Big Data PDF
Document Details
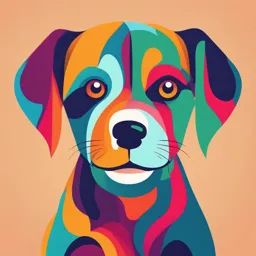
Uploaded by AngelicHelium
United Arab Emirates University
Tags
Summary
This document provides an overview of big data, including its definition, applications, advantages, and disadvantages. It covers topics such as structured and unstructured data, data size, the history of big data, and the motivations and importance of big data analysis.
Full Transcript
Big Dat C h a p t e r 6 Big Data Table Of Contents What is Big Data? 4 Structured Vs Unstructured data 6 Motivations & Importance 7 Data Size...
Big Dat C h a p t e r 6 Big Data Table Of Contents What is Big Data? 4 Structured Vs Unstructured data 6 Motivations & Importance 7 Data Size 8 History of Big Data 9 Statistics About Big Data (2022) 12 How Does Big Data Work? 14 How is Big Data Being Used? 15 Big Data System Architecture 15 Important Terminologies Related to Big Data 17 Characteristics of Big Data 18 Applications of Big Data 20 Areas Employing Big Data 24 Advantages of Big Data 26 Chapter 6 Page 1 Big Data Disadvantages of Big Data 27 Revision 29 Chapter 6 Page 2 Big Data What is Big Data? An evolving term that describes a large volume of data, structured, semi- structured and unstructured data that has the potential to be mined for information and used in machine learning projects and other advanced analytics applications. Unstructured data: Structured data: Doesn’t have any pre-defined Is clearly defined and searchable structure and comes in all its diversity types of data. It is commonly exists in of forms. tables similar to Excel files and Examples: image files, text files like PDF Google Docs spreadsheets. documents, video and audio files. Chapter 6 Page 3 Big Data Big data is: 1. an extremely large data sets that may be analyzed computationally to reveal patterns, trends, and associations, especially relating to human behavior and interactions. 2. a phrase used to mean a massive volume of both structured and unstructured data that is so large and difficult to process using traditional database and software techniques. 3. refers to the growth in the volume of structured and unstructured data, the speed at which it is created and collected, and the scope of how many data points are covered. Big Data often comes from multiple sources and arrives in multiple formats. 4. a blanket term for the non-traditional strategies and technologies needed to gather, organize, process, and gather insights from large datasets. Therefore, Big Data refers to: Big Datasets that are terabytes to petabytes (and even exabytes) in size, and the enormous sizes of these datasets exceeds the ability of normal database software methods to capture, store, manage, and analyze them effectively. Require tools other than the traditional ones to store, manage, analyze and visualize them in order to create actionable solutions to capable of influencing all aspects of our life. Chapter 6 Page 4 Big Data Structured Vs Unstructured data https ://lawtomated.com/s tructured-data-vs -uns tructured-data-what-are-they-and-why-care/ Chapter 6 Page 5 Big Data Motivations & Importance: Significant amount of useful knowledge is hidden in Big Data. Growing debate on how to maximize the benefits of it. Can help human uncover many life-related issues. Can reshape how people live, work, and communicate. Data volumes will continue to grow. Ways to analyze data will improve. Big Data will face challenges concerning privacy. More companies will appoint a Chief Data Officer (CDO). All companies are data businesses now. Businesses using data will see $430 billion in productivity benefits. Chapter 6 Page 6 Big Data Data Size: Different Sizes of Digital Data: One bit = 0 or 1 One Byte (B) = 8 bits Kilobyte (KB) = 1024 (210) B Megabyte (MB) = 1024 KB Gigabyte (GB) = 1024 MB Terabyte (TB) = 1024 GB Petabyte (PB) = 1024 TB Exabyte (EB) = 1024 PB Zettabyte (ZB) = 1024 BB Yottabyte (YB) = 1024 ZB https ://cloudtweaks.com/2015/03/how-much-data-is -produced-every-day/ Chapter 6 Page 7 Big Data History of Big Data The Foundation of Big Data: The earliest remembrance of modern data is from the 1881 when Herman Hollerith invented a computing machine that could read holes punched into paper cards in order to organize census data. The first major data project is created in 1937 and was ordered by the Franklin D. Roosevelt’s administration in the USA to keep track of contributions from 26 million Americans and more than 3 million employers. IBM got the contract to develop punch card-reading machine for this massive bookkeeping project. The first data-processing machine appeared in 1943 and was developed by the British to decipher/decode Nazi codes during World War II. This device, named Colossus, searched for patterns in intercepted messages at a rate of 5,000 characters per second. Thereby reducing the task from weeks to merely hours. In 1952 the National Security Agency (NSA) is created and within 10 years contract more than 12000 cryptologists. They are confronted with information overload during the Cold War as they start collecting and processing intelligence signals automatically. In 1965 the United States Government decided to build the first data center to store over 742 million tax returns and 175 million sets of fingerprints by transferring all those records onto magnetic computer tape that had to be stored in a single location. Chapter 6 Page 8 Big Data The Personal Computers and Internet Effect: Personal computers came on the market in 1977, and became a major stepping stone in the evolution of the internet, and subsequently, Big Data. As of the 90s the creation of data is spurred as more and more devices are connected to the internet. In 1995 the first super-computer is built, which was able to do as much work in a second than a calculator operated by a single person can do in 30,000 years. As more and more social networks start appearing and the Web 2.0 takes flight, more and more data is created on a daily basis. The term Big Data has been around 2005, when it was launched by O’Reilly Media. In 2010 Eric Schmidt speaks at the Techonomy conference in Lake Tahoe in California and he states that "there were 5 exabytes of information created by the entire world between the dawn of civilization and 2003. Now that same amount is created every two days.“ Chapter 6 Page 9 Big Data The Internet of Things (IoT): By 2013, the IoT had evolved to include multiple technologies, using the Internet, wireless communications, micro-electromechanical systems (MEMS), and embedded systems, GPS, and others. All of these transmit data about the person using them. Computing Power and Internet Growth: With the introduction of the term Big Data In 2005, which means a large set of data, at that time was almost impossible to manage and process using the traditional business intelligence tools available. Hadoop, which could handle Big Data, was created in 2005. Hadoop is an Open Source software framework, and can process structured and unstructured data, from almost all digital sources. In the past few years, there has been a massive increase in Big Data startups, all trying to deal with Big Data and helping organizations to understand Big Data and more and more companies are slowly adopting and moving towards Big Data. Chapter 6 Page 10 Big Data Statistics About Big Data (2022) Internet Users: Google processes roughly more than 48 PB of data every day. This includes more than 8.5 billion search queries per day. Whatsapp users exchange over 100 Billion messages daily. There are more than 2 billion active users for WhatsApp in a monthly basis. Facebook has a daily active user count of 1.93 billion from its 2.8 billion monthly active users and they generate a lot of data. Twitter has 192 million active users daily, with a growth rate of 27% within one year. An approximate number of 500 million tweets are received on Twitter per day. Big Data Growth: Data increases Exponentially. Chapter 6 Page 11 Big Data Digital data are there everywhere. Data proliferation is occurring continuously from different sources such as social media, sensors, mobile devices, cameras software logs, financial market data and individual archives. Nowadays, every day, we create roughly 2.5 quintillion bytes of data. With the growing popularity of IoT (Internet of Things), this data creation rate will become even greater. The influx of data provides both challenges and opportunities for adopters. Organizations that harness the potential of Big Data effectively, will no doubt beat their competitors. Google, Facebook, Amazon, Twitter, Microsoft and other top industry organizations are highly engaged in Big Data research and services. 97.2% of organizations are investing in Big Data and AI. Using Big Data, Netflix saves over $1 billion per year on customer retention. Chapter 6 Page 12 Big Data How Does Big Data Work? Big Data works on the principle that “the more you know about anything or any situation, the more reliably you can gain new insights and make predictions about what will happen in the future”. By comparing more data points, relationships begin to emerge that were previously hidden, and these relationships enable us to learn and make smarter decisions. Most commonly, this is done through a process that involves: Building models, based on the data we collect. Running simulations. Tweaking the values of data points each time Source: https ://www.bus ines s 2community.com and monitoring how it impacts our results. In most cases this process is completely automated – we have such advanced analytics tools that run millions of simulations to give us the best possible outcome. Chapter 6 Page 13 Big Data How is Big Data Being Used? This ever-growing stream of sensors information, photographs, text, voice and video data means we can now use data in ways that were not possible even a few years ago. Companies can now accurately predict what specific segments of customers will want to buy, and when, to an incredibly accurate degree. Big Data is also helping companies run their operations in a much more efficient way. This is revolutionizing the world of business across almost every industry. Big Data System Architecture Most Big Data architectures include some or all of the following components: Mostly, in Data Lake for Data is so large, it is divided to batches (groups) batch processing A way for storing data for real- time (stream) processing Real-time processing Data is prepared and stored in a format ready https ://www.oracle.com for analysis tools Chapter 6 Page 14 Big Data Model/Workflow/Framework of Big Data Analytics: https ://gacbe.ac.in The general categories of activities involved with Big Data processing are: 1. Ingesting data into the system. 2. Persisting the data in storage. 3. Analyzing data and computing insights. 4. Visualizing the results. Chapter 6 Page 15 Big Data Important Terminologies Related to Big Data Data Mining: Refers to deep dive into the data to extract the key knowledge / Patterns / Information from a small or large amount of data. Data Analytics: Is generally more focused than Big Data. Involves collecting data from different sources, preparing it in a way that it becomes available to be mined by analysts and finally deliver data products useful to the organization business. Analytics is gathered by means of software tools such as Hadoop and algorithms such as data mining, and predictive analytics models. Machine Learning: Is the field of study and practice of designing systems that can learn, adjust, and improve based on the data fed to them. This involves implementation of predictive and statistical algorithms that can continually zero in correct behavior and insights, as more data flows through the system. Chapter 6 Page 16 Big Data Characteristics of Big Data Big Data often characterized by what is became known as the 3Vs: Volume, Variety, and Velocity. These Vs are Challenges in deploying Big Data. Over time, other Vs have been added to characterize Big Data to be 6Vs, Veracity, Value, and Variability. Over time, they became 8Vs by adding Viscosity and Visualization. https ://s hreyans hmathur1998.medium.com/big-data-with-8vs -and-today-s -challenges - 9938f00363c5 Chapter 6 Page 17 Big Data Volume: The massive amount of data from so many sources Variety: The different types of data generated, unstructured, semi-structured, and structured data Velocity: The speed at which data is generated, collected and analyzed Veracity: Refers to how trustworthy data is. If the data is not accurate or of poor quality, it is of little use Value: The business value of data collected Variability: The ways in which the Big Data can be used and formatted Viscosity: Refers to the resistance when navigating through a data collection Visualization: It is difficult to use traditional graphs when trying to plot a billion data points, so we need different ways of representing data Visualization: Current big data visualization tools face technical challenges due to limitations of in-memory technology and poor scalability, functionality, and response time. We need different ways of representing data such as data clustering or using tree maps, sunbursts, parallel coordinates, circular network diagrams, or cone trees. Combine this with the multitude of variables resulting from Big Data's variety and velocity and the complex relationships between them, and you can see that developing a meaningful visualization is not easy. https ://www.finereport.com/en/data-vis ualization/data-vis ualization-2.html Chapter 6 Page 18 Big Data Applications of Big Data 1. Understanding and Targeting Customers: This is one of the biggest and most publicized areas of Big Data use today. Big Data is used to better understand customers and their behaviors and preferences. Companies are keen to expand their traditional datasets with social media data, browser logs as well as text analytics and sensor data to get a more complete picture of their customers. The main objective, in many cases, is to create predictive models. Example: Using Big Data, Wal-Mart can predict what products will sell; and car insurance companies understand how well their customers actually drive. 2. Understanding and Optimizing Business Processes: Big Data is also increasingly used to optimize business processes. Retailers are able to optimize their stock based on predictions generated from social media data, web search trends and weather forecasts. Example: One particular business process that is seeing a lot of Big Data analytics is supply chain or delivery route optimization. Geographic positioning and radio frequency identification (RFID) sensors are used to track goods or delivery vehicles and optimize routes by integrating live traffic data, etc. Chapter 6 Page 19 Big Data 3. Personal Quantification and Performance Optimization: Big data is not just for companies and governments but also for all of us individually. We can now benefit from the data generated from wearable devices such as smart watches or smart bracelets. Example: the UP band from Jawbone: The armband collects data on our calorie consumption, activity levels, and our sleep patterns. It gives individuals rich insights, the real value is in analyzing the collective data. 4. Improving Healthcare and Public Health: The computing power of Big Data analytics enables us to decode entire DNA strings in minutes and will allow us to find new cures and better understand and predict disease patterns. Just think of what happens when all the individual data from smart watches and wearable devices can be used to apply it to millions of people and their various diseases. The clinical trials of the future won't be limited by small sample sizes but could potentially include everyone! Chapter 6 Page 20 Big Data 5. Improving Sports Performance: Most elite sports have now embraced Big Data analytics. Example: the IBM Slam Tracker tool: Uses video analytics that track the performance of every player in a football or baseball game, and sensor technology in sports equipment such as basketballs or golf clubs that allows to get feedback (via smart phones and cloud servers) on the game and how to improve it. Many elite sports teams also track athletes outside of the sporting environment - using smart technology to track nutrition and sleep, as well as social media conversations to monitor emotional wellbeing. 6. Improving Science and Research: Science and research is currently being transformed by the new possibilities Big Data brings. Example: the CERN, the nuclear physics lab with its Large Hadron Collider, the world's largest and most powerful particle accelerator. Experiments to unlock the secrets of our universe - how it started and works - generate huge amounts of data. Chapter 6 Page 21 Big Data 7. Optimizing Machine and Device Performance: Big data analytics help machines and devices become smarter and more autonomous. Example: Big Data tools are used to operate Google's self-driving car. The car is fitted with cameras, GPS as well as powerful computers and sensors to safely drive on the road without the intervention of human beings. We can even use Big Data tools to optimize the performance of computers and data warehouses. 8. Improving Security and Law Enforcement: Big Data is applied heavily in improving security and enabling law enforcement. Big Data techniques can be used to detect and prevent cyber attacks. Police forces use Big Data tools to catch criminals and even predict criminal activity. Credit card companies use Big Data to detect fraud transactions. 9. Improving and Optimizing Cities and Countries: Big Data is used to improve many aspects of our cities and countries. Example: A number of cities are currently piloting Big Data analytics with the aim of turning themselves into Smart Cities, where the transport infrastructure and utility processes are all joined up. Where a bus would wait for a delayed train and where traffic signals predict traffic volumes and operate to minimize jams. Chapter 6 Page 22 Big Data 10. Financial Trading: High-Frequency Trading (HFT) is an area where Big Data finds a lot of use today. Here, Big Data algorithms are used to make trading decisions. Today, the majority of equity trading takes place via data algorithms that increasingly take into account signals from social media networks and news websites to make, buy and sell decisions in split seconds. Areas Employing Big Data In Education - Grading Systems: New advancements in grading systems have been introduced as a result of proper analysis of student data. In Healthcare: Big Data reduces costs of treatment since there is less chances of having to perform unnecessary diagnosis. In Government - Cyber Security: Big Data is hugely used for deceit recognition. Governments also use Big Data in catching tax evaders. In Media and Entertainment: Predicting the interests of audiences. Optimized or on-demand scheduling of media streams in digital media distribution platforms (Netflix). Chapter 6 Page 23 Big Data In Weather Patterns: To study global warming. Understanding the patterns of natural disasters In Transportation - Route Planning: Big Data can be used to understand and estimate the user’s needs on different routes and on multiple modes of transportation and then utilizing route planning to reduce the users wait times. Chapter 6 Page 24 Big Data Advantages of Big Data Cost Cutting: Big Data technologies such as Hadoop and other cloud-based analytics help significantly reduce costs when storing massive amounts of data. Increased Productivity: Modern Big Data tools like Hadoop and Spark are allowing analysts to analyze more data, more quickly, which increases their personal productivity so increases business productivity. Better Decision Making: Businesses are now able to analyze information instantly by the quick processing of Hadoop and in-memory analytics, added with the ability to analyze new sources of information, companies are able to take faster and better decisions. Fraud Detection: Big Data helps to automatically detect fraud attempts to hack into your organization and you will be instantly notified of a real-time safeguard system. Control Online Reputation: Big Data tools can help understand the company’s reputation through sentiment analysis. This gives you feedback about what people say about your company, which will allow you to improve your company’s online presence and reputation. Chapter 6 Page 25 Big Data Disadvantages of Big Data Chances of Failure: Many organizations may see other companies using Big Data, and its benefits being touted all over the internet as the best tool to grow one’s business. This may cause them to take hasty decisions and try to implement it immediately without understanding how to use it and whether it is suited to their business or not. Correlation Errors: A common technique used to analyze Big Data is to draw correlations by linking one variable to another to form a pattern. However, their correlations may not always stand for anything substantial or meaningful. In short, correlation does not always imply causation. Incompatible tools: Hadoop is the most commonly used tool for Big Data analytics. However, the standard version of Hadoop is not currently able to handle real-time data analysis. Chapter 6 Page 26 Big Data Security and Privacy Concerns: Data Privacy: The Big Data contains a lot of information about our personal lives, much of which we have a right to keep private. Increasingly, we are asked to strike a balance between the amount of personal data we distribute, and the convenience that Big Data-powered apps and services offer. Data Security: Even if we decide we are happy for someone to have our data for a particular purpose, can we trust them to keep it safe? Manipulation: Big Data can be used for manipulation of customer records. Data Discrimination: When everything is known, will it become acceptable to discriminate against people based on data we have on their lives? We already use credit scoring to decide who can borrow money, and insurance is heavily data-driven. We can expect to be analyzed and assessed in greater detail, and care must be taken that this isn’t done in a way that contributes to making life more difficult for those who already have fewer resources and access to information. Chapter 6 Page 27 Big Data Revision (By: Ms. Rola Abdallah) 1. Big data (BD): An evolving term that describes a large volume of structured, semi- structured, and unstructured data that has the potential to be mined for information and used in machine learning projects and other advanced analytics applications. Extremely large data sets may be analyzed computationally to reveal patterns, trends, and associations, especially relating to human behavior and interactions. A phrase used to mean a massive volume of both structured and unstructured data that is so large it is difficult to process using traditional database and software techniques. Big data refers to the growth in the volume of structured and unstructured data, the speed at which it is created and collected, and the scope of how many data points are covered. Big data often comes from multiple sources and arrives in multiple formats. Big dataset is terabytes to petabytes (and even exabytes) in size, and the enormous sizes of these datasets exceed the ability of normal database software methods to capture, store, manage, and analyze them effectively. It requires untraditional tools to generate, store, manage, analyze and visualize in order to create actionable solutions capable of influencing all aspects of our life. 2. Data mining: Refers to a deep drive into the data to extract the key knowledge / Pattern / Information from a small or large amount of data. 3. Data analytics: Data analytics is generally more focused than big data because instead of gathering huge piles of unstructured data, data analysts have a specific goal in mind and sort through relevant data to look for. Note: this s heet is only for revis ion purpos es. It may not include all material you need for your exams. Chapter 6 Page 28 Big Data 4. Hadoop: An open-source distributed processing framework that manages data processing and storage for big data applications running in clustered systems. It is the most commonly used tool for Big Data analytics. 5. Machine learning: The study and practice of designing systems that can learn, adjust, and improve based on the data fed to them. This typically involves the implementation of predictive and statistical algorithms that can continually zero in on "correct" behavior and insights as more data flows through the system. 6. BD characteristics: 7. Visualization types: a. Visualization a. Data clustering b. Velocity b. Treemaps c. Variety d. Volume c. Sunbursts e. Variability d. Parallel coordinates f. Viscosity e. Circular network diagrams g. Value f. Cone trees h. Veracity 8. Big data processing categories: a. Ingesting data into the system b. Persisting the data in storage c. Computing and Analyzing data d. Visualizing the results Note: this s heet is only for revis ion purpos es. It may not include all material you need for your exams. Chapter 6 Page 29 Big Data 9. BD applications: a. Education industry b. Healthcare industry c. Government industry- Cyber security d. Media and Entertainment industry e. Weather patterns f. Transportation Industry- Route planning 10. BD advantages: a. Cost cutting b. Increased productivity c. Better decision making d. Fraud detection e. Control online reputation 11. BD disadvantages: a. Incompatible tools b. Chances of failure c. Correlation errors d. Security and privacy concerns e. Data privacy f. Data security g. Data discrimination h. Manipulation Note: this s heet is only for revis ion purpos es. It may not include all material you need for your exams. Chapter 6 Page 30 Big Data Fourth Industrial Revolution Course Materials Reference Book: Schwab, K. with Davis, N. (2017). The Fourth Industrial Revolution. Currency. Publisher: Currency (2017) ISBN: 9781524758868 Course Materials Preparation: Lecture notes, videos, class discussions, student activities, case studies, and project guidelines for the Fourth Industrial Revolution course were prepared and edited by: Dr. Khaled Hamdan ([email protected]) Dr. Nabeel Al-Qirim ([email protected]). PDF file Content Contributor: Dr. Asmaa Hosni ([email protected]) Reviewed by: Mr. Marwan Fayyad ([email protected]) Graphic Designed by: Basheir Al-Rei ([email protected]) Klaus Schwab (2017). The Fourth Industrial Revolution. Publisher: Currency (January 3, 2017), ISBN-10: 9781524758868; ISBN-13: 978-1524758868; ASIN: 1524758868 Mitchell, L., & Groenewald, G. (2010). The pre-industrial cape in the twenty-first century. South African Historical Journal, 62(3), 435–443. https://docs.microsoft.com/en-us/azure/architecture/data-guide/big-data/ https://ischoolonline.berkeley.edu/data-science/what-is-data-science/ https://www.youtube.com/watch?v=iqxFL1Png https://www.cio.com/article/3292983/what-is-a- data-engineer.html https://www.sdxcentral.com/industry/career/skills/big-data-engineer- skills/ https://gcet.edu.om/en/programmes/msc-data-science/ https://www.digitalvidya.com/blog/big-data-applications/ https://www.geeksforgeeks.org/applications-of-big-data/ https://www.datamation.com/big- data/big-data-pros-and-cons/ https://honestproscons.com/pros-and-cons-of-big-data/ https://www.itransition.com/blog/the-future-of-big-data https://ritzherald.com/the-future-of-