Chapter 7 Demand Management PDF
Document Details
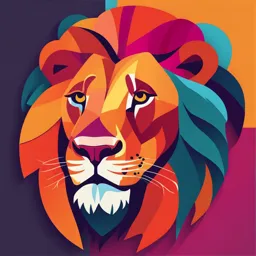
Uploaded by GentleCosecant
Stanford University
Tags
Summary
This chapter discusses demand management, a vital component of outbound-to-customer logistics systems in business. Key topics include forecasting, sales and operations planning (S&OP), and collaborative forecasting, providing an overview of their importance within the field of business and supply chain management.
Full Transcript
Chapter 7 DEMAN D M A N A G E M E N T Learning Objectives After reading this chapter, you should be able to do the following: 205 Copyright 2021 Cengage Learning. All Rights Reserved. May not be copied, scanned, or duplicated, in whole or in part. Due to electronic rights, some third party content m...
Chapter 7 DEMAN D M A N A G E M E N T Learning Objectives After reading this chapter, you should be able to do the following: 205 Copyright 2021 Cengage Learning. All Rights Reserved. May not be copied, scanned, or duplicated, in whole or in part. Due to electronic rights, some third party content may be suppressed from the eBook and/or eChapter(s). Editorial review has deemed that any suppressed content does not materially affect the overall learning experience. Cengage Learning reserves the right to remove additional content at any time if subsequent rights restrictions require it. 206 Chapter 7 Supply Chain Profile ATA’s Freight Forecast Report LOOKS AHEAD TO INCREASING FREIGHT VOLUMES When people think about trucking, many things come to mind. These days, some of the common themes include tight over-the-road capacity, ever-shifting regulations and the ongoing driver shortage. Even though these themes pose frequent challenges for freight transportation industry stakeholders, data issued last month in the most recent edition of the American Trucking Associations’ (ATA) “Freight Forecast” report reminds us all that this ubiquitous mode remains dominant when it comes to hauling a huge portion of our nation’s freight. The key takeaway of this year’s edition is that the ATA pegs freight volumes to increase 4.2% this year and by another 35.6% by 2029—which, if you think about it, is not that far away. However, that’s not the only astonishing number included in this data-rich report. Other numbers include: Total tonnage transported will reach nearly 16 billion tons in 2018—a figure that should rise 35.6% to 21.7 billion tons in 2029. Truck volumes are expected to grow 2.3% per year from 2019 to 2024 and 2.2% annually for the next five years. Changes in demand for commodities—notably commodities moved by pipeline—will alter trucking’s share of freight volumes. In 2018, trucks are projected to move 70.2% of total tonnage, and that share is expected to sink to 65.9% in 2029. Nonetheless, the trucking industry will remain the single largest mover of freight; and The transport of freight by rail, including intermodal, will account for 12.6% of tonnage this year, but that figure is expected to drop to 10% in 2029—again, due to strength in pipeline, not falling rail volumes. ATA chief economist Bob Costello said: “The movement of goods is such a critical component of our economy, and the growth we’re projecting in freight demand is a reflection of its strength.” Meanwhile, Chris Spear, ATA president and CEO, noted that these projected increases in freight volumes force the need to take on the myriad issues trucking and the supply chain are up against, like workforce development and infrastructure investment. “More freight means we’ll need more trucks and more drivers to continue safely delivering our nation’s goods,” he added. Spear is correct on the need for more trucks and more drivers, especially the latter part of the year. While the remedy remains elusive, progress is being made—albeit incrementally—through things like pay increases, tuition assistance for driver training, and carriers working to accommodate a work–life balance for drivers. The supply chain can appear to be heavily fragmented at times because of its multiple transportation modes, regulations, and emerging technologies and trends, but many links of the chain begin and end with a truck—and the data presented in this report make that very clear. Source: Jeff Berman, Logistics Management, October 2018, p. 15. Copyright 2021 Cengage Learning. All Rights Reserved. May not be copied, scanned, or duplicated, in whole or in part. Due to electronic rights, some third party content may be suppressed from the eBook and/or eChapter(s). Editorial review has deemed that any suppressed content does not materially affect the overall learning experience. Cengage Learning reserves the right to remove additional content at any time if subsequent rights restrictions require it. Demand Management 207 7-1 Introduction In an effort to better serve their customers, many organizations place significant emphasis on what might be termed their outbound-to-customer logistics systems. Also referred to as physical distribution, this essentially refers to the processes, systems, and capabilities that enhance an organization’s ability to serve its customers. For example, the ways in which retailers such as Walmart, Target, and Amazon fulfill their customers’ orders are examples of outbound logistics. This topic has been of significant historical interest in the study of logistics and supply chain management. This chapter will highlight key areas of concern related to this general topic. Correspondingly, the topic of inbound-to-operations logistics systems refers to the activities and processes that precede and facilitate value-adding activities such as procurement (see Chapter 5), operations (see Chapter 6), and assembly. Other terms that focus on these elements of the supply chain include materials management and physical supply. A typical example would be movements of automotive parts and accessories that need to move from supplier locations to automotive assembly plants. Although many of the principles of inbound logistics are conceptually similar to those of outbound logistics, some important differences must be recognized. Thus, the topic of inbound logistics systems will be the focus of Chapter 5, which is titled “Sourcing Materials and Services.” Considering the complexity of the topic at hand, this chapter has a relatively aggressive agenda of topics to be discussed. First, a discussion of demand management provides an overview of the importance of effectively managing outbound-to-customer processes. Second, the topic of forecasting is addressed. Third, an introduction to the sales and operations planning (S&OP) process is provided. Finally, the recent emphasis on collaborative forecasting approaches is covered. 7-2 Demand Management According to Blackwell and Blackwell, demand management might be thought of as “focused efforts to estimate and manage customers’ demand, with the intention of using this information to shape operating decisions.”1 Traditional supply chains typically begin at the point of manufacture or assembly and end with the sale of product to consumers or business buyers. Much of the focus and attention has been related to the topic of product flow, with significant concern for matters such as technology, information exchange, inventory turnover, delivery speed and consistency, and transportation. This notwithstanding, it is the manufacturers—many times far removed from the end user or consumer market—who determine what will be available for sale, where, when, and how many. If this seems to reflect a disconnect between manufacturing and demand at the point of consumption, that is exactly what it is. Thus, any attention paid to demand management will produce benefits throughout the supply chain. The essence of demand management is to further the ability of firms throughout the supply chain—particularly manufacturing through the customer—to collaborate on activities related to the flow of products, services, information, and capital. The desired end result should be to create greater value for the end user or consumer. There are a number of ways in which effective demand management will help to unify channel members with the common goals of satisfying customers and solving customer problems:2 Gathering and analyzing knowledge about consumers, their problems, and their unmet needs Identifying partners to perform the functions needed in the demand chain Copyright 2021 Cengage Learning. All Rights Reserved. May not be copied, scanned, or duplicated, in whole or in part. Due to electronic rights, some third party content may be suppressed from the eBook and/or eChapter(s). Editorial review has deemed that any suppressed content does not materially affect the overall learning experience. Cengage Learning reserves the right to remove additional content at any time if subsequent rights restrictions require it. Chapter 7 208 Moving the functions that need to be done to the channel member that can perform them most effectively and efficiently Sharing with other supply chain members knowledge about consumers and customers, available technology, and logistics challenges and opportunities Developing products and services that solve customers’ problems Developing and executing the best logistics, transportation, and distribution methods to deliver products and services to consumers in the desired format As organizations identify the need for improved demand management, several problems occur. First, the lack of coordination between departments (i.e., the existence of “functional silos”) results in little or no coordinated response to demand information. Second, too much emphasis is placed on forecasts of demand, with less attention on the collaborative efforts and the strategic and operational plans that need to be developed from the forecasts. Third, demand information is used more for tactical and operational purposes than for strategic ones. In essence, since in many cases historical performance is not a very good predictor of the future, demand information should be used to create collective and realistic scenarios for the future. Primary emphasis should be on understanding likely demand scenarios and mapping their relationships to product supply alternatives. The end result will be to better match demand as it occurs with appropriate availability of needed product in the marketplace. Figure 7.1 provides an overview of how supply and demand misalignment might impact overall supply chain effectiveness. Using the personal computer (PC) industry as an example, this figure charts production, channel orders, and true end-user demand over the life cycle of a product. Ignoring the early adopters, end-user demand for PCs typically is at its highest level at the time new products are launched, which is also the time that availability is most precarious. As new, competing products become available, end-user demand begins to taper off, eventually reaching a modest level, at which time the product, now much more available, is generally phased out. Figure 7.1 Supply/Demand Misalignment 1 True end-customer demand. Unit per period Channel orders 3 Channel fill and phantom demand 1 True end-customer demand Launch date 3 Channel partners over-order in an attempt to meet demand and stock their shelves. 5 Oversupply 2 Real shortage Production 2 Production cannot meet initial projected demand, resulting in real shortages. Returns/ cancellations 4 4 As supply catches up with demand, orders are canceled or returned. 5 Financial and production planning are not aligned with real demand; therefore, production continues. 6 End of life 6 As demand declines, all parties attempt to drain inventory to prevent write-down. Source: Accenture, Stanford University, and Northwestern University, Customer-Driven Demand Networks: Unlocking Hidden Value in the Personal Computer Supply Chain (Accenture, 1997): 15. Copyright 2021 Cengage Learning. All Rights Reserved. May not be copied, scanned, or duplicated, in whole or in part. Due to electronic rights, some third party content may be suppressed from the eBook and/or eChapter(s). Editorial review has deemed that any suppressed content does not materially affect the overall learning experience. Cengage Learning reserves the right to remove additional content at any time if subsequent rights restrictions require it. Demand Management 209 Looking more closely at Figure 7.1, we see that in the first phase of a new product launch, when end-user demand is at its peak and opportunities for profit margins are greatest, PC assemblers are not able to supply product in quantities sufficient to meet demand—thus creating true product shortages. Also during this time, distributors and resellers tend to “over-order,” often creating substantial “phantom” demand. In the next phase, as production begins to increase, assemblers ship product against this inflated order situation and book sales at the premium, high-level launch price. As channel inventories begin to grow, price competition sets in, as do product overages returns. This further depresses demand for the PC product, and the PC assemblers are the hardest hit. In the final phase noted in Figure 7.1, as end-user demand begins to decline, the situation clearly has shifted to one of oversupply. This is largely due to the industry’s planning processes and systems, which are primarily designed to use previous period demand as a gauge. Since much of the previous period’s demand was represented by the previously mentioned phantom demand, forecasts are distorted. The net result of aligning supply and demand is that a large majority of product is sold during the declining period of profit opportunity, thereby diminishing substantial value creation opportunities for industry participants. Adding insult to injury, substantial amounts of inventory are held throughout the supply chain as a hedge against supply uncertainty. According to Langabeer, there is growing and persuasive evidence that understanding and managing market demand are central determinants of business success.3 Aside from this observation, relatively few companies have successfully linked demand management with strategy. Table 7.1 provides a view of how demand data might be used strategically to Table 7.1 How Demand Management Supports Business Strategy STRATEGY EXAMPLES OF HOW TO USE DEMAND MANAGEMENT Growth strategy Perform “what if” analyses on total industry volume to gauge how specific mergers and acquisitions might leverage market share. Analyze industry supply/demand to predict changes in product pricing structure and market economics based on mergers and acquisitions. Build staffing models for merged company using demand data. Portfolio strategy Manage maturity of products in current portfolio to optimally time overlapping life cycles. Create new product development/introduction plans based on life cycle. Balance combination of demand and risk for consistent “cash cows” with demand for new products. Ensure diversification of product portfolio through demand forecasts. Positioning strategy Manage product sales through each channel based on demand and product economics. Manage positioning of finished goods at appropriate distribution centers, to reduce working capital, based on demand. Define capability to supply for each channel. Investment strategy Manage capital investments, marketing expenditures, and research and development budgets based on demand forecasts of potential products and maturity of current products. Determine whether to add manufacturing capacity. Source: Jim R. Langabeer II, “Aligning Demand Management with Human Strategy,” Supply Chain Management Review (May/June 2000): 58. Copyright © 2000 Reed Business Information, a division of Reed Elsevier. Reproduced by permission. Copyright 2021 Cengage Learning. All Rights Reserved. May not be copied, scanned, or duplicated, in whole or in part. Due to electronic rights, some third party content may be suppressed from the eBook and/or eChapter(s). Editorial review has deemed that any suppressed content does not materially affect the overall learning experience. Cengage Learning reserves the right to remove additional content at any time if subsequent rights restrictions require it. 210 Chapter 7 enhance an organization’s growth, portfolio, positioning, and investment strategies. As suggested, effective use of demand data can help organizations to guide strategic resources in a number of important ways. 7-3 Balancing Supply and Demand The essence of demand management, as previously stated, is to estimate and manage customer demand and use this information to make operating decisions. However, demand and supply in an organization will more than likely never be balanced to allow for zero stockouts and zero safety stocks. Many methods to manage this imbalance exist. However, there are four methods that are commonly used across many industries. Two of those, price and lead time, are referred to as external balancing methods. The other two, inventory and production flexibility, are called internal balancing methods. External balancing methods are used in an attempt to change the manner in which the customer orders in an attempt to balance the supply–demand gap. Dell has found these methods to be relatively effective in smoothing demand to meet supply. For example, Dell frequently refreshes its Web site with price changes and availability changes based on the demand for an item and its supply. If customer demand exceeds the current supply, Dell can increase the lead time for that item for customer delivery. By doing so, one of two results can occur. First, if the customer finds the increased lead time unacceptable, she or he might decide to specify an alternative item from Dell for which there is sufficient inventory. Second, if the customer decides the increased lead time is acceptable, Dell now has the opportunity to wait for the next delivery of that item from suppliers. If customer demand is less than current inventory levels for a particular item, a price reduction for that item will appear on the Web site, hopefully increasing demand for the item. Using both methods allows Dell to manage stockouts while minimizing safety stock inventories. Internal balancing methods utilize an organization’s internal processes to manage the supply–demand gap. Production flexibility allows an organization to quickly and efficiently change its production lines from one product to another. This is one principle of lean manufacturing. Being able to react quickly to changing demand by altering production schedules will allow for a minimum of safety stocks while reducing the possibility of a stockout. The On the Line Volatility in Demand Has Become the Norm In today’s business environment, companies remain “cautiously optimistic,” observes Ted Fernandez, chairman and CEO of global business advisory firm, The Hackett Group. “Most companies in the S&P 500 beat their guidance for the first quarter,” he says. “But mixed guidance has been the issue as companies continue to struggle to meet growth objectives.” Fernandez added that many companies have been quick to turn to productivity initiatives to hit earnings targets. “This should improve as the U.S. economy continues to show better signals, but for now there remains cautious optimism across the globe.” According to Fernandez, finding sustainable demand continues to be more challenging than expected as we now approach the sixth anniversary of the financial crisis. “Volatility in demand has become the norm, and companies understand that they need the ability to quickly recalibrate.” Source: Logistics Management, June 2014, p. 3. Copyright 2021 Cengage Learning. All Rights Reserved. May not be copied, scanned, or duplicated, in whole or in part. Due to electronic rights, some third party content may be suppressed from the eBook and/or eChapter(s). Editorial review has deemed that any suppressed content does not materially affect the overall learning experience. Cengage Learning reserves the right to remove additional content at any time if subsequent rights restrictions require it. Demand Management 211 tradeoff here is between production changeover costs and safety stock costs. Inventory is probably the most common, and maybe the most expensive, method used to manage the imbalance between supply and demand. Many organizations produce product to a forecast that includes safety stock to smooth the effects of both demand and lead time variability. This allows an organization to minimize the number of changeovers it needs to make in production but also results in high inventory levels. In these cases, stockout costs are usually high as are production changeover costs. These four methods are not mutually exclusive in most organizations. Some combination of all of them is used to manage safety stocks and stockouts. Their use and level of implementation will be determined by the nature of the product and the cost of stocking out. Also affecting their use will be the organization’s ability to properly forecast customer demand. Forecasting will be the topic of the next section. 7-4 Traditional Forecasting A major component of demand management is forecasting the amount of product that will be purchased, when it will be purchased, and where it will be purchased by customers. Although various statistical techniques exist to forecast demand, the common thread for all forecasts is that they will ultimately be wrong. The key to successful forecasting is to minimize the error between actual demand and forecasted demand. Although this sounds simple, many factors can arise in the marketplace that will change demand contrary to the forecast. However, forecasts are necessary because they serve as a plan for both marketing and operations to set goals and develop execution strategies. These goals and strategies are developed through the sales and operations planning (S&OP) process. This concept will be covered in a later section in this chapter. The remainder of this section will focus on the various basic types of forecasting techniques used throughout industry. 7-4a Factors Affecting Demand Two types of demand exist: (1) independent demand, which is the demand for the primary item, and (2) dependent demand, which is directly influenced by the demand for the independent item. For example, the demand for bicycles would be called independent. It is the demand for the primary, or finished, product and is directly created by the customer. The demand for bicycle tires would be called dependent, because the number of tires demanded is determined by the number of bicycles demanded. Most forecasting techniques focus on independent demand. For example, a bicycle manufacturer will forecast the demand for bicycles during a given period. Given that level of demand, the manufacturer knows that two tires will be required for each bicycle demanded. As such, there is no need for the bicycle manufacturer to forecast the demand for tires. From a different perspective, the tire manufacturer will need to forecast the demand for tires, because these are its independent demand items. However, the tire manufacturer will not need to forecast the demand for rims since each tire requires one rim. So, each organization in a particular supply chain will have different definitions for independent and dependent demand items. Forecasting, however, will still usually be done at the independent demand item level. Normally, the demand for independent demand items is known as base demand, that is, normal demand. However, all demand is subject to certain fluctuations. One type of demand fluctuation is caused by random variation, a development that cannot be anticipated and is usually the cause to hold safety stocks to avoid stockouts. For example, the hurricanes that Copyright 2021 Cengage Learning. All Rights Reserved. May not be copied, scanned, or duplicated, in whole or in part. Due to electronic rights, some third party content may be suppressed from the eBook and/or eChapter(s). Editorial review has deemed that any suppressed content does not materially affect the overall learning experience. Cengage Learning reserves the right to remove additional content at any time if subsequent rights restrictions require it. 212 Chapter 7 devastated parts of Louisiana caused an unexpected surge in the demand for building supplies in that region. A second type of demand fluctuation is caused by trend—the gradual increase or decrease in demand over time for an organization. The demand for advanced electronic components in the consumer market (e.g., iPods and DVD players) is trending upward. The demand for VCR players is trending downward. A third type of demand fluctuation is caused by seasonal patterns, which will normally repeat themselves during a year for most organizations. For example, chocolate manufacturers are normally faced with several seasonal patterns during the year, such as Valentine’s Day, Easter, and Halloween. Finally, demand fluctuations can be caused by normal business cycles. These are usually driven by the nation’s economy and can be growing, stagnant, or declining. These patterns usually occur over periods of more than one year. Almost every firm is subject to all of these demand influences, making forecasting an even more challenging task. The next section will briefly examine the concept of forecast error and discuss some of the more popular forecasting methods and will show how some of these demand variations can be included in an organization’s forecasts. 7-5 Forecast Errors As previously mentioned, almost all forecasts will be wrong. Some forecasts will be higher than demand, and some will be lower. Managing the forecasting process requires minimizing the errors between actual demand and forecasted demand. The key to successful forecasting is to choose the technique that provides the least amount of forecast error. To determine which forecasting technique is best for a set of data, the forecast error must be measured. Four types of forecast error measures can be used. The first is called the cumulative sum of forecast errors (CFE) and can be calculated using Formula 7.1. t 21 CFE 5 ∑ et n 7.1 CFE calculates the total forecast error for a set of data, taking into consideration both negative and positive errors. This is also referred to as bias and was used in Tables 7.2 through 7.4. This gives an overall measure of forecast error. However, taking into consideration both negative and positive errors, this method can produce an overall low error total although individual period forecasts can either be much higher or much lower than actual demand. The second measure of forecast error is mean squared error (MSE). This measure can be calculated using Formula 7.2. MSE 5 ∑E 2 t n 7.2 This measure squares each period error so the negative and positive errors do not cancel each other out. MSE also provides a good indication of the average error per period over a set of demand data. Closely related to MSE is the third type of forecast error measure, mean absolute deviation (MAD). It can be calculated using Formula 7.3. MAD 5 ∑|E | t n 7.3 Copyright 2021 Cengage Learning. All Rights Reserved. May not be copied, scanned, or duplicated, in whole or in part. Due to electronic rights, some third party content may be suppressed from the eBook and/or eChapter(s). Editorial review has deemed that any suppressed content does not materially affect the overall learning experience. Cengage Learning reserves the right to remove additional content at any time if subsequent rights restrictions require it. Demand Management Table 7.2 213 Forecast Calculation FORECASTS PERIOD FOUR-PERIOD MOVING AVERAGE Dt DEMAND FOUR-PERIOD WEIGHTED MOVING AVERAGE EXPONENTIAL SMOOTHING 2018 September 8,299 October 11,619 November 7,304 December 5,976 2019 January 10,210 8,300 7,204 10,500 February 9,226 8,777 8,998 10,355 March 9,717 8,179 8,839 9,791 April 11,226 8,782 9,506 9,754 May 9,718 10,095 10,573 10,490 June 9,135 9,972 9,995 10,104 July 10,702 9,949 9,594 9,620 August 11,289 10,195 10,267 10,161 September 10,210 10,211 10,770 10,725 October 10,704 10,334 10,446 10,468 November 12,179 10,726 10,692 10,586 December 11,683 11,095 11,544 11,383 Total 125,998 X 10,500 Source: Robert A. Novack, Ph.D. This measure is also calculated in Tables 7.2 through 7.4. By taking the absolute value of each error, the negative and positive signs are removed and a good indication of average error per period is calculated. This measure is popular because it is easy to understand and provides a good indication of the accuracy of the forecast. Another measure of forecast error is mean absolute percent error (MAPE). MAPE can be calculated using Formula 7.4. MAPE 5 ∑ (|E |/D )100 t t n 7.4 Finally, tracking signal can be used to measure forecast error. It is especially good at identifying if a bias exists in the forecast errors and can be calculated using Formula 7.5. Tracking signal 5 Cumulative forecast error (CFE)/Mean absolute deviation 7.5 (MAD) Copyright 2021 Cengage Learning. All Rights Reserved. May not be copied, scanned, or duplicated, in whole or in part. Due to electronic rights, some third party content may be suppressed from the eBook and/or eChapter(s). Editorial review has deemed that any suppressed content does not materially affect the overall learning experience. Cengage Learning reserves the right to remove additional content at any time if subsequent rights restrictions require it. 214 Chapter 7 The next section will examine three common forecasting techniques and will generate these five error terms for each. As will be seen, the closer each error term is to zero, the better the forecast. 7-6 Forecasting Techniques There are many different statistical techniques companies use to generate forecasts. All of these techniques require accurate data and rely on the assumption that the future will repeat the past. However, these requirements are usually violated and the forecast will be generating a forecast error. The key to good forecasting is to minimize forecast error by utilizing a forecasting technique that best fits the nature of the data. This section will briefly discuss three of the more popular forecasting techniques: simple moving average, weighted moving average, and exponential smoothing. All three will use the same historical data to develop a forecast to see which technique best fits the data. 7-6a Simple Moving Average The simple moving average is probably the simplest to develop method in basic time series forecasting. It makes forecasts based on recent demand history and allows for the removal of random effects. The simple moving average method does not accommodate seasonal, trend, or business cycle influences. This method simply averages a predetermined number of periods and uses this average as the demand for the next period. Each time the average is computed, the oldest demand is dropped and the most recent demand is included. A weakness of this method is that it forgets the past quickly. A strength is that it is quick and easy to use. Table 7.2 presents an example of using the simple moving average technique on the historical demand shown in column 2 for the end of 2018 and all of 2019. This example will use a four-period moving average. To determine the forecast for January, the demand for September, October, November, and December are averaged. This calculation is shown in Formula 7.6. Sum of last n demands n 5 Dt 1 Dt 21 1 Dt 22 1 …Dt 2n11 At 5 Where 7.6 Dt 5 Actual demand in period t n 5 Total number of periods in the average At 5 Average for period t This would result in the following calculation: (8,299 1 11,619 1 7,304 1 5,976)/4 5 8,300 The forecast for February drops the demand for September from its calculation and adds the demand for January. The calculation is as follows: (11,619 1 7,304 1 5,976 1 10,210)/4 5 8,777 Copyright 2021 Cengage Learning. All Rights Reserved. May not be copied, scanned, or duplicated, in whole or in part. Due to electronic rights, some third party content may be suppressed from the eBook and/or eChapter(s). Editorial review has deemed that any suppressed content does not materially affect the overall learning experience. Cengage Learning reserves the right to remove additional content at any time if subsequent rights restrictions require it. Demand Management 215 This process repeats itself until all forecasts are made. The four-period moving average is shown in column 3 in Table 7.2. Having created a forecast using historical data from 2018 and 2019, the next step is to apply this forecast to future demand for 2020. This example will take some liberties and assume that we have gone through the year 2020. Table 7.3 Table 7.3 (1) PERIOD Simple Moving Average (2) Dt (3) Ft (4) Et (5) |D t 2 Ft | (6) (7) (|et | 4 Dt ) 3 100 DEMAND FORECAST Dt 2 Ft ERROR ABSOLUTE DEVIATION et2 ABSOLUTE % DEVIATION 2020 January 9,700 8,300 11,400 1,400 1,960,000 February 8,765 8,777 212 12 144 March 9,231 8,179 11,052 1,052 1,106,704 11.40 April 10,664 8,782 11,882 1,882 3,541,924 17.65 May 9,233 10,095 2862 862 743,044 9.34 June 8,679 9,972 21,293 1,293 1,671,849 14.90 July 10,166 9,949 1217 217 47,089 2.13 August 10,725 10,195 1530 530 280,900 4.94 9,700 10,211 2511 511 261,121 5.27 October 10,169 10,334 2165 165 27,225 1.62 November 11,570 10,726 1844 844 712,336 7.29 December 11,100 11,095 15 5 25 September Total 119,702 X 9,975.2 Bias (total CFE) Bias x.045 1257.25 8,773 Absolute Deviation x (MAD) 731.08 Squared Error Total 10,352,361 Squared Error x (MSE) 862,696.75 Absolute % Error x (MAPE).1369 13,087 Absolute Deviation Total Absolute % Error Total 14.43 89.15 7.43 Copyright 2021 Cengage Learning. All Rights Reserved. May not be copied, scanned, or duplicated, in whole or in part. Due to electronic rights, some third party content may be suppressed from the eBook and/or eChapter(s). Editorial review has deemed that any suppressed content does not materially affect the overall learning experience. Cengage Learning reserves the right to remove additional content at any time if subsequent rights restrictions require it. 216 Chapter 7 shows the actual demand by month for 2020 in column 2 and the forecast generated in Table 7.2 is shown in column 3. The error term is simply the difference between the forecast and the actual demand and can be seen in column 4. Adding together the error terms for the forecasts results in what can be called bias or CFE—a measure of how accurate the forecast is compared to actual demand. A positive bias means that the demand was higher than forecast during the forecast period, resulting in stockouts; a negative bias means the demand was lower than the forecast, resulting in excess inventories. The closer the bias term is to zero, the better the forecast. In this example, the bias is 13,087 units or 1257.25 units per forecast period (13,087/12 periods). This means that the forecast was lower than actual demand by 3,087 cases in total and lower than actual demand per month by 257.25 cases. Absolute deviation removes the positive and negative signs from the error terms and is a measure of how accurate the overall forecast is. The closer to zero, the better the forecast is at estimating demand. The absolute deviation is shown in column 5 of Table 7.3. Adding this column results in a total absolute deviation of 8,773 units or a MAD of 731.08 (8,773/12). Squared error is shown in column 6. Adding this column results in a total squared error of 10,352,361 or a MSE of 862,696.75 (10,352,361/12). Column 7 represents the percent absolute deviation of the forecast. The total for this column is 89.15 and the MAPE is 7.43 (89.15/12). Finally, Table 7.3 calculates the tracking signal which is CFE/MAD and results in a tracking signal of 4.2. Except for bias, the magnitude of the other error terms in absolute numbers might not make intuitive sense. However, error terms are meant to be compared to each other to see which technique is best. The next technique will attempt to improve on this forecast accuracy. 7-6b Weighted Moving Average In the simple moving average method, each previous demand period was given an equal weight. The weighted moving average method assigns a weight to each previous period with higher weights usually given to more recent demand. The weights must be equal to one. The weighted moving average method allows emphasis to be placed on more recent demand as a predictor of future demand. The data in Table 7.2 will be used again to develop a weighted moving average forecast. Assume that the weights to be used will be 0.60 for the most recent period, 0.20 for the second most recent, 0.15 for the third most recent, and 0.05 for the fourth most recent period. The average for the next period will be calculated using Formula 7.7. At 5 0.60Dt 1 0.20Dt 21 1 0.15Dt 22 1 0.05Dt 23 7.7 Column 4 in Table 7.2 shows the results of this formula. The weighted moving average for January would be calculated as follows: (0.60 3 5,976) 1 (0.20 3 7,304) 1 (0.15 3 11,619) 1 (0.05 3 8,299) 5 7.204 These forecasts were rounded up or down using a 0.05 cutoff since neither a demand nor a forecast can be in partial units. This becomes the forecast for January. These forecasts can be seen in column 4. Once again, the error term is calculated and shown in column 4 in Table 7.4. The bias term for this method is 11,274 units with an average bias per period of 1106.2 units. The other error terms are MAD 5 886.2; MSE 5 1,169,415.5; MAPE 5 9.03; and tracking signal 5 1.44. The bias and tracking signal shown for this method are better than those found by using the simple moving average method. However, the other three error terms are not. This is primarily because the weighted moving average method does Copyright 2021 Cengage Learning. All Rights Reserved. May not be copied, scanned, or duplicated, in whole or in part. Due to electronic rights, some third party content may be suppressed from the eBook and/or eChapter(s). Editorial review has deemed that any suppressed content does not materially affect the overall learning experience. Cengage Learning reserves the right to remove additional content at any time if subsequent rights restrictions require it. Demand Management Table 7.4 217 Weighted Moving Average (1) PERIOD (2) (3) (4) (5) Dt Ft Et |D t 2 Ft | DEMAND FORECAST D t 2 Ft ERROR ABSOLUTE DEVIATION (6) (7) (|et | 4 Dt ) 3 100 et2 ABSOLUTE % DEVIATION 2020 January 9,700 7,204 12,496 2,496 6,230,016 25.73 February 8,765 8,998 2233 233 54,289 2.66 March 9,231 8,839 1392 392 153,664 4.25 April 10,664 9,506 11,158 1,158 1,340,964 10.86 May 9,233 10,573 21,340 1,340 1,795,600 14.51 June 8,679 9,995 21,316 1,316 1,731,856 15.16 July 10,166 9,594 1572 572 327,184 5.63 August 10,725 10,267 1458 458 209,764 4.27 9,700 10,770 21,070 1070 1,144,900 11.03 October 10,169 10,446 2277 277 76,729 2.72 November 11,570 10,692 1878 878 770,884 7.59 December 11,100 11,544 2444 444 197,136 4.00 September Total 119,702 X 9,975.2 Bias (total CFE) 11,274 Bias x 1106.2 Absolute Deviation Total 10,634 Absolute Deviation x (MAD) 886.2 Squared Error Total 14,032,986 Squared Error x (MSE) 1,169,415.5 Absolute % Error Total Absolute % error x (MAPE) 108.41 9.03 Tracking signal: CFE 4 MAD 5 11,274 4 886.2 5 1.44 aDt 5 0.6 , aDt 21 5 0.2, aDt 22 5 0.15, aDt 23 5 0.05 Source: Robert A. Novack, Ph.D. Copyright 2021 Cengage Learning. All Rights Reserved. May not be copied, scanned, or duplicated, in whole or in part. Due to electronic rights, some third party content may be suppressed from the eBook and/or eChapter(s). Editorial review has deemed that any suppressed content does not materially affect the overall learning experience. Cengage Learning reserves the right to remove additional content at any time if subsequent rights restrictions require it. 218 Chapter 7 not accurately reflect the patterns in demand. Second, using four periods to develop the forecast might not be the appropriate number of periods. Finally, the weighted moving average technique does not easily accommodate demand patterns with seasonal influences. In an attempt to improve on this forecast, another technique will be applied to the historical demand data. 7-6c Exponential Smoothing Exponential smoothing is one of the most commonly used techniques because of its simplicity and its limited requirements for data. It is also a technique that has variations on how it creates a forecast. The method used in this text is one example. Exponential smoothing needs three types of data: (1) an average of previous demand, (2) the most recent demand, and (3) a smoothing constant. The smoothing constant must be between 0 and 1. Using a higher constant assumes that the most recent demand is a better predictor of future demand. Formula 7.8 is used to calculate the forecast. At 5 a(Demand this period) 1 (1 2 a)(Forecast calculated last period) 5 aDt 1 (1 2 a)At21 7.8 Using the data contained in Table 7.2, the forecast using exponential smoothing is generated and can be seen in column 5. Assume that the average for the previous period (the average of the 12 periods was used for convenience) is 10,500 units. Alpha will be 0.50 in this example. The forecast for January is simply the average from the previous period (10,500 cases). The forecast for February is calculated as follows: Forecast 5 (0.5 3 10,210) 1 (0.5 3 10,500) 5 10,355 The forecast for March follows the same calculation. Forecast 5 (0.5 3 9,226) 1 (0.5 3 10,355) 5 9,791 Again, 0.50 was used for rounding. Table 7.5 shows the results of using the exponential smoothing forecast seen in Table 7.2. The error terms are CFE (bias) 5 24,235; MAD 5 853.58; MSE 5 893,754.75 ; MAPE 5 8.85 ; and, tracking signal 5 10.67. Exponential smoothing forecasts will lag actual demand. If demand is relatively constant, exponential smoothing will produce a relatively accurate forecast. However, highly seasonal demand patterns or patterns with trends can cause inaccurate forecasts using exponential smoothing. Table 7.6 summarizes the error terms for the three different forecasting techniques. While none of the three techniques were accurate, simple moving average scored best on three of the five error terms MAD, MSE, and MAPE with weighted moving average scoring best on CFE and tracking signal. As such it is reasonable to conclude that simple moving average is the best fit for the data. This is why multiple error terms should be used to assess the accuracy of a forecast. If only CFE were used to determine accuracy, a suboptimal technique would be used to develop future forecasts. This section analyzed the mathematical development of forecasts. The next section, Sales and Operations Planning (S&OP), will discuss how companies take this mathematical forecast and adjust it for sales and operations changes. Copyright 2021 Cengage Learning. All Rights Reserved. May not be copied, scanned, or duplicated, in whole or in part. Due to electronic rights, some third party content may be suppressed from the eBook and/or eChapter(s). Editorial review has deemed that any suppressed content does not materially affect the overall learning experience. Cengage Learning reserves the right to remove additional content at any time if subsequent rights restrictions require it. Demand Management Table 7.5 (1) PERIOD 219 Exponential Smoothing (2) Dt (3) Ft (4) (5) |D t 2 Ft | (6) Et (7) (|et | 4 Dt ) 3 100 DEMAND FORECAST Dt 2 Ft ERROR ABSOLUTE DEVIATION et2 ABSOLUTE % DEVIATION 2020 January 9,700 10,500 2800 800 640,000 8.25 February 8,765 10,355 21,590 1,590 2,528,100 18.14 March 9,231 9,791 2560 560 313,600 6.07 April 10,664 9,754 1910 910 828,100 8.53 May 9,233 10,490 21,257 1,257 1,580,049 13.61 June 8,679 10,104 21,425 1,425 2,030,625 16.42 July 10,166 9,620 1546 546 298,116 5.37 August 10,725 10,161 1564 564 318,096 5.26 9,700 10,725 21,025 1,025 1,050,625 10.57 October 10,169 10,468 2299 299 89,401 2.94 November 11,570 10,586 1984 984 968,256 8.50 December 11,100 11,383 2283 283 80,089 2.55 September Total 119,702 X 9,975.2 Bias (total CFE) Bias x 24,235 2352.92 Absolute Deviation Total 10,243 Absolute Deviation x (MAD) 853.58 Squared Error Total 10,725,057 Squared Error x (MSE) 893,754.75 Absolute % Error Total Absolute % error x (MAPE) 106.21 8.85 Tracking signal: CFE 4 MAD 5 24,235 4 853.58 5 24.96 Assume FJan 5 10,500 a 5 0.5 Source: Robert A. Novack, Ph.D. Copyright 2021 Cengage Learning. All Rights Reserved. May not be copied, scanned, or duplicated, in whole or in part. Due to electronic rights, some third party content may be suppressed from the eBook and/or eChapter(s). Editorial review has deemed that any suppressed content does not materially affect the overall learning experience. Cengage Learning reserves the right to remove additional content at any time if subsequent rights restrictions require it. 220 Table 7.6 Chapter 7 Forecast Accuracy Summary (1) (2) (3) (4) (5) BIAS (CFE) MAD MSE MAPE TRACKING SIGNAL Simple moving average 13,087 731.08 Weighted moving average 11,274 886.2 Exponential Smoothing 24,235 853.58 On the Line 862,696.75 7.43 4.2 1,169,415.5 9.03 1.44 893,754.75 8.85 24.96 S&OP Spans Horizons A major shift in S&OP over the last decade or two has been the analysis of more financial data and business what-ifs alongside demand and supply matching, according to Karen Bursa, executive vice president with Logility, a supply chain software provider. “One of the biggest opportunities that’s different today for many companies than say 15 years ago is the inclusion of more financial data within S&OP,” Bursa says. “So, as S&OP engages more senior executives, your supporting software needs to be able to look at plans not only in volumetric terms – SKU quantities or cases – but also in the financial terms of the business.” This more-evolved approach is referred to by many as integrated business planning (IBP). Typically done over a longer horizon than supply/demand matching under S&OP, IBP might examine alternatives such as whether a company should build a new plant, build or lease warehouse space, bring in contract manufacturers, or even acquire companies, says Bursa. Ideally, analytics for IBP should use the same data and planning models as software used for other horizons. “IBP is much more effective when you can use your current supply chain network as the foundation for those strategic plans,” Bursa says. An S&OP process might leverage multiple planning tools, from modeling of longer-term IBP issues, and the more classic demand planning, inventory planning, and replenishment planning. Again, says Bursa, planning should leverage a common data model, and it’s helpful if the vendor can offer a mechanism for cleansing master data from different systems. “Linking all of those time horizons together is a big part of the value proposition in sales and operations planning,” says Bursa. The benefits of software for S&OP includes less time spent by planner in prepping data, but also better plans that move the needle toward better performance. For example, Vista Outdoor, a manufacturer and marketer of sporting goods including brands such as Bushnell and Camelbak, has used Logility’s software to support S&OP. Since implementing Logility Voyager Solutions for S&OP in 2015, the company has cut the time for demand consensus preparation by 10%, increased in-stock rates by 14% on average, and reduced unplanned air expense by a third. The modeling for supply chain network design can set a useful foundation for more operational-level planning, says Toby Brzoznowski, a co-founder and executive vice president with LLamasoft, a supply chain software vendor. LLamasoft’s network design modeling and data management platform is now being leveraged for mid- to shorter-term planning as well, he adds. “With many of our clients, out of the gate, they focus on creating models more for the one-time decisions, but then they realize that the same model can be used to support other decision cycles that happen quarterly, monthly or weekly,” he says. Source: Adapted from Roberto Michel, “Smoother Execution through S&OP,” Logistics Management, April 2018, p. 48. Copyright 2021 Cengage Learning. All Rights Reserved. May not be copied, scanned, or duplicated, in whole or in part. Due to electronic rights, some third party content may be suppressed from the eBook and/or eChapter(s). Editorial review has deemed that any suppressed content does not materially affect the overall learning experience. Cengage Learning reserves the right to remove additional content at any time if subsequent rights restrictions require it. Demand Management 221 7-7 Sales and Operations Planning The previous section discussed statistical methods for arriving at a preliminary demand forecast for an organization. Historically, many organizations developed several functional forecasts for the same products during the same time period. It would not be unusual for a manufacturer to have a financial forecast, a manufacturing forecast, a marketing forecast, and a distribution forecast. What compounds the complexity of having multiple forecasts is that most times these functional forecasts did not agree. Marketing would forecast higher demands that neither manufacturing nor distribution could execute. Finance forecasts would be higher than marketing would be able to meet. It is necessary for an organization to arrive at a forecast internally that all functional areas agree upon and can execute. A process that can be used to arrive at this consensus forecast is called sales and operations planning (S&OP). The S&OP Benchmarking Consortium in the Center for Supply Chain Research adopted a five-step process in arriving at this consensus forecast.4 Figure 7.2 illustrates this process. Step 1 (run sales forecast reports) requires the development of a statistical forecast of future sales. This would be done using one or more of the forecasting techniques discussed in the previous section. Step 2 (demand planning phase) requires the sales and/or marketing departments to review the forecast and make adjustments based on promotions of existing products, the introductions of new products, or the elimination of products. This revised forecast is Figure 7.2 The Monthly Sales and Operations Planning Process Step 5 Step 4 Pre-S&OP meeting Executive S&OP meeting Decisions authorized game plan Recommendations and agenda for Executive S&OP Step 3 Step 2 Step 1 Demand planning phase Run sales forecast reports Supply planning phase Capacity constraints second-pass spreadsheets Management forecast first-pass spreadsheets Statistical forecasts field sales worksheets End of month Source: Thomas F. Wallace, Sales and Operations Planning: The How-To Book (Cincinnati, OH: T. F. Wallace and Company, 2000): 43. Copyright © 2000 by Thomas F. Wallace. Copyright 2021 Cengage Learning. All Rights Reserved. May not be copied, scanned, or duplicated, in whole or in part. Due to electronic rights, some third party content may be suppressed from the eBook and/or eChapter(s). Editorial review has deemed that any suppressed content does not materially affect the overall learning experience. Cengage Learning reserves the right to remove additional content at any time if subsequent rights restrictions require it. 222 Chapter 7 usually stated in terms of both units and dollars since operations are concerned with units and finance is concerned with dollars. Step 3 (supply planning phase) requires operations (manufacturing, warehousing, and transportation) to analyze the sales forecast to determine if existing capacity is adequate to handle the forecasted volumes. This requires analyzing not only the total volumes but also the timing of those volumes. For example, existing manufacturing capacity might be adequate if demand is stable over the forecast period. However, heavy promotions might produce a “spike” in demand that might exceed existing capacity. Two options to solve this capacity constraint are available. First, the promotional activity could be curtailed to bring demand to a more stable level. This could result in lost revenue. Second, additional manufacturing could be secured either by investing in more manufacturing capacity internally or securing contract manufacturing capacity externally. This would result in additional costs. The same types of capacity issues would need to be considered for both warehousing space and transportation vehicle capacity with similar resulting options if capacity does not meet demand: either curtail demand or invest in additional capacity. The decisions to these capacity issues are addressed in the next step. Step 4 (pre-S&OP meeting) asks individuals from sales, marketing, operations, and finance to attend a meeting that reviews the initial forecast and any capacity issues that might have emerged during Step 3. Initial attempts will be made during this meeting to solve capacity issues by attempting to balance supply and demand. Alternative scenarios are usually developed to present at the executive S&OP meeting (Step 5) for consideration. These alternatives would identify potential lost sales and increased costs associated with balancing supply and demand. The sales forecast is also converted to dollars to see if the demand/ supply plan meets the financial plan of the organization. Step 5 (executive S&OP meeting) is where final decisions are made regarding sales forecasts and capacity issues. This is where the top executives from the various functional areas agree to the forecast and convert it into the operating plan for the organization. Consensus among the various functional areas is critical in this meeting. Decisions regarding tradeoffs between revenue and costs are made here. Once the final plan is approved, it is important that the appropriate metrics are in place for each functional area to encourage compliance to the plan. For example, assume that the traditional operating metric for manufacturing is cost per pound manufactured. The lower the cost per pound, the better is the performance of the manufacturing group. However, the S&OP plan requires additional investment in capacity for manufacturing, which raises the cost per manufactured pound. This would make manufacturing performance unacceptable. While low cost is important, manufacturing has little or no control over the increased cost. A revised metric of compliance to schedule might be more appropriate. This new metric would reward manufacturing for making the planned quantities at the planned times. The point here is that the appropriate metrics must be in place for each functional area so that it is encouraged and rewarded for achieving the business plan. 7-8 Collaborative Planning, Forecasting, and Replenishment The S&OP process described how organizations are structuring their planning processes to arrive at a consensus forecast internally. The next logical step would be for members of a supply chain to also agree upon a consensus forecast. Many industry initiatives have Copyright 2021 Cengage Learning. All Rights Reserved. May not be copied, scanned, or duplicated, in whole or in part. Due to electronic rights, some third party content may be suppressed from the eBook and/or eChapter(s). Editorial review has deemed that any suppressed content does not materially affect the overall learning experience. Cengage Learning reserves the right to remove additional content at any time if subsequent rights restrictions require it. Demand Management 223 attempted to create efficiency and effectiveness through the integration of supply chain activities and processes. They have been identified by such names as quick response (QR), vendor-managed inventory (VMI), continuous replenishment planning (CRP), and efficient consumer response (ECR). All of these have had some success at integrating replenishment between supply chain members. However, they were all somewhat deficient in that they did not include a strong incentive for collaborative planning among supply chain members. One of the most recent initiatives aimed at achieving true supply chain integration is collaborative planning, forecasting, and replenishment (CPFR).5 CPFR has become recognized as a breakthrough business model for planning, forecasting, and replenishment. Using this approach, retailers, distributors, and manufacturers can utilize available Internet-based technologies to collaborate on operational planning through execution. Transportation providers have now been included with the concept of collaborative transportation management (CTM). Simply put, CPFR allows trading partners to agree to a single forecast for an item where each partner translates this forecast into a single execution plan. This replaces the traditional method of forecasting where each trading partner developed its own forecast for an item and each forecast was different for each partner. The first attempt at CPFR was between Walmart and Warner-Lambert (now a part of Johnson & Johnson) in 1995 for its Listerine product line. In addition to rationalizing inventories of specific line items and addressing out-of-stock occurrences, these two organizations collaborated to increase their forecast accuracy, so as to have the right amount of inventory where it was needed, when it was needed. The three-month pilot produced significant results and improvements for both organizations. This resulted in the adoption of CPFR by both Walmart and many of its other suppliers to manage inventories through collaborative plans and forecasts. Figure 7.3 shows the CPFR model as a sequence of several business processes that include the consumer, retailer, and manufacturer. The four major processes are (1) strategy and planning, (2) demand and supply management, (3) execution, and (4) analysis. Two aspects of this model are important to note. First, it includes the cooperation and exchange of data among business partners. Second, it is a continuous, closed-loop process that uses feedback (analysis) as input for strategy and planning. Figure 7.4 demonstrates how the process shown in Figure 7.3 is executed. As shown in Figure 7.4, CPFR emphasizes a sharing of consumer purchasing data (or point-of-sale data) as well as forecasts at retail among and between trading partners for the purpose of helping to manage supply chain activities. From these data, the manufacturer analyzes its ability to meet the forecasted demand. If it cannot meet the demand, a collaborative effort is undertaken between the retailer and manufacturer to arrive at a mutually agreed-upon forecast from which execution plans are developed. The strength of CPFR is that it provides a single forecast from which trading partners can develop manufacturing strategies, replenishment strategies, and merchandising strategies. The CPFR process begins with the sharing of marketing plans between trading partners. Once an agreement is reached on the timing and planned sales of specific products, and a commitment is made to follow that plan closely, the plan is then used to create a forecast, by stock-keeping unit (SKU), by week, and by quantity. The planning period can be for 13, 26, or 52 weeks. A typical forecast is for seasonal or promotional items that represent approximately 15 percent of sales in each category. The regular turn items, or the remainder of the products in the category, are forecast statistically. Then the forecast is entered into a system that is accessible through the Internet by either trading partner. Either partner may change the forecast within established parameters. Copyright 2021 Cengage Learning. All Rights Reserved. May not be copied, scanned, or duplicated, in whole or in part. Due to electronic rights, some third party content may be suppressed from the eBook and/or eChapter(s). Editorial review has deemed that any suppressed content does not materially affect the overall learning experience. Cengage Learning reserves the right to remove additional content at any time if subsequent rights restrictions require it. 224 Chapter 7 Figure 7.3 The CPFR Model Manufacturer Customer Scorecard Analysis Account Planning Performance Assessment Execution Monitoring Strategy & Planning Collaboration Arrangement Retailer Supplier Scorecard Exception Management Store Execution Consumer Logistics/ Distribution Order Fulfillment Logistics/ Distribution Market Joint Planning Vendor Business Managment Plan Category Management Buying/ Rebuying Order Generation Execution Production & Supply Planning Point-of-Sale Forecasting Replenishment Planning Sales Market Forecasting Data Analysis Order Planning/ Forecasting Demand Planning Demand & Supply Management Source: Larry Smith, “West Marine: A CPFR Success Story,” Supply Chain Management Review (March 2006): 31. Copyright © 2006 Reed Business Information, a division of Reed Elsevier. Reproduced by permission. Theoretically, an accurate CPFR forecast could be translated directly into a production and replenishment schedule by the manufacturer since both quantity and timing are included in the CPFR forecast. This would allow the manufacturer to make the products to order (based on the quantity and timing of demand) rather than making them to inventory, thus reducing total inventories for the manufacturer. The retailer would enjoy fewer out-ofstocks at the retail shelf. Although CPFR has not yet fully developed into a make-to-order environment, it has enjoyed the benefits of reduced supply chain inventories and out-ofstocks. West Marine employed the CPFR model with its suppliers and reaped some amazing results. More than 70 of its top suppliers are loading West Marine order forecasts directly into their production planning systems. In-stock rates at stores are close to 96 percent, forecast accuracy has risen to 85 percent, and on-time shipments are better than 80 percent.6 So, the use of collaborative efforts among supply chain partners can have positive results on the service and cost performance of these partners. Copyright 2021 Cengage Learning. All Rights Reserved. May not be copied, scanned, or duplicated, in whole or in part. Due to electronic rights, some third party content may be suppressed from the eBook and/or eChapter(s). Editorial review has deemed that any suppressed content does not materially affect the overall learning experience. Cengage Learning reserves the right to remove additional content at any time if subsequent rights restrictions require it. Demand Management 225 Figure 7.4 CPFR Business Model Develop Front-End Agreement 2 Create Joint Business Plan Distributor Exception Triggers Manufacturer Business Development Activities Create Sales Forecast Manufacturer Exception Triggers Identify Exceptions for Sales Forecast 4 Exception Items 5 Resolve/Collaborate On Exception Items Manufacturer Decision Support Data POS Data 6 Create Order Forecast Manufacturer Materials & Production Planning Order Forecast Frozen Forecast Identify Exceptions for Order Forecast 7 Distributor Exception Triggers Forecasting Distributor Decision Support Data Exception Criteria Exception Criteria 3 Planning Distributor Business Development Activities 1 Constraints Manufacturer Exception Triggers Exception Items Distributor Decision Support Data 8 Updated Data for Exception Items Consumer Long Term Resolve/Collaborate On Exception Items Short Term 9 Unresolved Supply Constraints Order/PO Produce Product Feedback Distributor Receiving Key: Product Delivery Execution Order Filling/ Shipment Execution Order filling feedback Distributor Activities Either/Joint Activities Replenishment Retail Store Order Generation Manufacturer Decision Support Data Manufacturer Activities Source: CPFR® is a registered trademark of the Voluntary Interindustry Commerce Standards (VICS) Association. Reproduced by permission of the Voluntary Interindustry Commerce Standards (VICS) Association. Copyright 2021 Cengage Learning. All Rights Reserved. May not be copied, scanned, or duplicated, in whole or in part. Due to electronic rights, some third party content may be suppressed from the eBook and/or eChapter(s). Editorial review has deemed that any suppressed content does not materially affect the overall learning experience. Cengage Learning reserves the right to remove additional content at any time if subsequent rights restrictions require it. 226 Chapter 7 SUMMARY Outbound-to-customer logistics systems have received the most attention in many companies; but even in today’s customer service environment, outbound and inbound logistics systems must be coordinated. Demand management may be thought of as “focused efforts to estimate and manage customers’ demand, with the intention of using this information to shape operating decisions.” Although many forecasts are made throughout the supply chain, the forecast of primary demand from the end user or consumer will be the most important. It is essential that this demand information be shared with trading partners throughout the supply chain and be the basis for collaborative decision making. Various approaches to forecasting are available, each serving different purposes. The S&OP process has gained much attention in industry today. It serves the purpose of allowing a firm to operate from a single forecast. The S&OP process is a continual loop involving participation from sales, operations, and finance to arrive at an internal consensus forecast. CPFR is a method to allow trading partners in the supply chain to collaboratively develop and agree upon a forecast of sales. This allows for the elimination of inventories held because of uncertainty in the supply chain. STUDY QUESTIONS 1. What are the differences and similarities between outbound and inbound logistics systems? Which types of industries would place heavier emphasis on outbound systems? On inbound systems? Explain your choices. 2. How do outbound logistics systems relate directly to the needs of the customer? 3. How can demand management help to unify channel members, satisfy customers, and solve customer problems? 4. What are some of the logistics problems that might arise when supply and demand for a product are not aligned properly? What are some of the methods used to soften the effects of this imbalance? 5. What are the basic types of forecasts? What are their strengths and weaknesses? 6. What are the basic elements of the S&OP process? How do marketing, logistics, finance, and manufacturing contribute to each element? 7. What are the critical elements of collaborative planning? What benefits do they provide for the supply chain? 8. What are the similarities between the CPFR and S&OP processes? What are the differences? Copyright 2021 Cengage Learning. All Rights Reserved. May not be copied, scanned, or duplicated, in whole or in part. Due to electronic rights, some third party content may be suppressed from the eBook and/or eChapter(s). Editorial review has deemed that any suppressed content does not materially affect the overall learning experience. Cengage Learning reserves the right to remove additional content at any time if subsequent rights restrictions require it. Demand Management 227 CASE 7.1 Tires for You, Inc. (TFY), founded in 1987, is an automotive repair shop specializing in replacement tires. Located in Altoona, Pennsylvania, TFY has grown successfully over the past few years because of the addition of a new general manager, Ian Overbaugh. Since tire replacement is a major portion of TFY’s business (it also performs oil changes, small mechanical repairs, etc.), Ian was surprised at the lack of forecasts for tire consumption for the company. His senior mechanic, Skip Grenoble, told him that they usually stocked for this year what they sold last year. He readily admitted that several times throughout the season stockouts occurred and customers had to go elsewhere for tires. Although many tire replacements were for defective or destroyed tires, most tires were installed on cars whose original tires had worn out. Most often, four tires were installed at the same time. Ian was determined to get a better idea of how many tires to hold in stock during the various months of the year. Listed below is a summary of individual tire sales by month: PERIOD TIRES USED 2018 October 9,797 November 11,134 December 10,687 2019 January 9,724 February 8,786 March 9,254 April 10,691 May 9,256 June 8,700 July 10,192 August 10,751 September 9,724 October 10,193 November 11,599 December 11,130 Ian has hired you to determine the best technique for forecasting TFY demand based on the given data. Copyright 2021 Cengage Learning. All Rights Reserved. May not be copied, scanned, or duplicated, in whole or in part. Due to electronic rights, some third party content may be suppressed from the eBook and/or eChapter(s). Editorial review has deemed that any suppressed content does not materially affect the overall learning experience. Cengage Learning reserves the right to remove additional content at any time if subsequent rights restrictions require it. 228 Chapter 7 CASE QUESTIONS 1. Calculate a forecast using a simple three-month moving average. 2. Calculate a forecast using a three-period weighted moving average. Use weights of 0.60, 0.30, and 0.10 for the most recent period, the second most recent period, and the third most recent period, respectively. 3. Calculate a forecast using the exponential smoothing method. Assume the forecast for period 1 is 9,500. Use alpha = 0.40. 4. Once you have calculated the forecasts based on the above data, determine the error terms by comparing them to the actual sales for 2020 given below: PERIOD TIRES USED 2020 January 10,696 February 9,665 March 10,179 April 11,760 May 9,150 June 9,571 July 8,375 August 11,826 September 10,696 October 11,212 November 9,750 December 9,380 5. Based on the three methods used to calculate a forecast for TFY, which method produced the best forecast? Why? What measures of forecast error did you use? How could you improve upon this forecast? Source: Robert A. Novack, Ph.D. Copyright 2021 Cengage Learning. All Rights Reserved. May not be copied, scanned, or duplicated, in whole or in part. Due to electronic rights, some third party content may be suppressed from the eBook and/or eChapter(s). Editorial review has deemed that any suppressed content does not materially affect the overall learning experience. Cengage Learning reserves the right to remove additional content at any time if subsequent rights restrictions require it. Demand Management 229 CASE 7.2 Playtime, Inc. manufactures toys for children under the age of 12 and has been in business for 50 years. While Playtime does not hold a major share of the toy market, it has experienced significant growth over the last five years because of its collaboration with major movie studios to introduce action figures to coincide with new movie releases. Playtime is a publicly held company. Playtime’s executive council consists of vice presidents of marketing (includes sales), operations (manufacturing), supply chain (procurement, inventory, warehousing, and transportation), and finance. This group is ultimately responsible for approving the forecast for the upcoming year. The forecast is developed using last year’s sales as historical data. This forecast is then given to the supply chain and operations groups to determine if capacity is sufficient to accommodate the new volumes. If capacity is sufficient, the forecast is then moved to finance where it is analyzed to determine if volumes are sufficient to satisfy the needs of the investors. Jim Thomas, manager of supply chain, and Gail Jones, manager of operations, had a meeting to discuss the first version of the forecast. “I know we use last year’s sales to project for next year, but this forecast has me worried,” said Jim. “One of our major movie studio partners is coming out with a blockbuster movie next year and we have no idea what the impact of that might be on our distribution capacity.” Gail agreed, saying “I know. We have the capacity right now based on this forecast, but if volumes surge we are in trouble from a manufacturing perspective.” Jim and Gail also know that if the projected volume does not satisfy the needs of inventors, finance will send the forecast back to marketing to increase volumes until financial goals will be met. This forecast process has resulted in a disconnect among the supply chain, operations, and marketing functions within Playtime. The managers in these functions typically end up developing their own forecasts based on what they think demand will really be, regardless of what finance presents to the investors. Over the last several years, this has presented some problems for the operations and supply chain areas because of manufacturing capacity issues (toys are very seasonal) and inventory issues. Although Playtime has been able to handle these issues, Jim and Gail are very concerned about next year because of the uncertainty the new movie release will have on the demand for their toys. CASE QUESTIONS 1. What are the issues associated with Playtime’s current forecasting process? What impacts, negative or positive, does this process have on the marketing, operations, supply chain, and finance functions? 2. Using the S&OP process discussed in this chapter, design a more effective and efficient forecasting process that will mitigate the negative impacts you identified in question 1. Source: Robert A. Novack, Ph.D. Copyright 2021 Cengage Learning. All Rights Reserved. May not be copied, scanned, or duplicated, in whole or in part. Due to electronic rights, some third party content may be suppressed from the eBook and/or eChapter(s). Editorial review has deemed that any suppressed content does not materially affect the overall learning experience. Cengage Learning reserves the right to remove additional content at any time if subsequent rights restrictions require it. Copyright 2021 Cengage Learning. All Rights Reserved. May not be copied, scanned, or duplicated, in whole or in part. Due to electronic rights, some third party content may be suppressed from the eBook and/or eChapter(s). Editorial review has deemed that any suppressed content does not materially affect the overall learning experience. Cengage Learning reserves the right to remove additional content at any time if subsequent rights restrictions require it.