Causal Inference 3 PDF
Document Details
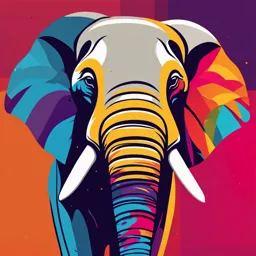
Uploaded by DazzledGiant4648
Stellenbosch University
Rhoderick Machekano
Tags
Summary
This document provides an analysis of observational studies, focusing on causal inference and estimating causal effects. It details the challenges of observational studies, and methods for overcoming these challenges.
Full Transcript
Analysis of Observational Studies: Causal Inference Estimating Causal Effects in Observational Studies Rhoderick Machekano, PhD MPH Stellenbosch University [email protected] Outline 1 Introduction Session Objectives...
Analysis of Observational Studies: Causal Inference Estimating Causal Effects in Observational Studies Rhoderick Machekano, PhD MPH Stellenbosch University [email protected] Outline 1 Introduction Session Objectives Estimating causal effects from Observational Data 2 Designing Observational Studies 3 Conditions for causal inference 4 Causal inference in Observational studies 5 Standardization Objectives 1 Review observational studies against randomized studies 2 Draw awareness of challenges encountered with observational studies 3 Highlight potential solutions 4 Establish conditions under which observational studies can be used to estimate causal effects Readings: 1 Chpt 3. MH JR 2 Holland, 1986 3 Chpt 1. PRR - DOS Observational Study Definition An empiric investigation in which the objective is to elucidate cause-and-effect relationships in which it is not feasible to use controlled experimentation in the sense of being able to impose the procedures or treatments whose effects it is desired to discover, or to assign subjects at random to different proceduresa a Cochran (1965) Example Cohort - prospective and retrospective studies Cross sectional studies Case-Control studies Why we need observational studies to evaluate interventions Randomized controlled studies are considered the ”gold standard” for causal effects estimation but are at times: 1 Unnecessary 2 Inappropriate - HIV as a causal agent for AIDS; smoking as a cause of lung cancer 3 Impossible - infrequent outcomes and rare events 4 Inadequate There are examples of observational studies that have provided evidence that have changed medical practice Complimentary roles What are the main challenges? 1 Lack of control lack of balance - confounding bias lack of comparability - selection bias 2 Unmeasured confounders 3 Time-varying confounders Definition Bias is a systematic error that results in an incorrect estimate. Arises when features of a study’s design lead to estimates that do not accurately reflect the relationship between the study variables Overt bias Hidden bias What are the main challenges? 1 Lack of control lack of balance - confounding bias lack of comparability - selection bias 2 Unmeasured confounders 3 Time-varying confounders Definition Bias is a systematic error that results in an incorrect estimate. Arises when features of a study’s design lead to estimates that do not accurately reflect the relationship between the study variables Overt bias Hidden bias What are the main challenges? 1 Lack of control lack of balance - confounding bias lack of comparability - selection bias 2 Unmeasured confounders 3 Time-varying confounders Definition Bias is a systematic error that results in an incorrect estimate. Arises when features of a study’s design lead to estimates that do not accurately reflect the relationship between the study variables Overt bias Hidden bias What are the main challenges? 1 Lack of control lack of balance - confounding bias lack of comparability - selection bias 2 Unmeasured confounders 3 Time-varying confounders Definition Bias is a systematic error that results in an incorrect estimate. Arises when features of a study’s design lead to estimates that do not accurately reflect the relationship between the study variables Overt bias Hidden bias Randomized Studies Making treatment or intervention groups as similar as possible within subgroups Treatment assignment independent of baseline characteristics and outcomes #Eliminate selection bias Flip a balanced coin #Ensure equal probability of being assigned to the treatment group The probability of receiving treatment, π, is known and is equal to 1 n #To ensure that any differences in the outcome may be attributed to the treatment/intervention. #The decision to assign individuals to the treatment groups it is not influenced by their existing characteristics or expected outcomes. Estimating Causal Effects from observed data In RCT treatment is independent of the potential outcome In randomized controlled studies, average causal effect = difference in outcomes between the two groups The mean outcome among treated equals mean treated counterfactual because treatment is independent of potential outcome In observational studies treatment is not independent of potential outcomes It is because the treatment is assigned based on various factors, this can lead to difference in baseline characteristics between the groups In observational studies, individuals receiving treatment A may not be comparable to those receiving treatment Bdue to differences in the baseline characteristics sicker, older, poorer, less adherent differences in outcomes may be a reflection of these differences Estimating causal effects from observational data Treatment may not be independent of counterfactuals - same characteristics that led to treatment exposure may also be associated with potential response Difference of observed average response may be a biased estimate of the causal effect Because the treatment groups may differ in a way that may affect the outcome of the treatment Solution: 1 Identify all confounders W 2 Potential responses (Y0 , Y1 ) are independent of treatment exposure among subject with the same W values Within each stratum defined by (W), the treatment 3 Estimate differences within strata assignment random Estimate the treatment effect within each stratum (treated vs untreated); This is to control for confounders Designing observational studies How would the study be conducted if it were possible to do it by controlled experimentation?” Define study population Eligibility and exclusion criteria - restriction Define Exposure - what are the treatments/intervention groups Pre-exposure covariates Define outcome Challenge Achieving balance between intervention groups Designing observational studies How would the study be conducted if it were possible to do it by controlled experimentation?” Define study population Eligibility and exclusion criteria - restriction Define Exposure - what are the treatments/intervention groups Pre-exposure covariates Define outcome Challenge Achieving balance between intervention groups Designing observational studies How would the study be conducted if it were possible to do it by controlled experimentation?” Define study population Eligibility and exclusion criteria - restriction Define Exposure - what are the treatments/intervention groups Pre-exposure covariates Define outcome Challenge Achieving balance between intervention groups Example observational study The potential outcome (mortality) should be independent of the treatment (transplant) within groups that have similar confounders. In an observational study of heart transplant and mortality, those who receive a transplant have a severe heart condition Those who receive the transplant would be expected to have a greater risk of mortality had they not received the transplant compared to patients who did receive a transplant This is a violation of Pr[Y a=1 = 1|A = 1] = Pr[Y a=1 = 1|A = 0] (exchangeability) An associational effect therefore does not estimate a causal effect Because the observed association between heart transplant and mortality, does not accurately reflect the causal effect; the groups are not comparable. To estimate the causal effect; we should account for the differences in the baseline characteristics (severity of the heart condition) between the groups. Addressing selection and confounding challenges Use novel methods to mimic randomization Stratification Matching Propensity scoring Matching or stratifying1 Inverse Probability Weighting (IPW) Instrumental Variables Sensitivity Analysis 1 Rosenbaum and Rubin, JASA 1984 Causal effects estimation in observational studies Conditional Randomization Analyze the data as if treatment was randomized, conditional on measured covariates. Conditions necessary: STUVA assumption 1 Values of treatment under comparison correspond to well define interventions corresponding to versions of treatment in the data 2 Conditional probability of receiving every value of the treatment depends only on the measured covariates within strata, the treatment assignment is as good as random. (Exchangeability) 3 conditional probability of receiving every value of treatment is greater than zero Positivity assumption Ensure sufficient overlap between the treatment groups across all levels of the covariates; for comparison With these conditions, an observational study can emulate a conditionally randomized experiment Condition 1: exchangeability Treatment is randomly assigned, making it independent of the potential outcome In marginally randomized experiments, Y a q A In conditionally randomized experiments, Y a q A|L In observational studies Reasons for receiving treatment are likely associated with some outcome predictors Distribution of outcome predictors vary between treated and untreated groups (just as in conditionally randomized experiments) But is there only one L Conditional exchangeability will not hold if there exits unmeasured independent predictors U of outcome such that probability of receiving treatment A depends on U with strata L Exchangeability is not verifiable in observational studies CRE: Treatment is randomly assigned within strata defined by ( L ). Within each stratum of ( L ), the treatment is independent of the potential outcomes Violation by unmeasured confounders; This is because the probability of receiving treatment ( A ) depends on ( U ) within strata ( L ) Non-verifiability; because we cannot observe the counterfactual outcomes (what would have happened if a different treatment had been received). We rely on the assumption that all relevant confounders have been measured and adjusted for. Condition 2: positivity There is probability greater than zero of being assigned to each of the treatment levels Pr[A = a] > 0 or Pr[A = a|L = l] > 0, ∀l : Pr[L = l] 6= 0 In observational studies, positivity is not guaranteed, however, it can be sometimes empirically verified Violation of positivity: If treatment levels are not observed all values of the covariates, this violates the condition of positivity, making it difficult to estimate the causal effect. Condition 3: Consistency A defined standardized treatment exists with no variation (no multiple versions of the same treatment) In observational data, we have no control over the versions of treatments - use restriction Inclusion and exclusion criteria (define study population) Example: If the participants had received a treatment, the outcome should be same as if they had not received treatment. Meaning that the treatment is standardized Causal effect of smoking cessation on weight gain seqn qsmk age sex race university wt71 smokeintensity smokeyrs exercise active 1 233 0 42 0 1 0 79.04 30 29 2 0 2 235 0 36 0 0 0 58.63 20 24 0 0 3 244 0 56 1 1 0 56.81 20 26 2 0 4 245 0 68 0 1 0 59.42 3 53 2 1 5 252 0 40 0 0 0 87.09 20 19 1 1 6 257 0 43 1 1 0 99.00 10 21 1 1 A = smoking cessation (qsmk: 1: quit smoking, 0: still smoking) L = (age, sex, race, university, wt71, smokeintensity, smokeyrs, exercise, active) Y = weight gain (wt8287) Associational Effect Mean weight gain among quitters, E[Y |A = 1] = 4.5 Mean weight gain among non-quitters, E[Y |A = 0] = 2.0 Associational Effect E[Y |A = 1] − E[Y |A = 0] = 2.5 Use R to estimate the associational effect and the associated 95% confidence interval Average causal effect E[Y a=1 ] − E[Y a=0 ] If quitters and non-quitters are different wrt characteristics that affect weight gain, then the associational effect will not equal the average causal effect Check the distribution of covariates W between levels of A Check covariates association with Y Age independently associated with both quitting and weight gain (regardless of quitting status) ⇒ confounder of effect of A on Y Formulations from conditional exchangeability Recall Y a q A|L There are two formulations for Pr[Y a = 1] Pr[Y a = 1] = w Pr[Y a = 1|W = w]Pr[W = w] P Pr[A=a] Pr[Y a = 1] = w Pr[Y a = 1|W = w] Pr[A|W P =w Estimating standardized mean outcome: Parametric G-formula Pr[Y a = 1] = w Pr[Y a = 1|W = w]Pr[W = w] = w Pr[Y |A = P P a, W = w]Pr[W = w] Consistency assumption W can be multi-dimensional including continuous variables No need to estimate Pr[W = w] Only estimate n1 ni=1 Ê[Y |A = a, Wi ] P P Why: i E[Y |A = a, W = w]Pr[W = w] = E[E[Y |A = a, W ]] Example code for G-formula E[Y a ] = E[Y |A = a, L = l] #General formula/computation > wt.lm.st = lm(wt82_71˜qsmk*smokeintensity+sex+race+university > wt.lm.st.hat = predict(wt.lm.st) > > # Estimate standardized estimates > newdata=nhef > newdata$qsmk=0 #Smoking > wt.0.hat = predict(wt.lm.st,newdata) > mean(wt.0.hat) #1/n *Sum[Y^1} 1.75 #E[Y^0] > newdata$qsmk=1 # Not Smoking > wt.1.hat = predict(wt.lm.st,newdata) > mean(wt.1.hat) 5.28 #model distribution depends on the type of data; e.g ordinal distribution for ordinal data Use bootstrapping to get 95% confidence intervals Reading The parametric g-formula for time-to-event data. Keil AP. Epidemiology 2014; 25:889-897 #Must read