Chapter 4 Back Up Book for Printing PDF
Document Details
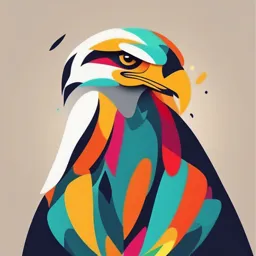
Uploaded by ColorfulBildungsroman
The Institute of Risk Management
calvin oyieke
Tags
Summary
This document is a chapter from a book on operational risk management. It covers the objectives, different data types, approaches, and challenges in creating and applying categorisation structures. The book is part of the IOR - Certificate in Operational Risk Management course.
Full Transcript
6/18/24, 10:19 AM Chapter 4 Back Up Book for Printing Chapter 4 Back Up Book for Printing Site: The Institute of Risk Management Printed by: calvin oyieke Co...
6/18/24, 10:19 AM Chapter 4 Back Up Book for Printing Chapter 4 Back Up Book for Printing Site: The Institute of Risk Management Printed by: calvin oyieke Course: IOR - Certificate in Operational Risk Management Date: Tuesday, 18 June 2024, 8:19 AM Book: Chapter 4 Back Up Book for Printing https://www.irmvle.org/mod/book/tool/print/index.php?id=4162 1/17 6/18/24, 10:19 AM Chapter 4 Back Up Book for Printing Description The back up book allows you to print this units course content. This can be done by clicking on More and simply clicking ‘Print Book’. https://www.irmvle.org/mod/book/tool/print/index.php?id=4162 2/17 6/18/24, 10:19 AM Chapter 4 Back Up Book for Printing Table of contents Chapter 4: Operational Risk Tools - Categorisation https://www.irmvle.org/mod/book/tool/print/index.php?id=4162 3/17 6/18/24, 10:19 AM Chapter 4 Back Up Book for Printing Chapter 4: Operational Risk Tools - Categorisation 4. Understand the nature and use of data categorisation in the management of operational risk. 4.1 Define the objectives of data categorisation and use of data categorisation in the management of operational risk. 4.2 Describe the different data types that need categorisation. 4.3 Distinguish between different approaches to creating and applying categorisation structures. 4.4 Explain the various challenges in creating and applying categorisation structures. Key themes The key themes are as follows: The basic concepts and terminology used for risk-related data categorisation. Criteria for developing a good data categorisation scheme and their benefits. The boundaries and attributes of data categorisation scheme. Getting the granularity right: the balance between granularity, simplicity and usability. Typical categories of operational risk data. The bow-tie model and how operational risk data categorisation schemes are used in practice. Challenges with scope/definition, the need for management buy-in, maintenance and data quality Introduction to Chapter 4 Creating a single, consistent data categorisation approach and structure for use across the firm should be one of the first activities to be undertaken by any operational risk management function. It is important in that it provides a common language for the rest of the operational risk framework, including policy development, programmes for risk identification and quantification, scenario assessment, risk or loss event management or the deployment of risk, control and performance metrics. The reason why categorisation is so important is that large quantities of unstructured data cannot deliver the information necessary for an operational risk manager to do their job. The data needs to be organised in a scientific way, with a clearly defined hierarchy, if it is to be useful. Data that is classified in a consistent way is easier to aggregate, analyse and report than data which is unstructured and uncategorised. It is important to understand that operational risk covers a wide range of risks and, compared with other risk disciplines, there is less consensus about what it does and doesn’t cover. This feeds through to the data used for operational risk, in stark contrast to the more financial risk disciplines such as credit risk where there are widely used common data and measures such as Loss Given Default and Expected Loss. A well-defined and implemented categorisation scheme provides good control over data and helps to make sense of it. https://www.irmvle.org/mod/book/tool/print/index.php?id=4162 4/17 6/18/24, 10:19 AM Chapter 4 Back Up Book for Printing 4.1 Define the objectives of data categorisation and use of data categorisation in the management of operational risk 4.1.1 Taxonomy In this Workbook, the term ‘taxonomy’ is used to describe the data attributes for risk events (e.g. losses, near misses and gains), as set out in section 7.2. A different term, either “data categorisation”, or “data categorisation scheme”, will be used to describe the many forms of data relevant to operational risk managers which need to be categorised. Risk events are part of that data universe. But in this Workbook they have their own chapter and hence this chapter will simply cross-reference to Chapter 7, Operational Tools - Events and Losses. Please be aware, though, that some firms may use the term ‘taxonomy’ for the entire data categorisation structure used for operational risk, or risk more widely. This Workbook deliberately does not use this approach, to try to keep the definitions clear. The use is consistent with the Basel II and Solvency II regulations, to describe the data structure for risk events only. 4.1.2 Industry practice The regulatory definitions around data are sometimes considered as a standard for operational risk data categorisation. But they are not the only ones; nor are they exhaustive. Inevitably, given such a broad ranging discipline as operational risk, there is no single industry standard that can be used by all firms for all purposes. Consequently most firms either (i) adapt an industry categorisation scheme (e.g. the Basel II standard, or perhaps a different scheme developed by an industry body for benchmark analysis) to meet their own particular internal needs; or (ii) create their own bespoke categorisation scheme. If they choose the second option they often map their own bespoke data scheme to regulatory or other external/industry definitions. Exchanging data with other firms via industry bodies is an increasingly common practice in operational risk management. So this kind of mapping of firms’ own internal data categories to regulatory or industry categories is an important feature of the operational risk data framework. Workplace reflection Does your firm make use of a single data categorisation scheme, or more than one? Check how functions such as IT & Information Security, Compliance and Internal Audit classify the data they use and see whether the different categorisation schemes are used in consistent way. 4.1.3 Benefits of a data categorisation scheme A firm needs a common language to describe the various data attributes which occur repeatedly in its business. The aim is to ensure a common understanding amongst all providers and consumers of that data as to what the data is and what it represents. This also reduces the variability in interpreting the data. The higher the percentage of data attributes that can be described using pre-defined characteristics, the less scope there will be for misinterpretation of that data. It also enables consolidation of data into matching groups, sometimes known as ‘data buckets’. The following highlight some of the other benefits of a successful data categorisation scheme. Scaling/aggregation: Where data has to be scaled or aggregated, the underlying source data has to be homogeneous; i.e. classified in a consistent way so that the scaling or aggregation calculation gives accurate and appropriate results. Completeness: A good data categorisation scheme gives assurance for risk managers and other control functions that the datasets they are looking at are complete. Let's say, for example, that a firm has a data categorisation scheme with 30 separate groups or categories in it. If the risk identification process for a business entity only generates exposures in, say, 12 of the 30 groups or categories, that provides a good basis for the control function to go back to the risk identification process or information source, and ask for data on the remaining 18 categories. Internal reporting: Categorisation facilitates the grouping of common data sets in a meaningful manner, to enable comprehensive, accurate and informative reporting. This goes beyond simply consolidating or aggregating data sets. There is also a need to facilitate comparisons between different types of data using common standards. This is most relevant in reporting data on risk events, as described in Chapter 7. But it also applies to other forms of operational risk reporting, for example on Risk & Control Self Assessment, as described in Chapter 5, and Scenarios, as described in Chapter 8. Regulatory reporting: In many jurisdictions, regulators require firms to submit various operational risk reports, typically in accordance with a pre-defined template and using some particular categorisation scheme. To meet these obligations a firm must be able to arrange its data in accordance with whatever structure is demanded by the regulator, something that is virtually impossible if the internal data is unstructured. Benchmarking: While well-structured reports for each business unit are important to management, equally important is the ability to benchmark different business units against each other, which provides a completely different perspective for risk management. To achieve such benchmarking, the data sets of the respective entities have to be comparable, i.e. categorised using the same data attributes. Similarly, even the most comprehensive suite of internal reports and data can only provide information about where the firm itself stands. Internal benchmarking can be the comparative analysis of a division, business line or product line. It may also support the analysis and confirmation of initial assumptions versus the actual data collected; are there gaps, is data volume and quality what was https://www.irmvle.org/mod/book/tool/print/index.php?id=4162 5/17 6/18/24, 10:19 AM Chapter 4 Back Up Book for Printing 4.1 Define the objectives of data categorisation and use of data categorisation in the management of operational risk expected? For an insight into where the firm stands in comparison with its peers, it is necessary to benchmark the firm’s data against its peers’ data. External benchmarking requires an acceptable common categorisation scheme, to which each participating firm can map its own data categorisation scheme. Without this a firm cannot participate in external benchmarking. Again the Basel II standard, or perhaps a different scheme developed by an industry body, is relevant for benchmark analysis. 4.1.4 Features of a good data categorisation scheme The following highlight some of the features associated with a good data categorisation scheme. A good balance between these factors is not easy to achieve but it is what firms should be striving for. Homogeneity: Each individual group in the data categorisation scheme must contain homogeneous data, i.e. data of a very similar nature. Often data structures are layered, or hierarchical, with parent-child relationships. The lower you go down the structure, the more similar the contents have to be. As an example, a ‘parent’ data category could be Vehicles, with ‘child’ categories being Haulage Vehicles, Passenger Vehicles and Sport Vehicles. At level 1 (parent level), cars and trucks are in the same bucket, but at level 2 (child level), they fall into different buckets. If we went one level further down we might define Cars as one of our level 3 categories below Passenger Vehicles, along with Buses and perhaps others. Cars is still a sufficiently homogeneous category for this to work. Granularity: On the other hand the categorisation scheme must also strive for enough granularity for whatever your purposes are, i.e. must have enough levels to accommodate unambiguous categories or groups at the lowest level. If we wanted a level 4 ‘child’ grouping below Cars we could go for a number of different approaches: colour, model, or engine cc (in defined ranges). It depends on the purpose for holding the data, and on how simple it is to use. The lowest level of grouping needs to be sufficiently homogeneous that it is possible and appropriate to group things together in that category. Simplicity: The data categorisation scheme must retain enough simplicity to be intuitive and workable. Too much granularity – e.g. model in the above example of a possible level 3 category below cars – might make it burdensome to use. There needs to be a balance: granular enough to make it meaningful, simple enough to make it useable https://www.irmvle.org/mod/book/tool/print/index.php?id=4162 6/17 6/18/24, 10:19 AM Chapter 4 Back Up Book for Printing 4.2 Describe the different data types that need categorisation 4.2.1 Boundaries, attributes and levels This section provides guidance on the kinds of data that may need to be categorised. This will require consideration of recurring features, or elements of the business environment that the firm might need to identify – now or at some point in the future - so it can correctly categorise them for one or more of the purposes set out in section 4.1. This is challenging, because until firms start to use the data categorisation scheme for real business purposes they can't be sure that the elements are correct, either in terms of the population of elements they may use, the way elements are described, or the level of granularity. The categorisation scheme therefore needs to be iterative, and capable of evolving. If some business data cannot be easily categorised in the categorisation scheme, the firm might need to change the data structure to capture it, if it is important enough. But it still needs to be consistent and comparable over time so the firm can compare performance of the data over time periods. There is a tension at work here. A firm needs a scheme which is consistent over time; yet it needs flexibility to evolve to address new business data that are not yet known. The firm needs an appropriate balance of simplicity against sufficient granularity. Creators of categorisation schemes have to be smart and sensible when defining the attributes of data categorisation scheme, designing them so that they can take account of things which haven’t yet happened. One important thing to know is what the data categorisation scheme will be used for and what it won’t be used for. In other words what are the boundaries of the scheme? This will facilitate decisions on what must be included and what might be left out. Once the boundaries are defined, the firm can assess the business environment and decide what elements it wants to capture. At the same time, the firm can assess the level of granularity needed for each element. It may not always be possible to know what level of granularity will be needed but informed judgements can be made based on what the data will be used for. Or a decision may not be needed if information is only available at a particular level of the data hierarchy. Let’s assume that a firm is categorising methods of transport, for the purpose of measuring accident rates. The firm decides it is interested in a wide universe of possible methods, so for level 1 it chooses Air, Water and Land. It then decides to have two levels of granularity, and after some thought arrives at the following categorisation scheme: Air: Light aircraft. Passenger aircraft. Cargo aircraft. Space re-entry vehicle. Hot air balloon. Water: Canoe. Yacht. Motor cruiser. Barge. Liner. Ferry. Submersible. Land: Bicycle. Passenger vehicle. Cargo vehicle. Farm vehicle. Motorcycle. Train In this structure, the category ‘passenger vehicle’ could include buses, mini-buses, sports cars, family cars and off-road vehicles. But it would exclude F1 racing cars, which do not fit into any category. This suggests – given the purpose established up front (which is to look at accident rates) – the firm needs to amend the categorisation scheme so it includes ‘Motor sport vehicles’ or something similar as an additional level 2 category. It is also clear from this scheme that the firm needs at least one additional (child) level below ‘passenger vehicle’, to allow it to differentiate what are actually quite different forms of transport. The question then arises as to how many further levels to use. https://www.irmvle.org/mod/book/tool/print/index.php?id=4162 7/17 6/18/24, 10:19 AM Chapter 4 Back Up Book for Printing 4.2 Describe the different data types that need categorisation The approach used is to go into sufficient detail that going one level further down would be describing specifics such as branding differences, colour differences or pricing differences. Consider a credit card – and compare a Visa platinum card to a MasterCard gold card. They are essentially the same type of product. They differ by issuing bank, managing card clearer, colour, interest rates, and other attributes. However, the processing activities and the operational risks are more or less identical. For operational risk purposes a firm would probably not need to distinguish between any attribute more detailed than ‘credit card’. However, assume for instance that a firm needed to distinguish by issuing bank or clearer – it would define a lower (child) level in the categorisation scheme. This would be below ‘credit card’ and allow it to distinguish by additional level of granularity, given the firm's particular needs https://www.irmvle.org/mod/book/tool/print/index.php?id=4162 8/17 6/18/24, 10:19 AM Chapter 4 Back Up Book for Printing 4.2.2 Elements commonly found in an operational risk categorisation schem The following section considers some of the common elements found in an operational risk data categorisation scheme. Process types The concept of process types relates to the activities which a firm undertakes and performs. In analysing these activities, the firm should consider common activities which occur on a repetitive basis across the enterprise, typically at a high level. Examples would be customer/client on-boarding, transaction capture and update, payments and settlements, and transaction accounting. Process types are one of the fundamental elements in a data categorisation scheme, as virtually everything that occurs within the firm relates to some specific process. Risks may arise in processes and affect other processes; risk events may manifest themselves in specific processes; while controls are applied to manage risk in specific processes. For example a multi-level process structure might include the following: Level 1:Business origination. Level 2: Customer and client relationship management. Level 3: New customer on-boarding. Level 4: Know Your Customer, Politically Exposed Persons, Blacklist checking. Level 4: Signature verification. Level 3: Customer file establishment and maintenance. Level 3: Ongoing customer relationship management. Level 3: Customer profitability and requirements review. This element of the categorisation scheme would need to be both comprehensive and granular so it describes each and every process undertaken by the bank, at a high level of granularity. This could run to many hundreds of processes. It is up to the firm to assess an appropriate balance between simplicity and granularity. Risk types or categories Risk types or categories are subject to considerable differences in interpretation from firm to firm, and are often heavily influenced by the primary industry in which the firm is based. Risk types for financial services firms tend to be high level. At level 1 this may cover risk types covered in Chapter 1 such as strategic risk, credit risk, market risk and operational risk. Typically, firms would define a three to four level hierarchy to capture the various risk types including operational risk. An example hierarchy may include the following: Level 1: Operational Risk. Level 2: External Theft and Fraud. Level 3: External Fraud. Level 4: Credit card fraud. Level 4: ATM fraud. Level 4: Identity fraud. The risk category structure will also be affected by the specific industry in which the firm is located, with different sub-categories, for instance, for banking, asset management or insurance. This element is the one which probably generates more disagreement amongst industry participants than any other, but is also the one which, if incorrectly defined, will cause more misclassification and ‘dirty data’ than any other element. In terms of an industry standard, certainly in banking, the Basel II operational risk event types (covered in Chapter 1) are probably the most widely used. But they only consist of two levels, which is relatively high-level. Industry associations, particularly the various loss data consortia, have in some cases created more granular structures to be used by their members for reporting purposes. As set out in more detail in Chapter 1, the Basel II taxonomy at level 1 reflects seven loss event types: Internal fraud. External fraud. Employment practices and workplace safety. Clients, products and business practices. Damage to physical assets. https://www.irmvle.org/mod/book/tool/print/index.php?id=4162 9/17 6/18/24, 10:19 AM Chapter 4 Back Up Book for Printing 4.2.2 Elements commonly found in an operational risk categorisation schem Business disruption and system failure. Execution, delivery and process failure. But an alternative level 1 structure to consider for loss events could be: Errors and omissions. Business disruption. Conduct and business practices. Financial crime Control types The first form of control-related categorisation originated from the audit profession and focused on the purpose of the control, that is, what the control was intended to do. Examples of this would include preventative, detective or corrective controls, which are covered in 6.6, The Nature and Role of Controls. As well as their purpose, controls can be categorised in different ways, if that helps risk management. One example is to categorise by the nature of the control: Physical controls e.g. fences, doors, locks and fire extinguishers. Procedural controls e.g. incident response processes, management oversight, security awareness and training. Information security controls e.g. user authentication (login) and logical access controls, antivirus software, firewalls. Legal and regulatory or compliance controls e.g. privacy laws, policies and clauses. In relation to information security, a further level of categorisation can be derived from international information security standards, such as ISO 27001:2013, where controls are divided into 14 groups, such as: Human resources security. Physical security of the organisation's sites and equipment. Secure communications and data transfer. Security for suppliers and third parties. Incident management. Industries An industry type hierarchy is particularly useful as an additional attribute in analysis of commercial clients and for credit risk purposes. From an operational risk perspective, it is a useful attribute to assist in the analysis of risks, exposures and losses to identify correlations with specific industries. However, rather than defining your own hierarchy, you could consider adopting a structure published by a national, regional or international authority, such as the World Bank or the United Nations. These structures may also be very granular, so consider cutting off your own use of these categorisation schemes at an appropriate higher level of granularity. Many will also provide a structured breakdown of the financial services industry which may be a useful alternative to the ‘business line’ structure discussed below. Business lines The concept of business lines as part of firms’ data categorisation schemes arises from Basel II where, for the Standardised Approach to calculating operational risk regulatory capital, firms are required to divide their business activity into eight revenue-generating business lines. This business line structure is also used in what is often referred to as the ‘7 by 8’ matrix, a mapping of the eight business lines against the seven Basel II level 1 loss event types noted earlier. The Basel II business lines are described in more detail at 7.2.6. Many firms have, however, amended the initial eight business lines suggested by Basel II. One common addition is for the corporate centre or for centralised, non-revenue-generating functions of the firm. Another common difference is for firms to divide its retail banking business line between generic retail or branch banking and private banking or wealth management. Products/services The product or service type hierarchy is essentially a lower level of categorisation below a business line or industry type. Most firms are only active in a single industry type (or sub-type), or only active in a sub-set of the business lines. This has led some firms to develop a categorisation element for the products and services they offer customers, or in which they transact in the financial markets. Many of these https://www.irmvle.org/mod/book/tool/print/index.php?id=4162 10/17 6/18/24, 10:19 AM Chapter 4 Back Up Book for Printing 4.2.2 Elements commonly found in an operational risk categorisation schem product or service elements tie back to a specific business line or generic area of activity. For example, a multi-level product type structure for commercial banking could be: Commercial Banking Commercial Branch Operations. Commercial Credit: Commercial Loans. Commercial Leasing. Commercial Real Estate Financing. Trade Finance. Project Finance. Factoring. Letters of Credit and Bank Guarantees. Commercial Cards. Commercial Deposit and Investment Accounts. Commercial Banking Services With a product or service type element hierarchy, remember the objectives of homogeneity, simplicity and granularity and avoid including actual product names into the structure. Customers/clients In certain jurisdictions, for example those subject to the second EU Markets in Financial Instruments Directive (MiFID II) and the accompanying Regulation on Markets in Financial Instruments and Amending Regulation (MiFIR), it is mandatory to segment customer and client bases and to classify each according to the nature of business transacted with that type of customer or client. However, using a customer or client categorisation structure is also useful for other forms of risk data analysis and is used by many firms to manage which product types are made available to which customer or client types. A common customer and client type hierarchy is also useful for comparison between different business units, where different nomenclature is common, for example, the use of ‘customer’ in retail banking compared to ‘client’ in asset management or retail brokerage. Distribution channels Distribution channels refer to the channels the firm uses to both secure business opportunities from existing and prospective customers and clients and to deliver its products and services through. It is common for different processes, different control structures and even different product types to be offered or delivered through different channels, or to restrict certain channels to specific customer or client types. Examples of distribution channels to include in a data categorisation scheme could be branch, website, call centre, mobile app, broker or other intermediary, business partners and sales staff. Geographies The geographical structure of business, from continents and countries down through provinces, states and counties, to cities and specific locations/buildings, all form part of data which are commonly used within the firm and thus form part of its data categorisation scheme. As with industry types, there are a variety of existing structures available for these geographical attributes, which it is often sensible to adopt. However, actual location data is usually firm-specific and often requires a unique naming convention. Causes This is covered in more detail in section 7.3, but the Basel II definition of operational risk is primarily causal. That is, it refers to the risk of loss arising from inadequate or failed processes, people, systems and/or external systems. Although few operational risk frameworks have historically focused on causal analysis, most firms have included a causal element in their data categorisation schemes, usually consisting of four level 1 instances: people, processes, systems and external factors. https://www.irmvle.org/mod/book/tool/print/index.php?id=4162 11/17 6/18/24, 10:19 AM Chapter 4 Back Up Book for Printing 4.3. Distinguish between different approaches to creating and applying categorisation structures 4.3.1 An approach to creating categorisation structures: bow-tie model The essence of the bow-tie model has been covered in Chapter 1. The essence of the model is that it characterises the relationship between cause, event and impact as illustrated below: Figure 4.3.1 (a): Bow-tie model The bow-tie model holds that events can only occur if one or more causal factor arise and impacts can only occur from events. What the bow-tie model does, firstly, is force differentiation in the risk event taxonomy, to ensure that it accurately distinguishes between causes, events and impacts; then secondly lead to appropriate categorisation of the different components or attributes of risks and risk events. A practical implementation of a bow-tie model in a financial services firm is illustrated in the diagram below: Figure 4.3.1(b): Different aspects of a bow-tie based data categorisation scheme https://www.irmvle.org/mod/book/tool/print/index.php?id=4162 12/17 6/18/24, 10:19 AM Chapter 4 Back Up Book for Printing 4.3. Distinguish between different approaches to creating and applying categorisation structures The connection between this bow-tie implementation diagram and the data categorisation scheme is that, however it is organised, the data scheme needs to support analysis of each and every component of the bow-tie model. You can see, for instance, that the yellow boxes in the above diagram represent data categories covered in section 4.2.2 above. Every item on this diagram should be capable of being analysed or supported by the data categorisation scheme we implement. If the cause-event-impact analysis breaks down, for example due to insufficient granularity or usability of the data framework, then the data scheme needs to be enhanced to ensure the logic of the bow-tie model can be followed. Workplace reflection Does your firm recognise the ‘bow-tie’ model within its operational risk management framework? If not, what impact would introducing the model have on your framework and the various supporting tools and techniques? https://www.irmvle.org/mod/book/tool/print/index.php?id=4162 13/17 6/18/24, 10:19 AM Chapter 4 Back Up Book for Printing 4.3.2 Applying categorisation structures The following section deals with how categorisation structures are used with other tools and processes in the operational risk management framework. Risk and control self-assessment (RCSA) See Chapter 5 for more detail on RCSA. As indicated in the previous section, a firm’s data categorisation scheme will typically include a group denoting risk types or risk categories. Many firms document their risk in so-called ‘risk registers’, describing the risk, then indicating which risk type or risk category the risk belongs to. The firm may adopt a slightly more granular approach and could also include any customer or client types which the risk is specific to, and/or any distribution channel types through which it may materialise. Turning to controls, these are often documented in a ‘control register’. As with a risk, it is also common to describe the control and to indicate which control type it belongs to. A control may also be mapped to other categorisations such as process types. The organisation hierarchy will provide additional level of details on where controls are executed. Moving on to assessment, in addition to the elements of data categorisation already used for the risk and its associated controls, it is common to define the process in which the risk may materialise and where the controls are situated, describing the process along with its process type. Some firms may also undertake some form of ‘root cause analysis’, describing the cause and applying the appropriate causal type to it. The final step of most assessments will include some form of quantification, either through ratings or actual monetary values. Risk and control indicators See Chapter 6 for more detail on indicators. Risk indicators are linked to key risk, implying the use of risk type or risk category. Some more advanced firms will also include causes into their risk indicators, thus including causal types into the mix of data categories they use. As with RCSAs the risk indicator framework could either source – or be sourced from – the data categorisation scheme; either way there is scope for integration between categorisation scheme and the wider operational risk framework. Risk events See Chapter 7 for more detail on risk events. Risk events (e.g. losses, near misses, gains and offsets) represent the record of a risk which has already manifested itself and, as a result, the data requirements for risk event recording encompasses all the data categorisation elements described in this chapter. Scenario analysis See Chapter 8 for more detail on scenario analysis. Scenario analysis considers the potential implications of a risk manifesting itself, on a forward looking basis. It describes in what situations a risk might arise (including risk type and risk category, process type, products or services, customers or clients and distribution channels); what might make the risk manifest itself (causes); and what might prevent the risk from manifesting itself (controls). There is therefore a considerable connection between the data categorisation scheme and the key features of the scenario analysis tool, which can be used to ensure consistent data usage and understanding. Capital estimation While simpler forms of capital estimation may only have a limited need for data categorisation support, more advanced modelling or estimation approaches require data to be arranged into clear, granular buckets. As these approaches may include the output of RCSA, scenarios, various indicators and internal and external loss event data, the categories used by each of these tools become a part of the capital estimation process. Other applications Other programmes, tools and functions across the firm may also use different elements of the data categorisation scheme, including business continuity management, information security, compliance and internal audit as well as back office process management functions. The firm’s data categorisation scheme can also be used in management information reporting, facilitating the presentation of consistently categorised information from across the firm expressed in a commonly understood and accepted language. Learning activity Select one or two (or all, if you wish) of the applications for a data categorisation scheme described in this sub-section, then analyse the comparable programme or tool within your own firm and compare the elements suggested here against what is used in practice. https://www.irmvle.org/mod/book/tool/print/index.php?id=4162 14/17 6/18/24, 10:19 AM Chapter 4 Back Up Book for Printing 4.3.2 Applying categorisation structures https://www.irmvle.org/mod/book/tool/print/index.php?id=4162 15/17 6/18/24, 10:19 AM Chapter 4 Back Up Book for Printing 4.4. Explain the various challenges in creating and applying categorisation structures There are many challenges in creating a data categorisation scheme and using it in practice to actually classify data. On the face of it a simple data scheme with few levels should be easy to create and to understand. Conversely a more expansive, more granular data categorisation might be more difficult to create and understand but easier to use. The real answer often lies in the degree of documentation and the extent to which the lowest levels of each element of the data hierarchy are truly unambiguous. 4.4.1 Scope/definition The first challenge is defining the scope or boundary of the categorisation scheme. Will it be restricted to operational risk, or to operational risk and compliance, or will it be made available more broadly? The broader the domain, the more difficult to align all stakeholders and the more comprehensive the content has to be. Conversely, the narrower the domain, the more likely it will be regarded as specific to that area and discounted for wider use. Boundary issues, as discussed in section 1.3, are a challenge, particularly if the categorisation scheme has low granularity, and if different standalone categorisation schemes are used for different risk types. Contradictory definitions, and the potential for different risk teams to use the ‘wrong’ categorisation scheme for their risk type, are challenges here. A further challenge is writing clear, concise yet complete definitions for different elements in the categorisation scheme: what they mean and how they differ from each other. Creating a meaningful, descriptive and unique name for each element is one thing; but without clear guidelines and definitions for each one, the probability of accidental misuse and incorrect categorisation increases. The lens or viewpoint used to categorise risk events can be another key challenge. As set out in the description of the bow-tie model above, many events result from so-called ‘causal chains’ that is a cause-event-impact chain occurs, where the impact becomes the cause in a different cause-event-impact chain. These chains can have numerous iterations. A software coding error (event) results in a defective system (impact) for the IT department; the defective system (cause) miscalculates interest (event) resulting in compensation claims (impact) for an operations unit; the miscalculated interest (cause) creates unhappy customers (event) who lodge complaints with the ombudsman (impact); etc. Depending on which unit is using the categories, the resultant risk or event may end up being documented in very different ways. 4.4.2 Granularity A challenge we refer to frequently within this chapter is the level of granularity. The more granular, the more unambiguous the data scheme can be. But, in general, the more granular, the more difficult it is to get the business to understand, adopt and use it. The solution has to be to pursue additional layers of granularity for those elements that really need it (e.g. risk category, control and products or services) and to keep the other elements at higher levels of granularity (e.g. risk type, industries, geographies). 4.4.3 Buy-in Another key challenge relates to getting management buy-in and adoption of the categorisation scheme. If too generic, or too granular, it may face challenges being adopted by the business. As indicated earlier in the chapter, it needs to achieve the balance between simplicity and granularity. Achieving buy-in from the business, as with many aspects of operational risk management, is highly dependent on senior management support and promotion. To achieve this buy-in, the categorisation scheme needs to use language the business is familiar with and can relate to. Labels such as ‘execution, delivery and process management’ or ‘clients, products and business practices’ may not mean much to people in the business; whereas ‘errors and omissions’ or ‘conduct and ethics’ would be more intuitive and understandable. At the beginning of a categorisation exercise, different areas within the firm may well use different terminology for similar things. The aim has to be consistency across the firm wherever possible. Some firms have adopted technology solutions whereby divisional or business unit- specific labels can be added to individual elements in the data categorisation scheme, and displayed or used in reporting for that division or business unit. 4.4.4 Maintenance and data quality Keeping the data categorisation scheme updated to reflect changes in the business environment is a challenge – as is applying any changes and re-categorising previously categorised data, where needed. Maintenance of data categorisation schemes lags business reality. The next challenge relates to staff capabilities, both in the use of the data categories and in applying them to different types of data. This challenge can be offset by training, but a robust, extensive and granular data categorisation scheme will require business knowledge and categorisation experience if it is to be applied properly. Consistent application of the categories is a further challenge. Individuals make mistakes and are biased. Biases are discussed in section 8.7. https://www.irmvle.org/mod/book/tool/print/index.php?id=4162 16/17 6/18/24, 10:19 AM Chapter 4 Back Up Book for Printing 4.4. Explain the various challenges in creating and applying categorisation structures Another challenge relates to effort and time. The amount of effort to categorise some data can be significantly reduced by a well-structured, comprehensive data categorisation structure. However if that same structure is relatively granular and staff are inadequately trained, the degree of effort to categorise the data will increase. Further, if the staff undertaking the data categorisation have little time, or are faced with unrealistic categorisation and reporting deadlines, short-cuts will be taken and an inappropriately categorised data set will result. Data categorisation is a critical component of risk management and reporting, highly dependent on the quality of the input data and staff should be allowed sufficient time to categorise data appropriately. A final challenge lies in where the data categorisation process takes place. The closer to the point of origin of a specific risk, the greater the awareness and knowledge of the specifics of the situation; conversely, the further away from the point of origin, the less will be known about the business and related risk information. However, staff in second line of defence risk functions tend to have greater knowledge of data categorisation schemes and can thus categorise data sets more quickly and easily. One solution which some firms have adopted is for the business to submit a comprehensive narrative describing the risk related data and for risk support staff to undertake the categorisation, then asking the business to confirm the categorisation. Workplace reflection Which of these challenges have you encountered within your firm? Discuss these challenges with your colleagues and see whether they have faced similar or different challenges. Are there other challenges which you or your colleagues have faced which are not included here? Summary This chapter has focused on the role of data categorisation within the operational risk environment, covering concepts and terminology, the objectives for data categorisation, different elements of a data categorisation taxonomy, some approaches and applications for data categorisation, finishing with an overview of some of the challenges, both in building and in using, a data categorisation scheme. As part of your understanding of this chapter, it is worth reviewing the IOR’s Sound Practice Guidance paper on Risk Categorisation. Key learning You will be ready to move to the next chapter when you can confidently answer the following questions: 1. What are some of the key benefits of data categorisation? 2. What industry data categorisation options are available to firms? 3. What are some of the key features of a good data categorisation scheme? 4. What types of operational risk data are typically structured using a data categorisation scheme? 5. How can the bow-tie model facilitate creation of a data categorisation scheme? 6. What are some of the common ways in which a data categorisation scheme is applied to operational risk activities (e.g. RCSA)? 7. What are some of the key challenges which need to be considered when developing a data categorisation scheme? https://www.irmvle.org/mod/book/tool/print/index.php?id=4162 17/17