FRM Exam Part I Quantitative Analysis 2023 PDF
Document Details
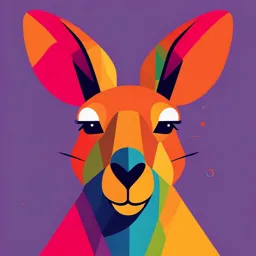
Uploaded by WellGalaxy
2023
GARP
Tags
Summary
This document is a table of contents from a GARP FRM Exam Part 1 Quantitative Analysis guide for 2023. It shows various chapters relevant to quantitative analysis in finance.
Full Transcript
2023 Quantitative Analysis 9780138052379_FRM23_P1_QA_TP.indd 1 9/27/22 3:25 PM 00006613-00000002_FM_pi-xii.indd 1 05/10/22 1:17 PM ...
2023 Quantitative Analysis 9780138052379_FRM23_P1_QA_TP.indd 1 9/27/22 3:25 PM 00006613-00000002_FM_pi-xii.indd 1 05/10/22 1:17 PM Copyright © 2023, 2022, 2021 by the Global Association of Risk Professionals All rights reserved. This copyright covers material written expressly for this volume by the editor/s as well as the compilation itself. It does not cover the individual selections herein that first appeared elsewhere. Permission to reprint these has been obtained by Pearson Education, Inc. for this edition only. Further reproduction by any means, electronic or mechanical, including photocopying and recording, or by any information storage or retrieval system, must be arranged with the individual copyright holders noted. All trademarks, service marks, registered trademarks, and registered service marks are the property of their respective owners and are used herein for identification purposes only. Pearson Education, Inc., 330 Hudson Street, New York, New York 10013 A Pearson Education Company www.pearsoned.com Printed in the United States of America ScoutAutomatedPrintCode 000022180-00000002 / A103000319319 EEB/MB ISBN 10: 0-138-05237-9 ISBN 13: 978-0-138-05237-9 00006613-00000002_FM_pi-xii.indd 2 14/09/22 8:00 PM Contents Chapter 1 Fundamentals Chapter 2 Random Variables 11 of Probability 1 2.1 Definition of a Random Variable 12 1.1 Sample Space, Event Space, 2.2 Discrete Random Variables 13 and Events 2 2.3 Expectations 14 Probability 2 Properties of the Expectation Operator 14 Fundamental Principles of Probability 4 Conditional Probability 4 2.4 Moments 14 Example: SIFI Failures 5 The Four Named Moments 16 Moments and Linear Transformations 17 1.2 Independence 5 Conditional Independence 6 2.5 Continuous Random Variables 18 1.3 Bayes’ Rule 7 2.6 Quantiles and Modes 20 1.4 Summary 7 2.7 Summary 22 Questions 8 Questions 23 Short Concept Questions 8 Short Concept Questions 23 Practice Questions 8 Practice Questions 23 Answers 9 Answers 24 Short Concept Questions 9 Short Concept Questions 24 Solved Problems 9 Solved Problems 25 iii 00006613-00000002_FM_pi-xii.indd 3 14/09/22 8:00 PM 4.2 Expectations and Moments 51 Chapter 3 ommon Univariate C Expectations 51 Random Variables 27 Moments 52 The Variance of Sums of Random Variables 53 3.1 Discrete Random Variables 28 Covariance, Correlation, Bernoulli 28 and Independence 54 Binomial 29 4.3 Conditional Expectations 55 Poisson 30 Conditional Independence 55 3.2 Continuous Random Variables 31 4.4 Continuous Random Variables 56 Uniform 31 Marginal and Conditional Distributions 57 Normal 32 Approximating Discrete Random 4.5 Independent, Identically Variables 34 Distributed Random Variables 57 Log-Normal 34 4.6 Summary 58 x2 36 Student’s t 37 Questions 59 Short Concept Questions 59 F 38 Practice Questions 59 Exponential 39 Beta 40 Answers 60 Mixtures of Distributions 40 Short Concept Questions 60 Solved Problems 60 3.3 Summary 43 Questions 44 Short Concept Questions 44 Chapter 5 Sample Moments 63 Practice Questions 44 Answers 45 Short Concept Questions 45 5.1 The First Two Moments 64 Solved Problems 45 Estimating the Mean 64 Estimating the Variance and Standard Deviation 65 Presenting the Mean and Standard Chapter 4 ultivariate Random M Deviation 66 Variables 47 Estimating the Mean and Variance Using Data 67 5.2 Higher Moments 67 4.1 Discrete Random Variables 48 5.3 The BLUE Mean Estimator 70 Probability Matrices 48 Marginal Distributions 49 5.4 Large Sample Behavior Independence 50 of the Mean 71 Conditional Distributions 50 5.5 The Median and Other Quantiles 73 iv Contents 00006613-00000002_FM_pi-xii.indd 4 14/09/22 8:00 PM 5.6 Multivariate Moments 74 Answers 96 Covariance and Correlation 74 Short Concept Questions 96 Sample Mean of Two Variables 75 Solved Problems 96 Coskewness and Cokurtosis 76 5.7 Summary 78 Questions 79 Chapter 7 Linear Regression 101 Short Concept Questions 79 Practice Questions 79 7.1 Linear Regression 102 Answers 80 Linear Regression Parameters 102 Short Concept Questions 80 Linearity 103 Solved Problems 80 Transformations 103 Dummy Variables 104 7.2 Ordinary Least Squares 104 Chapter 6 Hypothesis Testing 83 7.3 Properties of OLS Parameter Estimators 106 6.1 Elements of a Hypothesis Test 84 Shocks Are Mean Zero 106 Null and Alternative Hypotheses 84 Data Are Realizations from iid Random Variables 107 One-Sided Alternative Hypotheses 85 Variance of X 108 Test Statistic 85 Constant Variance of Shocks 108 Type I Error and Test Size 86 No Outliers 108 Critical Value and Decision Rule 86 Implications of OLS Assumptions 109 Example: Testing a Hypothesis about the Mean 86 7.4 Inference and Hypothesis Testing 110 Type II Error and Test Power 88 7.5 Application: CAPM 111 Example: The Effect of Sample Size on Power 89 7.6 Application: Hedging 113 Example: The Effect of Test Size and Population Mean on Power 90 7.7 Application: Performance Confidence Intervals 90 Evaluation 114 The p-value of a Test 91 7.8 Summary 116 6.2 Testing the Equality of Questions 117 Two Means 93 Short Concept Questions 117 6.3 Summary 94 Practice Questions 117 Questions 95 Answers 118 Short Concept Questions 95 Short Concept Questions 118 Practice Questions 95 Solved Problems 118 Contents v 00006613-00000002_FM_pi-xii.indd 5 14/09/22 8:00 PM Residual Plots 148 Chapter 8 Regression with Outliers 148 Multiple Explanatory 9.6 Strengths of OLS 149 Variables 121 9.7 Summary 151 Questions 152 8.1 Multiple Explanatory Variables 122 Short Concept Questions 152 Additional Assumptions 123 Practice Questions 152 8.2 Application: Multi-Factor Risk Answers 155 Models 124 Short Concept Questions 155 8.3 Measuring Model Fit 127 Solved Problems 155 8.4 Testing Parameters In Regression Models 129 Chapter 10 Stationary Time Multivariate Confidence Intervals 130 Series 161 The F-statistic of a Regression 133 8.5 Summary 133 10.1 Stochastic Processes 162 8.6 Appendix 134 Tables 134 10.2 Covariance Stationarity 163 Questions 136 10.3 White Noise 164 Short Concept Questions 136 Dependent White Noise 165 Practice Questions 136 Wold’s Theorem 165 Answers 137 10.4 Autoregressive (AR) Models 165 Short Concept Questions 137 Autocovariances and Autocorrelations 166 Solved Problems 137 The Lag Operator 166 AR(p) 167 10.5 Moving Average (MA) Models 171 Chapter 9 Regression 10.6 Autoregressive Moving Diagnostics 141 Average (ARMA) Models 173 10.7 Sample Autocorrelation 176 9.1 Omitted Variables 142 Joint Tests of Autocorrelations 176 Specification Analysis 176 9.2 Extraneous Included Variables 143 10.8 Model Selection 179 9.3 The Bias-Variance Tradeoff 143 Box-Jenkins 180 9.4 Heteroskedasticity 144 10.9 Forecasting 180 Approaches to Modeling Heteroskedastic Data 147 10.10 Seasonality 183 9.5 Multicollinearity 147 10.11 Summary 184 vi Contents 00006613-00000002_FM_pi-xii.indd 6 14/09/22 8:00 PM Appendix 184 Characteristic Equations 184 Chapter 12 Measuring Returns, Questions 185 Volatility, and Short Concept Questions 185 Correlation 213 Practice Questions 185 Answers 186 12.1 Measuring Returns 214 Short Concept Questions 186 12.2 Measuring Volatility and Risk 215 Solved Problems 186 Implied Volatility 216 12.3 The Distribution of Financial Chapter 11 Non-Stationary Returns 216 Power Laws 217 Time Series 189 12.4 Correlation Versus Dependence 218 11.1 Time Trends 190 Alternative Measures of Correlation 218 Polynomial Trends 190 12.5 Summary 221 11.2 Seasonality 192 Questions 222 Seasonal Dummy Variables 193 Short Concept Questions 222 11.3 Time Trends, Seasonalities, Practice Questions 222 and Cycles 193 Answers 223 11.4 Random Walks and Unit Short Concept Questions 223 Roots 196 Solved Problems 223 Unit Roots 196 The Problems with Unit Roots 196 Testing for Unit Roots 197 Seasonal Differencing 200 Chapter 13 Simulation and Bootstrapping 225 11.5 Spurious Regression 201 11.6 When to Difference? 202 13.1 Simulating Random Variables 226 11.7 Forecasting 203 Forecast Confidence Intervals 204 13.2 Approximating Moments 226 The Mean of a Normal 228 11.8 Summary 204 Approximating the Price of a Call Questions 206 Option 229 Short Concept Questions 206 Improving the Accuracy of Simulations 231 Practice Questions 206 Antithetic Variates 231 Answers 208 Control Variates 231 Short Concept Questions 208 Application: Valuing an Option 232 Solved Problems 208 Limitation of Simulations 233 Contents vii 00006613-00000002_FM_pi-xii.indd 7 14/09/22 8:00 PM 13.3 Bootstrapping 233 Questions 252 Bootstrapping Stock Market Returns 234 Short Concept Questions 252 Limitations of Bootstrapping 236 Practice Questions 252 13.4 Comparing Simulation Answers 253 and Bootstrapping 236 Answers to Short Concept Questions 253 13.5 Summary 236 Answers to Practice Questions 254 Questions 237 Short Concept Questions 237 Chapter 15 Machine Learning Practice Questions 237 and Prediction 257 Answers 238 Short Concept Questions 238 15.1 Dealing with Categorical Variables 258 Solved Problems 238 15.2 Regularization 258 Ridge Regression 258 LASSO 259 Chapter 14 Machine-Learning Elastic Net 259 Methods 241 Regularization Example 259 15.3 Logistic Regression 260 14.1 Types of Machine Learning 242 Logistic Regression Example 261 14.2 Data Preparation 243 15.4 Model Evaluation 261 Data Cleaning 243 15.5 Decision Trees 263 14.3 Principal Components Ensemble Techniques 266 Analysis 243 Bootstrap Aggregation 266 14.4 The K-means Clustering Random Forests 266 Algorithm 244 Boosting 267 Performance Measurement for K-means 245 15.6 K-Nearest Neighbors 267 Selection of K 245 15.7 Support Vector Machines 267 K-Means Example 245 SVM Example 268 14.5 Machine-Learning Methods for SVM Extensions 268 Prediction 246 15.8 Neural Networks 268 Overfitting 246 Neural Network Example 270 Underfitting 247 Questions 272 14.6 Sample Splitting and Short Concept Questions 272 Preparation 248 Practice Questions 272 Training, Validation, and Test Data 248 Answers 274 Cross-validation Searches 248 Answers to Short Concept Questions 274 14.7 Reinforcement Learning 249 Answers to Practice Questions 276 14.8 Natural Language Processing 250 Index279 viii Contents 00006613-00000002_FM_pi-xii.indd 8 14/09/22 8:00 PM PREFACE On behalf of our Board of Trustees, GARP’s staff, and particu- effects of the pandemic on our exam administration, we were larly its certification and educational program teams, I would able to ensure that none of the changes resulted in additional like to thank you for your interest in and support of our Financial charges to candidates. In addition to free deferrals, we provided Risk Manager (FRM®) program. candidates with new materials at no cost when those unavoid- able deferrals rolled into a new exam period, in which new The past few years have been especially difficult for the topics were covered due to curriculum updates. financial-services industry and those working in it because of the many disruptions caused by COVID-19. In that regard, our Since its inception in 1997, the FRM program has been the sincere sympathies go out to anyone who was ill, suffered a loss global industry benchmark for risk-management professionals due to the pandemic, or whose career aspirations or opportuni- wanting to demonstrate objectively their knowledge of financial ties were hindered. risk-management concepts and approaches. Having FRM hold- ers on staff gives companies comfort that their risk-management The FRM program has experienced many COVID-related chal- professionals have achieved and demonstrated a globally recog- lenges, but GARP has always placed candidate safety first. nized level of expertise. During the pandemic, we’ve implemented many proactive measures to ensure your safety, while meeting all COVID-related Over the past few years, we’ve seen a major shift in how individ- requirements issued by local and national authorities. For exam- uals and companies think about risk. Although credit and market ple, we cancelled our entire exam administration in May 2020, risks remain major concerns, operational risk and resilience and and closed testing sites in specific countries and cities due to liquidity have made their way forward to become areas of mate- local requirements throughout 2020 and 2021. To date in 2022, rial study and analysis. And counterparty risk is now a bit more we’ve had to defer many FRM candidates as a result of COVID. interesting given the challenges presented by a highly volatile and uncertain global environment. Whenever we were required to close a site or move an exam administration, we affirmatively sought to mitigate the impact The coming together of many different factors has changed and on candidates by offering free deferrals and seeking to create will continue to affect not only how risk management is prac- additional opportunities to administer our examinations at ticed, but also the skills required to do so professionally and at different dates and times, all with an eye toward helpingcan- a high level. Inflation, geopolitics, stress testing, automation, didates work their way through the FRM program in a timely technology, machine learning, cyber risks, straight-through pro- way. cessing, the impact of climate risk and its governance structure, and people risk have all moved up the list of considerations that It’s been our objective to assure exam candidates we will do need to be embedded into the daily thought processes of any everything in our power to assist them in furthering their career good risk manager. These require a shift in thinking and raise objectives in what is and remains a very uncertain and trying questions and concerns about whether a firm’s daily processes professional environment. Although we could not control the Preface ix 00006613-00000002_FM_pi-xii.indd 9 14/09/22 8:00 PM are really fine-tuned, or if its data and information flows are fully FRM candidates are aware of not only what is important but also understood. what we expect to be important in the near future. As can be readily seen, we’re living in a world where risks are We’re committed to offering a program that reflects the becoming more complex daily. The FRM program addresses dynamic and sophisticated nature of the risk-management these and other risks faced by both non-financial firms and those profession. in the highly interconnected and sophisticated financial-services We wish you the very best as you study for the FRM exams, and industry. Because its coverage is not static, but vibrant and in your career as a risk-management professional. forward looking, the FRM has become the global standard for financial risk professionals and the organizations for which they work. Yours truly, The FRM curriculum is regularly reviewed by an oversight com- mittee of highly qualified and experienced risk-management professionals from around the globe. These professionals include senior bank and consulting practitioners, government regulators, asset managers, insurance risk professionals, and academics. Their mission is to ensure the FRM program remains Richard Apostolik current and its content addresses not only standard credit and market risk issues, but also emerging issues and trends, ensuring President & CEO x Preface 00006613-00000002_FM_pi-xii.indd 10 14/09/22 8:00 PM FRM ® COMMITTEE Chairperson Nick Strange, FCA Senior Technical Advisor, Operational Risk & Resilience, Prudential Regulation Authority, Bank of England Members Richard Apostolik Keith Isaac, FRM President and CEO, GARP VP, Capital Markets Risk Management, TD Bank Group Richard Brandt William May MD, Operational Risk Management, Citigroup SVP, Global Head of Certifications and Educational Programs, GARP Julian Chen, FRM SVP, FRM Program Manager, GARP Attilio Meucci, PhD, CFA Founder, ARPM Chris Donohue, PhD MD, GARP Benchmarking Initiative, GARP Victor Ng, PhD Chairman, Audit and Risk Committee Donald Edgar, FRM MD, Head of Risk Architecture, Goldman Sachs MD, Risk & Quantitative Analysis, BlackRock Matthew Pritsker, PhD Hervé Geny Senior Financial Economist and Policy Advisor/Supervision, Former Group Head of Internal Audit, London Stock Exchange Regulation, and Credit, Federal Reserve Bank of Boston Group Samantha C. Roberts, PhD, FRM, SCR Aparna Gupta Instructor and Consultant, Risk Modeling and Analytics Professor of Quantitative Finance A.W. Lawrence Professional Excellence Fellow Til Schuermann, PhD Co-Director and Site Director, NSF IUCRC CRAFT Partner, Oliver Wyman Lally School of Management Sverrir Þorvaldsson, PhD, FRM Rensselaer Polytechnic Institute Senior Quant, SEB John Hull Senior Advisor Maple Financial Professor of Derivatives and Risk Management, Joseph L. Rotman School of Management, University of Toronto FRM® Committee xi 00006613-00000002_FM_pi-xii.indd 11 22/09/22 5:10 PM ATTRIBUTIONS Author Kevin Sheppard, PhD, Associate Professor in Financial Economics and Tutorial Fellow in Economics at the University of Oxford Contributor Chris Brooks, Ph.D., Professor of Finance, School of Accounting and Finance, University of Bristol Reviewers Paul Feehan, PhD, Professor of Mathematics and Director of the John Craig Anderson, FRM, Director—Debt and Capital Masters of Mathematical Finance Program, Rutgers University Advisory Services, Advize Capital Erick W. Rengifo, PhD, Associate Professor of Economics, Thierry Schnyder, FRM, CSM, Risk Analyst, Bank for Fordham University International Settlements Richard Morrin, PhD, FRM, ERP, CFA, CIPM, Financial Officer, Yaroslav Nevmerzhytskyi, FRM, CFA, ERP, Deputy Chief Risk International Finance Corporation Officer and Financial Risk Management Team Leader, Naftogaz of Ukraine Antonio Firmo, FRM, Senior Audit Manager—Trading Risk, Risk Capital, and Modeling, Royal Bank of Scotland Deco Caigu Liu, FRM, CSC, CBAP, Global Asset Liability Management, Manulife xii Attributions 00006613-00000002_FM_pi-xii.indd 12 22/09/22 5:10 PM Fundamentals of Probability 1 Learning Objectives After completing this reading you should be able to: Describe an event and an event space. Define and calculate a conditional probability. Describe independent events and mutually exclusive Distinguish between conditional and unconditional events. probabilities. Explain the difference between independent events and Explain and apply Bayes’ rule. conditionally independent events. Calculate the probability of an event for a discrete probability function. 1 00006613-00000002_CH01_p001-010.indd 1 26/07/22 8:36 PM Probability theory is the foundation of statistics, econometrics, Events are subsets of a sample space, and an event is denoted and risk management. This chapter introduces probability in its by v. An event is a set of outcomes and may contain one or simplest form. A probability measures the likelihood that some more of the values in the sample space, or it may even contain event occurs. In most financial applications of probability, events no elements.3 When an event includes only one outcome, it is are tightly coupled with numeric values. Examples of such often referred to as an elementary event. Events are sets and events include the loss on a portfolio, the number of defaults are usually written with set notation. For example, when inter- in a mortgage pool, or the sensitivity of a portfolio’s value to a ested in the sum of two dice, one event is that the sum is odd: rise in short-term interest rates. Events can also be measured by {(1,2), (1,4), (1,6),... , (4,5), (5,6)}. Another event is that the sum values without a natural numeric correspondence. These include is greater than or equal to nine: {(4,5), (4,6), (5,5), (5,6), (6,6)}. categorical variables, such as the type of a financial institution or The event space, usually denoted by F , consists of all combina- the rating on a corporate bond. tions of outcomes to which probabilities can be assigned. Note Probability is introduced through three fundamental principles. that the event space is an abstract concept and separate from any specific application. As an example, suppose that {A}, {B} are the 1. The probability of any event is non-negative. possible outcomes of an experiment. Without knowing what {A}, 2. The sum of the probabilities across all outcomes is one. {B} mean in practice, one might consider the following events. 3. The joint probability of two independent events is the prod- 1. A occurs, B does not. uct of the probability of each. 2. B occurs, A does not. This chapter also introduces conditional probability, an impor- 3. Both A and B occur. tant concept that assigns probabilities within a subset of all events. After that, the chapter moves on to discuss indepen- 4. Neither A nor B occur. dence and conditional independence. It concludes by examining This would give an event space consisting of four events Bayes’ rule, which provides a simple yet powerful expression {A, B, {A, B}, ∅}. Because this event space has a finite number of for incorporating new information or data into probability outcomes, it is called a discrete probability space.4 estimates. In the corporate default example, the event space is {Default, No Default, {Default, No Default}, ∅}. It might appear 1.1 SAMPLE SPACE, EVENT SPACE, surprising that the event space contains two “impossible” events for this example: AND EVENTS 1. {Default, No Default}, which contains both outcomes; and A sample space is a set containing all possible outcomes of an 2. The empty set ∅, which contains neither. experiment and is denoted as Ω. The set of outcomes depends on the problem being studied. For example, when examining However, note that a definite probability of 0 can be assigned to the returns on the S&P 500, the sample space is the set of all “impossible” events. Because the event space contains all sets real numbers and is denoted by ℝ.1 On the other hand, if the that can be assigned a probability, these two events are there- focus is on the direction of the return on the S&P 500, then the fore part of the event space. sample space is {Positive, Negative}. When modeling corporate defaults, the sample space is {Default, No Default}. When rolling Probability a single six-sided die, the sample space is {1, 2,... , 6}. The sample space for two identical six-sided dice contains 21 ele- Probability measures the likelihood of an event. Probabilities ments representing all distinct pairs of values: {(1,1), (1,2), are always between 0 and 1 (inclusive). An event with prob- (1,3),... , (5,5), (5,6), (6,6)}.2 If we are modeling the sum of two ability 0 never occurs, while an event with a probability 1 always dice, however, then the sample space is {2,... , 12}. occurs. The simplest interpretation of probability is that it is the frequency with which an event would occur if a set of indepen- 1 dent experiments was run. This interpretation of probability is The sample space in this example is truncated at the lower end since the return cannot be less than -100%. known as the frequentist interpretation and focuses on objective 2 This assumes that the dice are indistinguishable and therefore one can only observe the values shown (and not which die produced each value). 3 An event that contains no elements is known as the empty set. If the dice were distinguishable from each other (e.g., they have differ- 4 ent colors), then the sample space would contain 36 values (because A discrete probability space is one that contains either finitely many {1,2} and {2,1} are different). outcomes or (possibly) countably infinitely many outcomes. 2 Financial Risk Manager Exam Part I: Quantitative Analysis 00006613-00000002_CH01_p001-010.indd 2 26/07/22 8:36 PM probability. Note that this is a conceptual interpretation, denote subsets. Three important set operators shown in the fig- whereas finance is mostly focused on non-experimental events ure are intersections, unions, and complements: x is the intersection operator, which means that Ax B is (e.g., the return on the stock market). h is the union operator, which means that Ah B is the Probability can also be interpreted from a subjective point of view. the set of outcomes that appear in both A and B. Under this alternative interpretation, probability reflects or incor- porates an individual’s beliefs about the likelihood of an event set that contains all outcomes in A, or B, or both. occurring. These beliefs may differ across individuals and do not Ac is the complement of the set A, and is the set of all have to agree with the objective probability of an event. In fact, outcomes that are not in A. there might not even be a single objective probability that can Finally, the bottom-right panel illustrates mutually exclusive be assigned to each outcome in many real-world situations if the events, which means that either A or B could happen, but not true model or process generating the outcomes is unknown. An both together. Here, the intersection Ax B is the empty set (∅). example of this would be a senior executive assuming a specific probability for an event (e.g., a recession, an interest rate increase, Recall the earlier example of rolling two dice, which featured or a rating downgrade) as part of a scenario analysis or stress test. two events: 1. The event that the sum rolled is greater than or equal to Probability is defined over event spaces. Mathematically, it nine; and assigns a number between 0 and 1 (inclusive) to each event in the event space. As stated above, events are simply combina- 2. The event that the sum is odd. tions of outcomes (i.e., subsets of the sample space). It is there- The intersection of these two events contains the outcomes from fore possible to illustrate some core probability concepts using the sample space where both conditions are simultaneously satis- the language of sets. fied. This happens when the sum is equal to nine or 11, meaning Figure 1.1 illustrates the key properties of sets, with each rect- that the intersection of these two events consists of the outcomes angle representing a sample space (Ω) comprised of two events {(4,5), (5,6)}. The union of the two events includes all outcomes in (i.e., A and B). Note that the standard notation for a set uses both sets without duplication (i.e., the outcomes in the set of odd capital letters (e.g., A, B), while subscripts (e.g., Ai) are used to sums as well as the even sums greater than or equal to nine). The Intersection Union Complement Mutually Exclusive Figure 1.1 The top-left panel illustrates the intersection of two sets, which is indicated by the shaded area surrounded by the dark solid line. The top right panel illustrates the union of two sets, which is indicated by the shaded area surrounded by the dark solid line. The shaded area in the bottom-left panel illustrates Ac, which is the complement to A and includes every outcome that is not in A. The bottom-right panel illustrates mutually exclusive sets, which have an empty intersection (denoted by ∅). Chapter 1 Fundamentals of Probability 3 00006613-00000002_CH01_p001-010.indd 3 26/07/22 8:36 PM complement of the event that the sum is odd is the set of out- 2008), the probability of another failure is likely to be higher. comes where the sum of two dice is even: ({1,1}, {1,3}, {1,5},... , This difference is important, especially in risk management. (5,5), (6,6)}. Finally, the intersection of the event that the sum is Conditional probability can also be used to incorporate addi- odd with the event that the sum is even is the null set (because tional information to update unconditional probabilities. For there cannot be a sum which is simultaneously odd and even). example, consider two randomly chosen students preparing to This is an example of two mutually exclusive events. take a risk management exam, which is passed (on average) by 50% of test takers. Based on only this information, one would Fundamental Principles of Probability estimate that both Student X and Student Y have a 50% chance of passing the exam. The three fundamental principles of probability are defined using events, event spaces, and sample spaces. Note that Pr(v) Now suppose that the average length of study time needed to is a function that returns the probability of an event v. pass the exam is 200 hours. Suppose further that Student X studied for less than 100 hours, whereas Student Y studied for more than 1. Any event A in the event space F has Pr(A) Ú 0. 400 hours. Common sense would say that Student Y has a higher 2. The probability of all events in Ω is one and thus Pr(Ω) = 1. probability of passing compared to Student X. In fact, a survey Pr(A1 h A2) = Pr(A1) + Pr(A2). This holds for any number 3. If the events A1 and A2 are mutually exclusive, then might find that the probability of passing for students who studied n of mutually exclusive events, so that Pr(A1 h A2 ch An) less than 100 hours is 10%, whereas the probability of passing for students who studied more than 400 hours is 80%. Using this new = a i = 1Pr(Ai).5 n information, the conditional probability that X passes the exam is 10%, while the conditional probability that Y passes is 80%. These three principles—collectively known as the Axioms of Probability—are the foundations of probability theory. They The conditional probability of the event A occurring given that B imply two useful properties of probability. First, the probability has occurred is denoted as Pr(A B) and can be calculated as: of an event or its complement must be 1. Pr(Ax B) c c Pr(A B) = (1.3) Pr(Ah A ) = Pr(A) + Pr(A ) = 1 (1.1) Pr(B) Second, the probability of the union of any two sets can be The probability of observing an event in A, given that an event decomposed into: in B has been observed, depends on the probability of observ- Pr(A h B) = Pr(A) + Pr(B) - Pr(Ax B) ing events that are in both rescaled by the probability that an (1.2) event in B occurs. In other words, conditional probability oper- This result demonstrates that the total probability of two events ates as if B is the event space and A is an event inside this new, is the probability of each event minus the probability of the event restricted space. defined by the intersection. The elements in the intersection are in As a simple example, note that the probability of rolling a three on both sets, therefore subtracting this term avoids double counting. a fair die is 1/6. This is because the event of rolling a three occurs in one outcome in a set of six possible outcomes: {1, 2, 3, 4, 5, 6}. Conditional Probability However, if it is known that the number rolled is odd, then the Probability is commonly used to examine events in subsets of probability of rolling a three is 1/3 because this additional informa- the full event space. Specifically, we are often interested in the tion restricts the set of outcomes to three possibilities: {1, 3, 5}. probability of an event happening only if another event hap- Figure 1.2 demonstrates this idea using sets. The left panel pens first. This concept is called conditional probability because shows the unconditional probability where Ω is the event space we are computing a probability on that condition that another and A and B are events. The right panel shows the conditional event occurs. probability of A given B. The numeric values show the probabil- For example, we might want to determine the probability that a ity of each region, meaning that the unconditional probability of large financial institution fails given that another large financial A is Pr(A) = 16% and the conditional probability of A given B is institution has also failed. Note that the probability of a large Pr(A B) = 12%/48% = 25%. financial institution failing is normally quite low. However, when An important application of conditional probability is the Law of one large financial institution fails (e.g., Lehmann Brothers in Total Probability. This law states that the total probability of an event can be reconstructed using conditional probabilities under 5 This result holds more generally for infinite collections of mutually the condition that the probability of the sets being conditioned exclusive events under some technical conditions. is equal to 1. 4 Financial Risk Manager Exam Part I: Quantitative Analysis 00006613-00000002_CH01_p001-010.indd 4 26/07/22 8:36 PM Unconditional Probability Conditional Probability, Pr (A|B) Figure 1.2 The left panel shows the unconditional probability of A and B. The right panel shows the conditional probability of A given B. The numeric values indicate the probability of each region. For example, Pr(B) + Pr(Bc) = 1, and so: Example: SIFI Failures Pr(A) = Pr(A B) Pr(B) + Pr(A Bc) Pr(Bc) (1.4) Systemically Important Financial Institutions (SIFIs) are a cat- This property holds for any collection of mutually exclusive egory of designated large financial institutions that are deeply events {Bi}, where a Pr(Bi) = 1. Intuitively, this property must connected to large portions of the economy. They are usually hold because each outcome in A must occur in one (and only banks, although other types of institutions such as insurers, asset one) of the Bi. managers, or central counterparties may be designated as SIFIs as well. SIFIs are subject to additional regulation and supervi- Figure 1.3 illustrates the Law of Total Probability for events A, sion, as the failure of even one of them could lead to a major B1, B2, B3, and B4. Note that the probability that A occurs is disruption in financial markets. 9% + 12% + 14% = 35%. The four conditional probabilities corresponding to each set Bi are Suppose that the chance of at least one SIFI failing in any given year is 1%. Suppose further that when at least one SIFI fails, Pr(A B1) = 14%/30% = 46.6%, there is a 20% chance that the number of failing SIFIs is exactly Pr(A B2) = 9%/22% = 40.9%, 1, 2, 3, 4 or 5. In other words, there is a 0.2% chance that one Pr(A B3) = 12%/28% = 42.8%, SIFI fails, 0.2% chance that two institutions fail, and so on. Finally, assume that more than five SIFIs cannot fail, because Pr(A B4) = 0 governments and central banks would intervene. These conditional probabilities are then rescaled by the proba- If we define the event that one or more SIFIs fail as E1, then bilities of the conditioning event to recover the total probability. Pr(E1) = 1%. This is a small probability. However, if we are inter- a Pr(A Bi) Pr(Bi) = 46.6% * 30% + 40.9% * 22% + 42.8% 4 ested in the probability of two or more failures given that at i=1 least one has occurred, then this is Pr(E1 x E2) * 28% + 0 * 20% = 35%. Pr(E2) 0.8% Pr(E2 E1) = = = = 80% Pr(E1) Pr(E1) 1% Here, E2 is the event that two or more SIFIs fail, and it is a sub- failures). Because E1 x E2 = E2, Pr(E1 x E2) = Pr(E2). set of E1 by construction (because E1 is the event of one or more This conditional probability tells us that, while it would be sur- prising to see a SIFI failure in any given year, there is very likely to be two or more failures if there is at least one failure. The left panel of Figure 1.4 graphically illustrates this point. Figure 1.3 The four events B1 through B4 are mutually exclusive. A is the event indicated by the dark circle, 1.2 INDEPENDENCE and Pr (A) = 35%. The probability of each event Bi includes the values that overlap with A as well as those Independence is a core concept that is exploited throughout that do not. For example, Pr(B1) = 16% + 14% = 30%. statistics and econometrics. Two events are independent if the Chapter 1 Fundamentals of Probability 5 00006613-00000002_CH01_p001-010.indd 5 26/07/22 8:36 PM Unconditional Probability Conditional Probability Figure 1.4 The left panel illustrates the unconditional probability of at least one SIFI failing. The right panel ∼ illustrates the conditional probability of 1, 2, 3, 4 or 5 failures given that at least one SIFI has failed. The Ei are the events that exactly i SIFIs fail. These differ from Ei, which measure the event that i or more institutions fail. ∼ However, E5 = E5 because the maximum number of failures is assumed to be five. words, if A1, A2, c , An are independent events, then Pr(A1 x A2 x c x An) = Pr(A1) * Pr(A2) * c * Pr(An). probability that one event occurs does not depend on whether the other event occurs. When the two events A and B are inde- pendent, then: Pr(Ax B) = Pr(A) Pr(B) (1.5) Conditional Independence This implies that for independent events, the conditional prob- ability of the event is equal to the unconditional probability of Like probability, independence can be redefined to hold condi- the event. When A and B are independent, then: tional on another event. Two events A and B are conditionally independent if: Pr(Ax B) Pr(A) Pr(B) Pr(B A) = = = Pr(B) (1.6) Pr(A) Pr(A) Pr(Ax B C) = Pr(A C) Pr(B C) (1.7) It is also the case that Pr(A B) = Pr(A). The conditional probability Pr(Ax B C) is the probability of an outcome that is in both A and B occurring given that an out- If A and B are mutually exclusive, then B cannot occur if A come in C occurs. occurs. This means that the outcome of A affects Pr(B) and so mutually exclusive events are not independent. Another way to Note that two types of independence—unconditional and than 0, then Pr(A x B) = Pr(A) * Pr(B) 7 0 (i.e., there must be a see this is to note that when Pr(A) and Pr(B) are both greater conditional—do not imply each other. Events can be both uncon- ditionally dependent (i.e., not independent) and conditionally positive probability that both occur). However, mutually exclu- independent. Similarly, events can be unconditionally indepen- sive events have Pr(Ax B) = 0 and thus cannot be independent. dent, yet conditional on another event they may be dependent. Independence can be generalized to any number of events The left-hand panel of Figure 1.5 contains an illustration of using the same principle: the joint probability of the events two independent events. Each of the events A and B has a is the product of the probability of each event. In other 40% chance of occurring. The intersection has the probability Independence Conditional Independence Figure 1.5 The left panel shows two events are independent so that the joint probability, Pr(A ∩ B), is the same as the product of the probability of each event, Pr(A) : Pr(B). The right panel contains an example of two events, A and B, which are dependent but also conditionally independent given C. 6 Financial Risk Manager Exam Part I: Quantitative Analysis 00006613-00000002_CH01_p001-010.indd 6 26/07/22 8:36 PM Pr(A) * Pr(B) ( = 40% * 40% = 16%) occurring, and so the Combining these produces the conditional probability that the requirements for independence are satisfied. manager is a star given that she had a high return: Meanwhile, the right-hand panel shows an example where 20% * 10% 2% Pr(Star High Return) = = = 30.8% A and B are unconditionally dependent: Pr(A) = 40%, 6.5% 6.5% Pr(B) = 40% and Pr(Ax B) = 25% (rather than 16% as would be In this case, a single high return updates the probability that a required for independence). However, conditioning on the event manager is a star from 10% (i.e., the unconditional probability) to C restricts the space so that Pr(A C) = 50%, Pr(B C) = 50% 30.8% (i.e., the conditional probability). This idea can be extended and Pr(A C)Pr(B C) = 25%, meaning that these events are to classifying a manager given multiple returns from a fund. tional independence is that Pr(A B x C) = Pr(A C) = 50% and conditionally independent. A further implication of condi- Pr(B A x C) = Pr(B C) = 50%, which both hold. 1.4 SUMMARY Probability is essential for statistics, econometrics, and data 1.3 BAYES’ RULE analysis. This chapter introduces probability as a simple way to understand events that occur with different frequencies. Bayes’ rule provides a method to construct conditional prob- Understanding probability begins with the sample space, which abilities using other probability measures. It is both a simple defines the range of possible outcomes and events that are col- application of the definition of conditional probability and an lections of these outcomes. Probability is assigned to events, extremely important tool. The formal statement of Bayes’ rule is and these probabilities have three key properties. Pr(A B) Pr(B) Pr(B A) = (1.8) 1. Any event A in the event space F has Pr(A) Ú 0. Pr(A) 2. The probability of all events in Ω is 1 and therefore probability, because Pr(A B) = Pr(A x B)/Pr(B) so that This is a simple rewriting of the definition of conditional Pr(Ω) = 1. then Pr(A1 h A2) = Pr(A1) + Pr(A2). This holds for Pr(Ax B) = Pr(A B) Pr(B). Reversing these arguments also gives 3. If the events A1 and A2 are mutually exclusive, Pr(Ax B) = Pr(B A) Pr(A). Equating these two expressions and Pr(A1 h A2 c h An) = a i = 1Pr(Ai). solving for Pr(B A) produces Bayes’ rule. This approach uses the any number of mutually exclusive events, so that n unconditional probabilities of A and B, as well as information about the conditional probability of A given B, to compute the These properties allow a complete description of event prob- probability of B given A. abilities using a standard set of operators (e.g., union, inter- Bayes’ rule has many financial and risk management applica- section, and complement). Conditional probability extends tions. For example, suppose that 10% of fund managers are the definition of probability to subsets of all events, so that a superstars. Superstars have a 20% chance of beating their conditional probability is like an unconditional probability, but benchmark by more than 5% each year, whereas normal fund only within a smaller event space. managers have only a 5% chance of beating their benchmark by This chapter also examines independence and conditional inde- more than 5%. pendence. Two events are independent if the probability of both Suppose there is a fund manager that beat her benchmark by events occurring is the product of the probability of each event. 7%. What is the chance that she is a superstar? Here, event A is In other words, events are independent if there is no informa- the manager significantly outperforming the fund’s benchmark. tion about the likelihood of one event given knowledge of the Event B is that the manager is a superstar. Using Bayes’ rule: other. Independence extends to conditional independence, Pr(High Return Star) Pr(Star) which replaces the unconditional probability with a conditional Pr(Star High Return) = probability. An important feature of conditional independence Pr(High Return) is that events that are not unconditionally independent may be The probability of a superstar is 10%. The probability of a high conditionally independent. return if she is a superstar is 20%. The probability of a high return from either type of manager is Finally, Bayes’ rule uses basic ideas from probability to develop a precise expression for how information about one event can Pr(High Return) = Pr(High Return Star) Pr(Star) be used to learn about another event. This is an important prac- + Pr(High Return Normal) Pr(Normal) tical tool because analysts are often interested in updating their = 20% * 10% + 5% * 90% = 6.5% beliefs using observed data. Chapter 1 Fundamentals of Probability 7 00006613-00000002_CH01_p001-010.indd 7 26/07/22 8:36 PM The following questions are intended to help candidates understand the material. They are not actual FRM exam questions. QUESTIONS Short Concept Questions 1.1 Can independent events be mutually exclusive? 1.3 What are the sample spaces, events, and event spaces if 1.2 Can Bayes’ rule be helpful if A and B are independent? you are interested in measuring the probability of a corpo- What if A and B are perfectly dependent so that B is a rate takeover and whether it was hostile or friendly? subset of A? Practice Questions Pr(D Ah B h C) 1.4 Based on the percentage probabilities in the diagram a. Pr(AC) below, what are the values of the following? b. c. Pr(A A) A B d. Pr(B A) 12% 11% 16% e. Pr(C A) f. Pr(D A) 10% Pr(AC x DC) g. Pr((Ah D)C) 15% 18% h. i. Are any of the four events pairwise independent? 10% C 1.6 Continue the application of Bayes’ rule to compute the Ω probability that a manager is a star after observing two years of “high” returns. a. Pr(A) b. Pr(A B) 1.7 There are two companies in an economy, Company A Pr(Ax B x C) c. Pr(B A) and Company B. The default rate for Company A is 10%, d. and the default rate for Company B is 20%. Assume that e. Pr(B Ax C) defaults for the two companies occur independently. f. Pr(Ax B C) a. What is the probability that both companies default? g. Pr(Ah B C) b. What is the probability that either Company A or h. Considering the three distinct pairs of events, are any Company B defaults? of these pairs independent? 1.8 Credit card companies rapidly assess transactions 1.5 Based on the percentage probabilities in the plot below, for fraud. In each day, a large card issuer assesses what are the values of the following? 10,000,000 transactions. Of these, 0.001% are fraudulent. If their algorithm identifies 90% of all fraudulent transac- A B tions but also 0.0001% of legitimate transactions, what C is the probability that a transaction is fraudulent if it has 12% 9% 7% been flagged? 6% 15% 1.9 If fund managers beat their benchmark at random (meaning 9% 8% 7% a 50% chance that they beat their benchmark), and annual 12% returns are independent, what is the chance that a fund D Ω manager beats her benchmark for ten years in a row? 8 Financial Risk Manager Exam Part I: Quantitative Analysis 00006613-00000002_CH01_p001-010.indd 8 26/07/22 8:36 PM The following questions are intended to help candidates understand the material. They are not actual FRM exam questions. ANSWERS Short Concept Questions 1.1 No. For independent events, the probability of observing then Pr(B A) = 1, so that Pr(A B) is just the ratio of the both must be the product of the individual probabilities, probabilities, Pr(A)/Pr(B). Here the probability also only and mutually exclusive events have probability 0 of simul- depends on unconditional probabilities and so never taneously occurring. changes. Pr(B A)Pr(A) 1.3 Using the notation No Takeover (NT), Hostile Takeover 1.2 Bayes’ rule says that Pr(A B) =. If these Pr(B) (HT) and Friendly Takeover (FT), the sample space is {NT, events are independent, then Pr(B A) = Pr(B) so that HT, HT }. The events are all distinct combinations of the Pr(A B) = Pr(A). B has no information about A and so sample space including the empty set, f, NT, HT, FT, updating with Bayes’ rule never changes the conditional {NT, HT}, {NT, FT}, {HT, FT}, {NT, HT, FT }. The event probability. If these events are perfectly dependent, space is the set of all events. Solved Problems 1.4 a. Pr(A) = 12% + 11% + 15% + 10% = 48% c. This is trivially 100%. b. Pr(A B) = Pr(Ax B)/Pr(B) = (11% + 10%)/ d. Pr(B x A) = 9%. The conditional probability is (11% + 10% + 16% + 18%) = 38.2% 9% c. Pr(B A) = Pr(A x B)/Pr(A) = (11% + 10%)/48% = 30%. e. There is no overlap and so Pr(C x A) = 0. 30% = 43.8% d. Pr(Ax B x C) = 10% f. Pr(Dx A) = 9%. The conditional probability is 30%. e. Pr(B Ax C) = Pr(B x Ax C)/Pr(Ax C) g. This is the total probability not in A or D. It is 1 – Pr(Ah D) = 1 - (Pr(A) + Pr(D) - Pr(Ax D)) = 10%/(15% + 10%) = 40% f. Pr(Ax B C) = Pr(Ax B x C)/Pr(C) = 100% - (30% + 36% - 9%) = 43%. h. This area is the intersection of the space not in A with = 10%/(15% + 10% + 18% + 10%) = 18.9% g. Pr(Ah B C) = Pr((A h B)x C)/Pr(C) the space not in D. This area is the same as the area that is not in A or D, Pr((Ah D)C) and so 43%. = (15% + 10% + 18%)/53% = 81.1% i. The four regions have probabilities A = 30%, h. We can use the rule that events are indepen- B = 30%, C = 28% and D = 36%. The only region dent if their joint probability is the product of the that satisfied the requirement that the joint probability probability of each. Pr(A) = 48%, Pr(B) = 55%, is the product of the individual probabilities is A and B Pr(C) = 53%, Pr(Ax B) = 21% ≠ (48% * 55%), because Pr(Ax B) = 9% = Pr(A)Pr(B) = 30% * 30%. Pr(B x C) = 28% ≠ (55% * 53%). None of these Pr(Ax C) = 25% ≠ (48% * 53%), 1.6 Consider the three scenarios: (High, High), (High, Low) and (Low, Low). events are pairwise independent. We are interested in Pr (Star|High, High) using Bayes’ 1.5 a. 1 - Pr(A) = 100% - 30% = 70% b. This value is Pr(Dx (Ah B h C))/Pr(Ah B h C). rule, this is equal to Pr(High, High Star)Pr(Star) The total probability in the three areas A, B, and. Pr(High, High) C is 73%. The overlap of D with these three is 9% + 8% + 7% = 24%, and so the conditional prob- Stars produce high returns in 20% of years, and so 24% Pr(High, High Star) = 20% * 20% Pr (Star) is still 10%. ability is = 33%. Finally, we need to compute Pr (High, High), which is 73% Chapter 1 Fundamentals of Probability 9 00006613-00000002_CH01_p001-010.indd 9 26/07/22 8:36 PM The following questions are intended to help candidates understand the material. They are not actual FRM exam questions. Pr(Fraud x Flag)/Pr(Flag). The probability that a trans Pr(High, High Star) Pr(Star) + Pr(High, High Normal) 1.8 We are interested in Pr(Fraud|Flag). This value is Pr(Normal). This value is 20% * 20% * 10% + 5% * 5% = 0.000999%. The Pr(Fraud x Flag) = 0.001% * 90% = 90% = 0.625%. Combining these values, action is flagged is 0.001% * 90% + 99.999% * 0.0001% 20% * 20% * 10% = 64%. 0.0009% 0.625% = 0.0009%. Combining these values, = 90%. 0.000999% This is a large increase from the 30% chance after one year. This indicates that 10% of the flagged transactions are not 1.7 a. 0.10 * 0.20 = 0.02 S 2% actually fraudulent. b. Calculate this in two ways: 1.9 If each year was independent, then the probability of i. Using Equation 1.1: beating the benchmark ten years in a row is the product Pr(Ah B) = Pr(A) + Pr(B) - Pr(Ax B) of the probabilities of beating the benchmark each year, Pr(Ah B) = Pr(A) + Pr(B) - Pr(Ax B) 1 10 1 ¢ ≤ = ≈ 0.1%. = 10% + 20% - 2% = 28%. 2 1,024 ii. Using the identity: Pr(Ah AC) = Pr(A) + Pr(AC) = 1 Let C be the event of no defaults. Then Pr(C) = (1 - (1 - Pr(A)) * (1 - Pr(B)) = (1 - 10%) * (1 - 20%) = 0.9 * 0.8 = 72%. Then the complement of C is the event of any defaults Pr(CC) = 1 - Pr(C) = 28%. 10 Financial Risk Manager Exam Part I: Quantitative Analysis 00006613-00000002_CH01_p001-010.indd 10 26/07/22 8:36 PM Random Variables 2 Learning Objectives After completing this reading you should be able to: Describe and distinguish a probability mass function from Characterize the quantile function and quantile-based a cumulative distribution function, and explain the rela- estimators. tionship between these two. Explain the effect of a linear transformation of a random Understand and apply the concept of a mathematical variable on the mean, variance, standard deviation, skew- expectation of a random variable. ness, kurtosis, median, and interquartile range. Describe the four common population moments. Explain the differences between a probability mass func- tion and a probability density function. 11 00006613-00000002_CH02_p011-026.indd 11 26/07/22 8:36 PM Probabilities can be used to describe any situation with an Whereas a discrete random variable depends on a probability element of uncertainty. However, random variables restrict mass function, a continuous random variables depends on a prob- attention to uncertain phenomena that can be described with ability density function (PDF). However, this difference is of little numeric values. This restriction allows standard mathemati- consequence in practice, and the concepts introduced for dis- cal tools to be applied to the analysis of random phenomena. crete random variables all extend to continuous random variables. For example, the return on a portfolio of stocks is numeric, and a random variable can therefore be used to describe its uncertainty. The default on a corporate bond can be similarly 2.1 DEFINITION OF A RANDOM described using numeric values by assigning one to the event VARIABLE that the bond is in default and zero to the event that the bond is in good standing. The axioms of probability are general enough to describe This chapter begins by defining a random variable and relating many forms of randomness (e.g., a coin flip, a draw from a its definition to the concepts presented in the previous chapter. well-shuffled deck of cards, or a future return on the S&P 500). The initial focus is on discrete random variables, which take on However, directly applying probability can be difficult because distinct values. Note that most results from discrete random it is defined on events, which are abstract concepts. Random variables can be computed using only a weighted sum. variables limit attention to random phenomena which can be described using numeric values. This covers a wide range of Two functions are commonly used to describe the chance of applications relevant to risk management (e.g., describing asset observing various values from a random variable: the probability returns, measuring defaults, or quantifying uncertainty in param- mass function (PMF) and the cumulative distribution function eter estimates). The restriction that random variables are only (CDF). These functions are closely related, and each can be defined on numeric values simplifies the mathematical tools derived from the other. The PMF is particularly useful when defin- used to characterize their behavior. ing the expected value of a random variable, which is a weighted average that depends on both the outcomes of the random vari- A random variable is a function of v (i.e., an event in the sample able and the probabilities associated with each outcome. space Ω) that returns a number x. It is conventional to write a random variable using upper-case letters (e.g., X, Y, or Z) and to Moments are used to summarize the key features of random express the realization of that random variable with lower-case variables. A moment is the expected value of a carefully chosen letters (e.g., x, y, or z). It is important to distinguish between function designed to measure a characteristic of a random vari- these two: A random variable is a function, whereas a realization able. Four moments are commonly used in finance and risk man- is a number. The relationship between a realization and its corre- agement: the mean (which measures the average value of the sponding random variable can be succinctly expressed as: random variable), the variance (which measures the spread/dis- persion), the skewness (which measures asymmetry), and the x = X(v) (2.1) kurtosis (which measures the chance of observing a large devia- For example, let X be the random variable defined by the roll of tion from the mean).1 a fair die. Then we can denote x as the result of a single roll (i.e., Another set of important measures for random variables one event). The probability that the random variable is equal to includes those that depend on the quantile function, which is five can be expressed as: the inverse of the CDF. The quantile function, which can be used Pr(X = x) when x = 5 to map a random variable’s probability to its realization (i.e., the Univariate random variables are frequently distinguished based actual value that subsequently occurs), defines two moment-like on the range of values produced. The two classes of random vari- measures: the median (which measures the central tendency of ables are discrete and continuous. A discrete random variable is a random variable) and the interquartile range (which is an alter-