Unit XII Design and Analysis of Single-Factor Experiments PDF
Document Details
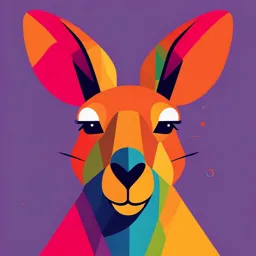
Uploaded by AuthoritativeParabola
Central Mindanao University
Tags
Summary
This document provides an introduction to single-factor experiments and analysis of variance (ANOVA). It covers design principles, explains how ANOVA is used to draw conclusions from experiments, and discusses the significance of randomization. The document also touches upon model adequacy and the difference between fixed and random factors.
Full Transcript
nit XII U ESIGN AND ANALYSIS OF SINGLE-FACTOR EXPERIMENTS: THE D ANALYSIS OF VARIANCE INTRODUCTION Experiments are a natural part of the engineering and scientific ec...
nit XII U ESIGN AND ANALYSIS OF SINGLE-FACTOR EXPERIMENTS: THE D ANALYSIS OF VARIANCE INTRODUCTION Experiments are a natural part of the engineering and scientific ecision-makingprocesses.Theseexperimentsconsistofdifferenttreatments d to gather data for testing. In comparing experiments with two factors, the hypothesis tests for thetwosamplesintroducedinthepreviouschaptersare sufficient. However, many experiments involved more than two levels of factors. Thus, theprimaryfocusofthisunitistheuseofanalysisofvariance (ANOVA) in determining differences between multiple levels of factors and populations,theanalysisofsingle-factorexperiments,andthesignificanceof randomizing experimental runs. OBJECTIVES At the end of this unit, the student must be able to: 1. Design and conduct analysis on engineering single factor experiments; 2. Understand how the analysis of variance is used to conclude the experiments; 3. Use multiple comparison procedures and assess model adequacy with residual plots; 4. Understand the difference between fixed and random factors; 5. Conduct experiments involving the randomized complete block design; 6. Apply the learnings in order to solve problems and draw conclusions in real-world scenarios. CONTENTS A. Completely Randomized Single Factor Experiments a. Analysis of Variance (ANOVA) Analysis of Variance (ANOVA) is a statistical method sed to analyze experimental data to compare and determine u differences between multiple population means. It involves partitioning components observed in data with a mathematical modeltoexplainhowdifferentfactorsinfluenceanexperiment's outcome. Statistically represented as: 𝑌𝑖𝑗 = µ + τ𝑖 + ϵ𝑖𝑗 { 𝑖=1 } ,2,...,𝑎 𝑗=1 ,2,...,𝑛 Engineering Data Analysis Page1 Where; 𝑌𝑖𝑗 - is a random variable denoting the (𝑖𝑗)th bservation o µ - is the overall mean of observations τ𝑖 - is the𝑖th treatment effect ϵ𝑖𝑗 - is a random error component oreover, ANOVA testshypotheses.Thenullhypothesis M states that all observation means are equal and there is no significant difference among the treatmentmeans,whereasthe alternativehypothesisstatesthatthereisasignificantdifference amongthe treatment means.Specifically, 𝐻0: τ1 = τ2 =···= τ𝑎 = 0 𝐻1: τ𝑖 ≠ 0 In order to test the hypothesis, the total variability in a sample data is subdivided into two parts describedbythetotal sum of squares 𝑎 𝑛 2 𝑆𝑆𝑇 = ∑ ∑ (𝑦𝑖𝑗 − 𝑦 ) 𝑖=1𝑗= 1 r simply obtaining the squares of the differences betweenthe o observed single data and the grand mean for all observations and treatments. The preceding equation can be partitioned according to its identity 𝑎 𝑛 2 𝑎 2 𝑎 𝑛 2 ∑ ∑ (𝑦𝑖𝑗 − 𝑦 ) = 𝑛 ∑ (𝑦𝑖 − 𝑦 ) = ∑ ∑ (𝑦𝑖𝑗 − 𝑦𝑖 ) 𝑖=1𝑗= 1 𝑖=1 𝑖= 1𝑗=1 or symbolically 𝑆𝑆𝑇 = 𝑆𝑆𝑇𝑟𝑒𝑎𝑡𝑚𝑒𝑛𝑡𝑠 + 𝑆𝑆𝐸 where 𝑆𝑆𝑇𝑟𝑒𝑎𝑡𝑚𝑒𝑛𝑡𝑠 denote the sum of squares of differences betweentreatmentmeansandthegrandmeanand𝑆𝑆𝐸 denoting the sum of squares of differences of observations within a treatment from the treatment mean. Additionally, the expected valuesof 𝑆𝑆𝑇𝑟𝑒𝑎𝑡𝑚𝑒𝑛𝑡𝑠 and 𝑆𝑆𝐸 willalsobeconsideredtobeused for the test statistic. Engineering Data Analysis Page2 The expected value of the treatment sum of squares is 𝑎 2 2 𝐸(𝑆𝑆𝑇𝑟𝑒𝑎𝑡𝑚𝑒𝑛𝑡𝑠) = (𝑎 − 1)σ + 𝑛 ∑ τ𝑖 𝑖=1 and the expected value of the error sum of squares is 2 𝐸(𝑆𝑆𝐸) = 𝑎(𝑛 − 1)σ ccordingly, the equivalent degrees of freedom for the A sum of squares identity is expressed as 𝑎𝑛 − 1 = 𝑎 − 1 + 𝑎(𝑛 − 1) Thisfollowsthatthemeansquarefortreatmentsisgiven as 𝑀𝑆𝑇𝑟𝑒𝑎𝑡𝑚𝑒𝑛𝑡𝑠 = 𝑆𝑆𝑇𝑟𝑒𝑎𝑡𝑚𝑒𝑛𝑡𝑠/(𝑎 − 1) and the error mean square as 𝑀𝑆𝐸 = 𝑆𝑆𝐸/ [𝑎(𝑛 − 1)] 2 hichareunbiasedestimatorsofσ ifthenullhypothesisistrue. w Then, the ratio of the mean squares follows the 𝐹-distribution with𝑎 − 1 and𝑎(𝑛 − 1) degrees of freedom. That is, 𝑆𝑆𝑇𝑟𝑒𝑎𝑡𝑚𝑒𝑛𝑡𝑠/( 𝑎−1) 𝑀𝑆𝑇𝑟𝑒𝑎𝑡𝑚𝑒𝑛𝑡𝑠 𝐹0 = 𝑆𝑆𝐸/[ 𝑎(𝑛−1)] = 𝑀𝑆𝐸 The only condition that the null hypothesis can be rejected is where the expected value of 𝑀𝑆𝑇𝑟𝑒𝑎𝑡𝑚𝑒𝑛𝑡𝑠 is greater than the expected value of 𝑀𝑆𝐸,implyinganupper-tail,one-tail critical region. Reject 𝐻0 if 𝑓0 > 𝑓α,𝑎−1,𝑎(𝑛− 1) , where 𝑓0 is the computed value from the test statistic. For equal sample sizes, 𝑆𝑆𝑇𝑟𝑒𝑎𝑡𝑚𝑒𝑛𝑡𝑠 and 𝑆𝑆𝑇 can be reduced into 𝑎 𝑛 2 𝑦 2 𝑆𝑆𝑇 = ∑ ∑ 𝑦𝑖𝑗 − 𝑁 𝑖=1𝑗= 1 and Engineering Data Analysis Page3 𝑎 𝑦2 2 𝑦 𝑖 𝑆𝑆𝑇𝑟𝑒𝑎𝑡𝑚𝑒𝑛𝑡𝑠 = ∑ 𝑛 − 𝑁 𝑖=1 The error sum of squares can then be obtained as the ifference between the total sum of squares and thetreatment d sum of squares 𝑆𝑆𝐸 = 𝑆𝑆𝑇 − 𝑆𝑆𝑇𝑟𝑒𝑎𝑡𝑚𝑒𝑛𝑡𝑠 The summary of this test procedure, called the ANOVA table, is provided in tabular form below: ource of S Sum of D egrees of Mean 𝐹0 Variation Squares F reedom Square Treatments 𝑆𝑆 𝑎 − 1 𝑀𝑆𝑇𝑟𝑒𝑎𝑡𝑚𝑒𝑛𝑡𝑠 𝑀𝑆𝑇𝑟𝑒𝑎𝑡𝑚𝑒𝑛𝑡𝑠 𝑇𝑟𝑒𝑎𝑡𝑚𝑒𝑛𝑡𝑠 𝑀𝑆𝐸 Error 𝑆𝑆𝐸 𝑎(𝑛 − 1) 𝑀𝑆𝐸 Total 𝑆𝑆𝑇 𝑎𝑛 − 1 Furthermore, several software applications analyze data singtheanalysisofvariance.ThisincludestheMinitabOutput, u which results in the following confidence interval definition: The confidence interval (CI) on the mean ofatreatment provides a range within which we expect the true mean of the treatment to fall. This is calculated as: 𝑀𝑆𝐸 𝑀𝑆𝐸 γ𝑖 − 𝑡α/2,𝑎(𝑛− 1) 𝑛 ≤ µ𝑖 ≤ γ𝑖 + 𝑡α/2,𝑎(𝑛− 1) 𝑛 Also a 100(1 − 𝑎) percent confidence interval on the difference in two treatment meansµ𝑖 − µ𝑗 is 2𝑀 𝑆𝐸 2𝑀𝑆𝐸 𝑌𝑖· − 𝑌𝑗· − 𝑡α/2,𝑎(𝑛−1) 𝑛 ≤ µ𝑖 − µ𝑗 ≤ 𝑌𝑖· − 𝑌𝑗· + 𝑡α/2,𝑎( 𝑛− 1) 𝑛 Additionally, in single-factor experiments, an unbalanced esign occurs when the number of observations per treatment d varies. Adjustments to the sums of squares formulas are Engineering Data Analysis Page4 needed. If 𝑛𝑖 represents thenumberofobservationsforthei-th 𝑎 treatment, the total number of observations,𝑁, is𝑁 = ∑ 𝑛𝑖. 𝑖=1 he sums of squares computing formulas for the ANOVA with T unequal sample sizes𝑛𝑖 in each treatment are: 𝑛𝑖 2 2 𝑦.. 1. 𝑆𝑆𝑇 = 𝑐 ∑ 𝑦𝑖𝑗 − 𝑁 𝑗=1 𝑎 2 𝑦𝑖. 2 𝑦.. 2. 𝑆𝑆𝑇𝑟𝑒𝑎𝑡𝑚𝑒𝑛𝑡𝑠 = ∑ 𝑛 − 𝑁 𝑖= 1 𝑖 3. 𝑆𝑆𝐸 = 𝑆𝑆𝑇 − 𝑆𝑆𝑇𝑟𝑒𝑎𝑡𝑚𝑒𝑛𝑡𝑠 hoosing a balanced design offers two keyadvantages. C First, the ANOVA is relatively insensitive to small departures from the assumption of equality of variances when the sample sizesareequal.Thisrobustnessisnotmaintainedwithunequal samplesizes.Second,thepowerofthetestismaximizedwhen the samples are of equal size, ensuring more reliable and effective results. b. Multiple Comparison following the ANOVA heAnalysisofVariance(ANOVA)isastatisticalformula T that is used to compare differences of means among different groups. However, theANOVAdoesn’tidentifywhichmeansare different. That is why multiple comparisonmethodisusedto investigate whether there are differences in population means among the different populations. TheFisher’sleastsignificantdifference(LSD)methodfor multiple comparisons is used in ANOVA to build on the equal variances t-test of the difference between the two means.This comparesallpairsofmeanswiththenullhypotheses𝐻0: µ𝑖 = µ𝑗 (for all𝑖 ≠ 𝑗) using the t-statistic formula 𝑦𝑖·−𝑦𝑗 · 𝑡0 = 2𝑀𝑆𝐸 𝑛 Where; 𝑡0 - is the t-statistic 𝑦𝑖· - is the treatment mean 1 𝑦𝑗· - is the treatment mean 2 𝑀𝑆𝐸 - is the mean squared error Engineering Data Analysis Page5 𝑛 - is the sample sizes If it’s a two-sided alternative hypothesis, the pair means µ𝑖 andµ𝑗 would be declare significantlydifferent if ||𝑦 − 𝑦 | | 𝑖· 𝑗·|| > 𝐿 𝑆𝐷 Where LSD (least significant difference) is 2𝑀𝑆𝐸 𝐿 𝑆𝐷 = 𝑡α/2,𝑎(𝑛− 1) 𝑛 However, if the sample sizes are different in each treatment, then the LSD is defined as 𝐿 𝑆𝐷 = 𝑡α/2,𝑁−𝑎 𝑀𝑆𝐸 ( 1 𝑖 𝑛 + 1 𝑗 𝑛 ) c. Residual Analysis and Model Checking In the analysis of variance (ANOVA), it is assumed that bservationsarenormallyandindependentlydistributedwiththe o same variance across all treatment or factor levels. These assumptions can be verified by examining the residuals,which are the differences between observed values (𝑦 𝑖𝑗) and their estimatedvalues(ŷ𝑖𝑗)fromthestatisticalmodel.Inacompletely randomizeddesign, ŷ𝑖𝑗 isthemeanoftheobservationsforeach treatment (𝑦𝑖) , and each residual (𝑒𝑖𝑗) is calculated as 𝑒𝑖𝑗 = 𝑦𝑖𝑗 − 𝑦𝑖· Thenormalityassumptioncanbecheckedusinganormal robability plot of the residuals; a straight line in this plot p suggestsnormality.Tochecktheassumptionofequalvariances, residuals are plotted againstthefactorlevelsandthespreadis compared. Plotting residuals against the fitted values (𝑦𝑖) also elpsverifythatresidualvariabilitydoesnotdependonthefitted h values. If a pattern appears, it might indicate the need for a transformation, such as taking the logarithm or square root of the data, to stabilize variance. Engineering Data Analysis Page6 dditionally, the independence of observations can be A assessed by plotting residuals against the time or run order of the experiment. A pattern in this plot may suggest that timeor run order affects the results, indicating that time-dependent variables need to be included in the experimental design. d. Determining Sample Size electing the appropriate sample size or number of S replicates in any experimental design problem is crucial. In making this selection, operating characteristics curves (OC curves) can be used to provide guidance. The OCcurveplots thechanceofatypeIIerror(β )fordifferentsamplesizesversus the critical difference in means to detect. OC curves can be utilized to determine howmanyreplicatesareneededtoobtain sufficient sensitivity. The power of the ANOVA test is { 1 − β = 𝑃 𝑅𝑒𝑗𝑒𝑐𝑡𝐻0 |𝐻0 𝑖𝑠𝑓𝑎𝑙𝑠𝑒 } = 𝑃{𝐹0 > 𝑓α,𝑎−1,𝑎(𝑛−1) |𝐻0 𝑖𝑠𝑓𝑎𝑙𝑠𝑒} If the null hypothesis is false, then weneedtoknowthe distribution of the test statistic 𝐹0 in order to evaluate this robabilitystatement.Thisisbecausethenullhypothesiscanbe p false in different ways, especially that the ANOVA compares different means. The OC curves plot β against the parameterϕ, where 𝑎 2 𝑛∑ τ𝑖 2 2 2 𝑛δ ϕ = 𝑖= 1 2 orΦ = 2 𝑎σ 2𝑎 σ 2 he parameter ϕ is the noncentrality parameter δ. T Curvesareavailablefor α = 0. 05 andα = 0. 01 andforseveral values of the number of degrees of freedom for numerator (denoted𝑣1) and denominator (denoted𝑣2). B. The Random Effects Model Differentiating between fixed and random factors is crucial in singlefactorexperimentdesignandanalysisbecauseitaffectshowthe results are interpreted and generalized. a. Fixed vs. Random Factors Engineering Data Analysis Page7 fixed factor is one whose levels are carefully set and A are ofprimaryinterest.Whenanalyzingdatafromafixed-factor experiment,anANOVA(analysisofvariance)istypicallyusedto assess if the means of the levels differ substantially. Results can't be generalized beyond the levels tested and are only applicable to those levels covered in the experiment. Arandomfactor,ontheotherhand,isafactorwithlevels chosen at random from a broader set of potential levels. The levels are considered a random sample from a broader category. When dealing with random factors, the focus is on estimatingthevariabilityamongthelevelsratherthancomparing specific levels. b. ANOVA and Variance Components ANOVA allowsyoutosimultaneouslycomparearithmetic eans across groups. You can determine whether the m differencesobservedareduetorandomchanceoriftheyreflect genuine, meaningful differences. On the same hand, the Variance Components Analysis procedure is designed to estimatethecontributionofmultiplefactorstothevariabilityofa dependent variable Y.Theanalysisofvarianceistheextension of the t-test for independent samples to more than two groups. Since we deal with continuous response variables as a function of one or more predictor variables, we use the linear statistical model. The model is identical in structure to the fixed-effectscase,buttheparametersareinterpreteddifferently, with the treatment effects τ𝑖 and the errors ϵ𝑖𝑗 as independent random variables. 2 If the variance of the treatment effects τ𝑖 is στ , by independence the variance of the response is 2 2 ( ) 𝑉 𝑌𝑖𝑗 = στ + σ 2 2 Thevariances στ a nd σ arecalledvariancecomponents, nd the model is called the components of thevariancemodel a or the random-effects model. Fortherandom-effectsmodel,testingthehypothesisthat the individual treatment effects are zero is meaningless. It is 2 more appropriate to test hypotheses aboutστ . Specifically, 2 𝐻0: στ = 0 2 𝐻1: στ > 0 Engineering Data Analysis Page8 2 2 If στ = 0, alltreatmentsareidentical;butifστ > 0, there is variabilitybetweentreatments.TheANOVAdecompositionof total variability is still valid. Furthermore,𝑀𝑆𝐸 and𝑀𝑆𝑇𝑟𝑒𝑎𝑡𝑚𝑒𝑛𝑡𝑠 are independent. Consequently, the ratio ( 𝐹0 = 𝐸 𝑀𝑆𝑇𝑟𝑒𝑎𝑡𝑚𝑒𝑛𝑡𝑠 𝑀𝑆𝐸 ) is an F random variable with𝑎 − 1 and𝑎(𝑛 − 1) degrees of freedom when𝐻0 is true. The nullhypothesis would e rejected at theα - level of significance ifthe computed value b of the test statistic𝑓0 > 𝑓α,𝑎−1,𝑎(𝑛−1) . quating Observed and Expected Mean Squares in the E One-way Classification Random-Effects Model: 2 2 2 𝑀𝑆𝑇𝑟𝑒𝑎𝑡𝑚𝑒𝑛𝑡𝑠 = σ + 𝑛στ and 𝑀𝑆𝐸 = σ Variance Components Estimates 2 𝑀𝑆𝑇𝑟𝑒𝑎𝑡𝑚𝑒𝑛𝑡𝑠−𝑀𝑆𝐸 2 στ = 𝑛 and σ = 𝑀𝑆𝐸 The only condition that the null hypothesis can be rejected is where the expected value of 𝑀𝑆𝑇𝑟𝑒𝑎𝑡𝑚𝑒𝑛𝑡𝑠 is greater than the expected value of 𝑀𝑆𝐸,implyinganupper-tail,one-tail critical region. Reject 𝐻0 if 𝑓0 > 𝑓α,𝑎−1,𝑎(𝑛− 1) , where 𝑓0 is the computed value from the test statistic. C. Randomized Complete Block Design a. Design and Statistical Analysis Experimentaldesignisastructuredapproachtoplanning and conducting experiments with the goal of obtaining valid, reliable,andinterpretableresults.Itinvolvescarefullycontrolling and manipulating variables to determine their effects while minimizing the impact of confounding factors. It helps ensure that the results of an experiment are reliable and can be attributedtothefactorsbeingtested,ratherthanotherunrelated variables. Then a statistical analysis involves the process of collecting, analyzing, interpreting, presenting, and organizing Engineering Data Analysis Page9 ata. It uses mathematical theories and formulas to derive d insights and make decisions based on data. Moreover, a randomized block design is anextensionof the paired t-test to situations where the factor of interest has morethantwolevels;thatis,morethantwotreatmentsmustbe compared. The observations for randomized complete block design may be represented by the linear statistical model 𝑌𝑖𝑗 =µ + τ𝑖 + β𝑗 +ϵ𝑖𝑗 { 𝑖=1 } ,2,...,𝑎 𝑗=1 ,2,...,𝑏 where µ an overall mean, τ𝑖 is the effect of the th treatment, β𝑗 istheeffectoftheth block,and ϵ𝑖𝑗istherandom error term, which isassumedtobenormallyandindependently 2 distributed with mean zero and varianceσ . In a randomized block experiment, it uses a sum of squares identity that partitions the total sum of squares into three components 𝑏 𝑎 2 𝑏 2 ( ) ( ∑ 𝑦𝑖𝑗 − 𝑦.. = 𝑏 ∑ 𝑦𝑖 − 𝑦.. 𝑗=1 𝑖=1 ) ( + 𝑎 ∑ 𝑦𝑗 − 𝑦.. 𝑗=1 ) 𝑎 𝑏 2 + ∑ ∑ 𝑖=1𝑗=1 ( 𝑦𝑖𝑗 − 𝑦𝑗 − 𝑦𝑖 − 𝑦.. ) symbolically : 𝑆𝑆𝑇 =𝑆𝑆𝑇𝑟𝑒𝑎𝑡𝑚𝑒𝑛𝑡𝑠 +𝑆𝑆𝐵𝑙𝑜𝑐𝑘𝑠 +𝑆𝑆𝐸 Where the degrees of freedom corresponding to the sums of squares are ab - 1 = ( a - 1) + ( b - 1) + ( a - 1)( b - 1) his follows the relevant mean square for a randomized T block design: 𝑆𝑆𝑇𝑟𝑒𝑎𝑡𝑚𝑒𝑛𝑡𝑠 𝑀𝑆𝑇𝑟𝑒𝑎𝑡𝑚𝑒𝑛𝑡𝑠 = 𝑎−1 𝑆𝑆𝐵𝑙𝑜𝑐𝑘𝑠 𝑀𝑆𝐵𝑙𝑜𝑐𝑘𝑠 = 𝑏−1 𝑆𝑆𝐸 𝑀𝑆𝐸 = (𝑎−1) (𝑏−1) Engineering Data Analysis Page10 lso, the expected values of this mean squares are A shown as 𝑎 2 𝑏 ∑ τ𝑖 2 𝐸(𝑀𝑆𝑇𝑟𝑒𝑎𝑡𝑚𝑒𝑛𝑡𝑠) = σ + 𝑖= 𝑎− 1 1 𝑎 2 𝑏 ∑ β𝑗 2 + 𝐸(𝑀𝑆𝐵𝑙𝑜𝑐𝑘𝑠) = σ 𝑗= 𝑏− 1 1 2 𝐸(𝑀𝑆𝐵𝑙𝑜𝑐𝑘𝑠) = σ To test the null hypothesis that the treatmenteffectsare 𝑀𝑆𝑇𝑟𝑒𝑎𝑡𝑚𝑒𝑛𝑡𝑠 all zero, we use the ratio 𝐹0 = 𝑀𝑆𝐸 which has an -distributionwitha-1and(a 1)(b 1)degreesoffreedomifthe F null hypothesis is true. We would reject the null hypothesis at the αlevel of significance if the computed value of the test statistic in equation is𝑓0 = 𝑓α,𝑎 −1,(𝑎− 1 )(𝑎−𝑏) . Additionally, the appropriate computing formulas of s quares in the analysis of variance for a randomized block experiment are: 𝑎 𝑏 2 𝑦.. 2 𝑆𝑆𝑇 = ∑ ∑ 𝑦𝑖𝑗 − 𝑎𝑏 𝑖=1𝑗 =1 𝑎 2 𝑦 1 2 𝑆𝑆𝑇𝑟𝑒𝑎𝑡𝑚𝑒𝑛𝑡𝑠 = 𝑏 ∑ 𝑦𝑖. - 𝑎.𝑏. 𝑖=1 𝑎 2 𝑦.. 1 2 𝑆𝑆𝐵𝑙𝑜𝑐𝑘𝑠 = 𝑎 ∑ 𝑦𝑗. - 𝑎𝑏 𝑗=1 𝑆𝑆𝐸 =𝑆𝑆𝑇 - 𝑆𝑆𝑇𝑟𝑒𝑎𝑡𝑚𝑒𝑛𝑡𝑠 -𝑆𝑆𝐵𝑙𝑜𝑐𝑘𝑠 b. Multiple Comparisons In the context of a Randomized Complete Block Design (RCBD), multiple comparisons refer to statistical procedures used to determine which treatment means are significantly different from each other after conducting anANOVA(Analysis of Variance). The RCBD is commonly used to control for variability among experimental units by grouping them into blocks, with each block containing a complete set of treatments. When ANOVA indicates significant differences between treatment means, it may be necessary to conduct additional tests to pinpoint the specific differences. Multiple comparison Engineering Data Analysis Page11 ethods, such as Fisher’s LSD method, can be employed for m this purpose. Computing Formulas for Multiple Comparisons in Randomized Complete Block Design: Sum of Squares (SS) Calculations: 𝑎 𝑏 2 𝑦.. 2 𝑆𝑆𝑇 = ∑ ∑ 𝑦𝑖𝑗 − 𝑎𝑏 𝑖=1𝑗 =1 𝑎 2 𝑦.. 1 2 𝑆𝑆𝑇𝑟𝑒𝑎𝑡𝑚𝑒𝑛𝑡𝑠 = 𝑏 ∑ 𝑦𝑖. - 𝑎𝑏 𝑖=1 𝑎 2 𝑦.. 1 2 𝑆𝑆𝐵𝑙𝑜𝑐𝑘𝑠 = 𝑎 ∑ 𝑦𝑗. - 𝑎𝑏 𝑗=1 𝑆𝑆𝐸 =𝑆𝑆𝑇 - 𝑆𝑆𝑇𝑟𝑒𝑎𝑡𝑚𝑒𝑛𝑡𝑠 -𝑆𝑆𝐵𝑙𝑜𝑐𝑘𝑠 Least Significant Difference: 2𝑀𝑆𝐸 𝐿 𝑆𝐷 = 𝑡α/2,α(𝑛−1) 𝑛 Degrees of Freedom (df): Total: 𝑎𝑏 − 1 Treatments: 𝑎 − 1 Blocks: 𝑏 − 1 Error: (𝑎 − 1)(𝑏 − 1) Mean Square 𝑆𝑆𝑇𝑟𝑒𝑎𝑡𝑚𝑒𝑛𝑡𝑠 𝑀𝑆𝑇𝑟𝑒𝑎𝑡𝑚𝑒𝑛𝑡𝑠 = 𝑎−1 𝑆𝑆𝐵𝑙𝑜𝑐𝑘𝑠 𝑀𝑆𝐵𝑙𝑜𝑐𝑘𝑠 = 𝑏−1 𝑆𝑆𝐸 𝑀𝑆𝐸 = (𝑎−1) (𝑏−1) c. Residual Analysis and Model Checking Residualanalysisisastatisticalmethodusedtoevaluate alinearregressionmodel'sperformancebyanalyzingresiduals. It helps identify and rectify model issues, assess assumptions, and detect outliers for improved efficiency. Residual analysis involves understanding residuals. This includes collecting data, Engineering Data Analysis Page12 c onstructing a regression model, calculating residuals, visualizing residuals, analyzing residuals, assessing model assumptions, refining the model if necessary, and interpreting results. This analysis aims to evaluate the goodness of fit ofa statistical model to the observed data. Inanydesignedexperiment,examiningtheresidualsand checkingforviolationsofbasicassumptionsthatcouldinvalidate the results is always important. As usual, the residuals for the RCBD are simply the difference between the observed and estimated (or fitted) values from the statistical model, say, ^ 𝑒𝑖𝑗 = 𝑦𝑖𝑗 − 𝑦𝑖𝑗 and the fitted values are represented by the equation: ^ 𝑦𝑖𝑗 = 𝑦𝑖· + 𝑦·𝑗 − 𝑦·· he fitted value represents the estimate of the mean T response when the 𝑖th treatment is run in the 𝑗th block. Then, the residuals would be best identified by examining them in a table.Hence,residualplotsareusuallyconstructedbycomputer software packages. Engineering Data Analysis Page13 GLOSSARY nalysis of variance (ANOVA): A method of decomposing the total A variabilityinasetofobservations,asmeasuredbythesumofthesquaresof these observations from theiraverage,intocomponentsumsofsquaresthat are associated with specific defined sources of variation. isher’s least significant difference (LSD) method: A series of pairwise F hypothesis tests of treatment means in an experiment to determine which means differ. evels of a factor: The settings (or conditions) used for a factor in an L experiment. andomized complete block design: A type of experimental design in R which treatment or factor levels are assigned to blocks in a random manner. eplicates: One of the independent repetitions of one or more treatment R combinations in an experiment. esidual: adifferencebetweenavaluemeasuredinascientificexperiment R and the theoretical or true value. REFERENCES McHugh, M. (2011). Multiple comparison analysis testing in ANOVA. Biochemia Media, 21(3),203 - 209. https://doi.org/10.11613/BM.2011.029 Montgomery, D. C., & Runger, G. C. (2010). Applied Statistics and Probability for Engineers. John Wiley & Sons. Multiple Comparisons (2024). https://online.stat.psu.edu/stat500/lesson/10/10.3#:~:text=Multiple%20c omparisns%20conducts%20an%20analysis,Brand%20A%20to%20Bra nd%20B Engineering Data Analysis Page14 Submitted by: 1. Atazan, Kyla Mei Q. 2. Bantaculo, Lorie Jane A. 3. Baroy, Britney Dawn Q. 4. Dadang, Carlrienyle 5. Dinlayan, Princess Hannah D. 6. Dueñas, Felix E. 7. Fabre, Angela P. 8. Miano, Kaye Roxette S. 9. Piloton, Marithe M. 10.Roz, James Michael 11.Tabor, Lex Aine June D. Engineering Data Analysis Page15