Chapter 4 - Establishing a New Analytical Process (Method Development) PDF
Document Details
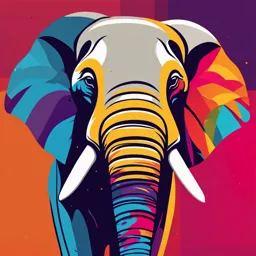
Uploaded by EnthusiasticLlama
Tags
Related
Summary
This chapter explores various aspects of establishing a new analytical process, including student learning outcomes, introduction to the concept of methods, and method development. It goes into detail about method development (analysis/instrument), method development and optimization (technique), and objectives of optimization, as well as various approaches to optimization including traditional and statistical design. A practical example of an OFAT approach is also included.
Full Transcript
CHAPTER 4 Student Learning Outcomes: At the end of this chapter, students should be able to: 1) differentiate between the experimental design approach and conventional method. 2) state the advantages of using experimental design approach. 3) explain the validation parameters ...
CHAPTER 4 Student Learning Outcomes: At the end of this chapter, students should be able to: 1) differentiate between the experimental design approach and conventional method. 2) state the advantages of using experimental design approach. 3) explain the validation parameters INTRODUCTION Method = A systematic procedure, technique, or way of doing something, especially in accordance with a definite plan (ordered sequence of fixed steps) Test method = A definitive procedure for the identification, measurement, and evaluation of a material, product, system, or service that produces a test result. A scientific method consists of the collection of data through observation and experimentation, the formulation and testing of hypotheses. Quality standards often favor the use of standard methods or collaboratively tested methods whenever possible. METHOD DEVELOPMENT Method development is the setting up of an analytical procedure that will be appropriate for the analysis of a particular sample. Method Development is a steps process that determines test method capabilities through selection and optimization of analytical test parameters. It provides a high degree of assurance that the test method will meet or surpass the established requirements. The method developed shall have been validated appropriately before use. As method-development proceeds, regular review should be carried out. Importance of Method Development Improvements in performance testing can be made by employing method development principles to standard test methods, when applied to new products or materials. During the method development process, the optimum test parameters are identified to ensure applicability and reliability of the data. Method Development (Analysis/Instrument) 1) Choice of analytical technique: Volatile substances are best separated using GC/HPLC; Provides the best resolution Shortest analysis time Highest sensitivity 2) Choice of phase system: based on the interactive character of the compound to be analysed, polar/non-polar/ionic 3) Detector selection which provide the required sensitivity, the necessary linearity and if needed the desired specificity. Method Development & Optimization (Technique) Critical test parameters need to be identified and evaluated through experiments.designed to determine a suitable operating range for each one. Critical test parameters were defined as the operating factors that, when varied (limited change), significantly affect the results. Method development activities should be documented. Objectives of Optimization 1) improve the efficiency of method. 2) reduce the cost of chemicals and operations by reducing the number of experiments. 3) environmentally friendly method Optimization Design Develop and optimize method by using one of the following approaches: Traditional: stepwise incremental (one-factor-at-a-time, OFAT) approach base on results from previous experiment. Statistical Design: uses statistical tools to study multiple factors simultaneously TRADITIONAL APPROACH One-factor at a time (OFAT) Change One Separate factor at a Time (COST) Holds all factors but one constant. Vary the levels of the factor of interest until we get the “best value”. Repeat for 2nd, then 3rd variables and so on… Example OFAT Journal of Separation Science (2018), SPME for determination NSAIDs via HPLC-UV A. Sample pH varies 1-5 Salt 0% Ext time 30min Desorp. solv. MeOH DT = 3 min B. Salt varies from 0-5% Sample pH 2 Ext time 30min Desorp. solv. MeOH DT = 3 min C. DS varies Sample pH 2 Salt 2% Ext time 30 min DT 3 min Problems associated with the OFAT approach: Does not lead to real optimum. Inefficient, unnecessarily many runs. Provides no information about what happens when factors are varied simultaneously (ignores interactions). Provides less information about the variability of the response. Isolated, unconnected experiments. Slow growth of knowledge as no mapping of experimental space. CHAPTER 4 STATISTICALLY DESIGN APPROACH Design of Experiments, DoE An experiment that uses statistical tools and methods to successfully study multiple factors in a single experiment. DoE is the methodology of how to conduct and plan experiments in order to extract the maximum amount of information in the fewest number of experiment runs. Design of Experiments (DOE) is; Planning experiments or tests to save resources (time and money) Analyzing results of experiments or tests to characterize your system DoE involves designing a set of ten to twenty experiments, in which all relevant factors are varied systematically. Analysis was conducted using ANOVA. analysis of variance is a statistical procedure used to test the degree to which two or more groups vary or differ in an experiment. Analysis form ANOVA help to identify; 1) optimal conditions 2) factors that most influence the results 3) existence of interactions and synergies between factors. Advantages of DoE; Requires less resources, time, money and materials (small number of experiments) Precisely estimate the effect of each factor. Interaction between factors can be estimated. Factor effects are studied over a wider range of level of the other factors. Comparison method Conventional Method Statistical Method One factor at a time (OFAT) / Design of Experiment (DoE) COST Time consuming Reduce lead time and improve efficiency Cannot interpret the interaction Could explain the interaction between 2 or more variables between the process variables (cause-and-effect relationships) Use the modeling to predict the behavior of process variables Phases in DoE Process Planning Experiment What is the purpose? What are the objectives? Identify factors, factor ranges and types of factors (quantitative or Designing Experiment qualitative). What is possible, experimentally, financially, environmentally? Conducting Experiment Analyzing Results Confirming Predicted Results Planning Determine the objective of the study experiment Variable selection The important factors such as extraction time, sample pH and desorption time (Qualitative or Quantitative) Designing Identify the design by selecting the level and range for each process variable. experiment Factor i. No of factors ii. Block iii. Level iv. Range Provide low to high input/factor Input: Extraction time Output: Response (peak area) Conducting Selecting the design of experiment (DOE) - Full factorial, CCD, Box-Behnken experiment Run the experiment according to the number of experiment design software ex:- Design Expert Analyzing Analyze the results –ANOVA (analysis of variance) result Choose the significant factors that influence the extraction from the ANOVA analysis The adequacy of the fitted model is checked by ANOVA using Fisher F-test The fit quality of the model can also be checked from their coefficient of correlation (R) and coefficient of determination (R2) Confirming Validate the results obtained by comparing the experiment value and the predicted Predicted value result Example of question: An analytical method for the determination of furan in vegetables by solid phase microextraction-gas chromatography-mass spectrometry (SPME-GCMS) was developed and validated. Design of experiment (DoE) was used to investigate the effects of temperature and extraction time. ANOVA was used to find the optimal SPME extraction. Discuss each step involved in method development. Example of answer: Planning – What are the objectives? – Identify factors, factor ranges and types of factors (quantitative or qualitative) Designing Experiment – Identify the design; eg Central Composite Design – Identify the Input: factors (eg. in this study extraction time, extraction temperature and desorption time) Output: Responses (eg. In this study amount of furan detected in vegetables) Conducting Experiment – Run the experiment according to the number of experiment design by the experimental design software Analyzing Results – Analyse the results obtained from all experiments. Choose the significant factor from the ANOVA analysis. Confirming Predicted Result Goal of DoE 1) To optimize the costs (by conducting as few experiments as possible) 2) to learn how the inputs (factors) affect the output (responses) 1 Screening many variables to determine which have the 2 largest impact on quality Finding optimal characteristics settings of the most important factors 3 Finding robust operating conditions where the process is insensitive to variations in uncontrollable noise factors Requirements of DoE Two types of variables: factors and responses Factors: input parameters that you can change eg time the values of input parameters can consist of several levels: 2 min, 3 min, 10 min. Responses: should provide useful information about the quality of the process eg; percent yield Selection of factors, levels and ranges: Based on previous research, provided range (low to high) Examples of Factors and Responses GROWING TOMATOES ; Input (factors): Fertilizer; soil pH; Seed hybrid; Water Output (responses): Yield CHEMICAL REACTION ; Input (factors): Pressure; Temperature; Catalyst concentration Output (responses): percent yield CHAPTER 4 How to select DoE Choose based on the research objectives (i) Comparative objective: We have several factors under investigation, but the primary goal is to make a conclusion about one a-prior important factor the question of interest is whether or not that factor is "significant", (i.e., whether or not there is a significant change in the response for different levels of that factor), then we have a comparative problem and we need a comparative design solution. (ii) Screening objective: To select out main factors which have the largest impact on quality characteristics. The primary purpose of the experiment is to select or screen out the few important main effects from the many less important ones. (iii) Response Surface Method (RSM) to estimate interaction, and therefore give us an idea of the (local) shape of the response surface we are investigating. RSM designs are used to: Find improved or optimal process settings Troubleshoot process problems and weak points Make a product or process more robust against external and non-controllable influences. "Robust" means relatively insensitive to these influences. (iv) Optimizing responses when factors are proportions of a mixture. have factors that are proportions of a mixture and we want to know what the "best" proportions of the factors to maximize (or minimize) a response, then we need a mixture design. (v) Optimal fitting of a regression model. If we want to model a response as a mathematical function (either known or empirical) of a few continuous factors and you desire "good" model parameter estimates (i.e., unbiased and minimum variance), then you need a regression design. to estimate a precise model, quantifying the dependence of response variable(s) on process inputs. Examples of DoE Full Factorial Designs Fractional Factorial Designs Central Composite Designs Plackett-Burman Design The choice of an experimental design Box-Behnken Designs depends on the objectives of the experiment and the number of factors to be investigated Full Factorial Design A factorial design consists of conduction all possible combinations of variables and levels Effects of all variables and all possible interactions can be determined. No of trials increase with no of variables and levels Fractional Factorial Design Retains some advantages of full factorial Permit study of large number of variables with reduced number of runs Some interaction effects can be confounded (confusion by many conflict situations) Can be confounded by alias’ Lose multifactor interactiors Central Composite Design Specially suited to fitting second and higher order surfaces Total number of treatment combinations = 2n +2n +1 If n = 2, treatments = 9 If n = 3, treatments = 15 If n = 4, treatments = 27 (compared to 81 for full factorial Useful for both screening and response surface 8 Analyzing the Design Multiple regression Analysis of Variance (ANOVA) Response Surface Plot Significant of the model coefficient The p-values help determine whether the relationships that you observe in your sample also exist in the larger population. The p-value for each independent variable tests the null hypothesis that the variable has no correlation with the dependent variable. A low p-value (< 0.05) indicates that you can reject the null hypothesis. If a p-value is 0.01< p < 0.05, it can be classifieds as strong evidence that the data has non-zero correlation. Changes in the independent variable are associated with changes in the response at the population level. Example: The regression output example below shows that the South and North predictor variables are statistically significant because their p-values equal 0.000. On the other hand, East is not statistically significant because its p-value (0.092) is greater than the usual significance level of 0.05. Example of DoE application Experimental Design Approach for the Optimization of Solid Phase Microextraction (SPME) for the Extraction of Benzene and Toulene from Water Identifying the factors which may affect the result of an experiment. Extraction time, Extraction temperature, Desorption time Choosing the experimental design Central composite design (Design-Expert Software Version 6.0.6) Coded Level Extraction Extraction Time Desorption Time Temperature -α 27oC 0.5 min 13 sec -1 30oC 6 min 40 sec 0 34oC 14 min 80 sec 1 38oC 22 min 120 sec α 41oC 27 min 147 sec Using statistical analysis to separate and evaluate the effects of the various factor involved. ANOVA + RSM FACTORS: Sets of experiments generated from RESPONSE: Amount of Benzene and Central Composite Design. Toluene extracted from water Extraction Time Extraction Temp. Desorption time Benzene extracted Toluene extracted Run Order (min) (oC) (sec) (GC area count) (GC area count) 1 14 34 80 61156 139925 2 14 34 80 56382 124331 3 14 40 80 59948 137036 4 0.5 34 80 12923 22252 5 14 34 13 0 0 6 14 34 80 51757 113327 7 27 34 80 53203 118705 8 22 38 40 50194 109196 9 14 34 80 48598 102567 10 22 38 120 51120 108560 11 6 38 40 53340 98946 12 6 30 120 48690 89840 13 22 30 120 70097 142073 14 14 34 147 63299 126841 15 14 34 80 61831 118660 16 14 27 80 58813 112530 17 6 38 120 51560 89957 18 14 34 80 59205 115688 19 22 30 40 64728 123955 20 6 30 40 45250 78363 Results of Significance test for benzene using the Quadratic Model Et = Extraction time. Variable p value T = Extraction temperature. Et 0.0737 Dt = Desorption time. T 0.7029 Dt 0.0546 Significant variable if p value is less than 0.05 Et2 0.1845 T2 0.3155 Dt2 0.1501 EtT 0.2951 EtDt 0.9106 TDt 0.8151 At the 95% confidence level (p is less than 0.05) Therefore, no variables are significant. Results of Significance test for toluene using the Quadratic Model Et = Extraction time. Variable p value T = Extraction temperature. Et 0.0155 Dt = Desorption time. T 0.8934 Significant variable if p value is Dt 0.0406 less than 0.05 Et2 0.1009 T2 0.3853 D t2 0.0561 EtT 0.3851 EtDt 0.8474 TDt 0.6168 At the 95% confidence level ( p is less than 0.05) two parameters, extraction time and desorption time are significant Response surface 3-D plot 132859 113143 93427 Toluene Recovery 73710 X = A: Extraction Time Toluene Recovery Y = C: Desorption Time 53994 Actual Factor B: Extraction Temperature = 34.00 6.0 120 10.0 100 14.0 80 Extraction Time (min) 18.0 60 Desorption Time (sec) 22.0 40 Example of DoE application: UiTM PG student’s research Arabica Coffee Beans Roasting Method: High In Flavour Compounds Pyrazine and Low In Acrylamide Roasting is the important step in the production of coffee because it enables the development of flavour, aroma and colour and also at the same time may lead to the formation of undesirable compounds such as acrylamide. Method: Coffee beans were roasted using a roaster (PROBAT) based on RSM with extraction conditions; temperature (T) of 150 °C- 185 °C and time (t) of 15-30 minutes (Note: T and t are factors) Central composite design arrangement for independent variables A (Temperature, °C) and B ( Time, minute) and their responses; 2-methylpyrazine, 2,3- dimethylpyrazine, 2,5-dimethylpyrazine, 2,3,5-trimethylpyrazine, 2,3,5,6- tetramethylpyrazine and acrylamide. 2,3- 2,5- 2,3,5- 2,3,5,6- Temperature Time 2-methyl Run dimethyl dimethy trimethyl tetramethy Acrylamide (°C) (minute) pyrazine pyrazine lpyrazine pyrazine lpyrazine 1 167.5 22.5 229.0 546.0 138.2 671.8 536.0 976.0 2 155.2 17.2 69.0 193.0 78.2 83.0 274.8 155.4 3 179.9 27.8 38.0 356.6 133.2 25.0 94.6 245.8 4 155.2 27.8 164.6 381.2 137.4 81.8 149.6 798.8 5 179.9 17.2 44.6 449.8 121.2 75.2 92.8 1754.4 6 167.5 22.5 326.4 443.4 216.6 703.2 485.4 951.6 7 167.5 22.5 239.0 477.2 173.2 778.0 468.8 109.0 8 185.0 22.5 51.2 414.4 217.4 237.8 166.4 676.4 9 167.5 15.0 103.2 289.6 73.0 213.4 124.6 531.0 10 167.5 22.5 249.2 585.6 178.6 531.2 390.6 114.0 11 167.5 22.5 335.6 598.2 174.8 486.0 369.6 96.0 12 167.5 30.0 139.0 92.6 247.2 312.0 103.8 887.2 13 150.0 22.5 36.4 54.6 27.2 32.6 145.4 294.6 14 167.5 22.5 315.8 543.0 188.8 517.4 350.0 60.4 2-Methylpyrazine The optimized conditions DESIGN-EXPERT Plot Sqrt(2 MP) X = A: Temperature (temperature and time) for Y = B: Time roasting of Arabica coffee 16. 8527 14. 4724 beans in order to produce 12. 0921 high quality of coffee beans Sqrt(2 MP) 9. 71177 7. 33146 with high concentration of flavour and low 27. 80 179. 90 concentration of 25. 15 22. 50 167. 53 173. 71 undesirable acrylamide B : T im e was temperature at 19. 85 161. 34 A : T e m p e ra t u re 17. 20 155. 15 167.68 °C for 22.50 Response surface 3-D plot minutes. CHAPTER 4 METHOD VALIDATION Method validation is the process of proving that an analytical method is acceptable for its intended purpose. verifying that a method is fit-for-purpose, i.e suitable for solving a particular analytical problem The process consists of the establishment of the performance characteristics and limitations of the method. Method validation is necessary in analytical laboratory to ensure that reliable analytical procedures are used under the defined conditions. ISO definition of method validation The confirmation by examination and the provision of objective evidence that the particular requirements for a specific intended use are fulfilled. confirmation = from comparison of requirement with evidence. objective evidence = experimental data (method performance parameters). specific intended use = analytical requirements. Importance of Method Validation To increase the value of test results To justify customer’s trust To trace criminal To prove what we claim is true Examples: – To value goods for trade purposes – To support health care – To check the quality of drinking water to assure that an analytical method is accurate, reproducible and rugged over the specific range Compliance to Standards: FDA, GLP, ISO 17025, cGMP etc. Assurance of Laboratory Reliability Examples of Standards FDA: Food and Drug Administration Responsible for protecting and promoting public health through the regulation and supervision of food safety, tobacco products, dietary supplements, prescription and over-the-counter pharmaceutical drugs (medications), vaccines, biopharmaceuticals, blood transfusions, medical devices, electromagnetic radiation emitting devices (ERED), veterinary products, and cosmetics. cGMP – current good manufacturing practice Provide systems that assure proper design, monitoring, and control of manufacturing processes and facilities. Example human pharmaceuticals. Consumers expect that each batch of medicines they take will meet quality standards so that they will be safe and effective. GLP - good laboratory practice set of principles intended to assure the quality and integrity of non-clinical laboratory studies that are intended to support research or marketing permits for products regulated by government agencies. Outline 2 3 1 Method Method Development and validation Quality in Validation selected Analytical samples Laboratory Validation of an analytical method is primarily concerned with: – the identification of the sources of potential errors. – quantification of the potential errors in the method. A method that is valid in one situation could be invalid in another situation. Validation of analytical procedures is the process of determining the suitability of a given methodology for providing useful analytical data. The process of the method validation begins with the planned and systematic collection by the application of the validation data to support the analytical procedures. When Method Need to be Validated 1) Before their introduction into routine use. 2) Whenever the conditions change for which the method has been validated, e.g., established methods are used in different laboratories/different analyst etc. 3) Revision of established methods/instruments e.g: whenever the method is changed, and the change is outside the original scope of the method. 4) Comparison of methods Method validation data submitted should INCLUDE the following issues: Accuracy Precision Method validation Limit of Detection (LOD) Limit of Quantitation (LOQ) Specificity Linearity and Range Ruggedness/Robustness System Suitability Example of method validation in journal/article Validation data of µ-EME of NSAIDs from spiked tap and river water Sample Analyte Linear Coefficient of LOD, EF Precision range, determination, (µg L-1) (RSD, %) (µg L-1) R2 (n = 3) Tap water KET 0.5-500 0.9984 0.21 71 7.5 DIC 0.5-500 0.9979 0.18 82 8.8 IBU 0.5-500 0.9953 0.30 73 9.2 MEF 0.5-500 0.9989 0.14 86 6.5 River water KET 0.5-500 0.9954 0.28 62 4.1 DIC 0.5-500 0.9942 0.22 74 6.6 IBU 0.5-500 0.9976 0.42 65 9.4 MEF 0.5-500 0.9991 0.15 81 3.6 R2 = 0 - the dependent variable cannot be predicted from the independent variable. R2 = 1 - the dependent variable can be predicted without error from the independent variable. R2 between 0 and 1 indicates the extent to which the dependent variable is predictable. example R2 = 0.10 means that 10 percent of the variance in Y is predictable from X CHAPTER 4 ACCURACY Accuracy is the measure of the closeness of agreement between the measured value and true value (accepted reference value). The true value for accuracy assessment can be obtained in several ways: i. compare results of the method with results from an established reference method. ii. analyzing a sample with known concentrations (certified reference material), and comparing the measured value with the true value as supplied with the material. iii. If such certified reference material is not available, a blank sample matrix of interest can be spiked with a known concentration by weight or volume and determined its recovery. ISO Definition Accuracy, Trueness, Bias Accuracy is the closeness of agreement of a test result and the accepted reference value. The term accuracy should only be used when dealing with an individual result.. Trueness is the closeness of agreement between the average value obtained from large set of test results and an accepted reference value. To evaluate the performance of a method, trueness is more useful because we are dealing with set of results. Bias is the different of mean value of sets of measurement and reference value. Calculation of bias; The determination of Accuracy usually requires a “gold standard” or an accepted method to which a new method can be compared. Accuracy assessment is normally calculated as Percent Recovery (%R) and is done by analyzing Matrix Spikes. % Recovery = amount detected x 100 amount spike The concentration should cover the range of concern and should particularly include one concentration close to the quantitation limit. Analyte recovery at different concentrations (Association of Official Analytical Chemists, AOAC 2002) Active Ingredient Analyte ratio Unit Mean recovery [%] [%] 100 1 100% 98-102 >=10 10-1 10% 98-102 >=1 10-2 1% 97-103 >=0.1 10-3 0.1 % 95-105 0.01 10-4 100 ppm 90-107 0.001 10-5 10 ppm 80-110 0.0001 10-6 1 ppm 80-110 0.00001 10-7 100 ppb 80-110 0.000001 10-8 10 ppb 60-115 0.0000001 10-9 1 ppb 40-120 PRECISION Precision is the degree of agreement among individual test results, when the procedure is applied repeatedly to multiple samplings of a homogeneous sample. Precision is the closeness of agreement between independent test results obtained under stipulated conditions. Precision is usually expressed in terms of standard deviation or Relative Standard Deviation percent (RSD). It is often expressed as a percentage. The two most common precision measures are: “repeatability” “reproducibility” How to measure the precision? At least, 5 or 6 determinations of three different matrices, at 2 or 3 different concentrations should be done, and the relative standard deviation was calculated. The acceptance criteria for precision depend very much on the type of analysis: Pharmaceuticals quality control, precision of better than 1 % RSD Biological samples (blood, urine) the precision is more like 10%- 15% Environmental and food samples, the precision is very much dependent on the sample matrix, the concentration of the analyte and on the analysis technique. It can vary between 2% and more than 20%. Repeatability (r) Reproducibility (R) same method on identical test items, same method on identical test items, in the same laboratory, by the same in different laboratories, with different operator, using the same equipment, operators, using different equipment. within short time intervals. e.g: Within- day variability. Usually, the sample is analyzed by a number of laboratories for comparative It gives a measure of variability to be purposes (Inter-laboratory test). expected when a method is performed by a single analyst on the same Determined by analyzing aliquots from equipment over a short timescale. homogeneous lots in different Variability is expected when a sample laboratories with different analysts and is analyzed in duplicate by using operational and environmental conditions that may differ from but are still within the specified parameters of the method. Factor affecting a method’s reproducibility 1) Differences in room temperature and humidity 2) Operators with different experience and thoroughness 3) Equipment with different characteristics, e.g. delay volume of an HPLC system 4) Variations in material and instrument conditions, e.g. in HPLC, mobile phases composition, pH, flow rate of mobile phase 5) Equipment and consumables of different ages 6) Columns from different suppliers or different batches 7) Solvents, reagents and other material with different quality Intermediate Precision Intermediate precision is performed by repeating testing on the different combinations of analyst, equipment, reagents and time within one laboratory. It is recommended to do 6-10 measurements on each of repeatability study. For example, calculate the precision under repeatability conditions (same method, same analyst but different equipment; or same equipment same method but different analyst). Requirements: According to International Conference on Harmonisation (ICH), both repeatability and intermediate precision should be tested. Evaluation of Precision 1) Measurement of 10 samples (n=10) fo each concentration level. 2) Calculate mean, 3) The standard deviation is a measure of how precise the average is, 4) Standard deviation, s = 5) The relative standard deviation (RSD) is often times more convenient. It is always expressed as percentage (%) 6) RSD (%) = Estimated precision data as a function the of analyte concentration Analyte concentration versus precision within or between days Analyte % Analyte ratio Unit RSD (%) 100 1 100% 1.3 10 10-1 10% 2.8 1 10-2 1% 2.7 0.1 10-3 0.1 % 3.7 0.01 10-4 100 ppm 5.3 0.001 10-5 10 ppm 7.3 0.0001 10-6 1 ppm 11 0.00001 10-7 100 ppb 15 0.000001 10-8 10 ppb 21 0.0000001 10-9 1 ppb 30 The lower the concentration of analyte, the higher the %RSD was obtained Accuracy vs Precision Example: “If you are playing soccer and you always hit the left goal post instead of scoring, then you are not………………, but you are …………………” CHAPTER 4 LIMIT OF DETECTION (LOD) LOD is the lowest concentration of an analyte in a sample that can be detected, but not necessarily quantified, under the stated conditions of the test. LOD of a method may be defined as the concentration of analyte which gives rise to a signal that is significantly different from the negative control or blank. The LOD is the lowest concentration of analyte that can be distinguished from background. The results obtained at the LOD are not necessarily precise or accurate or quantitated. Usually is expressed as concentration of analyte generating an instrument response equivalent to three times the noise (S/N = 3) LIMIT OF QUANTIFICATION (LOQ) LOQ is the lowest concentration of an analyte in a sample that can be determined with acceptable precision and accuracy under the stated conditions of test A typical acceptable signal-to-noise ratio 1:10 (S/N=10) A number of samples with decreasing amounts of the analyte are injected six times. The calculated RSD% of the precision is plotted against the analyte amount. The amount that corresponds to the previously defined required precision is equal to the limit of quantitation. LOQ with the EURACHEM method Linear regression Signal-to-noise Blank ratio (S/N) Determination Estimating LOD & LOQ Signal-to-noise ratio (SNR) The noise value was calculated based on the peak height of the blank (solvent) around the retention time of each analyte. Procedure: 1) Run blank and the lowest concentration of analyte 2) Run 10 replicates independently 3) Measure the peak height values (noise magnitude was measured by auto-integrator) 4) Calculate LOD: S/N = 3, and 5) LOQ: S/N = 10 LOD, LOQ and Signal-to-Noise Ratio (SNR) LOQ Signal to Noise = 10:1 LOD Signal to Noise = 3:1 Noise Blank Determination Analysis of independent sample blanks (10 replicates), and calculate the mean concentration and the standard deviations of the blank results LOD ≅ xbi + 3sbi LOQ ≅ xbi + 10 sbi xbi = mean concentration of the blank sbi = standard deviation of the blank Linear Regression Based on linear calibration curve: y = a + bx LOD ≅ 3sa b y: instrument réponses x: analyte concentration 10 sa a: y-intercept LOQ ≅ b: slope b sa = standard deviation of the blank b = slope of the calibration curve SPECIFICITY Degree to which the measured response is due to the analyte of interest only and not to other substances expected to be present in sample matrix. Defines the ability of the method to determine the analyte of interest to the exclusion of other relevant components. Defines the identity of an analyte among a mixture of similar components in a sample where the identity of the components is not important. It can be achieved by analysing either a known analyte among a mixture of structurally similar compounds (+) or a mixture of structurally similar molecules without the analyte (-). SELECTIVITY Describes the ability of an analytical method to differentiate various substances in a sample. Should be able to differentiate the analyte(s) of interest and IS [internal standard] from endogenous components in the matrix or other components in the sample.” Selectivity is like specificity except that the identification of all components in a mixture is mandatory. From the example above, selectivity requires identification of all the keys in the bunch and not just the one that opens the lock Sometimes, it is difficult to identify a compound by using one test alone. Therefore, another method needs to be used to give more information. Example: UV/Vis spectra of a drug molecule is used for identification purposes, however, the UV spectra of the drug is the same as the UV spectra of some drug impurities. Thus, the UV/Vis method on its own is not specific. But, by combining UV/Vis with another method ie. HPLC, the compound of interest was resolved from any other components of the sample and that the peak of interest wasn’t overlapped with another interfering peak – UV-Vis/ HPLC Application of GC/MS, LC/MS Examples of pure and impure HPLC peaks The chromatographic signal does not indicate any impurity in either peak. Spectral evaluation, however, identifies the peak on the left as impure. LINEARITY & RANGE Linearity The linearity of a method is its ability to provide measurement results that are directly proportional to the concentration of the analyte, or are directly proportional after mathematical transformation. Range The range of the method is the area between the lower and the upper limits of quantitation that is also linear. Within the range of the method, results are accurate, precise and “linear”. How to determine linearity & range 1) Five concentration levels are required to allow detection of curvature in the plotted data. Standard should be prepared and analyzed a minimum of three times. Measure the responses. 2) Plot the graph analyte concentration vs responses. 3) The line generated should be submitted, together with slope, intercept and correlation co-efficient data. 4) The measured slope should demonstrate a clear correlation between response and analyte concentration. 5) Acceptability of linearity data is often judge by examining the correlation coefficient r2 and y-intercept of the linear regression line for the response versus concentration plot. 6) r > 0.999 is generally considered as evidence of acceptable fit of the data to the regression line over the range (nominal ± 20%). Graphical presentations of linearity plot of a caffeine sample using HPLC. Plotting the sensitivity (response/amount) gives clear indication of the linear range. Plotting the amount on a logarithmic scale has a significant advantage for wide linear ranges. Rc = Line of constant response. 16 ROBUSTNESS Measure of its capability to remain unaffected by small, but deliberate variations in method parameters and provides an indication of its reliability during normal usage. The intra-laboratory (within/inside lab) study to examine the behaviour of an analytical process when small changes to the environment and/or operating conditions are made is called the ruggedness test eg: mobile phase composition, temperature, flow rate involves internal factors to the method How to test robustness of method? Example: Effects of the following changes in chromatographic conditions will be determined: Methanol content in mobile phase adjusted by + 2% Mobile-phase pH adjusted by + 0.1 pH units Column temperature adjusted by + 5oC. If these changes are within the limits that produce acceptable results, they will be incorporated in the method procedure or if the changes is insignificant, then the method is robust. RUGGEDNESS The degree of reproducibility of test results obtained by the analysis of same samples under a variety of conditions. eg: different lab, analyst, assay temperature, instruments, different lot of reagents, days etc. involves external factors to the method. SYSTEM SUITABILITY System suitability is the checking of a system to ensure system performance before or during the analysis of unknowns. To check system (equipment, electronics, samples, technique) is working properly before any samples are analysed. Parameters such as plate count, tailing factors, resolution and reproducibility (%RSD retention time and area for 6 repetitions) are determined and compared against the specifications set for the method. TYPES OF ERROR 3 types (Gross, random and systematic errors): 1) Gross errors - errors that are so serious that there is no real alternative to abandoning the experiment eg., instrument breakdown. 2) Random errors - these cause replicate results to differ from one to one another, so that the individual results fall on both sides of the average value. (Random errors affect the precision or repeatability of an experiment) 3) Systematic errors - these cause all the results to be in error in the same sense (whether too high or too low from the true value). Normally is called the bias of the measurements. Refer to CHM421 notes