Analysis of Observational Studies: Causal Inference PDF
Document Details
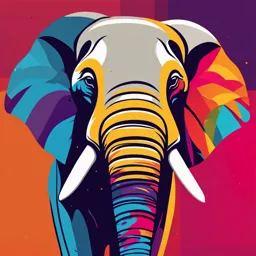
Uploaded by DazzledGiant4648
Stellenbosch University
Rhoderick Machekano
Tags
Summary
This document analyzes observational studies using structural nested mean models and G-estimation. It covers topics such as marginal structural models (MSM), and provides an outline, objectives, and a summary of the key concepts. The presentation includes diagrams of Directed Acyclic Graphs (DAGs).
Full Transcript
Analysis of Observational Studies: Causal Inference Structural Nested Mean Models and G-estimation Rhoderick Machekano, PhD MPH Stellenbosch University [email protected] Outline 1 Introduction Session Objectives 2 Margi...
Analysis of Observational Studies: Causal Inference Structural Nested Mean Models and G-estimation Rhoderick Machekano, PhD MPH Stellenbosch University [email protected] Outline 1 Introduction Session Objectives 2 Marginal Structural Models 3 Structural Nested Mean Models and G-estimation Exchangeability Objectives 1 Recap marginal structural models 2 Introduce structural nested mean models 3 Demostrate application to data Readings: 1 Chpt 14. MH & JR Single time point DAG Recap: MSM MSM provide a way to model causal effects by specifying models for the marginal densities f (Y a ) or expectations of potential outcomes Y a E(Y a |V ) = q(a, V ) q(a, V ) = βa a + βV V Under certain assumptions estimation can be done by IPTW or the g-formula 1 Model association between treatment A and covariates W 2 Derive weights 3 Use weights to create artificial population in which W not associated with A 4 Estimate MSM parameters βa , βV using weighted methods with robust SEs Recap: MSM MSM parameters can also be estimated using Standardization or parametric G-formula 1 Within each stratum L = l, estimate E(Y a |L = l = E[Y |A = a, L = l] 2 Estimate standardized means for each a by a weighted average of of the stratum conditional means using P(L = l) as weights 3 Causal effect is difference of standardized means Paramateric G-formula n1 P 4 Ê(Y |A = a, L = l) Motivating SNMM Estimating causal effects in a subset of the population Strata is formed by a combination of values of L E[Y a,c=0 |L] − E[Y a=0,c=0 |L] c=counterfactual (e.g subpopulation) Exchangeability Conditional exchangeability ⇒ Y a q A|L Pr[A = 1|Y a=0 , L] = Pr[A = 1|L] Consider the model: logit(Pr(A = 1|Y 0 , L)) = α0 + α1 Y a=0 + α2 L We cannot fit this model because Y 0 is incomplete Suppose we could fit the model, what is the expected value of α1 Structural nested mean model Estimating average causal effect of A within levels of L E(Y a=1 |L) − E(Y a=0 |L) = E(Y a=1 − Y a=0 |L) No effect modification means the effect are the same in at all levels of L, E[Y 1 − Y 0 |L] = β1 L=strata Structural model would be: E[Y a − Y 0 |L] = β1 a With effect modification by L: E[Y a − Y 0 |L] = β1 a + β2 aL Under conditional exchangeability: E[Y 1 − Y 0 |A = a, L] = β1 a + β2 aL subset of marginal structural model if B2 is zero, then it is equal to B1 Contrast with MSM: E[Y 1 − Y 0 |A = a, L] = β0 + β1 a + β2 aL + β3 L G-estimation E[Y 1 − Y 0 |A = a, L] = β1 a + β2 aL SNMM ignore β0 , β3 L - semi-parametric β1 , β2 are estimated by g-estimation SNMM make few assumptions and can be more robust to model misspecification In the presence of censoring, one cannot adjust for selection bias and confounding together Adjust for selection bias first using IPWC Apply g-estimation to the pseudo-population Time varying confounders Structural nested mean models for TVC Deal with confounders affected by treatment Interest is in estimating the joint effect of a sequence of treatments (A0 , A1 ) or actions in the presence of L SNMM are better at dealing with: i Violation of no unmeasured confounders ii Positivity iii Sequential ignorability (exchangeability) Estimating SNMM 1 Uses G-estimation - an estimating equations approach 2 Best implemented in statistical package Check sunlearn*