Descriptive Statistics PDF - University of Setif 1
Document Details
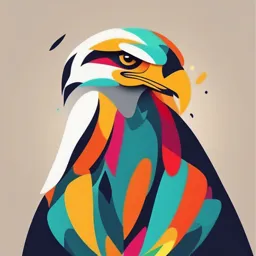
Uploaded by ImprovingAbstractArt
فرحات عباس سطيف 1
2024
Dr. Manel Rebbouh
Tags
Summary
This document is a lecture note on descriptive statistics for first semester 2024 at the University of Setif 1 in Algeria. It covers topics such as types of variables, frequency distributions, and applications in various fields. The document contains learning objectives and exercises.
Full Transcript
Descriptive Statistics Dr. Manel REBBOUH University of Setif 1 -Ferhat Abbas- Faculty of Economics, Commerce and Management Sciences Basic Education Department Email: [email protected] Engish Section First Semester 2024...
Descriptive Statistics Dr. Manel REBBOUH University of Setif 1 -Ferhat Abbas- Faculty of Economics, Commerce and Management Sciences Basic Education Department Email: [email protected] Engish Section First Semester 2024 Attribution - NonCommercial : http://creativecommons.org /licenses/by-nc/4.0/fr/ Table of contents Objectives 3 I - An Overview of Statistics 4 1. What is meant by statistics?............................................................................................ 5 1.1. The Concept of Statistics..................................................................................................................................... 6 1.2. The methodology of statistics/ Stages in Statistical Investigation....................................................... 7 1.3. Types of Statistics.................................................................................................................................................. 9 1.4. Statistics between computers and Ethical issues..................................................................................... 10 2. Applications of Statistics............................................................................................... 11 2.1. Social Sciences: Accounting, Finance and Economics.............................................................................. 12 2.2. Production & Quality Control.......................................................................................................................... 12 2.3. Information Systems.......................................................................................................................................... 13 II - Quiz : Check Your Progress 14 III - Quiz : Chooce one Correct Answer 15 IV - Types of Variable In Statistics 16 1. Quantitive Variables........................................................................................................ 16 1.1. Elements, Variables, and Observations........................................................................................................ 16 1.2. Continous Variable.............................................................................................................................................. 17 1.3. Discrete Variables................................................................................................................................................. 17 2. Categorical / Qualitative Variables.............................................................................. 18 2.1. Classification of Qualitative Variables........................................................................................................... 18 2.2. Data Sources.......................................................................................................................................................... 19 3. Frequency Distribution................................................................................................... 19 3.1. What is Frequency Distribution?..................................................................................................................... 20 3.2. Activity for More Clarification and fix the previous rules and terms.................................................. 22 Exercise solutions 23 Abbreviation 24 References 25 2 Dr. Manel REBBOUH Objectives The lectures in descriptive statistics aims to lay the foundation for understanding how to summarize, describe, and visualize data. Here are some specific objectives you might encounter: Introduce the concept of descriptive statistics: Explain what descriptive statistics are and how they differ from inferential statistics. Highlight the importance of descriptive statistics: Discuss how descriptive statistics are used in various fields and real-life situations. Define key data types: Differentiate between quantitative and qualitative data, and explore their measurement scales (nominal, ordinal, interval, ratio). Introduce basic data organization methods: Explain how to organize data into frequency tables and histograms to provide a preliminary overview. Introduce measures of central tendency: Define and explore the concepts of mean, median, and mode, and discuss their strengths and weaknesses in describing the "center" of the data. Introduce the concept of dispersion: Briefly introduce the idea of spread in the data and how it complements measures of central tendency. The first lecture might also include: Interactive activities: Engage students in activities that involve organizing and summarizing small datasets. Real-world examples: Showcase how descriptive statistics are used in different disciplines to analyze real-world problems. Preview of upcoming topics: Briefly mention some of the measures of dispersion and data visualization techniques that will be covered in subsequent lectures. By the end of the first lecture, students should have a basic understanding of the significance of descriptive statistics and be equipped to start exploring data organization, measures of central tendency, and the concept of data spread. Dr. Manel REBBOUH 3 An Overview of Statistics I 1. Introduction The first Chapter According to the Mindmap Module Have you ever stared at a mountain of data and felt overwhelmed? Numbers flying, graphs swirling, trends hiding in the shadows? Fear not, intrepid explorer! Descriptive statistics is your map and compass on this journey of discovery. Data surrounds us in every aspect of our lives. From weather patterns to customer reviews, numbers hold the key to understanding the world around us. But how do we make sense of this vast amount of information? Enter descriptive statistics, the cornerstone of data analysis. Descriptive statistics act as a translator, transforming raw data into a clear and concise story. Imagine a treasure chest overflowing with gems – each number a unique piece. Descriptive statistics help us organize these gems, identify the most common ones (the mode), the most valuable ones (the outliers), and where the majority lie (the mean and median). They don't just tell us what the data is, they reveal its essence – its central tendencies and how spread out the values are. Throughout this course, we'll work towards achieving the following objectives: Grasping the Fundamentals: We'll build a solid foundation in core statistical concepts like data collection, organization, and presentation. Understanding Descriptive Statistics: Learn how to summarize and describe data using measures of central tendency (mean, median, mode) and dispersion (variance, standard deviation). Unlocking the Power of Probability: Master the basics of probability theory, the foundation for making informed decisions and drawing inferences from data. Introduction to Inferential Statistics: We'll take a peek into inferential statistics, which allows us to draw conclusions about populations based on samples. Developing Critical Thinking Skills: Learn to analyze data critically and interpret results effectively, becoming a more informed decision-maker. Unlocking Applications: Explore the diverse applications of statistics in various fields, from making predictions in business to conducting research in science. By the end of this course, you'll be equipped with the essential skills to collect, analyze, and interpret data confidently. You'll see how statistics plays a crucial role in making informed decisions in your personal and professional life. So, buckle up and get ready to embark on this exciting adventure into the world of statistics! 4 Dr. Manel REBBOUH An Overview of Statistics What is Descriptive Statistics? 2. What is meant by statistics? Definition The word "statistics" is a term derived from two Latin words: Status means the status or Situation,Statos means the State. It can therefore be understood that statistics in a rudimentary definition reflects the state or status of the State in the language of the figures, but this concept remains simple and does not reflect the scientific reality of this field of knowledge. The tracker of the first signs of statistical science finds that they are due to very old times in primitive man for the transformation from a life of mobility to a life of stability. With this stability, the concept of occupation of the field, i.e. occupation of a plot of land and its consideration as a special area thereafter, has become interested in crossing the area, the number of fruitful trees in the field, the number of family cell members, the number of animals. All of this is expressed by a certain number of gravel. These are the same as the interests of the modern State but in a sophisticated manner where specialized offices and departments have been established to collect statistics in a country's various social and economic activities. For example, in Algeria the responsible is the National Statistical Office. The scientific signs of statistics as a theory emerged only from the 18th century, when researchers (mathematicians), such as : Gauss, Laplace and Bemouli, went towards statistical analysis and the creation of probable laws. It was not until the beginning of the twentieth century that statistics were completed as science for the collection, presentation, analysis and use of statistical data for the purpose of reasoning and decision-making p.25. Statistics can be understood as: 1. A branch of knowledge (science), i.e., a scientific course. 2. A specialized branch of applied science aimed at collecting, processing, analyzing, and publishing mass data about the events and processes of social life. 3. A collection of digital information that characterizes Statistics Definition & Meaninig any event or group of events. Dr. Manel REBBOUH 5 An Overview of Statistics 4. A term used to refer to the functions of the results of observation. 5. A specific research method. In conclusion, STATISTICS isThe science of collecting, organizing, presenting, analyzing, and interpreting data to assist in making more effective decision Extra Statistic and Statistics Statistic (not to be confused with Statistics) is characteristic or measure obtained from a sample. Statistics is collection of methods for planning experiments, obtaining data, and then organizing, summarizing, presenting, analyzing, interpreting, and drawing conclusions. 2.1. The Concept of Statistics Can be comprehended in terms of studying a quantitative aspect of a mass event or process in its inseparable connection to respective qualitative contents under certain conditions of place and time, i.e. context. The regularity of the connection between a necessity, an individual random event, and the description of a phenomenon is called statistical regularity. a) The Development of Statistics As A Science The word “statistics” was first used by German scientist Gottfried Achenwall who borrowed this word from the Italian language. During the Renaissance, a special course on the knowledge of policy was given the name ragione di stato or diciplina de statu and widely used in Italy. The words stato and statu mean “state” and are the origin of the German word Staat and the English word state. People dealing with policy matters and who were experts on matters related to other countries were called statista. In the seventeenth century, the phrase diciplina statistica (the discipline of statistics) was quite well known in Germany. Achenwall, transformed the adjectival use of statistica into a noun form and introduced the word Statistica, which referred to knowledge needed by merchants, politicians, the military, and the intelligentsia. i) Important Statistical Terms There are a set of terms related to the concept of statistics, which can be summarised as follows p.25: - Statistical Population: It is the collection of all possible observations of a specified characteristic of interest (possessing certain common property) and being under study. - Sample: It is a subset of the population, selected using some sampling technique in such a way that they represent the population. - Sampling: The process or method of sample selection from the population - Sample size: The number of elements or observation to be included in the sample. - Census: Complete enumeration or observation of the elements of the population. Or it is the collection of data from every element in a population - Parameter: Characteristic or measure obtained from a population. Foe example : average gross income of all people in the Algeria 2020. - Statistic: Characteristic or measure obtained from a sample. For Example: 2020 gross income of people in a sample of 3regions - Variable: It is an item of interest that can take on many different numerical values 6 Dr. Manel REBBOUH An Overview of Statistics 2.2. The methodology of statistics/ Stages in Statistical Investigation Includes a set of techniques, rules, and methods of statistical research into socioeconomic events. The typical characteristics of statistical methodology are: 1- Accurate determination of statistical objective/ target 2- Collection of statistical data: 3- Presentation of statistical data 4- Analysis of statistical data 5- Inference of data The Methodology of Statistics a) Accurate Determination of Statistical Objective/ Target This means limiting the type of data to be collected, which translates into questions that are included in a special document called a form. This requires good organization and full clarity of questions, and the statistical objective is derived from the overall objective of the statistical study. Example: We want to conduct a statistical study on the standard of living of the households in Algeria (general objective). Determining the statistical objective: family income - number of individuals in the family - type of housing - number of rooms. i) Collection of Statistical Data Statistical data are collected in different ways, depending on the objective of the study and the analytical method followed. Among the methods used in the collection of data are the following p.25 : 2-1-Direct and Indirect Method: -Direct Method: This method means the researcher gathered statistical information himself, from its initial sources, such as asking questions directly to households. Dr. Manel REBBOUH 7 An Overview of Statistics -Indirect Method: The method of secondary data, which includes all available data and statistical information from statistical documents, publications and publications produced by various entities and agencies, as well as various international entities and organizations, has many benefits. The most important of these methods is that they lead to a large economy at the time of the researcher and his expenses, but they complain of a number of disadvantages, including: Sometimes mismatches between the data provided by the secondary source and the data that the researcher expect to obtain; - Lack of data quantity and accuracy; -The statistical module used may not match the search plan. 2-2-Comprehensive inventory method and sample method: - Comprehensive inventory method : When all statistical units consisting of the statistical population under study are accounted for, one of the advantages of this method is that it gives us a complete picture of the statistical society, characterized by the required accuracy, however this method is difficult to execute and needs high costs and a large and specialized statistical apparatus. -Statistical Sample Method: Only part of the statistical POPULATION is studied by taking a random sample of the society, studying its characteristics and extracting the necessary information from it, and then circulating its results to the POPULATION from which it was withdrawn. Statistical Sample Method 1 Presentation of Statistical Data Summarizing data using graphical techniques üOR Summarizing Data for a Categorical Variable. The process of re-organization, classification, compilation, and summarization of data to present it in a meaningful form: 3-1-Frequency Distribution: We begin the discussion of how tabular and graphical displays can be used to summarize categorical data with the definition of a frequency distribution. Written Presentation: This method means the presentation of statistical data in the context of a prose paragraph, which is reasonable if the statistical information presented consists of a small number of numbers, but the statistics often consist of many numbers that are difficult to mention in the content of the written text. 8 Dr. Manel REBBOUH An Overview of Statistics Tabular Presentation: Data are shown in tables, by classifying and arranging information according to some of its characteristics, and the most important methods.The arrangement is: Historical ranking, alphabetical ranking, quantitative ranking, geographical ranking. The following information is required in the table. Be scientifically acceptable: Full and clear title of the table (specifying the subject, place, time), usually either at the top of the table or below it and number;Measurement unit: at the top of the table to right; The source of the table: i.e. the identification of the source of the data in the table, and be at the bottom of the table. üThe presentation method is accurate, so it is the most important method of presenting information, and what is taken on.This method is not to give a quick idea just one look at the table. 1 Analysis of Statistical Data This phase involves studying, arranging and analyzing statistical information to its primary elements and represent the relationships between them, information is analysed by the following steps -The ranking and classification of statistics, and the ranking can be by type or quantity, such as the classification of the population among the Single, married, divorced and widowed, and the arrangement can also be geographical, such as the distribution of Algeria's population by state, constituency and country; - Calculating the centralized values of the data collection and studying dispersion and twisting; -The study of R-relationships linking the factors of statistical society; - Devising estimates or predictions evidenced by the study. 1 Inference of Data It is well known that statistical studies are taken primarily in the preparation of policies and decision- making on economic, social and other topics, and that they adopt the trends of the state, companies or public and private institutions, hence the need for statisticians as the most knowledgeable and experienced people in understanding the content of the numbers to explain the results reached and to explain frankly what they mean. 2.3. Types of Statistics Within the field of statistics, two fundamental approaches exist for analyzing data: descriptive and inferential statistics. Descriptive statistics provide a concise characterization of a data set, employing measures of central tendency (like mean or median) and dispersion (like standard deviation). Additionally, descriptive statistics leverage visual representations such as charts and graphs to effectively communicate data patterns. In contrast, inferential statistics delve deeper, using sample data to draw conclusions about larger populations. This empowers researchers to test hypotheses, identify correlations between variables, and make predictions with a degree of certainty. By understanding and utilizing both descriptive and inferential techniques, statisticians can effectively extract knowledge from data, informing decision-making across various disciplines. a) Descriptive Statistics The study of statistics is usually divided into two categories: descriptive statistics and inferential statistics. The definition of statistics given earlier referred to "organizing, presenting,... data." This facet of statistics is usually referred to as descriptive statistics. Most of the statistical information in newspapers, magazines, company reports, and other publications consists of data that are summarized and presented in a form that is easy for the reader to understand. Such summaries of data, which may be tabular, graphical, or numerical, are referred to as descriptive statistics. Dr. Manel REBBOUH 9 An Overview of Statistics i) Inferential Statistics Another facet of statistics is inferential statistics-also called statistical inference or inductive statistics. Our main concern regarding inferential statistics is finding something about a population from a sample taken from that population. For example, a recent survey showed only 46 percent of high school seniors can solve problems involving fractions, decimals, and percentages. And only 77 percent of high school seniors correctly totaled the cost of soup, a burger, fries, and a cola on a restaurant menu. Since these are inferences about a population (all high school seniors) based on sample data, they are inferential statistics. The process of conducting a survey to collect data for the entire population is called a census. The process of conducting a survey to collect data for a sample is called a sample survey. As one of its major contributions, statistics uses data from a sample to make estimates and test hypotheses about the characteristics of a population through a process referred to as statistical inference. Leveraging inferential statistics is a cornerstone of robust data analysis, and SPSS p.24 serves as a powerful tool for researchers to execute these techniques. Inferential statistics allow researchers to move beyond simply describing their data by enabling them to draw conclusions about larger populations based on a representative sample. SPSS facilitates this process by providing a comprehensive suite of inferential tests. From assessing correlations between variables to meticulously testing hypotheses about group differences, SPSS streamlines the execution of these analyses. Beyond simply generating results, SPSS also offers valuable assistance in interpreting the statistical outputs, empowering researchers to translate complex data into meaningful insights that inform their research endeavors. ANOVA and t-tests are both inferential statistics tools you can use with SPSS, but they serve different purposes: T-test: Imagine you have a coin, but you suspect it might be biased. You flip it 100 times and get 70 heads. A t-test would help you analyze if this result is due to chance (fair coin) or if the coin truly favors heads. T-tests are specifically designed to compare the means (averages) of two groups only. ANOVA p.24: This is like having multiple coins. You flip a regular coin, a weighted coin likely to land on heads, and a toy coin that might not flip fairly at all. ANOVA allows you to see if there's a significant difference in the average landing (heads vs tails) among all three coins at once. It can handle three or more groups and helps identify if at least one group has a statistically different mean compared to the others. 2.4. Statistics between computers and Ethical issues In today's data-driven world, statistics and computers are two sides of the same coin. Statistical analysis unlocks the power hidden within vast datasets, while computers provide the processing muscle to crunch the numbers and reveal hidden patterns. This potent combination fuels progress in countless fields, from healthcare research to financial modeling. However, this powerful alliance also raises a critical question: how do we ensure the ethical use of statistics in the computer age? This exploration delves into the intricate relationship between statistics, computers, and the ethical challenges that arise. We'll examine how statistical methods can be manipulated to produce misleading results, how algorithms can perpetuate bias, and how the collection and storage of personal data raises crucial privacy concerns. By understanding these ethical dilemmas, we can leverage the power of statistics and computers for good, fostering responsible data analysis practices and ensuring a future where technology serves humanity without compromising ethical principles. 10 Dr. Manel REBBOUH An Overview of Statistics a) Computers and Statistical Analysis Computers have revolutionized statistical analysis. In the past, analyzing large datasets was a tedious and time-consuming manual process. Today, computers handle the heavy lifting. They can efficiently calculate complex statistical tests, generate informative visualizations, and manage enormous datasets with ease. This allows researchers and analysts to delve deeper into data, uncovering hidden patterns, trends, and relationships that might have been missed before. This newfound power has transformed numerous fields, from scientific research and healthcare to marketing and finance, leading to more informed decisions and groundbreaking discoveries. Statisticians use computer software to perform statistical computations and analyses Using Microsoft Excel and the statistical package Minitab to implement the statistical techniques Special data manipulation and analysis tools are needed for big data. Open-source software for distributed processing of large data sets such as Hadoop, open- source programming languages such as R, and commercially available packages such as SAS and SPSS are used in practice for big data. The Marriage of Machines and Meaning: Computers and Statistical Analysis i) Ethical Guidelines for Statistical Practice Ethical behavior is something we should strive for in all that we do. Ethical issues arise in statistics because of the important role statistics plays in the collection, analysis, presentation, and interpretation of data. In a statistical study, unethical behavior can take a variety of forms including improper sampling, inappropriate analysis of the data, development of misleading graphs, use of inappropriate summary statistics, and/or a biased interpretation of the statistical results. As you begin to do your own statistical work, we encourage you to be fair, thorough, objective, and neutral as you collect data, conduct analyses, make oral presentations, and present written reports containing information developed. As a consumer of statistics, you should also be aware of the possibility of unethical statistical behavior by others. When you see statistics in newspapers, on television, on the Internet, and so on, it is a good idea to view the information with some skepticism, always being aware of the source as well as the purpose and objectivity of the statistics provided. 3. Applications of Statistics Statistics permeate every aspect of our lives, playing a critical role in various fields. From business and finance, where statistics guide market research, product development, and risk assessment, to science and medicine, where they ensure the validity of research findings and drug trials. In government and public policy, statistics inform decisions on resource allocation, public health initiatives, and social programs. Even in the realm of sports, statistics are used to analyze player performance, optimize strategies, and predict game outcomes. Statistics are the language of data, empowering us to make informed decisions, uncover hidden patterns, and ultimately, gain a deeper understanding of the world around us. In today’s global business and economic environment, anyone Dr. Manel REBBOUH 11 An Overview of Statistics can access vast amounts of statistical information. The most successful managers and decision makers understand the information and know how to use it effectively. In this section, we provide examples that illustrate some of the uses of statistics in business and economics. Applications of Statistics 3.1. Social Sciences: Accounting, Finance and Economics Public accounting firms use statistical sampling procedures when conducting audits for their clients. For instance, suppose an accounting firm wants to determine whether the amount of accounts receivable shown on a client’s balance sheet fairly represents the actual amount of accounts receivable. Usually the large number of individual accounts receivable makes reviewing and validating every account too time-consuming and expensive. As common practice in such situations, the audit staff selects a subset of the accounts called a sample. After reviewing the accuracy of the sampled accounts, the auditors draw a conclusion as to whether the accounts receivable amount shown on the client’s balance sheet is acceptable. Financial analysts use a variety of statistical information to guide their investment recommendations. In the case of stocks, analysts review financial data such as price/earnings ratios and dividend yields. By comparing the information for an individual stock with information about the stock market averages, an analyst can begin to draw a conclusion as to whether the stock is a good investment. For example, The Wall Street Journal (June 6, 2015) reported that the average dividend yield for the S&P 500 companies was 2%. Microsoft showed a dividend yield of 1.95%. In this case, the statistical information on dividend yield indicates a lower dividend yield for Microsoft than the average dividend yield for the S&P 500 companies. This and other information about Microsoft would help the analyst make an informed buy, sell, or hold recommendation for Microsoft stock Economists frequently provide forecasts about the future of the economy or some aspect of it. They use a variety of statistical information in making such forecasts. For instance, in forecasting inflation rates, economists use statistical information on such indicators as the Producer Price Index, the unemployment rate, and manufacturing capacity utilization. Often these statistical indicators are entered into computerized forecasting models that predict inflation rates. 3.2. Production & Quality Control Today’s emphasis on quality makes quality control an important application of statistics in production. A variety of statistical quality control charts are used to monitor the output of a production process. In particular, an x-bar chart can be used to monitor the average output. Suppose, for example, that a machine fills containers with 12 ounces of a soft drink. Periodically, a production worker selects a sample of containers and computes the average number of ounces in the sample. This average, or x-bar value, is plotted on an x-bar chart. A plotted value above the chart’s upper control limit indicates over filling, and a plotted value below the chart’s lower control limit indicates underfilling. The process is termed “in control” and allowed to continue as long as the plotted x-bar values fall between the chart’s upper and lower control limits. Properly interpreted, an x-bar chart can help determine when adjustments are necessary to correct a production process. 12 Dr. Manel REBBOUH An Overview of Statistics 3.3. Information Systems Information systems administrators are responsible for the day-to-day operation of an organization’s computer networks. A variety of statistical information helps administrators assess the performance of computer networks, including local area networks (LANs), wide area networks (WANs), network segments, intranets, and other data communication systems. Statistics such as the mean number of users on the system, the proportion of time any component of the system is down, and the proportion of bandwidth utilized at various times of the day are examples of statistical information that help the system administrator better understand and manage the computer network. Conclusion In conclusion, this overview has provided a foundational understanding of statistics, a discipline essential for navigating the data-driven world we inhabit. Descriptive statistics serve as the cornerstone, summarizing data and revealing its central tendencies and variability. Inferential statistics then build upon this foundation, empowering us to make informed generalizations about populations and test hypotheses. This introductory foray has only hinted at the vast potential of statistics. As you delve deeper, you'll uncover a rich tapestry of specialized methods designed to analyze diverse data types and address intricate research questions. The mastery of statistics equips you to transform raw numbers into meaningful insights, navigate the complexities of data analysis, and ultimately, unlock the secrets hidden within data. Remember, statistics transcends mere formulas and calculations. It fosters critical thinking, problem- solving, and the ability to extract knowledge from the ever-growing ocean of information. So, embark on this intellectual odyssey, and prepare to be awestruck by the power of statistics to illuminate the world around us. Dr. Manel REBBOUH 13 Quiz : Check Your Progress [solution n°1 p. 23] II What is descriptive statistics? Summary calculations, graphs, charts and tables A method used to generalize from a sample to a population The process of measuring, gathering, assembling the raw data 14 Dr. Manel REBBOUH Quiz : Chooce one Correct Answer [solution n°2 p. 23] III What is inferential statistics? The process of measuring, gathering, assembling the raw data Summary calculations, graphs, charts and tables A method used to generalize from a sample to a population Dr. Manel REBBOUH 15 Types of Variable In Statistics IV 1. Quantitive Variables When the variable studied can be reported numerically, the variable is called a quantitative variable. Examples of quantitative variables are the balance in your checking account, the ages of company CEOs, the life of an automobile battery (such as 42 months), and the number of children in a family. Quantitative variables are either discrete or continuous. Discrete variables can assume only certain values, and there are usually "gaps" between the values. Examples of discrete variables are the number of bedrooms in a house (1, 2, 3, 4, etc.), the number of cars arriving at Exit 25 on 1-4 in Florida near Walt Disney World in an hour (326, 421, etc.), and the number of students in each section of a statistics course (25 in section A, 42 in section B, and 18 in section C). Typically, discrete variables result from counting. We count, for example, the number of cars arriving at Exit 25 on 1-4, and we count the number of statistics students in each section. Notice that a home can have 3 or 4 bedrooms, but it cannot have 3.56 bedrooms. Thus, there is a "gap" between possible values. Observations of a continuous variable can assume any value within a specific range. Examples of continuous variables are the air pressure in a tire and the weight of a shipment of tomatoes. Other examples are the amount of raisin bran in a box and the duration of flights from Orlando to San Diego. Typically, continuous variables result from measuring. 1.1. Elements, Variables, and Observations Elements are the entities on which data are collected. is an element with the nation or element name shown in the first column. With 60 nations, the data set contains 60 elements. A variable is a characteristic of interest for the elements. Measurements collected on each variable for every element in a study provide the data. The set of measurements obtained for a particular element is called an observation. a) Scales of Measurement Data collection requires one of the following scales of measurement: nominal, ordinal, inter val, or ratio. The scale of measurement determines the amount of information contained in the data and indicates the most appropriate data summarization and statistical analyses. When the data for a variable consist of labels or names used to identify an attribute of the element, the scale of measurement is considered a nominal scale The scale of measurement for a variable is considered an ordinal scale if the data exhibit the properties of nominal data and in addition, the order or rank of the data is meaningful Ordinal data can also be recorded by a numerical code, for example, your class rank in school. The scale of measurement for a variable is an interval scale if the data have all the properties of ordinal data and the interval between values is expressed in terms of a fixed unit of measure. Interval data are always numerical. College admission SAT scores are an example of interval-scaled data. For example, three students with SAT math scores of 620, 550, and 470 can be ranked or ordered in terms of best performance to poorest per formance in math. In addition, the differences between the scores are meaningful. For instance, student 1 scored 620 − 550 = 70 points more than student 2, while student 2 scored 550 − 470 = 80 points more than student 3. 16 Dr. Manel REBBOUH Types of Variable In Statistics The scale of measurement for a variable is a ratio scale if the data have all the properties of interval data and the ratio of two values is meaningful. Variables such as distance, height, weight, and time use the ratio scale of measurement. This scale requires that a zero value be included to indicate that nothing exists for the variable at the zero point. (see Scales of Measurement - Nominal, Ordinal, Interval, & Ratio Scale Data) 1.2. Continous Variable A continuous variable is one that gives us a score for each entity and can take on any value on the measurement scale that we are using. The first type of continuous variable that you might encounter is an interval variable. Interval data are considerably more useful than ordinal data and most of the statistical tests in this book rely on having data measured at this level. To say that data are interval, we must be certain that equal intervals on the scale represent equal differences in the property being measured. For example, on www.ratemyprofessors.com students are encouraged to rate their lecturers on several dimensions (some of the lecturers’ rebuttals of their negative evaluations are worth a look). Each dimension (i.e., helpfulness, clarity, etc.) is evaluated using a 5-point scale. For this scale to be interval it must be the case that the difference between helpfulness ratings of 1 and 2 is the same as the difference between say 3 and 4, or 4 and 5. Similarly, the difference in helpfulness between ratings of 1 and 3 should be identical to the difference between ratings of 3 and 5. Variables like this that look interval (and are treated as interval) are often ordinal Ratio variables go a step further than interval data by requiring that in addition to the measurement scale meeting the requirements of an interval variable, the ratios of values along the scale should be meaningful. For this to be true, the scale must have a true and meaningful zero point. In our lecturer ratings this would mean that a lecturer rated as 4 would be twice as helpful as a lecturer rated with a 2 (who would also be twice as helpful as a lecturer rated as 1!). The time to respond to something is a good example of a ratio variable. When we measure a reaction time, not only is it true that, say, the difference between 300 and 350 ms (a difference of 50 ms) is the same as the difference between 210 and 260 ms or 422 and 472 ms, but also it is true that distances along the scale are divisible: a reaction time of 200 ms is twice as long as a reaction time of 100 ms and twice as short as a reaction time of 400 ms. Continuous variables can be, well, continuous (obviously) but also discrete. This is quite a tricky distinction (Jane Superbrain Box 1.3). A truly continuous variable can be measured to any level of precision p.25 1.3. Discrete Variables Discrete Variable can take on only certain values (usually whole numbers) on the scale. What does this actually mean? Well, our example in the text of rating lecturers on a 5-point scale is an example of a discrete variable. The range of the scale is 1–5, but you can enter only values of 1, 2, 3, 4 or 5; you cannot enter a value of 4.32 or 2.18. Although a continuum exists underneath the scale (i.e., a rating of 3.24 makes sense), the actual values that the variable takes on are limited. A continuous variable would be something like age, which can be measured at an infinite level of precision (you could be 34 years, 7 months, 21 days, 10 hours, 55 minutes, 10 seconds, 100 milliseconds, 63 microseconds, 1 nanosecond old). Discrete variables, like the faces of a die, can only take on a specific set of values. There are a limited number of possible outcomes for a discrete variable. Examples include the number of siblings someone has, shoe size (which typically comes in whole or half sizes), test scores (often rounded to whole numbers), or the number of customers served in a day. Dr. Manel REBBOUH 17 Types of Variable In Statistics 2. Categorical / Qualitative Variables A categorical variable is a variable with categorical data, and a quantitative variable is a variable with quantitative data. The statistical analysis appropriate for a particular variable depends upon whether the variable is categorical or quantitative. If the variable is categorical, the statistical analysis is limited. We can summarize categorical data by counting the number of observations in each category or by computing the proportion of the observations in each category. However, even when the categorical data are identified by a numerical code, arithmetic operations such as addition, subtraction, multiplication, and division do not provide meaningful results. Section 2.1 discusses ways of summarizing categorical data. Arithmetic operations provide meaningful results for quantitative variables. For example, quantitative data may be added and then divided by the number of observations to compute the average value. This average is usually meaningful and easily interpreted. In general, more alternatives for statistical analysis are possible when data are quantitative. 2.1. Classification of Qualitative Variables To understand the different types that exist: Nominal Variables: Imagine sorting seashells – their colors (red, yellow, blue) are nominal variables. They classify data into distinct groups with no inherent order or ranking. Eye color, blood type, and political affiliation are all examples of nominal variables. Ordinal Variables: Think of ranking athletes in a race. Ordinal variables establish a specific order within categories, but the intervals between categories may not be equal. For instance, education level (high school, bachelor's, master's) or customer satisfaction ratings (poor, fair, good, excellent) are ordinal variables. Interval Variable: recorded at the interval level of measurement, the interval or the distance between values is meaningful. The interval level of measurement is based on a scale with a known unit of measurement.The Fahrenheit temperature scale is an example of the interval level of measurement. Ratio Variable: recorded at the ratio level of measurement are based on a scale with a known unit of measurement and a meaningful interpretation of zero on the scale. Examples of the ratio scale of measurement include wages, units of production, weight, changes in stock prices, distance between branch offices, and height. Levels of Measurment 18 Dr. Manel REBBOUH Types of Variable In Statistics 2.2. Data Sources Data can be obtained from existing sources, by conducting an observational study, or by conducting an experiment. In the realm of descriptive statistics, data serves as the cornerstone upon which all analysis is built. It represents the raw materials from which we extract insights and understanding. However, the quality and accessibility of this data vary greatly, and its origin plays a crucial role in shaping the strength of our statistical inferences. a) Observational Study In an observational study we simply observe what is happening in a particular situation, record data on one or more variables of interest, and conduct a statistical analysis of the resulting data. For example, researchers might observe a randomly selected group of customers that enter a Walmart supercenter to collect data on variables such as the length of time the customer spends shopping, the gender of the customer, the amount spent, and so on. Statistical analysis of the data may help management determine how factors such as the length of time shopping and the gender of the customer affect the amount spent. As another example of an observational study, suppose that researchers were interested in investigating the relationship between the gender of the CEO for a Fortune 500 company and the performance of the company as measured by the return on equity (ROE). To obtain data, the researchers selected a sample of companies and recorded the gender of the CEO and the ROE for each company. Statistical analysis of the data can help determine the relationship between performance of the company and the gender of the CEO. This example is an observational study because the researchers had no control over the gender of the CEO or the ROE at each of the companies that were sampled. Surveys and public opinion polls are two other examples of commonly used observational studies. The data provided by these types of studies simply enable us to observe opinions of the respondents. For example, the New York State legislature commissioned a telephone survey in which residents were asked if they would support or oppose an increase in the state gasoline tax in order to provide funding for bridge and highway repairs. Statistical analysis of the survey results will assist the state legislature in determining if it should introduce a bill to increase gasoline taxes. i) Experiment The key difference between an observational study and an experiment is that an experiment is conducted under controlled conditions. As a result, the data obtained from a well-designed experiment can often provide more information as compared to the data obtained from existing sources or by conducting an observational study. For example, suppose a pharmaceutical company would like to learn about how a new drug it has developed affects blood pressure. To obtain data about how the new drug affects blood pressure, researchers selected a sample of individuals. Different groups of individuals are given different dosage levels of the new drug, and before and after data on blood pressure are collected for each group. Statistical analysis of the data can help determine how the new drug affects blood pressure. The types of experiments we deal with in statistics often begin with the identification of a particular variable of interest. Then one or more other variables are identified and controlled so that data can be obtained about how the other variables influence the primary variable of interest 3. Frequency Distribution In descriptive statistics, a frequency distribution serves as a cornerstone for summarizing the occurrence of values within a data set. It meticulously categorizes each data point (or groups them into intervals) and tabulates the frequency of each category. Imagine a comprehensive inventory of customer ages instead of a simple tally. A frequency distribution acts like this inventory, meticulously recording the number of customers within each age range. This organized presentation allows for a deeper understanding of the data's central tendencies (like the most common age group) and its dispersion (how spread out the ages are). It can even reveal outliers, individuals whose ages fall Dr. Manel REBBOUH 19 Types of Variable In Statistics outside the expected range. By constructing a frequency distribution, researchers transform raw data into a structured and informative table, facilitating a clear picture of the data's underlying characteristics. By the end of this part, you will be able to: Define frequency and frequency distribution: We'll establish the basic concepts and terminology. Construct a frequency distribution table: Learn the step-by-step process of organizing data into a clear and concise table format. Interpret frequency distribution tables: We'll explore how to extract meaning from the data and draw valuable conclusions. Appreciate the importance of frequency distribution: Discover how this technique applies to various real-world scenarios and facilitates informed decision-making. 3.1. What is Frequency Distribution? Recall from Chapter 1 that we refer to descriptive statistics. To put it another way, we use descriptive statistics to organize data in various ways to point out where the data values tend to concentrate and help distinguish the largest and the smallest values. The first procedure we use to describe a set of data is a frequency distribution. FREQUENCY DISTRIBUTION A grouping of data into mutually exclusive classes showing the number of observations in each. Also xe can say a frequency distribution is a tabular summary of data showing the number (frequency) of observations in each of several nonoverlapping categories or classes. How do we develop a frequency distribution? The first step is to tally the data into a table that shows the classes and the number of observations in each class. p.25 a) Class Intervals and Class Midpoints We will use two other terms frequently: class midpoint and class interval. The midpoint is halfway between the lower limits of two consecutive classes. It is computed by adding the lower limits of consecutive classes and dividing the result by 2. Referring to Table 2-4, for the first class the lower class limit is $15,000 and the next limit is $18,000. The class midpoint is $16,500, found by ($15,000 + $18,000)12. The midpoint of $16,500 best represents, or is typical of, the selling price of the vehicles in that class. To determine the class interval, subtract the lower limit of the class from the lower limit of the next class. The class interval of the vehicle selling price data is $3,000, which we find by subtracting the lower limit of the first class, $15,000, from the lower limit of the next class; that is, $18,000 - $15,000 = $3,000. You can also determine the class interval by finding the difference between consecutive midpoints. The midpOint of the first class is $16,500 and the midpoint of the second class is $19,500. The difference is $3,000. i) Frequency Distributions (Absolute & Relative ) A frequency distribution shows the number (frequency) of observations in each of several nonoverlapping classes. However, we are often interested in the proportion, or percentage, of observations in each class. The relative frequency of a class equals the fraction or proportion of observations belonging to a class. for a data set with n observations, the relative frequency of each class can be determined as follows: The percent frequency of a class is the relative frequency multiplied by 100. A relative frequency distribution gives a tabular summary of data showing the relative frequency for each class. A percent frequency distribution summarizes the percent frequency of the data for each class 20 Dr. Manel REBBOUH Types of Variable In Statistics It may be desirable to convert class frequencies to relative class frequencies to show the fraction of the total number of observations in each class. To convert a frequency distribution to a relative frequency distribution, each of the class frequencies is divided by the total number of observations. The percent frequency of a class is the relative frequency multiplied by 100. A relative frequency distribution gives a tabular summary of data showing the relative frequency for each class. A percent frequency distribution summarizes the percent frequency of the data for each class Relative Frequency Rule 1 Ascending cumulative frequency distribution A set of (ascending) cumulative frequency values is always increasing, whereas a set of descending cumulative frequency values is always decreasing. "Ascending cumulative frequency" is a term used in statistics to describe the number of events that have occurred up to a certain point in time, arranged in order from least to greatest. It is a way of summarizing how frequently events happen over time. For example, imagine you are tracking the number of website visitors you have each day. You could create a table that shows the ascending cumulative frequency of website visitors. The first row might show that you had 1 visitor on the first day. The second row might show that you had 3 visitors on the second day (1 from the first day, plus 2 on the second day). And so on. Ascending cumulative frequency can be a useful way to see how things are changing over time. For example, you could use it to see if the number of website visitors to your site is increasing, decreasing, or staying the same. Example for Ascending Cumulative Frequency 1 Descending Cumulative Frequency In descriptive statistics, descending cumulative frequency refers to the number of observations in a data set that are greater than or equal to a specific value. Unlike ascending cumulative frequency, which builds from the lowest value to the highest, descending cumulative frequency starts with the highest value and works its way down. Imagine a dataset representing exam scores. Descending cumulative frequency would tell you, for each score value, how many students scored equal to or higher than that value. Dr. Manel REBBOUH 21 Types of Variable In Statistics Here's a breakdown of the key points: Focus: Descending cumulative frequency focuses on values greater than or equal to a specific point. Direction: It starts with the highest value and decreases as we move down the data set. Interpretation: It helps us understand how many observations fall above (or at) a particular value. How to Calculate The Descending Cumulative Frequency? 3.2. Activity for More Clarification and fix the previous rules and terms Example 1: The following data are the results of a statistical study on the number of rooms in one building, for a sample of 50 buildings in a town. Data of The Example Conclusion In conclusion, our exploration of variable types has shed light on the fundamental elements that constitute your data. By differentiating between continuous and discrete variables, nominal and ordinal categories, and independent and dependent variables, you have acquired a robust foundation for effective data analysis within the realm of descriptive statistics. 22 Dr. Manel REBBOUH Exercise solutions [exercice p. 14] Solution n°1 What is descriptive statistics? Summary calculations, graphs, charts and tables A method used to generalize from a sample to a population The process of measuring, gathering, assembling the raw data [exercice p. 15] Solution n°2 What is inferential statistics? The process of measuring, gathering, assembling the raw data Summary calculations, graphs, charts and tables A method used to generalize from a sample to a population Dr. Manel REBBOUH 23 Abbreviation ANOVA : Analysis Of Variance SPSS : Statistical Package for the Social Sciences. 24 Dr. Manel REBBOUH References Natalia Kovtun, Basic Statistics for Economists, 2022, Lady Stephenson Library, UK. https://www.scribd.com/document/525625555/Stages-in-Statistical-Investigation Michael Barrow, Statistics for Economics, Accounting and Business Studies, Seventh edition, Pearson Education, Harlow, 2017. Douglas A. Lind, William G. Marchal,Samuel A. Wathen , Basic Statistics for BUSINESS & ECONOMICS, McGraw-Hill Education,NINTH EDITION, New York, 2019 K Alagar, Business Statistics, Tata McGraw-Hill Education, New Delhi, 2009§. Dr. Manel REBBOUH 25