Introduction to Business Analytics BADM3400 Lecture Notes PDF
Document Details
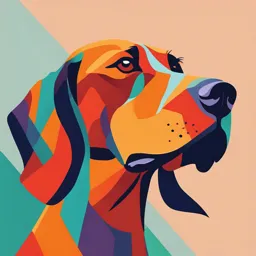
Uploaded by BalancedLion
Jason Chan, PhD
Tags
Related
Summary
This document is a lecture on trend lines and regression analysis. It covers different types of trend lines, mathematical functions for predictive models, using Microsoft Excel, and analysis of variance in regression models. Topics include least squares regression and residuals to illustrate different examples. It also includes an interpretation of business data for regression analysis.
Full Transcript
Introduction to Business Analytics BADM3400 Lecture Trend lines and Regression Analysis Chapter 8 Jason Chan, PhD 1 Modeling Relationships and Trends in Data Create charts to bet...
Introduction to Business Analytics BADM3400 Lecture Trend lines and Regression Analysis Chapter 8 Jason Chan, PhD 1 Modeling Relationships and Trends in Data Create charts to better understand data sets. For cross-sectional data, use a scatter chart. For time series data, use a line chart. 2 Common Mathematical Functions Used for Predictive Analytical Models 3 Excel Trend line Tool Right click on data series and choose Add trend line from pop-up menu. Check the boxes Display Equation on chart and Display R-squared value on chart. 4 R-Squared (R 2 ) R2 is a measure of the “fit” of the line to the data The value of R2 will be between 0 and 1 A value of 1.0 indicates a perfect fit and all data points would be lie on the line; the larger the value of R2 the better the fit 5 Example 1: Modeling a Price-Demand Function Linear demand function: Sales = 20512 – 95116(price) 6 Example 2: Predicting Crude Oil Prices Line chart of historical crude oil prices 7 Example 2: Predicting Crude Oil Prices 8 Example 2: Predicting Crude Oil Prices Third order polynomial trend line fit to the data 9 Caution About Polynomials The R2 value will continue to increase as the order of the polynomial increases; that is, a 4th order polynomial will provide a better fit than a 3rd order, and so on. Higher order polynomials will generally not be very smooth and will be difficult to interpret visually. –Thus, we don’t recommend going beyond a third-order polynomial when fitting data. Use your eye to make a good judgment! 10 Regression Analysis Regression analysis is a tool for building mathematical and statistical models that characterize relationships between a dependent (ratio) variable and one or more independent, or explanatory variables (ratio or categorical), all of which are numerical. Simple linear regression involves a single independent variable. Multiple regression involves two or more independent variables. 11 Simple Linear Regression Finds a linear relationship between: one independent variable X and one dependent variable Y First prepare a scatter chart to verify the data has a linear trend. Use alternative approaches if the data is not linear. 12 Example 3: Home Market Value Data Size of a house is typically related to its market value. X = square footage , Y = market value ($) 13 Example 3: Home Market Value Data The scatter chart of the full data set (42 homes) indicates a linear trend. 14 Finding the Best-Fitting Regression Line Market value = a + b x where x is square feet Two possible lines are shown below. Line A is clearly a better fit to the data. We want to determine the best regression line. 15 Example4: Using Excel to Find the Best Regression Line 16 Example4: Using Excel to Find the Best Regression Line The regression model explains variation in market value due to size of the home. It provides better estimates of market value than simply using the average. 17 Least-Squares Regression 18 Residuals 19 Least Squares Regression 20 Example 5: Using Excel Functions to Find Least-Squares Coefficients 21 Example 5: Using Excel Functions to Find Least-Squares Coefficients 22 Simple Linear Regression with Excel 23 Home Market Value Regression Results 24 Regression Statistics 25 Formula 26 Example 6: Interpreting Regression Statistics for Simple Linear Regression 27 Regression as Analysis of Variance 28 Example 7: Interpreting Significance of Regression 29 Example 7: Interpreting Significance of Regression 30 Testing Hypotheses for Regression Coefficients 31 Testing Hypotheses for Regression Coefficients 32 Example 8: Interpreting Hypothesis Tests for Regression Coefficients 33 Confidence Intervals for Regression Coefficients 34 Example 9: Interpreting Confidence Intervals for Regression Coefficients 35 Residual Analysis and Regression Assumptions 36 Example 10: Interpreting Residual Output 37 Example 10: Interpreting Residual Output 38 Checking Assumptions Linearity – examine scatter diagram (should appear linear) – examine residual plot (should appear random) Normality of Errors – view a histogram of standard residuals – regression is robust to departures from normality Homoscedasticity: variation about the regression line is constant. – examine the residual plot Independence of Errors: successive observations should not be related. – This is important when the independent variable is time. 39 Example 11: Checking Regression Assumptions for the Home Market Value Data Linearity - linear trend in scatterplot no pattern in residual plot 40 Example 11: Checking Regression Assumptions for the Home Market Value Data Normality of Errors - residual histogram appears slightly skewed but is not a serious departure. 41 Example 11: Checking Regression Assumptions for the Home Market Value Data Homoscedasticity - residual plot shows no serious difference in the spread of the data for different X values. 42 Example 11: Checking Regression Assumptions for the Home Market Value Data Independence of Errors - Because the data is cross- sectional, we can assume this assumption holds. 43 Multiple Linear Regression 44 Estimated Multiple Regression Equation 45 Excel Regression Tool 46 ANOVA for Multiple Regression 47 Example 12: Interpreting Regression Results for the Colleges and Universities Data Predict student graduation rates using several indicators 48 Example 12: Interpreting Regression Results for the Colleges and Universities Data 49 Example 12: Interpreting Regression Results for the Colleges and Universities Data The value of R-square indicates that 53% of the variation in the dependent variable is explained by these independent variables All coefficients are statistically significant 50 Building Good Regression Models A good regression model should include only significant independent variables. However, it is not always clear exactly what will happen when we add or remove variables from a model; variables that are (or are not) significant in one model may (or may not) be significant in another. – Therefore, you should not consider dropping all insignificant variables at one time, but rather take a more structured approach. 51 Systematic Model Building Approach 1. Construct a model with all available independent variables. Check for significance of the independent variables by examining the p-values. 2. Identify the independent variable having the largest p-value that exceeds the chosen level of significance. 3. Remove the variable identified in step 2 from the model and evaluate adjusted R2 Don’t remove all variables with p-values that exceed a at the same time, but remove only one at a time.) 4. Continue until all variables are significant. 52 Example 13: Identifying the Best Regression Model Banking Data 53 Example 13: Identifying the Best Regression Model Home value has the largest p-value; drop and re-run the regression. 54 Example 13: Identifying the Best Regression Model Regression output after removing Home Value 55 Multicollinearity Multicollinearity occurs when there are strong correlations among the independent variables, and they can predict each other better than the dependent variable. – When significant multicollinearity is present, it becomes difficult to isolate the effect of one independent variable on the dependent variable, the signs of coefficients may be the opposite of what they should be, making it difficult to interpret regression coefficients, and p-values can be inflated. 56