Summary Although human core body temperature is known to decrease with age, the age dependency of facial temperature and its potential to indicate aging rate or aging-related diseases remains uncertain. Here, we collected thermal facial images of 2,811 Han Chinese individuals 20–90 years https://www.cell.com/cell-metabolism/fulltext/S1550-4131(24)00188-8 1/55 7/11/24, 2:47 PM Thermal facial image analyses reveal quantitative hallmarks of aging and metabolic diseases: Cell Metabolism old, developed the ThermoFace method to automatically process and analyze images, and then generated thermal age and disease prediction models. The ThermoFace deep learning model for thermal facial age has a mean absolute deviation of about 5 years in cross-validation and 5.18 years in an independent cohort. The difference between predicted and chronological age is highly associated with metabolic parameters, sleep time, and gene expression pathways like DNA repair, lipolysis, and ATPase in the blood transcriptome, and it is modifiable by exercise. Consistently, ThermoFace disease predictors forecast metabolic diseases like fatty liver with high accuracy (AUC > 0.80), with predicted disease probability correlated with metabolic parameters. Graphical abstract View Large Image | Download Hi-res image | Download (PPT) Keywords facial phenotype aging thermal infrared imaging quantitative analysis aging marker body temperature disease detection facial temperature aging clock metabolic disorder https://www.cell.com/cell-metabolism/fulltext/S1550-4131(24)00188-8 2/55 7/11/24, 2:47 PM Thermal facial image analyses reveal quantitative hallmarks of aging and metabolic diseases: Cell Metabolism Introduction Aging is a major risk factor for many complex human diseases.1,2 The rate of biological aging is heterogeneous across different individuals and, thus, many aging clocks have been developed to 3,4,5,6,7 monitor the aging rate at various levels, from molecular to phenotypical. Due to the complicated sample preparation and data acquisition steps needed for most molecular clocks, it is hard to provide real-time or on-the-fly aging rate assessment for an individual. How to obtain data rapidly and inexpensively, derive a clock accurately based on large cohorts, and make the clocks applicable to general populations is still a challenge. Body temperature, an often overlooked but important factor in aging and longevity, is a key homeostatic parameter that affects cell function and organism survival and is closely related to metabolism, protein denaturation, membrane fluidity, ion fluxes, and enzyme properties.8 In many endothermic species, individuals with lower body temperatures live longer and age more slowly than those with higher body temperatures.9 Cold temperature increases lifespan and delays aging in invertebrate organisms.10,11,12 Transgenic mice with reduced core body temperature have extended lifespans relative to normal body temperature mice.13 The decrease in body temperature under lifespan-extending calorie restriction (CR) is associated with metabolic rate.14 Metabolic rate is regulated at the organ (e.g., cardiovascular regulation rate) and cellular (e.g., mitochondrial capacity) level and is always influenced by ambient temperature.15 Interestingly, human core body temperature tends to decrease with age.16 High body temperature often implies high metabolic rates17,18 and can be induced by psychological and metabolic stress.19 For example, individuals with type 1 diabetes have been shown to have an impaired ability to dissipate heat, with impaired thermoregulation,20 and in acute human stroke there is a positive association between body temperature and initial stroke severity, infarct size, mortality, and outcome.21 Infrared temperature images of the human body have been tested for screening cardiovascular diseases (CVDs), with the best goodness of fit (adjusted r2) reaching 0.563 for abnormal total cholesterol and 0.568 for high- density lipoprotein (HDL) based on the average surface temperature from head to toe.22 The human face contains a wealth of information. We have demonstrated previously that three- dimensional (3D) facial images can be used to reliably assess the aging rate of an individual, especially when coupled with deep learning.23,24 The facial vascular and fat distribution is enriched and closely correlated with body surface temperature.25,26 Yet the current analyses of facial temperature are limited to a rough association between emotion and the total (or average) facial area temperature.27,28 Research on facial recognition by thermal imaging has gradually come into view, and some studies can roughly confirm the identity of individuals through facial recognition.29,30 Precise quantification of temperature distribution features of the human face with aging and various https://www.cell.com/cell-metabolism/fulltext/S1550-4131(24)00188-8 3/55 7/11/24, 2:47 PM Thermal facial image analyses reveal quantitative hallmarks of aging and metabolic diseases: Cell Metabolism diseases has not been explored, and it is still a mystery whether facial thermographic sub-regional features can be used as a marker of aging or diseases, as advocated by traditional Chinese medicine.31 Here, we collected thermal facial images of 2,811 individuals and developed the “ThermoFace” (TF) method that integrates a facial recognition and temperature extraction system by matching infrared and visible facial images and generates thermal-image-based aging clocks. We further identified lifestyle, health, and molecular features associated with accelerated and decelerated TF aging. The fast and convenient thermal infrared data acquisition and the accuracy of our models make TF an ideal monitoring and screening tool for healthy aging. Results Overview of thermal facial image data and the TF algorithm We collected 2,811 thermal facial images and parallel two-dimensional (2D) images, using the Fluke Ti401pro thermal image camera (details in STAR Methods), from Han Chinese in Jidong, China, in 3 batches from 2020 to 2022, with ages varying from 21 to 88 years in both sexes (female n = 1,339, male n = 1,472) (Figures 1A, S1A, and S1B; Table S1). Based on these data, we developed TF, a thermal infrared face mesh recognition and area division algorithm to align faces across infrared facial images (Figure 1A; STAR Methods). This is achieved by first identifying 54 feature landmarks on the face from the thermal facial images and 486 feature landmarks on corresponding 2D visible images. 2D visible image landmarks formed the regional division template, FaceMesh (STAR Methods); then, using shared feature landmarks in both thermal and visible images, we fit FaceMesh to each thermal facial image. To enable comparison of different thermal facial images, the FaceMesh is standardized to a grid of 897 triangles by Delaunay trianglution32 constructed from landmarks, which we termed a TF mesh (Figure 1A; STAR Methods). https://www.cell.com/cell-metabolism/fulltext/S1550-4131(24)00188-8 4/55 7/11/24, 2:47 PM Thermal facial image analyses reveal quantitative hallmarks of aging and metabolic diseases: Cell Metabolism Figure 1 ThermoFace and thermal facial aging patterns Show full caption (A) Schematic diagram of thermal facial image collection and the ThermoFace pipeline for data processing and analysis. (B) Fuzzy c-means clustering identified 4 distinct temporal patterns of temperature changes. The x axis represents six age stages, while the y axis represents log2-transformed, normalized intensity ratios in each stage. The facial areas of each cluster are displayed as white on gray background. See also Figures S1 and S2. View Large Image | Download Hi-res image | Download (PPT) To maximize the information retained in each TF mesh grid, we kept the max value (Max), min value (Min), and temperature standard deviation (SD) of each triangle (Figure 1A). Also, to avoid the noise caused by the environment temperature, we implemented a strict set of criteria (STAR Methods) in the sample collection process and, most importantly, applied Z score normalization to TF samples to https://www.cell.com/cell-metabolism/fulltext/S1550-4131(24)00188-8 5/55 7/11/24, 2:47 PM Thermal facial image analyses reveal quantitative hallmarks of aging and metabolic diseases: Cell Metabolism avoid the overall temperature difference and enable cross-sample comparisons; we term these normalized images TF, based on which all the following analyses are performed. The TF samples of batch2020 and batch2021 have a similar distribution on the principal component analysis (PCA) plot (Figure S1C). For a subset of 102 and 82 samples in females and males, respectively, who have thermal images in both years, the TF patterns are highly correlated between the 2 years (Spearman correlation [RCC] = 0.92; Figure S1D). For a subset of 41 subjects, 2–3 TF images from the same sample collected over 30 min to 2 h are clustered by PCA, with within-individual distances significantly smaller than between-individual distances (Figure S1E; p < 2.2e−16). These indicate that the TF patterns are relatively stable individual-wise and might be regarded as individual signatures. Aging-associated thermal facial pattern changes We first examined the average TF-normalized pattern in each decade. This shows an obvious decrease in the nose, cheeks, and eyebrows with age in both females and males. However, the decrease starts at around 50 years in females but around 60 years in males (Figure S1F). We then clustered all the triangles in the TF Max using a soft clustering algorithm33 (Figure 1B). Four clusters can be clearly observed: cluster 1, highlighting the lower nasolabial folds and lower cheeks, shows a near-linearly age-dependent increase in temperature; cluster 2, highlighting the nose and nasal- mouth region, shows an abrupt, age-dependent temperature drop starting from 50 years old; cluster 3, highlighting the forehead, eyes, and upper nasal labial folds, shows an opposite pattern to cluster 2; and cluster 4, highlighting the eye corners, shows the lowest temperature in middle age (Figure 1B). The TF Min patterns are similar to Max, but SD patterns are different (Figures S2A, S2B, S2D, and S2E). To verify the correlation between the average normalized temperature and chronological age, we plotted the average normalized temperature for the nose area (cluster 2) against age and observed that it indeed decreased with increasing age, particularly after 50 years old (Figure S2C). Average temperature of other clusters, even the age-increased cluster 1, also slightly drops with age, suggesting the age-dependent increases in the normalized TF are due to the relatively larger temperature drops in other facial areas (Figure S2C). Overall, our reconstructed average face profiles reveal previously unrecognized patterns of facial temperature change during aging. TF aging clocks To quantitatively detect aging-induced TF patterns, we performed partial least-squares regression (PLSR) (STAR Methods), which maximizes the variance of age-dependent changes when regressing based on age.34 Because of differences in TF aging between men and women (Figures S1F and S3A), we analyzed them separately. The cumulative variances in the TF data and https://www.cell.com/cell-metabolism/fulltext/S1550-4131(24)00188-8 6/55 7/11/24, 2:47 PM Thermal facial image analyses reveal quantitative hallmarks of aging and metabolic diseases: Cell Metabolism age explained by the first three components are 52.23% and 62.32% in females and males, respectively. The first three PLS components’ prediction is highly correlated with age (Pearson correlation coefficient [PCC] = 0.79 and 0.72 for males and females, respectively) (Figure 2A). Figure 2 ThermoFace aging clocks Show full caption (A) Heatmap of ThermoFace effects displaying efficient values of age-correlated PLS component 1 and the combination of the first 3 components for females and males. The effect coefficients were multiplied by 1,000 and are derived from the PLSR model using all samples used for training. Red and blue denote, respectively, higher and lower values for Max, Min, and SD of temperature. https://www.cell.com/cell-metabolism/fulltext/S1550-4131(24)00188-8 7/55 7/11/24, 2:47 PM Thermal facial image analyses reveal quantitative hallmarks of aging and metabolic diseases: Cell Metabolism (B) Correlation of ThermoFace age predicted by three different models with chronological age. Predictors are trained separately in females and males, using 10-fold cross-validation (CV) method (STAR Methods) to predict the age of the held-out samples. Trend line is loess fitted. Fast, normal, and slow aging groups are labeled with different colors, categorized by |cAgeDiffs| > 5 years. (C) The normalized Max temperature average profiles of the predicted fast agers, slow agers, and well- predicted female and male subjects in age groups older than 50 years. n indicates the number of subjects in each class of each age group. (D) Projections of ThermoFace PLSR cAgeDiff on a 3D facial image (left: color scale in arbitrary units). Red and blue denote, respectively, higher and lower values along x, y, and z axes (left). Synthesized female and male average profiles between −4 and +4 SD of loading values of PLS component 1 and 2 (right). See also Figures S3–S6. View Large Image | Download Hi-res image | Download (PPT) With the increase in PLS component 1, which is highly correlated with age (PCC = 0.66 and 0.53 for males and females, respectively), there is the large decrease in temperature of the nose and a small decrease in the cheeks (Figure 2A), as shown by cluster 2 in the TF clustering (Figure 1B). However, this pattern disappears when the top 3 PLS components are analyzed together due to the orthogonal changes of PLS2 and 3 to PLS1. Instead, the temperature increase and high variability around the eyes and mouth (nasolabial folds) with age remain the most common salient features revealed by PLS1 and the top 3 PLS components (Figure 2A), which is often associated with various diseases (see below). Using the first 13 components, which explained 82.42% and 85.49% of the total variance in the female and male TF data, respectively, we generated the TF PLSR clock. We also trained another linear model using elastic net (EN). Based on 10-fold cross-validation, PLSR has a slightly better performance in predicting chronological age for both females and males with a mean absolute deviation (MAD) of 6.58 (PCC = 0.80, p < 2.2e−16) and 6.45 years (PCC = 0.84, p < 2.2e−16), respectively, while EN possesses an MAD of 6.67 (PCC = 0.80, p < 2.2e−16) and 6.58 years (PCC = 0.80, p < 2.2e−16). We also constructed a TF-based convolutional neural network (CNN) model, using the same method we previously used to build a 3D facial image aging model.23 Based on 10-fold cross- validation, the CNN model is better than linear models, with MAD decreasing to 5.17 years in females and 5.08 years in males (PCCfemale = 0.90, p < 2.2e−16; PCCmale = 0.90, p < 2.2e−16) https://www.cell.com/cell-metabolism/fulltext/S1550-4131(24)00188-8 8/55 7/11/24, 2:47 PM Thermal facial image analyses reveal quantitative hallmarks of aging and metabolic diseases: Cell Metabolism (Figures 2B and S5A). All three TF-based clocks show that the high correlation between the TF predictions for the same subjects over 2 years and the high within-individual correlation confirm that TF can be utilized to estimate the aging status of individuals (Table S2). Saturation analysis shows that MAD and PCC both plateau at approximately 60% of the current sample size for TF PLSR and TF EN models (Figure S4A), but for the ThermoFace CNN (TF.CNN) model, only PCC plateaus; the MAD still shows a slight decrease when approaching 100% of sample size, indicating that more samples may still increase its accuracy, but not dramatically. Furthermore, an independent cohort of 75 healthy individuals (without major chronic diseases) was recruited at Beijing’s Guang’anmen Hospital (Beijing cohort, n = 75, age range [18,69]); adjusting for the differential age distribution (Figure S4B), the cAgeDiff from 3 TF-based clocks trained on the Jidong cohort exhibited similar performance on this independent cohort (TF PLSR model, MAD of 4.40, PCC = 0.91, p < 2.2e−16; TF EN model, MAD of 4.47, PCC = 0.92, p < 2.2e−16; TF.CNN model, MAD of 5.18, PCC = 0.86, p < 2.2e−16 ) (Figure S4C). Both analyses above suggest the saturation and stability of the models. However, none of the models are as accurate as the CNN models trained on the relatively smaller sample size of 3D facial images (n = 2,381) collected in the same cohort, which have MADs of 3.09 and 3.15 years for females and males, respectively (Figures S5B and S5C). For convenient comparison with the 3D facial clock in the subsequent analysis, we collectively refer to the three clocks derived from different methods as the “thermal facial clocks.” We also tested using different TF pattern clusters to generate cluster-specific models and found that, individually, they are all inferior to the whole face-based clocks (Table S3), implying that the information contained in the whole face is richer and more comprehensive for aging assessment. To explore the biological relevance of the thermal facial aging rate versus the 3D facial aging rate, we first utilize the difference between chronological age and predicted age from cross-validation models (AgeDiff) to identify outliers in the aging rate (STAR Methods). We find that, as in many other clock models, AgeDiff slightly overpredicts in young age and underpredicts in old age; to make all four AgeDiffs independent of chronological age, we corrected age effect on AgeDiff by loess to obtain cAgeDiff (Figure S5D; STAR Methods). The lack of correlation between the corrected cAgeDiff and chronological age makes the cAgeDiff comparable across different chronological ages (Figure S5D). Based on cAgeDiff, our TF PLSR clock detected faster and slower agers (|cAgeDiff| > 5 years) and, indeed, shows an aging and opposite-to-aging pattern, respectively (Figure 2C). The consistency of outliers given by the different models significantly overlapped (p < 0.05), especially between the three TF clock models, while the overlap of the TF.CNN model to the 3DFace CNN model (3D.CNN) model is larger than the two linear TF clocks (TF.PLSR and TF.EN) (Figure S4D; Table S4). Furthermore, the projection of TF cAgeDiff on the 3D face in the x axis direction shows an aging pattern of contraction of the upper half of the face and expansion of the https://www.cell.com/cell-metabolism/fulltext/S1550-4131(24)00188-8 9/55 7/11/24, 2:47 PM Thermal facial image analyses reveal quantitative hallmarks of aging and metabolic diseases: Cell Metabolism lower half of the face, which is consistent with the 3D facial aging pattern (Figures 2D, S6A, and S6B). A clear difference between men and women appears in the z axis, where women display a prominence in the cheek and nose regions and depression above the brow bone, while men show a depression in the overall face, except for the eye and periocular regions, which become more prominent during aging (Figure 2D). This is perhaps related to the different patterns of facial fatty tissue accumulation during the aging process in males and females. These correlations with 3D aging pattern further confirm that the TF age predictor recapitulates at least part of the 3D facial aging patterns. Health and lifestyle parameters associate with cAgeDiffs To compare aging differences, we compiled parameters—including routine anthropometry, blood pressure, biochemical test, and lifestyles—that were collected for over 50% of the participants. The parameters can be clustered into 3 groups (Figures S7B, S7C, and S8). One of these groups (cluster 1) is highly positively correlated with cAgeDiffs and includes blood platelets, white blood cells, and lymphocyte count in both sexes, and some other parameters are sex-specific, grouped with age and cAgeDiff (Figures S7B and S7C). Analyzing the relationship between each parameter and cAgeDiff in greater depth by multiple regression analysis (STAR Methods), we find that the 3 TF cAgeDiffs are all significantly positively correlated with metabolic disease indicators more than the 3D facial clock cAgeDiff (Figure 3A), such as BMI, fasting blood glucose, and apolipoprotein B (p < 0.05 and false discovery rate [FDR] < 0.1; Table S6), suggesting that metabolic states are better reflected on thermal facial patterns than in 3D structure. Figure thumbnail gr3 Figure 3 cAgeDiff heterogeneity difference and molecular pathways associated with cAgeDiff Show full caption (A and B) Association of cAgeDiff with anthropometric, blood pressure, and biochemical test (A) and lifestyles (B), determined by multi-regression coefficient with Benjamini-Hochberg corrected p value. The edge width and color intensity represent the strength of association. (C) Patterns corresponding to the first two principal components on the ThermoFace regressing to sleep time with PLSR. Sleep time is divided into 5 grades according to the length of time (STAR Methods). The panel shows the Max temperature value PLSR loading multiplied by 1,000. n refers to the number of samples used to generate the ThermoFace feature. (D) KEGG pathway enrichment scores (NES) for thermal cAgeDiff-associated pathways. ∗p < 0.05, ∗∗p < 0.01. GSEA enrichment of KEGG pathway over genes ranked by their expression RCC to each cAgeDiff across 57 https://www.cell.com/cell-metabolism/fulltext/S1550-4131(24)00188-8 10/55 7/11/24, 2:47 PM Thermal facial image analyses reveal quantitative hallmarks of aging and metabolic diseases: Cell Metabolism individuals. All four thermal cAgeDiffs must have significant associations to be included, enrichment scores for RNA-seq and 3D cAgeDiffs are shown for comparison. (E) Network among genes within the KEGG pathways correlated with thermal AgeDiffs determined by GSEA. The color of each edge represents the RCC value of the gene, with the average thermal cAgeDiff. Edges indicate the membership of a gene to a pathway. Each circle marks a gene complex (comprising at least 3 genes) identified through cellular component GO terms. (F) ThermoFace patterns of thermal cAgeDiff positively and negatively associated pathways. The panel shows the Max temperature PLSR first 3 components’ total loading values to each pathway’s Z score-normalized median gene expression values multiplied by 1,000 among 57 individuals (PBMC cohort). The red and blue bars above the images denote the positive and negative GSEA NES (>1.5 and 0.80) are hypertension in females (AUC = 0.817, accuracy = 0.782, specificity = 0.800, sensitivity = 0.723) and fatty liver in both males (AUC = 0.892, accuracy = 0.800, specificity = 0.765, sensitivity = 0.863) and females (AUC = 0.812, accuracy = 0.797, specificity = 0.898, sensitivity = 0.756) (Figures 4C, S16A, S17A, and S17C; Table S10). Saturation analysis conducted by randomly selecting data shows that AUC and predictor accuracy plateau after 60% or 80% of the sample size for hypertension, but not completely saturated for fatty liver, thus there is still room for improvement (Figure S16B), indicating that there is a great potential for using TF to detect these diseases. Reserving part of the data as an independent validation dataset, the models gave a similar predictive performance on this independent dataset as cross-validations (Figure S17B), demonstrating the stability and statistical power of the models. TF disease predictors also showed some ability to differentiate between diseases (Table S11). TF disease predictors reached better performance when separately predicted for each sex (Figures 4C and S17A), and facial patterns are clearer than predictors trained from both sexes (Figures 4B, S18A, and S18B). In addition, the effect of taking medication or not on disease predictors was negligible (Figure S18C). When the same method was used to examine the effectiveness of the 3D face in predicting diseases, it was found the 3D face predictor was inferior to the TF predictor, especially for the determination of hypertension (Figure S19). Although displaying a differential increase in temperature on cheeks, forehead, and peri-mouth regions, hypertension shows increased temperature in the eye area in both sexes and a relatively colder nose in males (Figure 4B), which are similar to the pattern of cAgeDiffs and aging, and also increased temperature on the cheeks, which is opposite to the pattern of cAgeDiffs and aging (compare to Figures 2A and 2C). Interestingly, these disease TF patterns are more similar to the inflammation-related molecular pathway expression patterns than cAgeDiff and aging (compare to Figure 3F), suggestive of chronic inflammation as a major contributor to the disease TF patterns. In TF regression prediction for systolic blood pressure (SBP) and diastolic blood pressure (DBP) (with a predicted to SBP PCC of 0.620, p < 2.2e−16 and predicted to DBP PCC of 0.573, p < 2.2e−16), the TF features of SBP and DBP have similar patterns of increased temperature on the cheeks and decreased temperature on the forehead area, https://www.cell.com/cell-metabolism/fulltext/S1550-4131(24)00188-8 14/55 7/11/24, 2:47 PM Thermal facial image analyses reveal quantitative hallmarks of aging and metabolic diseases: Cell Metabolism and both are similar to hypertension TF patterns (Figure S18A). However, unlike in hypertension, which has a temperature rise in the periocular region, the TF pattern of SBP and DBP exhibited lower temperatures in the eyes and periorbital regions, which might suggest the involvement of additional factors contributing the hypertension disease other than blood pressure itself (Figure S20). BIC-SKMeans43 clustering (Figure 4D) of the impact on TF of aging and cAgeDiff versus diseases and cAgeDiff-associated lifestyles (Figure S20) reveals that most diseases and smoking cluster with various cAgeDiffs in the orange cluster, which are similar to aging (red cluster) but less pronounced and opposite to the sleep time and yogurt intake cluster (green cluster). Specifically, region 1 is concentrated in the nose region, which shows a strong decrease in temperature with aging and weaker decrease with cAgeDiffs, smoking, and hypertension; region 2 contains the forehead center, outer eye corner regions, and chin, and an increase in temperature with aging but a decrease with cAgeDiffs, smoking, and diseases; region 3 contains side of forehead, temples, upper eye lids, nasolabial folds, and mouth, where both aging and cAgeDiffs strongly enhance the temperature, similar to hypertension, diabetes, and smoking; region 4 mainly includes eyeballs and under eye, where hypertension, fatty liver, diabetes, and smoking all strongly enhance the temperature, similar to aging and thermal cAgeDiff patterns, thus making it the most consistent aging and aging-acceleration pattern of all conditions examined (followed by the opposite in region 1); and region 5 includes the cheek area, where temperature weakly decreases with aging and cAgeDiffs but is strongly increased by hypertension, fatty liver, and diabetes, similar to 3D facial cAgeDiff (Figure 4D). As expected, sleep time and yogurt intake have the opposite patterns in region 1 and region 3 compared with age and cAgeDiff patterns. Additionally, in region 5, sleep time and yogurt intake both have the strongest temperature decrease on the cheeks, opposite to the strong temperature increase of hypertension and fatty liver (Figure 4D). These suggest a partial or disconcerted aging acceleration induced by these aging-related metabolic syndromes and smoking, which is more similar to aging acceleration than aging itself. Multi-regression models corrected by age and sex reveal that higher body weight, blood pressure (SBP and DBP), and BMI and lower HDL are the most influential physiological factors for disease prediction in patients with fatty liver and hypertension (true positives [TPs]) compared with age- matched healthy controls (true negatives [TNs]) (Figure 4E). Interestingly, healthy individuals predicted to have a disease (false positives [FPs]), or individuals with a disease but predicted to be healthy (false negatives [FNs]), often have the expected disease or health patterns of these physiological parameters (Figure 4E). Thus, the FPs and FNs of models can be partially explained by metabolic status of the subjects and, in turn, may guide disease prevention. Similar associations can be seen by the significant differential levels of these parameters when pairwise-comparing FP versus TN or TP versus FN (Figure S21). https://www.cell.com/cell-metabolism/fulltext/S1550-4131(24)00188-8 15/55 7/11/24, 2:47 PM Thermal facial image analyses reveal quantitative hallmarks of aging and metabolic diseases: Cell Metabolism We then asked whether the metabolic disease can mediate the lifestyle impact on TF aging rates (cAgeDiffs) or the other way around by causal inference test (CIT) (STAR Methods). As inferred by CIT analysis, diabetes and hypertension can both mediate the impact of various lifestyles on TF aging acceleration (Figure 4G) and be promoted by TF aging acceleration (Figure 4F), with most causal lifestyle factors shared between the two models, except adequate sleep, which seems to only counteract the diseases’ impact on TF aging acceleration (Figure 4G), but not the other way around (Figure 4F), which implies that adequate sleep can alleviate the effects on aging caused by these diseases, but not vice versa. Similar patterns can be found when separating individuals by sex and by age, specifically middle-aged and older individuals (Figure S22), suggesting the robustness of these associations. Exercise reduces thermal facial aging Jump training enhances cardiovascular health, effectively burns calories, provides full-body exercise, and improves coordination and agility.44,45 Previous research has demonstrated the benefits of jump rope training on improving overall health, including heart, bone, respiration, and metabolic health (https://runrepeat.com/benefits-of-jumping-rope).46 In order to investigate whether TF responds to anti-aging interventions, we established an exercise cohort at Hainan Medical University based on jump rope training, with >10 min and >800 jump times between 5:30 p.m. and 6:30 p.m. for 2 weeks (STAR Methods) (n = 30, age range [18,49] years, 7 in control, 23 in jump rope group; t test shows non-significant age distribution [p > 0.05]). All thermal images were taken at a calm state without any acute effect of physical activity. After 2 weeks of training, there was a significant decrease in TF PLSR cAgeDiff, with an average decrease of 5 years (Figure 4H; t test, p = 0.025) in the training group, or 5.5 years when compared with the controls after 2 weeks (p = 0.11), while a slight but insignificant increase of 0.5 years was observed in the control group. cAgeDiff of baseline between the control group and the jump rope group showed no significant difference (t test, p > 0.05). For EN and CNN clocks, comparing the before and after jump rope training in the training group, there is a decrease in the average cAgeDiff, but the decrease is statistically insignificant. However, we noticed that the control group had an opposite but insignificant change in cAgeDiff after 2 weeks, indicating a slight upward shift in baseline measured by these two clocks. Considering this, comparing the cAgeDiff between the jump rope training group and the control group after 2 weeks (column 4 versus 2), the EN cAgeDiff shows a significant decrease (p = 0.027) and the CNN cAgeDiff shows a marginally significant decrease (p = 0.071) (Figure S14). Visualization of baseline and the jump rope group’s mean facial temperatures after 2 weeks shows that the facial temperatures of the jump rope group (at a calm state) were overall higher than those of the non-exercise group (Figure 4I); the differential thermal pattern (p < 0.05) indicates that temperatures in the nose region of the jump rope exercise group were significantly https://www.cell.com/cell-metabolism/fulltext/S1550-4131(24)00188-8 16/55 7/11/24, 2:47 PM Thermal facial image analyses reveal quantitative hallmarks of aging and metabolic diseases: Cell Metabolism elevated in contrast to the age-dependent decrease in this region (cluster 2 in Figure 1B). This indicates the possibility of mediating thermal facial aging with something as simple as 2 weeks of daily jump rope exercise. Discussion In this study, we established that thermographic facial images can be used to estimate a thermal biological age and the extent or rate of aging, and they can promisingly predict several metabolic diseases, even in independent cohorts. In recent studies,47,48 distinctions between aging markers and aging rate markers have been proposed. Our data demonstrated that the differential TF AgeDiffs (aging rate) of people with the same chronological age are associated with their health and disease states and that a specific health intervention, such as jump rope, could decrease the TF AgeDiffs, indicating that TF ages can be used to assess biological age, placing them within the category of biomarkers of biological age. We developed a complete set of standardized extraction methods for infrared facial information based on infrared and matching 2D visible images of faces acquired by infrared thermography—TF. We quantified the changes of facial thermographic features and patterns with aging. Our results indicate that the 3D facial aging clock predicts the chronological age better than the TF aging clock (with a lower MAD), which may be due to the larger amount of information it contains. Each 3D facial image contains 7,200 data points, while TF currently contains 897 data points. Nonetheless, our further findings show that TF images have a wider association with physiological parameters than do 3D facial images. Our TF cAgeDiffs reveal similar molecular associations with inflammation/infection signatures and ribosome levels in the blood cells as other published aging clocks, but show stronger associations with metabolic health and diseases, consistent with the notion that body temperature distribution is a direct readout of cellular metabolism. Thus, it is not surprising that TF, but not 3D facial images, can well predict several metabolic diseases. Overall, despite lacking the same level of precision in estimating the chronological age as 3D facial images, TF is more related to an individual's health, hence biological age. The association of periocular temperature increases with various metabolic diseases points to a common feature of metabolic disorders. At least in the case of diabetes, ocular phenotypes are not only a manifestation of the disease but are also now fully established as an early diagnosis marker.49 It would be interesting to see whether the eye area—due to its intense vascularization and metabolic activities—is the most sensitive to fluctuations in metabolic status. Other than this common feature, different diseases display differential temperature changes on the cheeks, https://www.cell.com/cell-metabolism/fulltext/S1550-4131(24)00188-8 17/55 7/11/24, 2:47 PM Thermal facial image analyses reveal quantitative hallmarks of aging and metabolic diseases: Cell Metabolism forehead, and peri-mouth regions, suggesting the possibility of differentiating these diseases based on thermal facial patterns. Limitations of the study 50 Facial thermography has been shown to have a correlation with participant emotion, outdoor temperatures, and seasonal influences throughout the year, all of which might influence the result; as such, image collections were carried out in the morning in air-conditioned rooms with room temperature between 20°C and 25°C after participants enter a calm state of mind and body. Thus, in this study, we tried our best to avoid the influence of emotion and environment temperature changes, which by themselves are interesting topics to explore in the future. In this study, the thermal images of all but the Hainan jump rope cohort were taken in the early morning before breakfast, while those from the Hainan jump rope cohort were taken at random times between 9 a.m. and 12 p.m. or 3 and 6 p.m. Because circadian fluctuations were largely excluded when the clocks were built, it is unclear whether images taken in the afternoon or after meals may introduce confounding factors, and this awaits further investigation. Our current results indicate that thermal facial aging can be altered by a 2-week daily jump rope exercise; limitations in sample size and tracking duration necessitate further in-depth investigation into the long-term metabolic effects of sustained physical activity. Additionally, other than the jump rope cohort, which is from a subtropical region, this study was mainly based on the thermal facial imaging samples in the regions of Hebei and Beijing, which share similarities in terms of climate. Our current study only involves Han Chinese. To extend it to other ethnic groups, populations from different ethnic backgrounds and climates to need to be tested for the effectiveness of TF as an aging and health assessment across diverse human populations. STAR★Methods Key resources table https://www.cell.com/cell-metabolism/fulltext/S1550-4131(24)00188-8 18/55 7/11/24, 2:47 PM Thermal facial image analyses reveal quantitative hallmarks of aging and metabolic diseases: Cell Metabolism REAGENT or SOURCE IDENTIFIER RESOURCE Chemicals, peptides, and recombinant proteins Ficoll-Paque PLUS Cytiva 17144002 TRIzol Reagent Invitrogen 15596018CN Critical commercial assays DNF-471 STANDARD SENSITIVITY RNA AATI DNF-471-0500 ANALYSIS KIT Optimal Dual-mode BGI LR00R96 mRNA Library Prep Kit Qubit ssDNA Assay Kit Invitrogen Q10212 DNBSEQ-T7RS High- throughput MGI 1000028454; RRID:SCR_024847 Sequencing Set (FCL PE150) Open table in a new tab Resource availability Lead contact Further information and requests for reagents should be directed and will be fulfilled by the lead contact (Jing-Dong J. Han, [email protected]). Materials availability The study did not generate new unique reagents. Data and code availability https://www.cell.com/cell-metabolism/fulltext/S1550-4131(24)00188-8 19/55 7/11/24, 2:47 PM Thermal facial image analyses reveal quantitative hallmarks of aging and metabolic diseases: Cell Metabolism All mapped and batch corrected RNA-seq reads in this study are uploaded in CNCB (https://www.cncb.ac.cn/ or https://ngdc.cncb.ac.cn/bioproject/). Project: PRJCA025703, OMIX ID: OMIX006341. The package is available at https://github.com/JackieHanLab/ThermoFace. Any additional information required to reanalyze the data reported in this paper is available from the lead contact upon request. Experimental model and study participant details Participants for this study were recruited from multiple cohorts and were carried out according to the guidelines of the Declaration of Helsinki63: 1. Jidong Cohort: Three batches were recruited from 2020 to 2022 during annual physical examinations at the Staff Hospital of Jidong Oil-field and Kailuan Majiagou Hospital. The cohort comprises individuals of both sexes, ranging in age from 21 to 88 years. The cohort consists of 1,339 females and 1,472 males (refer to Figure 1A, S1A, and S1B; Table S1). 2. Beijing Cohort: 75 healthy individuals were recruited from Guang'anmen Hospital, with ages ranging from 18 to 69 years old (refer to Figure 1A). 3. PBMC Cohort: 57 healthy individuals were recruited from Guang'anmen Hospital, with ages ranging from 25 to 78 years old (refer to Figure 1A). 4. Exercise Cohort: 30 healthy individuals were recruited from Hainan Medical University, with ages ranging from 18 to 49 years old, 7 in control group, 23 in jump rope group (refer to Figure 1A). All participants included in this study are of Han Chinese ethnicity. Recruitment for all cohorts was conducted through voluntary advertisement, with participants required to be in a non-emergency state, self-reporting as healthy, and without major chronic diseases. Ethical approval for each cohort was obtained from respective hospitals or university. Method details Data acquisition https://www.cell.com/cell-metabolism/fulltext/S1550-4131(24)00188-8 20/55 7/11/24, 2:47 PM Thermal facial image analyses reveal quantitative hallmarks of aging and metabolic diseases: Cell Metabolism We collected Thermal Infrared facial images of the Han Chinese cohort at Tangshan in Heibei province (Jidong cohort) and Beijing (Beijing cohort and PBMC cohort) using the Fluke Ti401pro (640 x 480 resolution, Thermal sensitivity ≤0.075 °C at 30 °C target temp) (https://www.fluke- direct.com/). The Jidong cohort was recruited in Staff Hospital of Jidong oil-field and Kailuan Majiagou Hospital during their annual physical examination. The Beijing cohort and PBMC cohort were recruited in Guang'anmen Hospital. Ambient temperature (Te) at the time of image acquisition was recorded by the camera. As a requirement, volunteers sat straight and looked forward to the Thermal image device for 1 minute, without any facial expression, for the Thermal Infrared facial images to be taken. The Fluke system returned IS2-formatted images. Also, for each participant, baseline information including age, sex, education level, anthropometry, and lifestyle factors (for example, frequency of smoking, alcohol drinking, diet, and exercise) was collected from questionnaires, weight, height, and blood pressure was measured and routine blood tests were done. At the same time, we collected 3D facial images of the same volunteers using the 3dMDface System (https://3dmd.com/). Acquisition requirements are the same as for IR. The 3dMDface system returned OBJ-formatted 3D facial surfaces with point clouds and corresponding texture images. The detailed procedure of photography capture has been described in our previous work.23 The standard for collecting and inspecting results of infrared facial thermal imaging data included: 1. Once the infrared camera was set up and the imaging system was available, the camera was not moved again; 2. The imaging location was as spacious as possible and the test volunteer should not lean against the wall; 3. During the image acquisition, only the test volunteer was present; if there were multiple people, the data was invalid and the facial data of the test volunteer in the acquired image was more than 35% of the frame; 4. Maintained adequate indoor lighting and avoided taking pictures with backlight. If the external sunlight was bright, curtains were pulled. Visible light data without clear facial features of the test subject were considered invalid. 5. Ensured that the time fluctuation of each image acquisition was minimized. Recorded different collection time periods in the table using the 24-hour format, such as 2021.04.03.09:34.06. Maintained a constant temperature environment (between 20-25 °C with an air conditioner) and ensured that the test subject was not directly facing the air conditioner. Recorded the environmental temperature before each shoot and adjusted the imager's background temperature accordingly. 7. Prior to image collection, ensured that test volunteers refrained from intense activities or consuming beverages. Documented volunteers with fever or makeup. Allowed at least 15 minutes of buffer time for the test subject in cases of significant indoor-outdoor temperature differences. 8. Directed the test subject to maintain a neutral facial expression, looking straight into the lens without smiling, tilting, or opening https://www.cell.com/cell-metabolism/fulltext/S1550-4131(24)00188-8 21/55 7/11/24, 2:47 PM Thermal facial image analyses reveal quantitative hallmarks of aging and metabolic diseases: Cell Metabolism their mouth. Ensured consistent distance between each test subject and the lens. 9. Conducted laser calibration before each sampling batch, proceeding with image capture only after aligning the visible light and infrared contours of the object. 10. Activated the fill light option during data collection to prevent facial backlighting. 11. Immediately reviewed collected visible light images to ensure clear facial recognition. Retook images lacking clear facial features. 12. Ensured the test volunteer's forehead was fully exposed, providing a hair band for those with longer bangs to lift their hair. No glasses were allowed. When analyzing the data, we excluded onsite drinking, smoking or makeup to ensure that our result is not influenced by transient states. Also, facial images with obvious facial hair were excluded from further analyses. Exercise cohort data acquisition Volunteer of exercise cohort was recruited under the guidance of the standard for collecting and inspecting results of infrared facial thermal imaging data. When collecting exercise cohort data, the volunteers were randomly assigned to “Control group” and “Jump Rope Group”. While the control group was not required to do anything to alter their lifestyle, the jump rope group’s volunteers were required to adhere to the daily jump rope training of more than 10 minutes and no less than 800 jump times during the time between 5:30 pm and 6:30 pm for two weeks, and volunteers were encouraged to take the initiative to increase the training minutes as well as the capacity after adaptation. After two weeks, both the control and the jump rope group were recalled for thermal image taking. Data structure of IS2 The thermal infrared images were captured and stored in IS2 format. It could be opened by SmartView 4.3 software to view the image quality directly. The uncompressed folder contained IR.data and visible images, one of which was in high resolution. IR.data contained the raw data collected by the thermal infrared CCD, which needed to be corrected for Tc (Original temperature of CCD signal) because the transmittance and background temperature had an important effect on the accuracy of the thermal imaging camera. The corrected temperature Tb was Tb = e ∗ t ∗ Tc + (2 − e − t) ∗ Te https://www.cell.com/cell-metabolism/fulltext/S1550-4131(24)00188-8 22/55 7/11/24, 2:47 PM Thermal facial image analyses reveal quantitative hallmarks of aging and metabolic diseases: Cell Metabolism where Tc was original temperature of CCD signal, e was the Efficiency, t was the Transmission, and Te was the ambient temperature at the time of image shooting (°C), calculated in batch using MATLAB R2010b, and the temperature matrix was output in the range of 27-38 degrees Celsius and saved in PNG format. Thermal imaging noise removal Photonic noise exists in the photosensitive material of the IR detector and needed to be removed. Fluke Ti401pro captured images exist pretzel noise, which means the noise was small and had a clear boundary with the surrounding points. The image was filtered by the median filtering method, using cv2.medianBlur from the opencv2 (Python) package. Landmark recognition Detection of facial feature points (landmarks) and accurate standardization of facial regions provided the framework for the construction of facial region delineation for the face network. We used the manually labeled feature points dataset provided by the GitHub project Speaking Faces (based on python 3.6)52 and based on thermal face labeled feature point the functions in dlib package (based on python 3.6) dlib.simple_object_detector() and dlib.shape_predictor() was trained firstly to generate an SVM thermal face 54 feature points detection model that is used to detect 54 feature landmarks on our thermal facial images as shown in Figure 1A. Visible image facial raster network construction To limit information loss and retain most of the spatial structure, the region segmentation of the face was performed using the face mesh building part of Google Mediapipe.51 MediaPipe Face Mesh provided a face geometry segmentation method that can estimate 468 3D face feature locations in real time from 2D visible photos. Machine learning (ML) was used to infer the 3D surface geometry and only a 2D camera input is required. From MediaPipe Face Mesh we obtain a 468-point FaceMesh for each visible image. Mediapipe used mean absolute distance between the ground truth vertex locations and the predictions, normalized by interocular distance (IOD), defined as the distance between the eye centers (estimated as midpoints of eye corner connecting segments to avoid gaze direction dependence). The estimated IOD mean absolute distance error of FaceMesh was 2.56%. Infrared and visible feature points registration The construction of Face Mesh could only be based on the visible face images, the collection of facial infrared images and facial visible images Spatio-temporal overlap, in order to achieve infrared https://www.cell.com/cell-metabolism/fulltext/S1550-4131(24)00188-8 23/55 7/11/24, 2:47 PM Thermal facial image analyses reveal quantitative hallmarks of aging and metabolic diseases: Cell Metabolism facial region Face Mesh segmentation, we developed a method to match visible feature points and thermal infrared feature points as the following. First, the corresponding feature points were extracted in FaceMesh for thermal infrared detection, and 10 points in the thermal image were extracted (points 50, 49, 51, 52, 37, 40, 43, 46, 1, 17, 31, 34, 9, 13, 5) po={(x1o,y1o),(x2o,y2o),…, (x10o,y10o)}, corresponding to the points pc={(x1c,y1c),(x2c,y2c),…,(x10c,y10c)} in FaceMesh. Then the Procrustes transformation was applied to obtain the corresponding face mesh of the thermal face. Procrustes transformed by iteration such that: 2 arg min ∑ni=1 ∥s ⋅ R ⋅ pio T + t − pic ∥ s,θ,t cos θ − sin θ where, R =[ ]. The other points detected by FaceMesh are transformed sin θ cos θ correspondingly according to the obtained Procrustes transformation matrix to obtain the infrared light region feature points of ThermoFace. Delaunay mesh construction To cut the thermal imaging facial area, the Delaunay triangle network was constructed based on 468 feature points: a) Computed the enclosing box of the region and generated two hyper-triangles as the initial triangular mesh. Generated boundary points based on the boundary curve. b) Inserted the boundary points into the triangular mesh one by one. After each insertion step, a new Delaunay triangular mesh was obtained using the Bowyer-Watson algorithm. c) Removed the auxiliary vertices inserted in the first step (and also removed the triangles connected to them) to obtain a Delaunay triangulated mesh with respect to all boundary points (i.e., Delaunay triangulation of the boundary points). d) For the triangles inside the region, the center of gravity (centroid) was inserted into the triangle mesh according to certain conditions and adjusted according to the Bowyer-Watson algorithm to obtain a new Delaunay triangle profile. Until there were no more center of gravity points to be inserted, at which point the complete Delaunay triangulated mesh of the region was obtained. By the above procedure, 897 triangular regions of the thermal image were obtained, and the maximum value (TMax1,TMax2,…,TMaxn), minimum value(TMin1,TMin2,…,TMinn)and standard https://www.cell.com/cell-metabolism/fulltext/S1550-4131(24)00188-8 24/55 7/11/24, 2:47 PM Thermal facial image analyses reveal quantitative hallmarks of aging and metabolic diseases: Cell Metabolism deviation (TSD1,TSD2,…,TSDn)of the temperature were extracted by cutting the facial regions one by one using opencv2. Fuzzy C-means clustering for ThermoFace ThermoFace regions of samples separated into 6 age groups were grouped into different clusters using Mfuzz package in R with fuzzy c-means algorithm.33 Age groups were used as time stages. Visualization of ThermoFace projection The value of each area (e.g., maximum or minimum temperature, coefficients intensity) was linearly scaled to 10-255 by Vp = y (VT ) = αVT + b where VT was temperature value, Vp was Image corresponding color value, α was the scaling factor and b was the scaling intercept, and setting the original data 0 value was the midpoint. Then picking the color corresponding to Vp from the reference file ('jet', 'Rdbu', or 'seismic', from Matplotlib, python) to fill the standard ThermoFace surface mesh. MAD Definition In this study, the mean absolute deviation (MAD) was employed as the evaluation metric for the age model. MAD represented the average absolute difference between the predicted age and the actual age for all samples within a model, 1 MAD = n ∑ni=1 |xi − f (xi )| Where n was the number of samples, xi was the actual age value, and f (xi ) was the predicted age. Cross Validation To validate the prediction ability for age using ThermoFace matrix, we divided all our 2,811 samples into 10 groups randomly for 10-fold cross-validation. The model was trained with nine of the ten groups as a training set, a new linear mode was built based on training data and tested in the one not used for training data. MAD was the average MAD of each model for 10 cross-validation. https://www.cell.com/cell-metabolism/fulltext/S1550-4131(24)00188-8 25/55 7/11/24, 2:47 PM Thermal facial image analyses reveal quantitative hallmarks of aging and metabolic diseases: Cell Metabolism CNN model During each evaluation process, the model was trained with eight of the ten groups as a training set, hyperparameters were determined with one of the remaining groups as the validation set and the we predictive performance was tested on the last group as the test set. Finally, usedall ten models generated from the 10 cross-validation to test the datasets independently, and used the average performance level as the final prediction capability. Chronological age Birth dates were obtained by the Chinese-government-issued official Resident Identity Card. Age was rounded to the nearest year for model training. PLSR based age predictor The ‘pls’ package34 in R was used for PLS regression. Both chronological age and ThermoFace matrix (z-score normalized within samples) were combined into one matrix as the input. The 10-fold cross-validation (CV) method was applied to obtain the age prediction of each sample. MAD was calculated by the difference between predicted age and chronological age. Saturation analysis was conducted by randomly selecting a designated percentage of all the samples with the same age distribution of all samples and training the PLS model on such samples, and then was evaluated using both MAD and PCC. Components number used was determined by examining RMSE calculated in each PLSR model which was also verified by 10-fold CV. Elastic Net based age predictor The ‘glmnet’ package61 in R was used for Elastic Net regression. Input to train was as same as PLSR based age predictor. Saturation analysis was conducted as well. Best alpha and lambda parameters were determined by EN model using all the samples as training data and applied to CV models. Preparation of ThermoFace faces for deep learning The affine transformation (Affine) was performed first. The equation was shown below, where the matrix composed of a,b,c,d was the rotation matrix, tx,ty was the translation transformation, x,y were the matrix elements before the transformation, and xt,yt was the matrix elements after the transformation. [ ]=[ ][ ]+[ ] xt a b x tx yt c d y ty https://www.cell.com/cell-metabolism/fulltext/S1550-4131(24)00188-8 26/55 7/11/24, 2:47 PM Thermal facial image analyses reveal quantitative hallmarks of aging and metabolic diseases: Cell Metabolism The affine transformation was applied to the image using the OpenCV.warpAffine function. Since warpAffine could only perform rectangular transformations, it found the outer minimum rectangular border in each face mesh triangle, cropped the rectangular area from the source image, and applied the affine transform to the cropped image to obtain the output image. To create the output triangle mask, which preserved the color of all pixels inside the triangle, face mesh standard surface mesh map as input to ‘affine’ for it to transform all the samples corresponding to the triangles and the temperature in their regions, match the corresponding regions of the face mesh template. Repeated the same number of times as the number of triangles to obtain the normalized facial temperature distribution results independent of the facial structure morphology. The resulting images were cut according to the top and bottom boundary points and subsequently resized to 224 × 224 pixels before using deep learning. Data augmentation During training of deep CNN models, we flipped all samples from left to right, which could increase the amount of training data. Deep CNNs We combined 3 CNN architectures, GoogleNet, VGG and ResNet,64,65,66 in Python to predict age. For all three CNNs, we used one node as the last layer for regression. Ensemble of deep CNNs We used an ensemble of three CNNs, GoogleNet, VGG with 16 layers and ResNet with 50 layers to predict age. We trained and generated the results of the three networks independently, and generated an ensemble by taking their average, with the formula y = (GoogleNet + VGG + ResNet) /3 where GoogleNet, VGG and ResNet represented the ages predicted by these three CNNs, respectively, and y represented the ensemble predicted age. https://www.cell.com/cell-metabolism/fulltext/S1550-4131(24)00188-8 27/55 7/11/24, 2:47 PM Thermal facial image analyses reveal quantitative hallmarks of aging and metabolic diseases: Cell Metabolism Age prediction using 3D facial images with CNN model The prediction of age with CNN had been described in detail in previous work.23 AgeDiff correction To study age-independent associations of AgeDiff, we corrected AgeDiff by fitting to a polynomial model to age as follows, with span 0.8 and degree 2: cAgeDiff = AgeDiff − loess (AgeDiff ∼ Age) cAgeDiffs association with health parameters cAgeDiffs Pearson’s correlation coefficient and corresponding p-values to each health parameter were calculated. Benjamini–Hochberg correction was used for FDR calculation. R package ‘pheatmap’ was used for hierarchical clustering of correlations. Correlation between cAgeDiff SD and chronological age A non-overlapping sliding-window approach was used to study the relationship between cAgeDiff SD and age. Samples were sorted by age, and then a non-overlapping sliding window with bin size 25 was used to group samples and calculate mean of age and SD of cAgeDiffs in each sliding window. Amplification, normalization, and sequencing of RNA Each participant had 10 ml of total fasting blood drawn in the early morning. Venous blood samples in EDTA-K3 anticoagulated tubes were obtained from the subjects. Peripheral blood mononuclear cells (PBMCs) were isolated by Ficoll density gradient centrifugation (Ficoll-Paque PLUS, Cytiva). RNA was extracted using TRIzol (Invitrogen), following the manufacturer's instructions. The DNF- 471 STANDARD SENSITIVITY RNA ANALYSIS KIT was used to prepare samples with RNA integrity numbers (RIN) greater than 8 before they were sequenced on a MGIDNBSEQ-T7 device in accordance with the manufacturer's recommendations. Using STAR(version 2.4.0), paired-end reads (126 bp) were mapped to the GRCh38 reference human genome.54 RNA normalization Only reads with a unique match to the reference genome were kept. GENCODE v43 annotation was used to quantify the protein-coding genes. Cufflinks carried out the quantification and https://www.cell.com/cell-metabolism/fulltext/S1550-4131(24)00188-8 28/55 7/11/24, 2:47 PM Thermal facial image analyses reveal quantitative hallmarks of aging and metabolic diseases: Cell Metabolism normalization.67 Ribominus RNA-seq of the PBMC samples The raw clean data was first aligned to GRCh38 genome using Hisat2 (version 2.2.1)59 with the exclusion of reads that are flagged as poor quality based on the QSEQ filter. Fragment counting and gene annotation were done by using featureCounts (version 2.0.3)58 against GENCODE v43. Read counts were then transformed into transcripts per million (TPM). Then ComBat function from the ‘SVA’ R package68 was used to mitigate any batch-related discrepancies between two batches. KEGG pathway association enrichment analysis We first filtered the transcript expression matrix in TPM (transcripts per million) to retain only transcripts with log(TPM+1)>1 in at least 3 samples and then ranked the transcripts based on the Spearman correlation coefficient (RCC) between cAgediff and the log(TPM+1) values of transcripts. ResKEGG function from the clusterprofiler package in R55 was used to perform KEGG pathway enrichment analysis on the filtered transcripts. Only pathways significantly associated with 3 thermal cAgediffs and the average of them (p-value < 0.05) were retained as significantly associated with thermal cAgeDiffs, which were further corrected for age within the PBMC cohort to ensure their independence to age. SleepTime grading Sleep time was divided into 5 grades according to the length of time. Grade 1-5 represent for sleep time less than 4 hours, 4∼5.9 hours, 6∼6.9 hours, 7∼7.9 hours and 8∼9.9 hours, respectively. Yogurt intake grading Yogurt intake habit was divided into 4 grades according to frequency. Grade 1-4 represented for hardly, occasionally, often and almost daily, respectively. PLS-DA disease prediction ThermoFace Matrix data and PLS-DA in ‘mixOmics’ R package were used for constructing disease predictor. The classification label of the disease was based on the clinically diagnosed disease from the questionnaire, with the classification of mild and severe fatty liver based on the ultrasound findings of "expected mild fatty liver" and "expected severe fatty liver", respectively. The corresponding data for the healthy population were those who did not have hypertension, diabetes, liver cyst, fatty liver, cerebrovascular disease and cardiovascular disease, and their age distribution is the same with the disease population. Similarly, we split the data 10 folds, cross-validate, and https://www.cell.com/cell-metabolism/fulltext/S1550-4131(24)00188-8 29/55 7/11/24, 2:47 PM Thermal facial image analyses reveal quantitative hallmarks of aging and metabolic diseases: Cell Metabolism took the data as a test set of results to represent the results given by the disease predictor to draw ROC curves and confidence interval tests. The classification performance of the PLS-DA model was evaluated by the perf function using 5-fold cross-validation with 10 repetitions. The number of repetitions was necessary to ensure a good estimate of the classification error rate. Based on the max.dist performance results, we could decide the ncomp(component number) value of the final PLS model. Testing the effect of medication taking on predictors To test if medicine intake would influence the accuracy of ThermoFace disease detector, we applied roc.test in R package ‘pROC’. Patients with and without medicine were separated into two groups, with the same age distribution of healthy control to redraw ROC curve. The curves of the same disease with or without medicine taking were combined to test for the significance of difference between disease and control. To calculate corresponding P values, 'bootstrap' method was used with boot.n=200. Projection of features on the ThermoFace mesh and average 3D facial image With the ‘pls’ package in R, the disease or the ThermoFace PLSR cAgeDiff was treated as the dependent variable, while the ThermoFace matrices or 3D facial images were treated as independent variables for PLS regression. The loadings of the first two components weighed by the score of each component were combined and projected to the standard surface mesh feature map or average 3D facial image synthesized from the whole cohort. Questionnaire parameters filter We collected medical examination data and questionnaires of lifestyles. In Figures S9 and S10, we first filtered all parameters for all samples, filtering out parameters with null values in 30% of the samples, followed by filtering the samples with null values in 20% of the parameters, and finally comparing the parameter differences for each row of the remaining samples, the parameters with samples with a difference in parameters fewer than 10% of total samples were filtered out. 432 male and 546 female samples remained. Because some questionnaire information is missing for Batch2022, we had to split the data into two, in Figures S7B and S7C, the data from Batch2020 and Batch2021 were used for filtering, but in Figure S8, only the data from Batch2022 were used, so differences exist in Figures S7B, S7C, and S8 parameters. 560 males and 173 females from Batch2022 remained in Batch2022 in Figure S8. Lifestyle bias comparison of predicted patient groups https://www.cell.com/cell-metabolism/fulltext/S1550-4131(24)00188-8 30/55 7/11/24, 2:47 PM Thermal facial image analyses reveal quantitative hallmarks of aging and metabolic diseases: Cell Metabolism Wilcox.test was used for pairwise comparison of the significance of the differences in lifestyle habits for the four predicted outcomes (DD, DH, HD, HH). Benjamini-Hochberg correction was used for FDR calculation. log2FC=log2(treatLifestyleFre)-log2(conLifestyleFre), treatLifestyleFre and conLifestyleFre were the mean of the treated and control, where treatLifestyleFre was the treated lifestyle frequencies, conLifestyleFre was the control lifestyle frequencies. Lifestyle–regulator–cAgeDiff causal-relationship inference The causal-inference test was adopted with the R package ‘cit’62. Details in our previous paper. A causal inference met the following four criteria: (1) lifestyle and cAgeDiff were correlated; (2) lifestyle was associated with regulator after adjusting for cAgeDiff; (3) regulator was associated with cAgeDiff after adjusting for lifestyle; and (4) lifestyle was independent of cAgeDiff after adjusting for regulator. To summarize the p-values of the four tests, the intersection-union test was used as the p- value for the whole causal-inference test. Significant results with p-value< 0.05 and FDR < 0.1 (calculated by the ‘cit’ package62 ) were retained. The network was visualized by Cytoscape (version 3.5.0). Datasource for Thermoface details ThermoFace was used for thermal facial image extraction and ThermoFace Matrix construction. SpeakingFaces (https://github.com/IS2AI/SpeakingFaces) was used for thermal face 56 feature points SVM predictor building. ImageNet (http://image-net.org/) was used for CNN pre-train. Thermal images, 3D images, and other personal identification sensitive meta data could not be publicized or shared according to our participant consent agreement. Quantification and statistical analysis Statistical analyses were performed with R version 4.1.1, using two-tailed Student’s t-tests or Wilcoxon tests as appropriate. Pearson correlation and Spearman rank-correlation coefficient were used to measure the correlations. All of the statistical tests performed were indicated in the figure legends. The data were presented as mean or individual points with box plots or violin plots that display the median and quartiles. Error bars represented standard error intervals around the mean, as specified in the figure legends. Acknowledgments This work was supported by grants from the National Natural Science Foundation of China (92374207, 92049302, 32088101, and 32330017) and Ministry of Science and Technology of the https://www.cell.com/cell-metabolism/fulltext/S1550-4131(24)00188-8 31/55