Business Statistics Semester-II PDF
Document Details
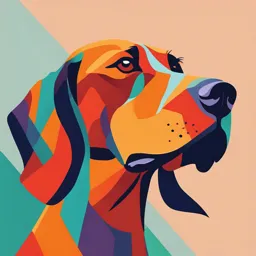
Uploaded by FinerTangent
Uttaranchal University
Tags
Summary
This document is a textbook on Business Statistics for a Bachelor of Arts program, specifically for Semester II. It covers topics like data organization, data types, and analysis methods.
Full Transcript
BACHELOR OF ARTS SEMESTER-II BUSINESS STATISTICS OBA-126 1 Prefix 2 CONTENT Unit - 1 Introduction Organization of Data...................................................................................
BACHELOR OF ARTS SEMESTER-II BUSINESS STATISTICS OBA-126 1 Prefix 2 CONTENT Unit - 1 Introduction Organization of Data................................................................................ 4 Unit - 2 Presentation of Data................................................................................................... 44 Unit - 3 Uni-Variate Data Analysis –I..................................................................................... 85 Unit - 4 Uni-Variate Data Analysis - II................................................................................. 125 3 UNIT - 1 INTRODUCTION ORGANIZATION OF DATA STRUCTURE 1.0 Objectives 1.1 Introduction 1.2 Origin, Scope, Definition of Statistics in Singular and Plural Form 1.3 Limitations of Statistics 1.4 Functions of Statistics 1.5 Mouse of Statistics with Case Studies 1.6 Basic Concepts in Statistics 1.7 Population in Statistics 1.8 Sample 1.9 Variable Attribute 1.10 Types of Data: Nominal, Ordinal Scales, Ratio and Interval Scales 1.11 Cross-Sectional and Time Series 1.12 Discrete and Continuous Classification-Tabulation of Data 1.13 Summary 1.14 Keywords 1.15 Learning Activity 1.16 Unit End Questions 1.17 References 1.0 LEARNING OBJECTIVES After studying this unit, you will be able to: Describe the origin and scope of statistics Identify the limitations of statistics State cross- sectional and time series List the functions of statistics 4 1.2 INTRODUCTION Statistics, which is a branch of mathematics that focuses on the gathering, tabulation, and interpretation of numerical data, simply means numerical data. In reality, it is a type of mathematical analysis that use various quantitative models to generate a set of experimental data or investigations of real-world phenomena. Data collection, analysis, interpretation, and presentation are all issues that are addressed in this area of applied mathematics. Statistics focuses on the use of statistics to the resolution of challenging issues. Some individuals believe statistics to be a separate mathematical science as opposed to a subfield of mathematics. Statistics simplify your work and give you a clear, concise image of the tasks you accomplish on a regular basis. The gathering, examination, and analysis of data are under the purview of statistics, a subfield of mathematics. It is renowned for using quantified models to infer conclusions from data. The process of gathering, analyzing, and summarizing data into a mathematical form is known as statistical analysis. The study of data collection, analysis, interpretation, presentation, and organization is known as statistics. It is a mathematical instrument that is used to gather and compile data, to put it simply. Only statistical analysis can be used to determine the degree of uncertainty and fluctuation in various sectors and factors. The probability, which is a key concept in statistics, determines these uncertainties. What are Statistics? Statistics is the study and manipulation of pre-existing data, to put it simply. It deals with the computation and analysis of provided numerical data. review some further definitions of statistics provided by various authors here: Statistics are "The specific data or facts and conditions of a people within a state - especially the values that can be expressed in numbers or in any other tabular or classified way," according to the Merriam-Webster dictionary. Statistics are & numerical statements of facts or values in any department of inquiry placed in specific relation to each other. according to Sir Arthur Lyon Bowley. In business statistics, the organization of data is a crucial step in the data analysis process. It involves arranging, summarizing, and presenting data in a meaningful and understandable manner to gain insights and make informed decisions. Properly organized data enables businesses to identify trends, patterns, and relationships, which can guide strategic planning, problem-solving, and decision- making processes. Let's explore the key aspects of organizing data in business statistics: 5 Data Collection and Preparation: Data organization begins with collecting relevant data from various sources. This could involve surveys, sales records, financial statements, customer feedback, and more. Once collected, the data needs to be cleaned and preprocessed to remove errors, inconsistencies, or outliers that could affect the analysis. Data Types: Business data can be categorized into different types: Quantitative Data: Numeric data that can be measured. This includes variables like sales revenue, number of products sold, or customer age. Qualitative Data: Categorical data that represents qualities or characteristics. Examples include product categories, customer segments, or survey responses. Data Organization Methods: There are several methods to organize data, depending on the type of data and the objectives of analysis: Tabulation: Creating frequency tables for qualitative data to show the distribution of categories. Grouping: Grouping quantitative data into intervals or classes for creating frequency distributions, histograms, or bar charts. Sorting: Arranging data in a particular order (e.g., ascending or descending) based on relevant variables. Aggregation: Combining data into larger categories for easier analysis, such as grouping sales by quarters or regions. Data Summarization: Summarizing data involves condensing large datasets into more manageable and informative forms: Measures of Central Tendency: Calculating mean, median, and mode to understand the central values of quantitative data. Measures of Dispersion: Computing range, variance, and standard deviation to assess the spread of data points. Percentiles: Dividing data into hundredths to understand how values are distributed across the dataset. Data Visualization: Visual representations help in presenting data patterns effectively: 6 1. Bar Charts: Used for comparing categorical data. 2. Histograms: Depict the distribution of quantitative data. 3. Scatter Plots: Illustrate relationships between two quantitative variables. 4. Line Charts: Display trends over time, suitable for time-series data. 5. Pie Charts: Show the composition of a whole based on parts. Data Presentation and Interpretation: Organized data should be presented in a clear and concise manner, with appropriate labels, titles, and annotations. Interpretation involves analyzing the organized data to draw meaningful conclusions and make informed decisions for the business. In conclusion, the organization of data in business statistics is a foundational step that facilitates effective analysis, decision-making, and strategic planning. Properly organized and presented data enhances business understanding, leading to improved operational efficiency, better customer insights, and a competitive advantage. The data collected by an investigator is in raw form and cannot offer any meaningful conclusion; hence, it needs to be organized properly. Therefore, the process of systematically arranging the collected data or raw data so that it can be easy to understand the data is known as organization of data. With the help of organized data, it becomes convenient for the investigator to perform further statistical treatments. The investigator can also compare the mass of similar data if the collected raw data is organized systematically. Classification of Data A method of organization of data for the distribution of raw data into different classes based on their classifications is known as classification of data. In other words, classification of data means converting raw data collected by an investigator into statistical series in a way that provides meaningful conclusions. According to Conner, “Classification is the process of arranging things (either actually or notionally) in groups or classes according to their resemblances and affinities, and gives expression to the unity of attributes that may exist amongst a diversity of individuals.” Based on the definition of classification of data by Conner, the two basic features of this process are: The raw data is divided into different groups. For example, on the basis of marital status, people can be classified as married, unmarried, divorced and engaged. 7 The raw data is classified based on class similarities. All similar units of the raw data are put together in one class. For example, every educated person can be put together in one class and uneducated in another. Each group or division of the raw data classified on the basis of their similarities is known as Class. For example, the population of a city can be classified or grouped based on their age, education, income, sex, marital status, etc., as it can provide the investigator with better conclusions for different purposes. Objectives of Classification of Data The major objectives of the classification of data are as follows: Brief and Simple: The main objective of the classification of data is presentation of the raw data in a systematic, brief and simple form. It will help the investigator in understanding the data easily and efficiently, as they can draw out meaningful conclusions through them. Distinctiveness: Through classification of data, one can render obvious differences from the collected raw data more distinctly. Utility: Classification of data brings out the similarities within the raw diverse data of the study that enhances its utility. Comparability: With the classification of data, one can easily compare data and can also estimate it for various purposes. Effective and Attractive: Classification makes raw data more attractive and effective. Scientific Arrangement: The process of classification of data facilitates proper arrangement of raw data in a scientific manner. In this way, one can increase the reliability of the collected data. Characteristics of a Good Classification Clarity: Classification of the raw data is beneficial for an investigator only when it provides a clear and simple form of information. Clarity here means that there should not be any kind of confusion regarding any element or part of a class. Comprehensiveness: There should be comprehensiveness in the classification of the raw data so that each of its items gets a place in some class. In other words, a classification is good if no item is left out of the classes. Homogeneity: Each and every item of a class must be similar to each other. Homogeneity in the different items of a class ensures the best results and further investigations. 8 Stability: Stability in the same set of classification of data for a specific kind of investigation is essential, as it does not confuse the investigator. Therefore, the base of classification of data should not change with every investigation. Suitability: The classes in the data classification process must suit the motive of enquiry. For example, classifying children of a city based on their weight, age, and sex for the investigation of literacy rate makes no sense. The data for literacy rate investigation must be done into classes, like educated and uneducated. Elastic: Data classification can provide better results only if it is elastic and hence, has scope for change if there is any change in the scope or objective of the investigation. 1.3 ORIGIN, SCOPE, DEFINITION OF STATISTICS IN SINGULAR AND PLURAL FORM Origin of Statistics: Statistics can be traced back to ancient civilizations where records were maintained for administrative, economic, and social purposes. For instance, ancient civilizations like the Babylonians, Egyptians, and Romans collected data on population, land, and resources for governance. However, the formal development of statistics as a systematic discipline began in the 17th century. The Belgian mathematician and astronomer Adolphe Quetelet played a significant role in shaping modern statistics. He introduced the concept of "average" and advocated for the application of statistics to social sciences. In the 19th century, Sir Francis Galton, a British scientist, made contributions to the fields of regression and correlation, paving the way for statistical methods used in various disciplines. Scope of Statistics: The scope of statistics is vast and multidisciplinary. It encompasses both descriptive and inferential aspects: Descriptive Statistics: Involves summarizing and presenting data using measures like mean, median, mode, range, and standard deviation. Descriptive statistics provide insights into central tendencies, variability, and the distribution of data. Inferential Statistics: Focuses on making predictions, drawing conclusions, and testing hypotheses about a population based on a sample. Techniques like hypothesis testing, regression analysis, and analysis of variance fall under inferential statistics. 9 Biostatistics: Applied in healthcare and medical research to analyze clinical trials, disease patterns, and medical interventions. Business Statistics: Used to analyze market trends, consumer behavior, financial data, and make informed business decisions. Economic Statistics: Aids in assessing economic indicators, inflation rates, GDP growth, and unemployment rates. Social Sciences: In fields like sociology and psychology, statistics is used to analyze social patterns, conduct surveys, and study human behavior. Natural Sciences: Used to analyze experimental data, test hypotheses, and model physical phenomena. Environmental Statistics: Applies statistical methods to environmental data, such as analyzing pollution levels and climate patterns. Education: Used to assess student performance, evaluate teaching methods, and conduct educational research. Definition of Statistics (Singular Form): In its singular form, "statistics" refers to the entire field of study that encompasses the collection, analysis, interpretation, and presentation of data. It involves methods for designing surveys, experiments, and observational studies, as well as techniques for analyzing and drawing meaningful conclusions from data. Definition of Statistics (Plural Form): In its plural form, "statistics" refers to individual numerical data points or measures derived from data sets. These measures can include averages, percentages, ratios, and more, providing concise summaries of data characteristics. Statistics has become an essential tool in decision-making across various sectors. It empowers researchers, policymakers, analysts, and professionals to make informed choices by quantifying uncertainty and extracting meaningful insights from data. Whether used to predict stock market trends, assess public health concerns, or understand social phenomena, statistics plays a pivotal role in modern society. 10 1.3 LIMITATIONS OF STATISTICS Statistics, while a powerful tool for data analysis and decision-making, has several limitations that users should be aware of when interpreting and applying statistical results. These limitations include: Sampling Bias: If a sample used for analysis is not representative of the entire population, it can lead to biased results that do not accurately reflect the larger group. This can occur due to factors such as non-random sampling or self-selection bias. Nonresponse Bias: In surveys or studies, when participants do not respond, the sample may not accurately represent the intended population, leading to potential inaccuracies in conclusions drawn from the data. Measurement Errors: Errors in data collection, such as inaccuracies in recording or interpreting information, can introduce noise and affect the quality of the results. These errors can be systematic or random. Misleading Averages: Reliance solely on measures like the mean can be misleading if the data contains outliers or is not normally distributed. Outliers can disproportionately influence the mean, leading to inaccurate representations of the central tendency. Causation vs. Correlation: Statistical analysis can identify correlations between variables, but it cannot prove causation. Just because two variables are correlated does not necessarily mean that changes in one directly cause changes in the other. Small Sample Sizes: Small sample sizes can lead to higher variability in results and decrease the reliability of statistical analysis. It may also limit the generalizability of findings to a larger population. Confounding Variables: Factors that are not accounted for in an analysis but still influence the variables being studied can lead to incorrect conclusions. These confounding variables can distort relationships between variables. Regression to the Mean: Extreme values in a dataset are likely to move closer to the mean when measured again, which can lead to misinterpretation as a treatment effect when it's simply a result of random variation. Overfitting: In predictive modeling, overfitting occurs when a model fits the noise in the data rather than the underlying pattern. This can result in poor generalization to new data. Inaccurate Assumptions: Many statistical methods rely on certain assumptions about the data, such as normality or independence. If these assumptions are not met, the results can be unreliable. 11 Voluntary Response Bias: When individuals self-select to participate in a survey or study, it can lead to a biased sample that may not accurately represent the larger population's opinions or characteristics. Data Availability: Sometimes, the required data might not be available or might be incomplete, limiting the scope and accuracy of the analysis. Ethical Considerations: Statistics can be used to manipulate or misrepresent data, potentially leading to unethical decisions or policies if not used responsibly and transparently. It's crucial to recognize these limitations and exercise caution when interpreting statistical results. Combining statistical analysis with domain knowledge, critical thinking, and a clear understanding of the data's context can help mitigate these limitations and lead to more informed decisions. here are some additional limitations of statistics: Simpson's Paradox: This paradox occurs when trends that appear in different groups of data disappear or reverse when these groups are combined. It emphasizes the importance of considering subgroup effects and not oversimplifying complex data. Data Transformation: Transforming data (e.g., logarithmic, exponential) can sometimes lead to misinterpretation or the loss of meaningful insights. The transformed data might not accurately represent the original context. Data Interpretation: Misinterpretation of statistical results due to lack of expertise or understanding can lead to incorrect conclusions and misguided decisions. Publication Bias: Studies with statistically significant or positive results are more likely to be published, creating a bias in the available literature and potentially leading to overestimation of effects. Time Dependency: Statistical relationships that hold true at one time might not necessarily hold true at a different time due to changing conditions, making predictions less reliable. Multicollinearity: In regression analysis, when predictor variables are highly correlated, it can be challenging to attribute the effects of individual predictors accurately. Selection Bias: When certain groups or data points are excluded from the analysis, it can skew the results and limit the generalizability of findings. Ecological Fallacy: Drawing conclusions about individuals based on group-level data can be misleading because individual characteristics might differ within a group. Non-Stationarity: In time series analysis, assumptions like constant mean and variance might not hold true, leading to inaccurate forecasts or conclusions. 12 Misleading Visualizations: Poorly designed charts and graphs can distort data representation and mislead interpretation. Underpowered Studies: Studies with small sample sizes or inadequate statistical power might fail to detect real effects, leading to inconclusive results. Regression Fallacy: Assuming that if two variables are correlated over a certain period, they will remain correlated over other periods without accounting for external factors. Survivorship Bias: Focusing on successful outcomes while ignoring unsuccessful cases can lead to skewed conclusions, especially in fields like finance and business. Changing Data Dynamics: Some phenomena change over time due to external factors, making historical data less applicable to present circumstances. Homogeneity Assumption: Some statistical tests assume homogeneity of variance or distribution, which might not hold true in all cases. Random Variation: Even in the absence of a real effect, random fluctuations in data can sometimes produce statistically significant results by chance. Interactions: Statistical interactions between variables can complicate interpretations and require careful consideration. Recognizing these limitations and potential pitfalls helps ensure a more accurate and responsible use of statistics in decision-making, research, and analysis. It underscores the need for a critical approach that considers both the strengths and weaknesses of statistical methods. 1.4 FUNCTIONS OF STATISTICS Statistics serves various functions across different fields and contexts, contributing to informed decision-making, research, analysis, and understanding of data. Some of the key functions of statistics include: Data Collection: Statistics plays a fundamental role in collecting data through surveys, experiments, observations, and sampling methods. It guides the process of gathering information from various sources. Data Description: Descriptive statistics summarize and present data in a concise and understandable manner. Measures like mean, median, mode, range, and standard deviation provide insights into the central tendency, variability, and distribution of data. 13 Data Analysis: Statistical techniques allow researchers and analysts to uncover patterns, trends, and relationships within data. Methods such as regression analysis, correlation analysis, and time series analysis help draw meaningful conclusions. Data Interpretation: Statistical results are interpreted to extract insights, make predictions, and draw conclusions. Interpretation involves understanding the significance of relationships, identifying outliers, and assessing the practical implications of findings. Inference and Generalization: Inferential statistics involve making predictions or drawing conclusions about a larger population based on a sample of data. It enables generalizing findings from a subset to a larger group. Hypothesis Testing: Statistical hypothesis testing assesses the validity of claims or hypotheses by comparing sample data to a known or assumed population parameter. It aids in making informed decisions about the validity of certain propositions. Prediction and Forecasting: Statistics is used for predicting future outcomes based on historical data and trends. Time series analysis and regression models are commonly used for forecasting. Quality Control: In manufacturing and industry, statistics helps monitor and control product quality by analyzing production data, identifying defects, and ensuring products meet specified standards. Risk Assessment: In finance and insurance, statistics is used to assess risks, calculate probabilities of events, and make informed decisions regarding investments, pricing, and coverage. Experimental Design: Statistics guides the design of experiments, ensuring that variables are controlled and manipulated in a way that allows researchers to draw meaningful conclusions from the results. Sampling Techniques: Statistics provides methods for selecting representative samples from larger populations, ensuring that collected data accurately reflects the characteristics of the entire group. Comparative Analysis: Statistical methods facilitate the comparison of data sets, allowing for the identification of differences and similarities between groups, conditions, or time periods. Data Visualization: Statistics helps create graphical representations such as charts, graphs, and plots that make data visually accessible and aid in conveying insights to a wider audience. Policy and Decision-Making: Government agencies and organizations use statistical data to inform policy decisions, assess the impact of interventions, and allocate resources effectively. 14 Scientific Research: Statistics is essential in various scientific disciplines to test hypotheses, validate theories, and analyze experimental results. Social and Behavioral Sciences: It assists in studying human behavior, analyzing survey responses, and understanding social phenomena. Healthcare and Medicine: Statistics supports clinical trials, epidemiological studies, and medical research to analyze treatment efficacy, disease patterns, and public health trends. Market Research: In business, statistics aids in market analysis, consumer behavior research, and making informed marketing strategies. By fulfilling these functions, statistics contributes to a better understanding of complex data, facilitates evidence-based decision-making, and enables the extraction of valuable insights from various domains. 1.5 MISUSE OF STATISTICS WITH CASE STUDIES Misuse of statistics refers to the improper, misleading, or manipulative use of statistical techniques, data, or interpretations to support a particular agenda, draw false conclusions, or deceive others. This can occur due to lack of understanding, intentional manipulation, or bias. Here are some common ways in which statistics can be misused: Cherry-Picking Data: Selectively presenting data that supports a desired conclusion while ignoring or omitting data that contradicts it. This distorts the overall picture and can lead to inaccurate interpretations. Correlation vs. Causation Fallacy: Assuming that because two variables are correlated, one must cause the other. This ignores other factors that could influence the relationship and confuses coincidence with causality. Misleading Visualizations: Presenting graphs, charts, or visuals in a way that distorts data representation, such as altering scales, using inappropriate axes, or truncating data to exaggerate differences. Misinterpreting Averages: Using averages (mean, median, mode) without considering the distribution of data, leading to misleading conclusions. For instance, quoting an average without mentioning the high variability can misrepresent the data. Equivocation: Using terms with multiple meanings interchangeably to create confusion or misleading comparisons. For example, using "increase" in terms of percentages to exaggerate a change. 15 Statistical Jargon Misuse: Using complex statistical terms inaccurately or out of context to make claims appear more authoritative or convincing than they actually are. Overgeneralization: Applying findings from a specific context to a broader one without considering potential differences that might affect the results. Data Transformation Bias: Manipulating data through transformations (e.g., logarithms) to create the appearance of a trend or relationship that might not exist in the original data. Ignoring Outliers: Disregarding data points that are outliers without proper justification, which can lead to biased conclusions. Data Mining and P-Hacking: Repeatedly testing a hypothesis with different variations until a statistically significant result is found, without adjusting for the increased likelihood of a false positive. False Precision: Presenting results with excessive decimal places or significant figures, suggesting a level of accuracy that the data doesn't support. Sample Size Misrepresentation: Drawing broad conclusions from small sample sizes without acknowledging the limitations of generalizability. Misleading Comparisons: Making misleading comparisons by using different units or scales, such as comparing absolute numbers to percentages. Suppressing Context: Presenting data without proper context or omitting relevant information that might provide a more accurate understanding. Confusing Relative and Absolute Measures: Presenting relative measures (percentages) without context of the absolute values they're derived from, leading to misinterpretations. Fabrication and Falsification: Intentionally creating or altering data to support a desired outcome, which is unethical and undermines the integrity of statistical analysis. Misleading Survey Questions: Designing survey questions in a biased or leading manner to elicit specific responses that align with a particular viewpoint. Misuse of statistics can have significant consequences, leading to misguided decisions, false beliefs, and public mistrust. It's crucial to approach statistical information critically, evaluate the methodology, consider the context, and seek expert guidance when interpreting data. let's delve deeper into each of the mentioned cases of statistics misuse: The Case of DDT and Cancer Risk: In the 1981 study, researchers reported an association between DDT exposure and cancer risk. However, the study didn't consider confounding factors such as smoking or occupational exposures. Additionally, the study used data from a skewed population that didn't accurately represent the general population's DDT exposure levels. Despite these limitations, the media 16 sensationalized the findings, causing unnecessary panic. Subsequent studies with proper methodology found no significant link between DDT and cancer, emphasizing the importance of rigorous analysis and cautious interpretation. The Correlation-Causation Fallacy in Autism and Vaccines: The Wakefield study's flawed methodology included a small sample size of only 12 children, and it lacked proper controls. Despite this, the study claimed a causal link between the MMR vaccine and autism. The media widely publicized the study, leading to reduced vaccine uptake and subsequent outbreaks of preventable diseases. This case demonstrates the need to critically assess research methodologies and avoid drawing causation from correlation without proper evidence. Simpson's Paradox in Gender Bias at Berkeley: The initial report suggested that UC Berkeley displayed gender bias in admissions. However, disaggregating the data by department revealed that some departments were actually biased in favor of women. The paradox arises from the fact that different departments had varying acceptance rates and gender distributions. It underscores the importance of examining subgroup data and avoiding hasty generalizations. Dow Jones vs. Bitcoin Prices: The manipulated graph juxtaposed the significant growth of Bitcoin with the Dow Jones Index during a short period. However, the longer-term data showed no consistent correlation between the two. This case exemplifies the power of selective cropping and data visualization to convey misleading narratives. Misleading Graphs in Climate Change Debates: Climate change skeptics have used misleading graphs to downplay temperature increases. For instance, they might show temperature trends over a short period to suggest no significant change. Such manipulation obscures the broader context of long-term climate patterns and human impact. Election Polling in the 2016 U.S. Presidential Election: Pollsters' failure to anticipate the 2016 election outcome revealed limitations in polling methods. Many polls didn't account for non-response bias, where certain demographics were less likely to respond to surveys. Additionally, some Trump supporters might have been hesitant to disclose their preference, leading to skewed predictions. This case underscores the challenges of accurately predicting outcomes in complex systems. 17 Cherry-Picking Data in Marketing: Companies might highlight positive testimonials or select favorable data points to create the illusion of high product efficacy. While this might not be outright falsehood, it presents a skewed representation of reality and can mislead consumers. Lies, Damned Lies, and Statistics - Political Manipulation: Politicians can manipulate statistics to support their narratives. By selectively presenting data or framing numbers in a particular way, they can influence public opinion. This highlights the importance of transparent reporting and critical analysis of political claims. These cases emphasize the necessity of understanding the underlying data, scrutinizing research methods, considering context, and applying critical thinking when encountering statistical claims. Proper statistical analysis and responsible reporting are crucial to ensure accurate representation of data and informed decision-making. 1.6 BASIC CONCEPTS IN STATISTICS 1. Population and Sample: Population: It refers to the entire group of individuals, items, or events that a researcher aims to study. For example, if you're interested in studying the heights of all students in a school, the population would include all students in that school. Sample: A subset of the population that is selected for data collection. Since studying an entire population might be impractical, a sample is used to draw conclusions about the entire population. 2. Variable: A variable is a characteristic or attribute that can take different values. For instance, age, gender, height, and income are examples of variables. Categorical Variable: Represents distinct categories or labels, such as "Gender" with categories like "Male" and "Female." Numerical Variable: Represents quantities that can be measured and can take numerical values. Numerical variables can be further categorized into discrete and continuous variables. 3. Data Types: Categorical Data: Also known as qualitative data, it represents categories or labels. Examples include eye color, marital status, and types of animals. 18 Numerical Data: Also called quantitative data, it represents quantities and can be measured. Numerical data can be: Discrete: Consists of separate, distinct values (e.g., the number of students in a class). Continuous: Takes any value within a certain range (e.g., height or weight). 4. Descriptive Statistics: These statistics summarize and describe data using measures like: Mean: The average of all values in a data set. Median: The middle value when data is arranged in ascending order. Mode: The value that appears most frequently in a data set. Range: The difference between the maximum and minimum values. Standard Deviation: A measure of the spread of data around the mean. Descriptive statistics provide insights into the central tendencies and variability of data. 5. Inferential Statistics: Inferential statistics involve making predictions or inferences about a population based on a sample. Common techniques include: Hypothesis Testing: Assessing the validity of a claim based on sample data. Confidence Intervals: Estimating a range within which a population parameter is likely to fall. Regression Analysis: Modeling relationships between variables to make predictions. 6. Sampling Techniques: Various methods are used to select a representative sample from a population. Some common techniques include: Random Sampling: Each individual in the population has an equal chance of being selected. Stratified Sampling: Dividing the population into subgroups and then selecting samples from each subgroup. Cluster Sampling: Dividing the population into clusters and then randomly selecting entire clusters to include in the sample. 7. Central Tendency: Measures of central tendency provide information about the center of a data set: Mean: The sum of all values divided by the number of values. Median: The middle value when data is arranged in order. Mode: The value that appears most frequently. Dispersion or Variability: Dispersion measures indicate how spread out the values in a data set are. They include: Range: The difference between the maximum and minimum values. 19 Variance: The average of the squared differences between each value and the mean. Standard Deviation: The square root of the variance, providing a measure of the average distance from the mean. 8. Frequency Distribution: A frequency distribution tabulates the number of occurrences of each value or category in a data set. This helps visualize the distribution pattern of data. 9. Probability: Probability quantifies the likelihood of an event occurring. It ranges from 0 (impossible event) to 1 (certain event) and is used to model uncertainty. 10. Normal Distribution: A normal distribution is a bell-shaped curve characterized by its symmetry and defined by its mean and standard deviation. Many natural phenomena follow this distribution. 11.Hypothesis Testing: Hypothesis testing involves: Formulating a null hypothesis (H0) and an alternative hypothesis (Ha). Collecting sample data and calculating a test statistic. Comparing the test statistic to a critical value to determine if the null hypothesis is rejected or not. 12.Confidence Interval: A confidence interval provides a range of values within which a population parameter is likely to fall. It includes a level of confidence, such as 95%. 13. Regression Analysis: Regression analysis models the relationship between one or more independent variables and a dependent variable. It helps predict outcomes and understand the influence of variables. 14. Correlation: Correlation measures the strength and direction of a linear relationship between two numerical variables. A correlation coefficient ranges from -1 (perfect negative correlation) to 1 (perfect positive correlation). 15. Outliers: Outliers are data points that significantly deviate from the rest of the data. They can skew results and should be carefully examined for their impact. 16. Bias and Variance: Bias: It's the extent to which a statistical measure consistently deviates from the true value. Variance: It represents the variability of a statistical measure across different samples. 20 17. Confounding Variable: A confounding variable is an extraneous factor that affects both the independent and dependent variables, leading to incorrect conclusions about their relationship. Understanding these basic concepts is essential for building a solid foundation in statistics. They provide the tools necessary to interpret data, conduct meaningful analyses, and draw accurate conclusions. Other terms that can also be used in statistics are: 1. Alternative hypothesis A theory that conflicts with the null hypothesis is an alternative hypothesis. A null hypothesis is an educated guess regarding the truth of your premise or the existence of any connection between the words. You can reject the alternative hypothesis if the evidence you gather show that your original hypothesis or the null hypothesis was true. 2. Analysis of covariance A tool for assessing data sets with two variables—effect, sometimes known as the variate, and treatment, which is the categorical variable—is analysis of covariance. When another variable, known as the covariate, appears, the categorical variable follows. This analysis reduces potential bias and improves a study's accuracy. 3. Analysis of variance An analysis of variance (ANOVA) compares the relationship between more than two factors to determine whether there’s a link between them. 4. Average The average refers to the mean of data. You can calculate the average by adding up the total of the data and dividing it by the number of data points. 5. Bell curve The bell curve, also called the normal distribution, displays the mean, median and mode of the data you collect. It usually follows the shape of a bell with a slope on each side. 6. Beta level The beta level, or simply beta, is the probability of committing a Type II error in a hypothesis analysis, which entails agreeing to the null hypothesis when it isn’t true. 7. Binomial test You can use a binomial test when a test has two possible outcomes—failure or success—and you know the chances of success. A binomial test is used to assess whether an observed test result differs from its predicted result. 21 8. Breakdown point When an estimator reaches the breakdown point, it becomes useless. A larger number indicates that there is less risk of resistance, while a lower number indicates that the information may not be valuable. 9. Causation Direct correlation between two variables is known as causation. If a change in one variable's value results in a change in the other, the two variables are said to be directly related. In that situation, one becomes the cause and the other the result. 10. Coefficient A multiplier is used to measure a variable by a coefficient. The coefficient is frequently a numerical value that multiplies by a variable to give you the variable's coefficient when performing research and computing equations. A variable's coefficient is always one if it lacks a value. 11. Confidence intervals A confidence interval calculates how uncertain a group of data is. If you repeat the same experiment, this is the range in which you predict your values to fall within a given level of confidence. 12. Correlation coefficient The correlation coefficient expresses how closely two variables are correlated or dependent on one another. If the value is outside of this range, the coefficient was measured incorrectly. This value is a number between -1 and +1. 13. Cronbach's alpha coefficient Internal consistency is quantified by Cronbach's alpha coefficient. It demonstrates the type of connection between several variables in a set of data. Additionally, if you add more items, Cronbach's alpha coefficient rises, and vice versa. 14. Dependent variable A value that depends on another variable to change is referred to as a dependent variable. You can utilise dependent variables to calculate statistical analyses and draw inferences about the causes of events, changes, and other statistical translations. 15. Descriptive statistics Descriptive statistics depict the features of data in a study. This may include a representation of the total population or a sample population. 22 16. Effect size A statistical concept known as "effect size" measures the strength of a relationship between two specific variables. We can discover, for instance, how therapy affects patients with anxiety. The effect size is used to assess how successful or ineffective a therapy is. 17. F-test Any test that applies the F-distribution to a null hypothesis is known as an F-test. The F-test can be used by researchers to assess the parity between two population variances. They utilise it to determine whether or not the two independent samples drawn from a typical population exhibit comparable variability. 18. Factor analysis A large number of variables must be reduced to a manageable number of factors in order to do a factor analysis. With this approach, the biggest common variance among all variables is extracted and reduced to a single number. This quantity serves as an index of all components in the additional analysis. 19. Frequency distribution Frequency distribution is the frequency with which a variable occurs. It provides you with data on how often something repeats. 20. Friedman's two-way analysis of variance Using different parameters for each group, researchers compare groups to see if there are statistically significant differences between them. This is done using Friedman's two-way analysis of variance test. 21. Hypothesis tests A technique for testing outcomes is a hypothesis test. The researcher formulates a hypothesis or theory regarding what they hope the findings will demonstrate before beginning the research. Then, a study verifies that hypothesis. 22. Independent t-test The independent t-test analyses the averages of two independent samples to see if there’s statistical proof that a related population average or mean differs substantially. 23. Independent variable An independent variable in a statistical experiment is one that you change, regulate, or manipulate in order to study its effects. Since no other research variable has an impact on it, it is referred to as independent. 23 24. Inferential statistics A test called inferential statistics is used to compare a particular collection of data within a population in various ways. Parametric and nonparametric tests are included in inferential statistics. An inferential statistical test involves taking data from a small group and drawing conclusions about whether the results will be the same in a bigger population. 25. Marginal likelihood The likelihood that a parameter variable may marginalise is known as the marginal likelihood. In this context, the term "marginalise" refers to comparing the probabilities of brand-new hypotheses to those of current hypotheses. 26. Measures of variability The degree to which a database is spread or scattered is indicated by measures of variability, often known as measures of dispersion. The range, standard deviation, variance, and interquartile range are the four primary measurements of variability. 27. Median The centre of the data is referred to as the median. A data collection with an odd number of elements typically has the median located exactly in the middle of the numbers. You can determine the simple mean between the two middle values when calculating the median of a set of data with an even number of elements. 28. Median test A nonparametric test called a median test compares the medians of two independent groups. The null hypothesis states that the median remains constant for both groups. 29. Mode Mode refers to the value in a database that repeats the greatest number of times. If none of the values repeat, there’s no mode in that database. 30. Multiple correlations Multiple correlations are an estimate of how well you can predict a variable using a linear function of other variables. It uses predictable variables to derive a conclusion. 31. Multivariate analysis of covariance A method for analysing statistical differences between numerous dependent variables is called a multivariate analysis of covariance. Depending on the sample size, you can utilise more variables. The analysis accounts for a third variable, the covariate. 24 32. Normal distribution Normal distribution is a method of displaying random variables in a bell-shaped graph, indicating that data close to the average or mean occur more frequently than data distant from the average or mean value. 33. Parameter A parameter is a quantitative measurement that you use to measure a population. It’s the unknown value of a population on which you conduct research to learn more. 34. Pearson correlation coefficient A statistical test that ascertains the relationship between two continuous variables is the Pearson's correlation coefficient. Since it is based on covariance, experts agree that it is the best method for quantifying the relationship between relevant variables. 35. Population Population refers to the group you’re studying. This might include a certain demographic or a sample of the group, which is a subset of the population. 36. Post hoc test Researchers perform a post hoc test only after they’ve discovered a statistically relevant finding and need to identify where the differences actually originated. 37. Probability density The probability density is a statistical measurement that measures the likely outcome of a calculation over a given range. 38. Quartile and quintile Quartile refers to data divided into four equal parts, while quintile refers to data divided into five equal parts. 39. Random variable A random variable is a variable in which the value is unknown. It can be discrete or continuous with any value given in a range. 40. Range The range is the difference between the lowest and highest values in a collection of data. 41. Regression analysis Regression analysis is a useful technique for identifying the variables that influence an interest variable. Regression analysis allows you to precisely identify the aspects that are most crucial, which ones you may ignore, and how these factors interact with one another. Regression analysis comes in many different forms, but they all focus on how independent factors affect a dependent variable. 25 42. Standard deviation The standard deviation is a metric that calculates the square root of a variance. It informs you how far a single or group result deviates from the average. 43. Standard error of the mean The likelihood of a sample's mean deviating from the population mean is evaluated using a standard error of mean. Divide the standard deviation by the square root of the sample size to obtain the standard error of the mean. 44. Statistical inference Statistical inference occurs when you use sample data to generate an inference or conclusion. Statistical inference can include regression, confidence intervals or hypothesis tests. 45. Statistical power Statistical power is how likely a study is to find statistical significance in a sample, assuming the effect is present across the board. The null hypothesis is likely to be rejected by a strong statistical test. 46. Student t-test When the standard deviation of a small sample with a bell-shaped distribution is unknown, a student t-test is used to test the hypothesis. Correlated means, correlation, independent proportions, and independent means can all be used in this. 47. T-distribution T-distribution refers to the standardised deviations of the mean of the sample to the mean of the population when the population standard deviation is unknown and the data come from a bell-curve population. 48. T-score A t-score in a t-distribution refers to the number of standard deviations a sample is away from the average. 49. Z-score A z-score, also known as a standard score, is a measurement of the distance between the mean and data point of a variable. You can measure it in standard deviation units. 50. Z-test A z-test is a test that determines if two populations' means are different. To use a z-test, you need to know the differences in variances and have a large sample size. 26 1.7 POPULATION IN STATISTICS In statistics, the concept of a population refers to the entire group or collection of individuals, items, or events that researchers are interested in studying or making inferences about. It encompasses all the entities that share common characteristics relevant to the research question or study objective. The population serves as the target of investigation, and the goal is often to gather insights, draw conclusions, or make predictions about this entire group based on available data. Here are the key aspects of the concept of population in statistics: Population Parameters: Within a population, there are specific characteristics or attributes that researchers aim to measure or analyze. These characteristics are known as population parameters. For example, if you're studying the population of adult females in a city, parameters of interest might include average age, income distribution, and educational attainment. Defining the Population: Defining the population precisely is crucial to ensure the research is focused and results are applicable. The definition should clearly outline the scope and criteria for inclusion in the population. For instance, if you're studying the population of all college students in a country, you need to specify what qualifies as a "college student." Finite and Infinite Populations: A population can be finite or infinite. A finite population has a specific, countable number of members, such as the students in a particular school. An infinite population, on the other hand, represents a group with an unlimited number of potential members, such as all possible coin toss outcomes. Access to the Entire Population: While studying the entire population is ideal, it's often impractical due to factors like time, cost, and logistics. In such cases, researchers work with samples—representative subsets of the population—to make inferences about the population as a whole. Parameters vs. Statistics: Parameters are the characteristics of the entire population, such as the population mean or population standard deviation. Statistics, on the other hand, are the corresponding values calculated from sample data, like the sample mean or sample standard deviation. Types of Populations: Finite Population: A population with a specific and countable number of members. 27 Infinite Population: A population with an unlimited number of potential members. Accessible Population: The portion of the population that researchers have access to and can study. Target Population: The specific subset of the population that researchers are interested in studying. Examples of Populations: Populations can vary widely based on the research context. Examples include: The entire world population. All registered voters in a country. All products manufactured by a company. All medical records of a specific hospital. Sampling from the Population: Due to practical limitations, researchers often work with samples to draw conclusions about populations. Proper sampling techniques ensure that the sample is representative of the population and provides valid insights. Understanding the concept of population is fundamental for designing valid research studies, conducting accurate analyses, and drawing meaningful conclusions. Whether you're working with finite or infinite populations, defining the scope of the population and its parameters is essential for sound statistical analysis and effective decision-making. 1.8 SAMPLE In statistics, a sample is a subset of individuals, items, or events selected from a larger group known as the population. Samples are used to gather data and make inferences about the characteristics of the entire population. Properly collected and representative samples can provide valuable insights without the need to study the entire population, which can be time- consuming, costly, or impractical. Here are the key aspects of the concept of a sample in statistics: Representativeness: A sample is considered representative when its characteristics mirror those of the population it is drawn from. The goal is to ensure that the sample accurately reflects the diversity and distribution of attributes present in the entire population. 28 Sampling Methods: Different techniques are used to select samples from populations: Random Sampling: Each member of the population has an equal chance of being selected. Stratified Sampling: Dividing the population into subgroups (strata) and then selecting samples from each stratum. Cluster Sampling: Dividing the population into clusters and then selecting entire clusters as samples. Convenience Sampling: Choosing individuals or items based on their accessibility. Systematic Sampling: Selecting every nth individual from a population. Sampling Error: Sampling error refers to the difference between the characteristics of a sample and the characteristics of the entire population. It's a natural consequence of working with a subset rather than the entire population. Sample Size: The size of a sample refers to the number of individuals or items included in the sample. The choice of sample size depends on factors such as the desired level of precision and the variability of the population. Sampling Frame: A sampling frame is a list or representation of all individuals or items in the population from which the sample will be drawn. A well-constructed sampling frame ensures that all members of the population have a chance of being included in the sample. Sampling Bias: Sampling bias occurs when the sample is not representative of the population due to factors such as improper sampling methods or non-random selection. This can lead to inaccurate and biased results. Simple Random Sample: A simple random sample is a subset of the population where every possible sample of a given size has an equal chance of being selected. It ensures that every individual or item has the same probability of inclusion. Sampling Variability: Sampling variability refers to the fact that different random samples from the same population will yield slightly different results due to the inherent randomness of sampling. 29 Sampling Distribution: A sampling distribution is the distribution of a sample statistic (e.g., sample mean) across multiple random samples from the same population. It provides insights into the variability of sample statistics. Uses of Sampling: Samples are used for various purposes, such as estimating population parameters, hypothesis testing, and making predictions. They are common in surveys, experiments, and research studies. Sampling Techniques and Goals: Different sampling techniques are chosen based on the research goals and available resources. The choice of technique influences the representativeness and validity of the sample. Margin of Error: The margin of error is a measure of the uncertainty associated with using a sample statistic to estimate a population parameter. It's influenced by the sample size and sampling variability. Using samples effectively and ensuring their representativeness is essential for making valid inferences about populations. Proper sampling techniques, careful consideration of sample size, and understanding potential biases contribute to the accuracy and reliability of statistical analyses and conclusions. 1.9 VARIABLE ATTRIBUTE Categorical Variables: Categorical variables represent attributes or characteristics that fall into distinct categories or groups. These categories are often qualitative in nature and don't have a numerical value associated with them. Categorical variables can be further divided into two main subtypes: nominal and ordinal. Nominal Variables: Nominal variables are categorical variables that don't have any inherent order or ranking among their categories. Examples: Eye color, country of origin, type of fruit. When working with nominal variables, you can calculate frequencies and proportions for each category, which can help you understand the distribution of data. You can also create bar charts or pie charts to visualize the distribution. 30 Ordinal Variables: Ordinal variables are categorical variables where the categories have a specific order or ranking. Examples: Educational level (e.g., "High School," "Bachelor's," "Master's"), socioeconomic status ("Low," "Middle," "High"). While you can calculate frequencies and proportions for ordinal variables, you can also create bar charts or histograms that maintain the order of categories. However, the intervals between categories may not be equal or meaningful. Numerical Variables: Numerical variables represent quantities that can be measured and expressed using numbers. Numerical variables can be either discrete or continuous. Discrete Variables: Discrete variables are numerical variables that can only take specific, distinct values, often as a result of counting or enumerating. Examples: Number of siblings, number of pets, number of items sold. With discrete variables, you can calculate frequencies, proportions, and measures of central tendency (e.g., mean, median). You might also create bar charts or histograms to visualize the distribution of values. Continuous Variables: Continuous variables are numerical variables that can take on any value within a certain range. Examples: Height, weight, temperature, age. With continuous variables, you have more options for analysis. You can calculate descriptive statistics like mean, median, range, and standard deviation. Histograms and density plots are useful for visualizing the distribution. You can also use techniques like scatter plots, correlation, and regression analysis to explore relationships between continuous variables. Understanding the distinction between categorical and numerical variables is essential because it determines the appropriate methods for analyzing and interpreting data. Different types of variables require different statistical techniques and visualization methods. When conducting analyses, researchers need to choose the appropriate tools based on the nature of the variables they are working with to ensure accurate and meaningful results. 31 1.10 TYPES OF DATA: NOMINAL ORDINAL SCALES, RATIO AND INTERVAL SCALES What are Scales of Measurement in Statistics? The process of data analysis after data collection for a study depends on the methods used to gather the data. For instance, if we wish to get qualitative data, we can ask respondents to choose an option from a set of labels (a nominal scale). Interval and ratio scales can be used to represent quantitative data numerically, allowing the researcher to visualise the data. Let's use data collection as an example to determine the types of vehicles individuals prefer to drive. Use a scale with names like electric automobiles, diesel cars, hybrid cars, etc. to capture this kind of data. For this reason, a nominal scale of measurement will be employed. A ratio scale of measurement can also be utilised if the researcher wants to determine the average weight of residents in a municipality. In the sections below, we will learn about the characteristics of each of the four measuring scales. The four scales of measurement in statistics are listed below: Nominal Ordinal Interval Ratio Fig :1.1 Measurement in Statistics 32 Nominal Scale: The nominal scale represents categorical data where items are placed into distinct categories without any inherent order or ranking. Examples: Gender (Male, Female, Non-binary), Color (Red, Blue, Green), Country of Origin (USA, Canada, France). Nominal data can be summarized using frequencies and proportions. Bar charts and pie charts are common visualization methods. Ordinal Scale: The ordinal scale also represents categorical data, but in this case, categories have a specific order or ranking. Examples: Education Level (High School, Bachelor's, Master's), Likert Scale (Strongly Disagree, Disagree, Neutral, Agree, Strongly Agree), Socioeconomic Status (Low, Middle, High). Ordinal data allows comparisons of relative positions or rankings, but the intervals between categories may not be uniform. It can be summarized using frequencies and proportions, and bar charts or histograms can be used for visualization. Interval Scale: The interval scale represents numerical data with equal intervals between values, but it lacks a true zero point. Examples: Temperature in Celsius, IQ scores. On an interval scale, you can perform arithmetic operations like addition and subtraction, but ratios are not meaningful due to the lack of a true zero. Data can be summarized using measures like mean and standard deviation. Histograms and line charts are often used for visualization. Ratio Scale: The ratio scale represents numerical data with equal intervals and a true zero point, making meaningful ratios possible. Examples: Height, Weight, Age. Ratio data allow for meaningful comparisons using ratios, such as "twice as heavy" or "three times as tall." In addition to arithmetic operations, you can calculate meaningful ratios, proportions, and percentages. Summary statistics like mean and standard deviation are applicable. Histograms, line charts, and scatter plots are common visualizations. When choosing the appropriate statistical analysis and visualization methods, understanding the type of data scale is crucial. Each type of scale has implications for the level of measurement, the types of calculations that can be performed, and the interpretation of results. 33 It's important to apply methods that are appropriate for the specific type of data you're working with to ensure accurate and meaningful analysis. Difference between Discrete and Continuous Data Discrete Data Continuous Data The type of data that has clear spaces This information falls into a continuous series. between values is discrete data. Countable. Measurable There are distinct or different values in Every value within a range is included in discrete data. continuous data. Depicted using bar graphs Depicted using histograms Ungrouped frequency distribution of Grouped distribution of continuous data discrete data is performed against a tabulation frequencies is performed against a single value. value group. Look at the table below showing the properties of all four scales of measurement. Properties Nominal Ordinal Interval Ratio Labeled variables ✔ ✔ ✔ ✔ Meaningful order of variables ✖ ✔ ✔ ✔ Measurable difference ✖ ✖ ✔ ✔ The absolute value of zero ✖ ✖ ✖ ✔ By substituting extensive class functionality for primitives, these refactoring strategies assist with data handling. Untangling of class relationships, which increases the portability and reusability of classes, is another significant outcome. Data organisation is the process of classifying and categorising data to improve its usability. You must arrange your data logically and neatly, just like we arrange important documents in a file folder, so that you and anybody else who accesses it may quickly find what they're looking for. Organizing data in statistics is a crucial step in the data analysis process. It involves arranging raw data into a structured and manageable form to facilitate better understanding, analysis, and interpretation. There are several methods for organizing data, depending on the type of data and the specific goals of the analysis. Below are some common techniques used to organize data in statistics: 34 Frequency Distribution: Frequency distribution is a tabular representation that shows the number of times each data value (or data range) occurs in a dataset. It organizes data into classes or categories (bins or intervals) and records the frequency of observations falling within each class. Frequency distributions are commonly used for categorical or discrete data. Example: Consider a dataset of exam scores: Score Range Frequency 70-79 5 80-89 8 90-99 12 100-109 6 Histograms: Histograms are graphical representations of frequency distributions. They display data using bars, where the height of each bar corresponds to the frequency of observations within a particular data range. Histograms are useful for visualizing the distribution of continuous or interval data. Cumulative Frequency Distribution: Cumulative frequency distribution shows the total frequency of observations up to a given value or class interval. It provides insights into the cumulative behavior of the data, making it easier to identify percentiles and quartiles. Example: Consider a dataset of exam scores: Score Range Frequency Cumulative Frequency 70-79 5 5 80-89 8 13 90-99 12 25 100-109 6 31 Frequency Polygon: A frequency polygon is a line graph that represents the frequency distribution of continuous or interval data. It connects the midpoints of each class interval with straight lines, depicting the shape of the data distribution. 35 Stem-and-Leaf Plot: The stem-and-leaf plot is a graphical representation that displays individual data points while maintaining their original numerical values. It organizes data by separating the leading digits (the stem) and trailing digits (the leaf) for each data point. Example: Consider a dataset of exam scores: 89, 78, 92, 84, 95, 88, 76 Stem Leaf 7 68 8 489 9 25 Cross Tabulation (Contingency Table): Cross tabulation is used to summarize categorical data by creating a table that displays the relationship between two or more variables. It shows the frequency or count of observations for each combination of the variables. 1.11 CROSS-SECTIONAL AND TIME SERIES Cross-sectional and time series data are two different types of data collection methods used in various fields, including statistics, economics, social sciences, and more. Let's explore each type in detail: Cross-Sectional Data: Cross-sectional data is collected by observing multiple subjects or entities at a single point in time or within a very short time frame. It provides a snapshot of data from a specific moment, allowing comparisons among different subjects. Examples: Survey responses collected from different individuals at the same time, financial data from multiple companies in a single year. Analysis: Cross-sectional data is used to explore relationships between variables, perform descriptive statistics, and investigate differences among groups. Time Series Data: Time series data involves observing and recording data points for a single subject or entity over multiple time periods. It captures trends, patterns, and changes that occur over time. 36 Examples: Stock prices over several months, temperature measurements taken daily for a year. Analysis: Time series data is used to analyze trends, seasonality, and patterns using techniques such as moving averages, exponential smoothing, and autoregressive integrated moving average (ARIMA) modeling. Differences between Cross-Sectional and Time Series Data: Nature of Data: Cross-sectional data involves observations from multiple subjects or entities at a specific point in time, providing information about their characteristics or attributes. Time series data tracks the behavior of a single subject or entity over consecutive time intervals, revealing patterns and changes over time. Purpose: Cross-sectional data is useful for making comparisons among different subjects at a specific point in time to identify variations or relationships. Time series data helps analyze trends, seasonality, and long-term patterns in data over time, enabling predictions and forecasting. Analysis Techniques: Cross-sectional data analysis includes techniques like comparing means, frequencies, and correlations among variables. Time series data analysis involves methods like time series decomposition, moving averages, and autoregressive models to understand trends and forecast future values. Visualization: Cross-sectional data can be visualized using bar charts, pie charts, and scatter plots to compare attributes across different subjects. Time series data is often represented using line charts, where the x-axis represents time and the y-axis represents the variable of interest. Data Collection: Cross-sectional data is collected by observing multiple subjects simultaneously or within a short time frame. Time series data is collected over consecutive time intervals, capturing observations at regular intervals (e.g., daily, weekly, monthly). Both cross-sectional and time series data play significant roles in various research and analysis contexts. The choice between them depends on the research question, the type of data needed, and the analytical goals. Researchers often combine both types of data to gain a comprehensive understanding of a phenomenon or make informed decisions. 37 1.12 DISCRETE AND CONTINUOUS CLASSIFICATION- TABULATION OF DATA Discrete and continuous classification refer to two distinct types of data in statistics. Discrete data consists of distinct, separate values, often as a result of counting or enumerating, while continuous data can take any value within a certain range. Let's explore each type and how data tabulation is done for them: Discrete Data: Discrete data consists of individual, separate values that are often whole numbers and cannot be subdivided further without losing their meaning. Examples include counts of items, number of occurrences, and distinct categories. When tabulating discrete data: Frequency Distribution: Create a table that lists the distinct values (categories) of the variable. Alongside each category, list the frequency or count of occurrences for that value. The sum of the frequencies equals the total number of data points. Interval Frequency ---------------------- 2-4 5 5-7 9 8-10 12 11-13 10 ---------------------- Total 36 Relative Frequency Distribution: Similar to frequency distribution, but express frequencies as proportions or percentages of the total. Divide each frequency by the total number of data points and multiply by 100 to get the percentage. Cumulative Frequency Distribution: In addition to listing frequencies, include a column for cumulative frequencies. Cumulative frequency represents the total count up to a certain value or category. 38 Continuous Data: Continuous data can take on any value within a given range and can be measured with varying levels of precision. Examples include measurements like height, weight, temperature, and time. Tabulating continuous data involves creating intervals or ranges, often referred to as "bins," to group the data. This is necessary since continuous data can have infinite possible values. Grouping Data into Intervals (Binning): Determine the range of values in your data. Decide on the width or size of the intervals (bins). Create intervals that cover the entire range of values, and group data points into the appropriate interval. Frequency Distribution for Intervals: Similar to discrete data, create a table that lists the intervals along with the frequency of data points falling into each interval. Relative Frequency and Cumulative Frequency Distribution: Similar to the methods for discrete data, you can calculate relative frequencies and cumulative frequencies for intervals. Histogram: A histogram is a graphical representation of frequency distribution for both discrete and continuous data. In the case of continuous data, it consists of bars that represent intervals and their corresponding frequencies. The width of each bar is proportional to the width of the interval, and the height represents the frequency. Frequency Polygon: A frequency polygon is a line graph that shows the frequency distribution of data. It is particularly useful for continuous data when you want to visualize the distribution of values. In both cases, tabulating data helps to summarize, visualize, and understand the distribution of values within a dataset, whether they are discrete or continuous. It's important to choose appropriate intervals and methods based on the nature of the data and the goals of your analysis. Discrete Data Continuous Data The type of data that has clear spaces This information falls into a continuous series. between values is discrete data. 39 Countable. Measurable There are distinct or different values in Every value within a range is included in discrete data. continuous data. Depicted using bar graphs Depicted using histograms Ungrouped frequency distribution of Grouped distribution of continuous data discrete data is performed against a tabulation frequencies is performed against a single value. value group. 1.8 SUMMARY The term "statistics" has two different meanings: singular and plural. When used in the plural, it denotes a collection of numbers, also referred to as statistical data. In its singular form, statistics refers to a scientific approach to the gathering, evaluation, and interpretation of data. Any collection of numbers cannot be regarded as statistical information or data. A set of numerical figures gathered for a study into a particular issue may only be regarded as data if they are comparable and influenced by a variety of variables. Statistics is a scientific method that is applied in practically all areas of the natural and social sciences. Theoretical Statistics and Applied Statistics are the two main divisions of statistics as a method. Applied statistics and theoretical statistics. Descriptive, inductive, and inferential statistics are subcategories of theoretical statistics. To gather, present, and analyses numerical data on a scientific foundation, statistics are utilized. To make it easier to compare characteristics in two or more scenarios, several statistical methods are used to provide complex masses of data in a simplified manner. Additionally, statistics offer crucial methods for the investigation of relationships between two or more features (or variables), forecasting, hypothesis testing, quality assurance, decision-making, etc. Nearly all areas of the natural and social sciences make use of statistics as a scientific method. 40 For the modern government to guarantee effective administration and the achievement of welfare goals, statistics are a crucial tool. Without numbers, planning is essentially impossible to imagine. In the contemporary business world, statistics are becoming increasingly important. Every company, large or little, employs statistics to analyses various business circumstances, including whether it would be feasible to start a new business. It is important to always be aware of the limitations of statistics. Only when data can be stated in terms of numbers do statistical approaches apply. The analysis' findings only hold true on average and are applicable to collections of people or units. 1.9 KEYWORDS Applied Statistics: It consists of the application of statistical methods to practical problems. Design of sample surveys, Descriptive Statistics: All those methods which are used for the collection, classification, tabulation, diagrammatic presentation of data and the methods of calculating average, dispersion, correlation and regression, index numbers, etc., are included in descriptive statistics. Inductive Statistics: It includes all those methods which are used to make generalizations about a population on the basis of a sample. The techniques of forecasting are also included in inductive statistics. Inferential Statistics: It includes all those methods which are used to test certain hypotheses regarding characteristics of a population. National income accounting: The system of keeping the accounts of income and expenditure of a country is known as national income accounting. 1.10 LEARNING ACTIVITY 1.“Statistics are numerical statements of facts, but all facts stated numerically are not statistics”. Clarify this statement and point out briefly which numerical statements of facts are statistics. ___________________________________________________________________________ ___________________________________________________________________________ 41 2. “Statistics are the straws out of which one like other economists have to make bricks”. Discuss. ___________________________________________________________________________ ___________________________________________________________________________ 1.11 UNIT END QUESTIONS A. Descriptive Questions Short Questions 1. Define the term statistics. 2. Explain basic terms of statistics 3. Explain primary data? 4. Explain Secondary data? 5. Explain Discrete and Continuous Data? Long Questions 1. Explain different types of data? 2. What are Scales of Measurement in Statistics? 3. Difference between Quantitative data and Qualitative data? 4. Difference between Discrete and Continuous Data? 5. Discuss the scope and significance of the study of statistics. B. Multiple Choice Questions 1. Which of the following is a branch of statistics? a. Descriptive statistics b. Inferential statistics c. Industry statistics d. Both A and B 2. The control charts and procedures of descriptive statistics which are used to enhance a procedure can be classified into which of these categories? a. Behavioural tools b. Serial tools c. Industry statistics 42 d. Statistical tools 3. Specialised processes such as graphical and numerical methods are utilised in which of the following? a. Education statistics b. Descriptive statistics c. Business statistics d. Social statistics 4. A parameter is a measure which is computed from a. Population data b. Sample data c. Test statistics d. None of these 5. To which of the following options do individual respondents, focus groups, and panels of respondents belong? a. Primary data sources b. Secondary data sources c. Itemised data sources d. Pointed data sources Answers: 1-d, 2-d, 3-b, 4-c, 5-a 1.12 REFERENCES References book 1. Statistics for Management, T N Srivastava &Shailaja Rego, Tata Mc Graw Hill Publishing Company 2. Business Statistics, Ken Black, Wiley 3. Statistics using SPSS, Sharon Lawner Weinberg & Sarah Knapp Abramowitz, Cambridge University Press 43