Healthcare Sustainability and Analytics PDF
Document Details
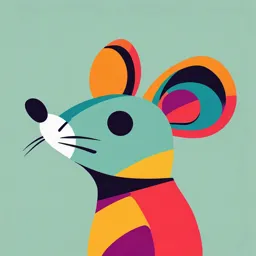
Uploaded by ShinyMorningGlory
Tags
Related
- Applied Analytics for Business – Healthcare PDF
- Applied Analytics for Business – Healthcare PDF
- Specimen Collection, Transportation and Storage in Medical Microbiology PDF
- Introduction to Healthcare Analytics PDF
- Healthcare Analytics Value Management PDF
- Healthcare Analytics for Quality and Performance Improvement PDF
Summary
This document discusses the challenges and opportunities for healthcare transformation. It highlights the importance of analytics in improving healthcare quality and sustainability. The author explains that although healthcare organizations claim to implement quality improvement initiatives, only a few achieve sustainable improvements. Increased spending does not necessarily lead to improved quality.
Full Transcript
How sustainable is healthcare in its current state? Most healthcare organizations (HCOs) claim to be undertaking quality improvement (QI) initiatives, but only a few are consistently improving the quality of healthcare in a sustainable fashion. Despite increased spending on healthcare in the Unit...
How sustainable is healthcare in its current state? Most healthcare organizations (HCOs) claim to be undertaking quality improvement (QI) initiatives, but only a few are consistently improving the quality of healthcare in a sustainable fashion. Despite increased spending on healthcare in the United States, there is little evidence that the quality of healthcare can be improved by increasing spending alone. Health information systems is one technology with the potential to transform healthcare because, among its many capabilities, it can deliver the best evidence to the point of care, employs intelligent algorithms to reduce and prevent medical mistakes, and collects detailed information about every patient encounter. Even with growing volumes of data to analyze resulting from the continuing proliferation of computer systems, HCOs are struggling to become or remain competitive, highly functioning enterprises. This chapter will highlight current challenges and pressures facing the healthcare system, identify opportunities for transformation, and discuss the important role that analytics has in driving innovation and achieving healthcare transformation goals. **Healthcare Transformation---Challenges** **and Opportunities** Healthcare delivery is undergoing a radical transformation. This is occurring as the result of both necessity and opportunity. Change is necessary because, in many ways, the provision of healthcare is less efficient, less safe, and less sustainable than in the past. The opportunity, however, arises from the advancement of technology and its impact on healthcare delivery. Technology now allows increasingly intelligent medical devices and information systems to aid in clinical decision making, healthcare management, and administration. The challenge facing HCOs is to leverage advances in both clinical device technology and information technology (IT) to create and sustain improvements in quality, performance, safety, and efficiency. Data generated via healthcare information technology (HIT) can help organizations gain significantly deeper insight into their performance than previous technologies (or lack of technology) allowed. HCOs, however, face the very real risk of information overload as nearly every aspect of healthcare becomes in some way computerized and subsequently datagenerating. For example, radio frequency identification (RFID) devices can report the location of every patient, staff member, and piece of equipment within a facility; sampled every second, the location data captured from these devices accumulates quickly. Portable diagnostic equipment now captures and stores important patient clinical data, such as vital signs, and can forward that data to electronic medical records (EMRs) or other computerized data stores. Similarly, devices with embedded "labs on a chip" can now perform point-of-care testing for many blood-detectable diseases, and generate enormous volumes of data while doing so. HCOs must find a way to harness the data at their disposal and take advantage of it to improve clinical and organizational performance. Data analytics is critical to gaining knowledge, insight, and actionable information from these organizations' health data repositories. Analytics consists of the tools and techniques to explore, analyze, and extract value and insight from healthcare data. Without analytics, the information and insight potentially contained within HCOs' databases would be exceedingly difficult to obtain, share, and apply. But insight without action does not lead to change; data overload can risk impeding, not improving, the decision-making ability of healthcare leaders, managers, and QI teams. In my experience, the true potential of analytics is realized only when analytics tools and techniques are combined with and integrated into a rigorous, structured QI framework. This powerful combination helps to maintain the focus of QI and management teams on achieving the quality and business goals of an organization. Analytics can also be used to explore the available data and possibly identify new opportunities for improvement or suggest innovative ways to address old challenges. When an HCO uses analytics to focus improvement efforts on existing goals and to identify new improvement opportunities, healthcare can become more effective, efficient, safe, and sustainable. **The Current State of Healthcare Costs and Quality** A discussion on the topic of healthcare analytics must first begin with a discussion of healthcare quality. This is because analytics in healthcare exists for the purpose of improving the safety, efficiency, and effectiveness of healthcare delivery. Looking at the current and emerging challenges facing healthcare the way we looked at problems in the past can and will only result in more of the same. And it seems that many people, from healthcare providers who are overworked to patients who must endure unacceptably long waiting lists for relatively common procedures, are extremely dissatisfied with the way things are now. Despite the seemingly miraculous capabilities of the healthcare system to maintain the health of, and in many cases save the lives of, patients, the system itself is far from infallible. The question of how safe is healthcare delivery must continually be asked. The often-cited Institute of Medicine (IoM) report *To Err Is Human: Building a Safer Health System* declares that a "substantial body of evidence points to medical errors as a leading cause of death and injury."1 The report cites two studies that estimate between 44,000 and 98,000 patients die every year in hospitals because of medical errors that could have been prevented. These are people who expected the healthcare system to make them well again or keep them healthy and were horribly let down. According to the IoM report, the types of errors that commonly occur in hospitals include "adverse drug events and improper transfusions, surgical injuries and wrong-site surgery, suicides, restraint-related injuries or death, falls, burns, pressure ulcers, and mistaken patient identities." Not surprisingly, emergency departments, operating rooms, and intensive care units experience the highest error rates and those with the most serious consequences. Not only do hospital errors result in a staggering yet largely preventable human toll, but they result in a tremendous financial burden as well. It is estimated that the cost to society of these preventable errors ranges between \$17 billion and \$29 billon in both direct and indirect financial costs. Of course, the majority of these errors are not caused by deliberate malpractice, recklessness, or negligence on the part of healthcare providers. Rather, according to the IoM report, the most common causes of healthcare errors are "due to the convergence of multiple contributing factors" and that "the problem is the system needs to be made safer."2 In the near decade and a half that has passed since the release of the 1999 Institute of Medicine report, most of its findings are as relevant today as they were in 1999. Despite dramatic innovations in biomedicine and healthcare technology since the IoM report, many HCOs today still find themselves under immense pressures, some of which include: Improving quality and patient safety Ensuring patient satisfaction Adapting to changes in legislation and regulations Adopting new technologies Demonstrating improved patient outcomes Remaining sustainable and competitive The challenge facing HCOs today is to balance the need to innovate by adopting new technologies and improving processes while providing the essentials of safe, efficient, and effective patient care. While these two needs are complementary, with improved patient care as the ultimate goal, they both require financial, human, and technical resources that are drawn from a limited, and in some cases shrinking, resource pool. **The Cost of Healthcare** HCOs must endeavor to reduce unnecessary deaths, injuries, and other hardships related to medical errors and other issues stemming from substandard quality. But given that the cost of healthcare delivery seems to be increasing unabatedly, could healthcare be at risk of becoming unsustainable in its current form? Direct and indirect costs attributed to healthcare represent a significant and increasing burden on the economies of countries providing modern healthcare, and may not be sustainable at current growth rates. Figure 1.1 illustrates the immense cost of healthcare by showing the percentage of healthcare expenditures as a proportion of the gross domestic product (GDP) of selected countries.3 Of the countries in Figure 1.1, total health expenditure as a share of GDP ranges from 2.4 percent (Indonesia) to 17.4 percent (United States). Of significance is that healthcare expenditures in the United States totaled over 17 percent of its GDP---5 percent more than the next highest country, and almost 8 percent more than the OECD average of 9.6 percent. But not only have expenditures on healthcare increased in the United States from approximately 5 percent of GDP in 1960 to over 15 percent in 2008, they are expected to grow still further, reaching approximately 20 percent of GDP by 2018. Andy Grove, former chief operating office and chief executive officer of Intel Corporation and a pioneer in the semiconductor industry, once stated, "There is at least one point in the history of any company when you have to change dramatically to rise to the next level of performance. Miss that moment---and you start to decline." Given the numerous pressures and escalating costs facing the healthcare systems of many nations, *now* is the time for HCOs to innovate using available tools and technologies to transform into more sustainable, efficient, effective, and safe providers of care. **The Analytics Opportunity in Healthcare** The good news is that HCOs can take the necessary action to improve quality of care, increase value to patients, and raise the bottom line. Advances in HIT, and particularly the field of healthcare analytics, are now helping HCOs to reveal and act on opportunities for transformative improvement. The term "analytics" has been described in myriad ways. For the purposes of this book, I will refer to analytics as the systems, tools, and techniques that help HCOs gain insight into current performance, and guide future actions, by discerning patterns and relationships in data and using that understanding to guide decision making. Analytics enables leaders, managers, and QI teams within HCOs to make better decisions and take more appropriate actions by providing the right information to the right people, at the right time, in the right format, with the right technologyHealthcare analytics consists of the systems, tools, and techniques that help HCOs gain insight into current performance, and guide future actions, by discerning patterns and relationships in data and using that understanding to guide decision makingOne doesn't need to look far to observe the impact that analytics has had on other industries. Companies such as Google, Amazon, and others whose very existence depends on users' ease of access to highly targeted, tailored, and user-friendly information demonstrate the realm of the possible--- that the tools, techniques, algorithms, and data now exist to drive our analytics-powered world. The use of analytics in healthcare, however, has lagged behind other industries. Internet search engines make it incredibly easy to enter a search term and almost immediately retrieve a list of web pages that contain information pertaining to the search term ranked in order of relevance and likely usefulness. Yet anyone who has used an EMR or a reporting tool to look up information on a patient, or a group of patients, knows how difficult finding the necessary information can be. And anybody who has tried to get the information they need for a healthcare quality and/or performance improvement project would not be faulted for thinking that obtaining *any* information of value is downright impossible. **WHY QUALITY IMPROVEMENT PROJECTS FAIL** HCOs are always working to improve the quality of their care and the efficiency of their business operations. Many HCOs do not see much improvement in quality and performance despite engaging in multiple improvement initiatives. Unfortunately, some HCOs will undertake QI projects without an overall quality strategy or long-term evaluation plan and end up with many disconnected, halfevaluated projects that never seem to achieve their objectives. Some HCOs focus on improving quality in bursts, with intense activity and enthusiasm that lasts only for a short period of time. Such torrents of QI activity is usually in reaction to some negative event such as a critical incident, or after a "eureka" moment occurs in which an executive member learns something new at a conference, after seeing a product demonstration, or while speaking with a consultant. Once the initial excitement wears off the initiative, the unit, department, program, facility, or entire enterprise may revert back to its initial or some other suboptimal state if a solid quality framework and sustainability plan are not in place. Even HCOs with QI entrenched in their organizational culture, a proven track record, and well-evolved QI frameworks in place rarely achieve totalsuccess and must revisit areas of improvement (often multiple times) to help ensure that improvement results are maintained. This is because achieving change within HCOs is difficult and, much like breaking a bad habit, rarely is sustained after the first try. *Health care is the most difficult, chaotic, and complex industry* *to manage today \[and the hospital is\] altogether the most complex* *human organization ever devised.* *---Peter Drucker* Making changes to an HCO is difficult because healthcare is a very dynamic environment and in a constant state of flux. Innovations in healthcare technology are ushering in changes at a rapid pace, emerging diseases and changing patient demographics are presenting new treatment challenges to clinical staff, and organizations themselves face an ongoing barrage of new regulations and changes to funding models. What might have been an effective and/or necessary process, workflow, or policy 20 years ago (or even two years ago) may be no longer relevant, or in need of major updating to be made relevant once again. HCOs must evolve and adapt not merely to maintain and improve quality, performance, and patient safety, but to survive. Of course, the standard principles of providing safe, efficient, and effective patient care will never change---but exactly *how* that is done must *always* evolve. **LEVERAGING INFORMATION TECHNOLOGY** Although HIT is one of the largest drivers of healthcare innovation (or disruption, as some healthcare providers would claim), HIT provides the tools required to monitor, evaluate, and improve healthcare quickly and with clarity. In fact, improving quality in a modern HCO to the extent and at the pace necessary *without* the benefit of the information derived from HIT would be an onerous task. A NOTE ON TERMINOLOGY I will use the term "healthcare information technology" (HIT) when referring to systems that are mainly clinical in nature such as electronic medical record (EMR), radiology information system (RIS), and other similar systems. I will use the term "information technology" (IT) more generically to include both clinical and nonclinical systems (such as financial, supply chain management, and other such tools). Despite what some vendors may promise, it takes more than simply adopting HIT to improve quality and performance within an HCO. In fact, it is ironic that a mere decade ago many healthcare improvement efforts were likely stymied due to lack of data. Now it is entirely possible that improvement efforts could be hindered by having *too much* data available without the necessary experience and tools to analyze it and put it to good use. This is not to say that healthcare improvement cannot occur without the use of IT, but at some point every HCO must use data to monitor and evaluate ongoing changes and fine-tune improvements. I have seen mediocre HCOs become top performers as a result of the intelligent use of information in combination with strong leadership, a clear vision, a culture of innovation, and a drive to succeed. Although technology is never the *only* solution, analytics consists of many tools, technologies, and techniques that HCOs can employ to leverage the data amassed from the increasing number of HIT systems in operation. These innovations in combination with competent, effective leadership enable HCOs to become more efficient and adept at achieving, evaluating, and sustaining improvements in healthcare. **THE ANALYTICS KNOWLEDGE GAP** In pursuit of clinical and operational excellence, HCOs are drawing from diverse, nontraditional professions (from a healthcare perspective) to form QI and innovation teams. In addition to nurses, physicians, and administrators, it is not uncommon to see engineers, computer scientists, and other specialist roles working within healthcare. Although having traditional and nontraditional roles working side by side to solve the many problems facing healthcare brings incredible diversity and flexibility, this arrangement also poses some challenges. Successful healthcare quality and performance improvement initiatives require strong executive sponsorship and support, QI expertise, subject matter expertise, and information management and analysis expertise. Bringing these various disciplines together provides diversity that can lead to the synergistic development of innovations but also exposes significant knowledge gaps between these groups. (See Figure 1.2 for an illustration of this knowledge gap.) Each professional group brings with it its own particular skill sets, knowledge, and comfort levels working with data and analytics. The analytics knowledge gap may make it seem like nobody is speaking the same language, which can prevent teams from working effectively and cohesively together. To reduce friction and misunderstanding on healthcare quality and leadership teams, it is necessary to bridge the knowledge gap. Bridging the gap enables team members to communicate more effectively, to ask the right questions, and to frame the answers and insights in ways that make sense and are relevant to the improvement challenges at hand. **Leveraging Information for Healthcare Improvement** As HCOs turn to technological solutions to manage business operations and treat patients, many are literally becoming awash in data. In fact, some estimates are that healthcare data in the United States alone totaled approximately 150 *exabytes* (150 × 1018 bytes) in 2011 for clinical, financial, and administration systems; of course, this number will only continue to grow. In fact, a single large American healthcare provider alone is estimated to have accumulated up to 44 petabytes (a petabyte is 1015 bytes) of patient data from electronic health record data (including images and annotations).4 As HCOs continue to amass large quantities of data, that data is only of any value if it gets used. Many HCOs are becoming more "data centered," in **Healthcare** **Management** **& Leadership** **Information Gap** **Quality** **Improvement** **Information** **Technology** **FIGURE 1.2** The Analytics Information Gap between QI, IT, and Healthcare Leadership "BIG DATA" IS A RELATIVE TERM Although "big data" is a term commonly used to describe the very large data sets of today, there is no doubt that the anticipated future growth in healthcare data will make today's "big data" seem minuscule. I still remember when having 16 megabytes of random access memory on a computer was a big deal, and a 1-gigabyte hard drive was considered more storage than you'd ever need. that they are making conscious efforts to make better use of the data available to assist with decision making and QI initiatives. Of course, HCOs vary in the extent and degree of sophistication by which they are leveraging their available data for informed decision making and performance improvement. **TRADITIONAL TOOLS ARE OUTDATED AND INEFFECTIVE** As analytical tools become more commonly used in healthcare beyond executive-suite analysts and biostatisticians, the questions that are being asked are increasingly complex. It is becoming clear that traditional reporting approaches are becoming woefully inadequate and outdated---they are unable to deliver information that is accurate and timely enough to drive decision making, and they can only scratch the surface of today's growing healthcare databases. Healthcare leaders are dealing with a multitude of regulatory, quality, and financial pressures and need accurate, timely, and readily available information to make decisions. In fact, HCOs do not require more reports to achieve desired improvement goals. HCOs require better insight into their own operations, transparency across boundaries, and accountability for their performance. The limiting, conventional views about decision making, data, and reporting must be challenged to allow for creative use of the available data and emerging analytics tools to foster data-based (not gutbased) decision making---in real time and near the point of care. **INFORMING DECISION MAKING** It is commonly said that data must be used to "drive decisions" in order to impact quality and performance improvement. What does "drive decisions" really mean, however, and how do we measure and judge how well information is being used? Much information is produced by analysts and other users of healthcare business intelligence (BI) systems, and most of this information is consumed by managers and other healthcare leaders. But how does (or how can) all this information actually drive decision making? Unfortunately, the default position for many organizations with respect to using information is the same type of reporting on which they have always relied. I am sure that after installing new HIT and healthcare BI solutions, every organization requests the BI and analytics team to develop the exact same reports as before. This discomfort of leaving behind what never really worked anyway means that many HCOs fall into an information rut that inhibits them from truly leveraging the information at their disposal. It is not my intention to give the term "report" a bad name, as if reports are the root of all that is wrong with the use of healthcare data. The truth is that a report can come in many guises. One example is the old-fashioned monthly multipage report that is distributed throughout an organization but rarely makes it out of the e-mail in-box. (Nobody distributes *printed* reportsanymore, do they?) Dashboards, of course, are also reports, but good dashboards present up-to-date indicators, consisting of relevant metrics with targets to maintain accountability, that truly assist with making decisions. In fact, the usefulness of information has absolutely *nothing* to do with the medium in which it is presented. A graphical, interactive dashboard can be just as disadvantageous as a stale, printed multi-page report in tabular format if the information contained within does not help answer the pressing business problems facing an HCO. Tip The usefulness of information has absolutely nothing to do with the medium in which it is presented. Rather than getting caught up in which medium information is presented, I believe that analytics professionals need to focus on ensuring that the information that is being used for decision making and QI has most (if not all) of the following attributes, which will be described later in this book. It is: Accurate Timely Relevant (to the questions being asked) Directed (at the right individual or stakeholders) Analyzed (appropriately given the types of data and questions being asked) Visualized (in a way that makes sense to the stakeholder) **Beginning the Analytics Journey in Healthcare** QI is often considered to be a "journey" in healthcare because of the constant evolution the HCO undergoes, because of the constant learning required to adapt to a changing environment, and because quality is a moving target. An HCO should never strive for good enough, but should always be improving. The use of analytics within an HCO to improve quality and performance is a journey in much the same way. Analytics must be developed in an agile manner to keep pace with the changing needs of quality and performance improvement initiatives. Analytics specialists must keep their professional knowledge up to date and relevant because the technology thatenables analytics is always changing as are the analytic techniques (such as algorithms and statistical models) that are used to gain insight into healthcare data. Analytics is very much a moving target---what is sufficient (and even leading-edge) in today's healthcare environment most likely will not be five years from now. The role of analytics professionals in healthcare will continue to grow both in scope and in importance. I believe that for analytics to become a true game changer, analytics professionals must no longer be relegated to the back rooms of IT shops simply building reports and fulfilling endless data requests. Analytics must be brought to the front lines, where the innovative and transformational QI work takes place. Analytics professionals must be willing and prepared to engage with frontline QI teams and clinical staff directly, participate on quality initiatives, and experience what information is needed and how analytics is, and has the potential to be, used on the front lines. Information served up on a "report development request" basis *cannot* play a transformational role in healthcare improvement; transformation is possible only with embedded, agile, and motivated analytics teams working side by side with other QI team members to achieve the quality and performance goals and objectives of the organization. It is incumbent on healthcare leaders to enable QI, IT, and analytics teams to work together with frontline staff to support analytics-driven evidence- and data-informed quality and performance improvement initiatives. In order for that to happen, there must be some common understanding around the topics of technology, data, and QI so that professionals in these different disciplines can communicate effectively within a team-based project environment. Unfortunately, many QI professionals and QI team members have limited knowledge of the technology involved in healthcare analytics, what data is available, or even what analyses, visualizations, and other aspects of analytics can even be requested. Technology experts in IT who develop the code to transfer data from source systems to data warehouses (or other data stores) may not know the best format in which to make data available to BI and analytics tools, and so they may choose default data types based on how the data "looks" rather than on contextual knowledge of what the data means and how it will be used. Finally, analytics professionals who are building dashboards and other analytics for QI teams may not know the terminology around Six Sigma or Lean, and may not be familiar with the specific types of visualizations (e.g., statistical process control charts) or other analyses common with such methodologies. Despite where your HCO is on its analytics journey, remember that although the tools and technology of analytics will likely change at a rapid pace, the *people* are the most important component of healthcare analytics. The future of healthcare analytics will involve professionals from manyA NOTE ABOUT TERMINOLOGY It has been an enigma throughout the writing of this book how to name analytics professionals within the HCO. It is challenging to attach a label to a group of professionals who come from such diverse backgrounds, bring such an amazing range of skills, and play such an important role in bringing data to life within an HCO. As is typical in this book, I have shied away from using the trendy term of the day, and instead have leaned more toward classical or enduring terminology. I have opted to use the term "analytics professional," or sometimes "analytics developer," to be as inclusive as possible. I know that not everyone will agree with this term, and I am ambivalent about it myself, but it is a term I believe is nonetheless both inclusive and descriptive. disciplines, with a common understanding of how analytics and QI must work together, using information made possible via analytics to create an environment able to provide patients with safe and effective healthcare of the absolute highest quality possible. **Notes** **Fundamentals of** **Healthcare Analytics** *If you always do what you always did, you will always get* *what you always got.* ---Albert Einstein Effective healthcare analytics requires more than simply extracting information from a database, applying a statistical model, and pushing the results to various end users. The process of transforming data captured in source systems such as electronic medical records (EMRs) into information that is used by the healthcare organization to improve quality and performance requires specific knowledge, appropriate tools, quality improvement (QI) methodologies, and the commitment of management. This chapter describes the key components of healthcare analytics systems that enables healthcare organizations (HCOs) to be efficient and effective users of information by supporting evidence-informed decisions and, ultimately, making it possible to achieve their quality and performance goals. **How Analytics Can Improve Decision Making** Healthcare transformation efforts require decision makers to use information to understand all aspects of an organization's performance. In addition to knowing what *has* happened, decision makers now require insight into what is likely going to happen, what the improvement priorities of the organization should be, and what the anticipated impacts of process and other improvements will be. Simply proliferating dashboards, reports, and data visualizations drawn from the HCO's repository of health data is not enoughto provide the insight that decision makers need. Analytics, on the other hand, can help HCOs achieve understanding and insight of their quality and operational performance by transforming the way information is used and decisions are made throughout the organization. Analytics is the system of tools and techniques required to generate insight from data. The effective use of analytics within an HCO requires that the necessary tools, methods, and systems have been applied *appropriately* and *consistently*, and that the information and insight generated by analytics is accurate, validated, and trustworthy. In modern healthcare, substantial quality and performance improvement may be stymied without changes to the way information is used and acted upon. With this in mind, the fundamental objective of healthcare analytics is to "help people to make and execute rational decisions, defined as being data driven, transparent, verifiable and robust":1 **Data driven.** Modern healthcare standards demand that clinical decisions be based on the best possible evidence that is generated from extensive research and data. Yet administrative decisions, process and workflow design, healthcare information technology (such as EMRs), and even some clinical decisions are often not held to these standards. Analytics in healthcare can help ensure that *all* decisions are made based on the best possible evidence derived from accurate and verified sources of information rather than gut instinct or because a process or procedure has always been done in a certain way. **Transparent.** Information silos are still a reality in healthcare due to the belief by some that withholding information from other departments or programs best maintains autonomy and control. This belief, however, often has the opposite effect and invariably leads to misunderstandings and a deterioration of trust. A key objective of analytics in healthcare is to promote the sharing of information and to ensure that the resultant insight and information is clearly defined and consistently interpreted throughout the HCO. **Verifiable.** Consistent and verifiable decision making involves a validated decision-making model that links the proposed options from which to choose to the decision criteria and associated methodology for selecting the best available option. With this approach, the selected option "can be verified, based on the data, to be as good as or better than other alternatives brought up in the model."2 **Robust.** Because healthcare is a dynamic environment, decisions must often be made quickly and without perfect data on which to base them. Decision-making models must be robust enough to perform in nonoptimal conditions. That is, they must accommodate biases that might be introduced as a result of missing data, calculation errors, failureconsider all available options, and other issues. Robust models can benefit from a feedback loop in which improvements to the model are made based on its observed performance. Analytics and Decisions Healthcare analytics improves decision making by replacing gut instinct with data-driven, transparent, verifiable, and robust decision methods. **Analytics, Quality, and Performance** The techniques and technologies of analytics provide insight into how well an HCO is performing. Analytics enables healthcare leaders and QI stakeholders to make evidence-informed decisions through techniques, tools, and systems that: Clarify and improve understanding of patterns seen in data. Identify when (and why) change has occurred. Suggest (and help validate) the next logical steps to achieve desired change. First and foremost, analytics must help answer questions and drive decision making related to achieving and maintaining safe, effective, and efficient delivery of healthcare. Effective healthcare analytics, however, consists of more than pointing statistical analysis software at large databases and applying algorithms and visualization techniques. What distinguishes analytics from most currently deployed reports and dashboards are the graphical, mathematical, and statistical tools and techniques to better *understand* quality and performance issues, and more importantly, to identify what possible actions to take. Figure 2.1 illustrates the ways in which information can be used to support decision making for quality and performance improvement initiatives. Most HCOs use reports and dashboards to review past performance (circle 1). Although a solid understanding of past performance is essential in identifying quality issues and monitoring progress toward meeting targets, relying *solely* on retrospective data provides little insight into what an HCO should be doing now or in the future. Many HCOs are adopting the capability for real-time performance monitoring, which may include real-time (or short-cycle) dashboards that provide a reasonable picture of what is currently happening within the HCO (circle 2). To be effective, real-time monitoring must encompass appropriateindicators that are aligned with strategic and/or tactical performance goals and be linked to triggers within business processes that can signal that an action or decision is required. Tip To be effective, real-time monitoring must encompass appropriate indicators that are aligned with strategic and/or tactical performance goals and be linked to triggers within business processes that can signal that an action or decision is required. The reports and dashboards typical of circles 1 and 2 may help highlight what has occurred in the past, or what is currently occurring. But on their own, the information typical of circles 1 and 2 provides little insight into *why* performance is the way it is. Analytics goes one step further and helps answer questions such as why problems likely are occurring, highlights relationships between events and issues (circle 3), and, given the right models and data, can even begin to anticipate future outcomes and occurrences (circle 4). Analytical approaches (such as regression modeling and data mining techniques, for example) help to highlight relationships between various factors that, to various degrees, may be impacting quality and performance. For example, within existing reports and dashboards, an HCO might see that there has been a steady hospital-wide drop in patient satisfaction over the last quarter, and that an increase in central line infections has occurred over a similar period. Reports and dashboards may also highlight an increase in emergency department lengths of stay, and an increase in staff absenteeism rates. But most standard methods of reporting are incapable of providing any insight into *why* these issues are arising; charting methods such as basic bar or line graphs would be able to illustrate a trend over time and the amount of change in a measure that has occurred. Analytics tools and techniques go one step further to help provide better insight into why these quality issues are present, determine if they are related, and predict future trends and possible outcomes. **Applications of Healthcare Analytics** One benefit of analytics is to enable healthcare leaders, QI teams, and other decision makers to ensure that the decisions being made are evidencebased, transparent, verifiable, and robust. Most areas of healthcare can benefit from decision making that meets these expectations; a few examples are outlined next. **Process and workflow improvement.** Efficient, effective, affordable, and safe patient care begins with processes and workflows that are free of barriers to quality and from which waste is reduced or eliminated. Determining what to improve, and how to improve it, is the responsibility of dedicated multidisciplinary QI teams. The productivity of these QI teams, however, is greatly enhanced when they can leverage analytics to provide detailed insight into the processes and workflows that comprise the management and provision of healthcare. QI teams rely on analytics for superior analysis of baseline data to identify bottlenecks and other causes of poor quality and performance. Analysis of baseline performance and quality data helps QI teams to identify and prioritize these causes so that the improvement initiatives selected are the most likely to have an impact and be successful. Analytics is also necessary for monitoring ongoing performance of processesand workflows, after improvements have been made, to ensure that the improvements are sustained in the long term. **Clinical decision support (CDS).** Many people incorrectly consider analytics as merely an extension of reporting. But analytics is not just a back-office capability. Analytics in support of clinical decision making can take on many roles, ranging from providing suggestions and evidence regarding the management of a single patient to helping manage an entire unit or department during a surge in patients. CDS is perhaps the ultimate use of healthcare analytics, which is disseminating timely, actionable information and insight to clinical providers at the point of care when that information is required and is the most useful. CDS leverages the information available within the entirety of the enterprise data warehouse (EDW) and clinical source systems to give providers insight into many clinical issues, ranging from possible diagnosis suggestions to predictions for excessive length of stay or adverse outcomes. An example of analytics in CDS is computerized provider order entry (CPOE) systems. The best of these systems automatically check the order with medical guidelines and compare ordered medications with other medications a patient is taking to check for the possibility of adverse drug interactions. Benefits of CDS systems are already being realized; one study demonstrated a 40 percent reduction in adverse drug reactions and other critical events in just two months.3 Other examples of analytics in CDS include flagging a patient as being at risk for an extended emergency department visit, or assisting with the triage of multiple patients presenting with an unknown respiratory ailment during influenza season. In the first case, the patient may be placed on special protocols to prevent unnecessarily long stays in the emergency department. In the second, analytics can help fill gaps in patient information and identify which new cases may be high-risk, allowing care providers to take appropriate isolation and infection control precautions. **Population health management.** Population health management is "the coordination of care delivery across a population to improve clinical and financial outcomes, through disease management, case management and demand management."4 Analytics helps HCOs achieve these improvements by identifying patient subpopulations, risk-stratifying the subpopulations (that is, identifying which patients are at highest risk of poor outcomes), and using CDS tools and best evidence to manage patients' and populations' care in the best way possible. Analytics also contributes to the ongoing tracking of patients to determine overall compliance and outcomes. **Payer risk analysis and fraud prevention.** One contributing factor to the high cost of healthcare is fraud and other improper billingto healthcare insurance. Healthcare data analytics is expected "to fundamentally transform medical claims payment systems, resulting in reduced submissions of improper, erroneous or fraudulent claims."5 This transformation in fraud prevention is possible because computer algorithms are able to analyze healthcare databases, scanning for patterns and other clues in the data that might indicate fraudulent activity and other irregularities. Once a manual, painstaking, and imprecise process, this is now an automated, immensely more efficient process, saving healthcare systems billions of dollars. For example, the Centers for Medicare and Medicaid Services (CMS) achieved \$4 billion in recoveries because of the fraud detection abilities possible with data analytics.6 In addition to improving understanding within each of these and other components of healthcare, analytics offers the potential to break through traditional barriers and allow understanding across so-called silos. **Components of Healthcare Analytics** Analytics consists of much more than back-office analysts applying computer algorithms to ever-growing volumes of data. Analytics exists in healthcare to enhance the quality and safety of patient care while reducing costs. Patient care is a human-driven endeavor, therefore healthcare analytics requires the *input* of stakeholders to define what is useful and necessary. The *output* that healthcare analytics provides must be utilized by leaders, QI teams, and other decision makers in order to have any effect. Between the initial input and the resultant output, there are many levels and components to an analytics system that make evidence-based decision making possible. Forrester Research, Inc., identifies the "business intelligence \[BI\] stack" 7 to consist of the following layers: Infrastructure Performance management Supporting applications Analytics Discovery and integration Data Infrastructure The Forrester Research BI stack (and similar models from other organizations) provides a highly detailed summary of the components required to construct a BI infrastructure within a business enterprise (of which healthcare is but one example). The purpose of this book is to focus on theessentials of analytics for healthcare quality and performance improvement, so I have employed a modified stack optimized for healthcare analytics that focuses on business problem identification and insight generation. Figure 2.2 illustrates this "analytics stack," a representation of what is required of an analytics system within an HCO to provide insight and support evaluation of outcomes. Although not strictly necessary for analytics, a well-developed BI infrastructure will definitely support and enable analytics and decision making throughout the HCO. For an excellent healthcare BI resource, I recommend *Healthcare Business Intelligence: A Guide to* *Empowering Successful Data Reporting and Analytics*.8 The analytics stack described here does not focus on the particulars of any one data warehouse model or technology but instead assumes that a mechanism is in place for data to be made available for analytics in a suitable format. The basic layers of this analytics system for performance and QI are: Business context Data Analytics Quality and performance management Presentation **Business context layer.** This layer is the foundation of an analytics system and represents the quality and performance goals and objectives of the HCO. Included in the business context is the "voice of the patient" as a reminder that, above all, the goal of HCOs is to provide value to patients by delivering effective, efficient, and safe medical care. Every organization will have its own set of goals and objectives because of varying circumstances, demographics, and other factors. The goals and objectives of the business, and the strategies the HCOs employ to achieve them, drive requirements at every other level. **Data layer.** This layer of the analytics stack represents the quality, management, integration, and storage of data and the associated infrastructure. With the generation and accumulation of healthcare data comes the need to extract and integrate data from source systems such as electronic medical records (EMRs), store the data securely, and make high-quality data available for analytics and BI uses. Aspects of the data layer include: **Data sources.** These are the source systems such as EMRs, plus financial, supply chain, and other operational systems, that providers and other staff utilize in their day-to-day work. By and large, data in source systems is optimized for transactions, not analysis. When more than one data source exists, the data sources must be integrated to achieve true enterprise-wide visibility. **Operational data store.** As part of the integration process of bringing multiple data sources together into a single enterprise view, an HCO may opt for an operational data store (ODS) as an intermediary level of data integration. The ODS forms the basis for additional data operations (such as cleaning and integrity checks). **Enterprise data warehouse.** An EDW is built when available sources of data must be cleaned, transformed, and integrated for analysis and reporting to provide an enterprise-wide view of data. The data warehouse contains key indicators and other performance data pertinent to the quality and performance of multiple domains throughout the HCO. **Analytic sandbox.** The data in the EDW may be stored in a way that is aggregated to allow for faster, more efficient queries and analysis. Analysts may require access to lower-level data (for example, linelevel patient data) to test new business rules or to run data-mining algorithms. The analytic sandbox is an area set aside for data for these purposes that does not negatively impact the performance of other operations on the EDW outside the analytics sandbox. **Data marts.** It may not be necessary, or advisable, for somebody to see all the possible data from across the entire enterprise that is available in an EDW. In these cases, data marts are instantiated; datamarts are subsets of data from the data warehouse (or the entire data set when only one source system exists), are usually organized by lines of business or healthcare domain, and represent what somebody within a particular line of business would need to see to best understand the performance of his or her program, department, or unit. **Integration.** Combining multiple source systems into a connected EDW is the process of integration. Without proper integration, an EDW would be nothing more than a collection of data points without any clear logic linking them. Integration can occur through a process of Extraction/Transformation/Load (ETL), which, in the most typical scenario, copies data from the source system(s), applies logic to transform it to the analysis needs of the organization, and loads it into an EDW. Other forms of integration, including virtualization, which defines a single interface that links to every point of data in the HCO, are increasingly common as volumes of data expand and new approaches to data management are required. **Analytics layer.** This layer is comprised of the tools and techniques that analytics teams use to generate information and actionable insight that drives decision making. Components of this layer include the intellectual knowledge of analytics teams and the computer software tools to apply that know-how. In this layer, analytics helps to identify quality and performance problems, develop analytical models appropriate to the problem, perform statistical analyses, generate insight into problemsolving approaches, and trigger necessary action. The analytics layer requires strong involvement from stakeholders, who provide the requirements for analytics that link the strategic-level goals and objectives for the organization to more tactical-level analytics for decision making on the front lines by managers and QI teams. Consideration of how analytics projects and teams are to be managed to ensure a successful deployment is also necessary. There are several key features of the analytics layer: **Online analytical processing (OLAP).** OLAP tools typically accompany data sets that are preaggregated and stored in a multidimensional format (that is, based on dimensions and facts) that allows users to quickly and interactively analyze data from multiple perspectives. OLAP typically consists of three types of operations: drill-down, which allows users to obtain and navigate through additional detail (for example, viewing revenue from each line of business of an HCO), roll-up (the opposite of drill-down, or the consolidation or aggregation of data), and slice-and-dice (with which users can extract a subset of data and view it in multiple dimensions). **Ad hoc analytics.** When more complex analysis is required than is available through OLAP tools, analysts will use more statistical-based or other specialized tools to conduct deeper analysis. This type of analysis usually relies on nonaggregated data, and is often best performed in an analytics sandbox away from other EDW activities. **Text mining.** Text mining involves extracting value (by deriving patterns and trends) from unstructured text data. This is data that is stored in progress notes and wherever else codified data entry is not performed. **Data mining/predictive analytics.** These two disciplines consist of the process of determining patterns and trends in the data, and using the knowledge and understanding of those patterns and trends to make predictions about future performance or occurrences. **Quality and performance layer.** This layer aligns analytics to the processes that need to be improved, the indicators by which processes and outcomes will be evaluated, and the performance targets desired by the HCO. The actual improvement strategies and methodologies to be used (such as Lean and Six Sigma) should also be considered in this layer. This is important because improvement projects usually require extensive analysis of baseline performance and typically utilize indicators to evaluate project outcomes in order to sustain improvements in the long term. **Processes.** Data is a by-product of the work that clinical providers and other healthcare workers perform. When these workflows and processes are documented, data can be aligned with them to increase understanding of what the data means. **Indicators.** These are measures of certain aspects of an HCO's performance. **Targets.** These are values that represent what the performance levels of a process or workflow should be, and represent the ideal range of an indicator. **Improvement strategy.** This describes how an HCO intends to address quality and performance issues, and what methodology the organization intends to employ (such as Lean or Six Sigma). **Evaluation strategy.** This is how organizations plan to monitor and evaluate the performance of key processes and indicators within the HCO. **Presentation layer.** This layer of the analytics stack can be considered the analytics "user interface." The presentation layer manages the form in which insights and information are delivered to the decision makers. This layer is comprised of elements ranging from traditional reports to contemporary dashboards and can include more specialized tools such as geospatial visualization (or mapping). Although much of the heavylifting of healthcare analytics is situated within the data, analytics, and quality management layers, the presentation layer is critical because how well information is communicated will impact its usefulness to decision makers, QI teams, and other stakeholders. Given the different components that must work in concert to provide meaningful insight to decision makers, the effectiveness of an analytics system for quality and performance improvement will be greatly diminished without an analytics strategy. (See Chapter 3 for further information about developing an analytics strategy.) The purpose of the analytics strategy is to guide the HCO's ability to rapidly respond to the information needs of stakeholders while maintaining a consistent direction in supporting the quality and business goals of the HCO. The analytics strategy provides a guide for sorting through the many and perhaps conflicting analytics needs of the HCO, and ensuring that each of these layers is configured, aligned, and/or developed appropriately to achieve the quality goals of the HCO. The strategy must guide decisions regarding what projects to undertake, what tools to invest in, and how to maximize return on investment in analytics tools. The analytics strategy will align with, or be a component of, the overall BI strategy, since many analytics capabilities will depend on the extent to which a BI infrastructure is in place. Beyond the layers of data and technology of an analytics system is how the data is used---that is, the problem-solving that spans all of these layers. For example, many dashboards and reports merely reflect what has happened, and provide data in typical, predictable ways. But analytics encourages and assists people to think differently about the data they have and the problems they are solving. Sometimes a simple change such as applying a new visualization or applying a new statistic can help illuminate an existing problem in a whole new light. Other times, more sophisticated analytical techniques will be required to solve a particularly perplexing problem. All components of the analytics stack require careful consideration to ensure that the known questions of today are being addressed, and that an analytics infrastructure is being built that ultimately will address the *unknown* questions of the future. 3 **Developing an Analytics** **Strategy to Drive Change** *You've got to think about big things while you're doing small* *things, so that all the small things go in the right direction.* ---Alvin Toffler An analytics strategy is more than simply a data utilization strategy, a data analysis strategy, a technology strategy, or a quality improvement strategy. In fact, elements of all these are required for an effective analytics strategy. An analytics strategy is necessary to ensure that an organization's analytics capabilities are aligned with its quality and performance improvement needs. This chapter discusses what an analytics strategy is, and will outline the steps necessary to develop an effective analytics strategy. In developing a strategy, the chapter will discuss the components of and inputs to an analytics strategy, stakeholders who must be involved in developing the strategy, communicating the strategy, and how to implement it for maximum success. **Purpose of an Analytics Strategy** The purpose of an analytics strategy is to guide a healthcare organization's (HCO) ability to rapidly respond to the information needs of stakeholders while maintaining a consistent direction in supporting the quality and business goals of the HCO. It provides a guide for sorting through many, perhaps conflicting information and analysis needs, and prevents the HCO from being too swayed by vendor hype and other distractions. The strategy provides analytics teams with the focus and direction needed to establish analytics and business intelligence (BI) as a strategic resource for healthcare leaders, quality improvement teams, and other decision makers within the HCO. Ultimately, the analytics strategy must aid the HCO to determine: What data is most required to address key quality, efficiency, and performance issues facing the HCO; What major analytics development projects to undertake and on what tasks to focus the analytics team; What skills and knowledge are necessary in the HCO's analytics team; What data and integration infrastructure is necessary to support analytics initiatives; What analytics software and hardware tools to invest in; and How to maximize return on investment in analytics tools, teams, and training by demonstrating value to the HCO. One definition of strategy is "a bridge that connects a firm's internal environment with its external environment, leveraging its resources to adapt to, and benefit from, changes occurring in its external environment," and as "a decision-making process that transfers a long-term vision into day-to-day tactics to effect the long-term plan."1 This definition is pertinent to an analytics strategy because the analytics strategy will enable the HCO to leverage its information and analytics resources as it responds to and begins to control the many factors, both internal and external, that impact overall quality and performance. An analytics ANALYTICS AND BUSINESS INTELLIGENCE The analytics strategy is a critical adjunct to an HCO's BI strategy, because the hardware, data integration, and data management required for BI also enables the use of analytics. If an HCO is just embarking on the development of a BI infrastructure (perhaps including enterprise data warehouse development), then analytics requirements should be considered during the requirements gathering phase. If a BI infrastructure is already in place, an analytics strategy can help to identify any gaps that exist in BI that might need to be addressed to fully enable the desired analytics requirements of the HCO. strategy also helps to guide day-to-day decisions regarding systems, people, tools, and techniques, with the long-term goal of enabling analytics to provide information and insight regarding the most pressing problems facing the HCO. HCOs should develop a strategy for analytics to ensure that the information resources of the organization are aligned with the activities necessary for achieving the HCO's quality and performance goals. Having a strategy cannot guarantee success, but without a strategy, analytics and IT development, team formation, and infrastructure procurement will proceed without the benefit of any clear plan or mandate. This likely will result in an investment of money and time (both resources usually in short supply) in analytics infrastructure, technology, and development projects that *may not* contribute to the fundamental goals of the organization, and may distract the HCO from achieving its goals. One of the most challenging aspects of working in a healthcare environment is the "emergencies." Not the medical emergencies---those are the domain of the clinicians---but the frequent and urgent need for data and information. These urgent requests range from information required by government agencies, to data for critical incident occurrence reviews, to a quick aggregation of data for a researcher racing to meet a grant deadline. These are a fact of life when working with healthcare data and cannot be avoided, but they should not result in complete and utter chaos within an analytics team. One struggle for healthcare analytics teams is to maintain sight of "true north," that is, to know where and when to resume work on strategic priorities despite many competing demands. The analytics strategy can help prevent analytics teams from becoming overwhelmed and underproductive by keeping the organizational priorities in focus. Without a strategy that outlines what the analytics priorities are and against which to judge the priority of new and urgent requests, what gets done is usually the request initiated by the person who is the most persuasive, or the problem that seems the most interesting to the analytics team, not necessarily the issue or problem that is the most important to the organization as a whole. An analytics strategy that aligns with the quality and performance goals of an organization will help the analytics team balance competing requests with strategic priorities and help the team maintain their productivity by reducing the feeling of being overwhelmed. A solid analytics strategy will help enable the analytics team to become a strategic information resource for business improvement and not simply purveyors of reports and data. When analytics teams are primarily occupied fulfilling the data requests of others, the result is that not much time is available for the strategic development of the group. STRATEGIC DEVELOPMENT VERSUS DEVELOPMENT BY AGGREGATION I often joke (somewhat ruefully) that analytics tools and capabilities within an HCO are developed through aggregation instead of through design and strategy. For example, whenever an analytics team gets a request for information, they might add the report, dashboard, or other analytics tool to the general analytics or BI repository because "somebody else might need it." The result is a sizable collection of reports and other tools that even the team doesn't remember what they all do. To make matters worse, this causes work to be replicated because one analyst may not be aware of what somebody else has done, or because the original work has been lost in the collection. **Analytics Strategy Framework, with a Focus on** **Quality/Performance Improvement** In most healthcare information technology (HIT) initiatives, the information technology (IT) department of an HCO is primarily responsible for the implementation and maintenance of the technology itself (that is, the hardware, software, implementation, testing, and maintenance). The primary *users* of HIT, on the other hand, reside within the business side of the organization, and it is also the business side that gains benefit and value from having such tools in place. The partnership between the business side and IT in development of an effective BI and analytics infrastructure may at times be at odds, not because of competing interests necessarily, but because each group may not be aware of or fully understand the interests and priorities of the other. Building an Analytics Strategy---Templates To download sample templates and worksheets for developing an analytics strategy within your organization, please visit this book's web site, http://HealthcareAnalyticsBook.com. Healthcare analytics is not immune to this requirements tug-of-war between IT and the business side of an organization. For example, with **Analytics Strategy Framework, with a Focus on** **Quality/Performance Improvement** In most healthcare information technology (HIT) initiatives, the information technology (IT) department of an HCO is primarily responsible for the implementation and maintenance of the technology itself (that is, the hardware, software, implementation, testing, and maintenance). The primary *users* of HIT, on the other hand, reside within the business side of the organization, and it is also the business side that gains benefit and value from having such tools in place. The partnership between the business side and IT in development of an effective BI and analytics infrastructure may at times be at odds, not because of competing interests necessarily, but because each group may not be aware of or fully understand the interests and priorities of the other. Building an Analytics Strategy---Templates To download sample templates and worksheets for developing an analytics strategy within your organization, please visit this book's web site, http://HealthcareAnalyticsBook.com. Healthcare analytics is not immune to this requirements tug-of-war between IT and the business side of an organization. For example, with quality and performance improvement. The areas that should be considered in a comprehensive analytics strategy include: Business and quality context Stakeholders and users Processes and data Tools and techniques Team and training Technology and infrastructure These components of an analytics strategy framework are discussed in the following sections. **Business and Quality Context** The business and quality context outlines the business problems facing the HCO, and the quality, financial, and performance goals to which the HCO is committing to address those problems. It is essential to start drafting the analytics strategy with a clear understanding of the needs and requirements of the business; without clear guidance from the needs of the business, analytics may not provide the insight and information required to support the evidence-based decision making necessary to achieve the desired quality and performance goals. To this end, all elements of an analytics environment should be aligned in support of the needs of the business. The root of every successful analytical venture in which analytics is actively used throughout an HCO by decision makers is a detailed description of the problem being addressed and a clear articulation of why solving that problem is important to the organization. A well-articulated business problem defines a gap between the current (undesirable) state and the future (more desirable) state. Without a clear and concise problem definition, much effort and resources may be focused on addressing mere *symptoms* of a much deeper-rooted problem, or on issues that are not really a priority at all. There are many types of problems facing HCOs, ranging from financial pressures to regulatory requirements; problem statements identify which are the most pressing for an individual HCO to address at a given time. The types of problems that HCOs need to address will also direct the types of analytics (and supporting data) required. Some problems typical of those experienced and expressed by HCOs include: **Clinical quality.** Is the HCO providing the best possible care and diagnostics at the right time, to the right patients, and in the most efficient and safe manner possible? **Financial.** Is the HCO making clinical, operational, and administrative decisions that are the most financially sound while still in the best interest of the patients? **Patient throughput and value.** Is the HCO providing value to its patients by minimizing the time they must wait for appointments, assessments, treatments, or other services within the organization, and are they satisfied with the performance and care they experience? **Human resources.** Is the morale and well-being of the HCO's staff consistent with HR guidelines and, more importantly, consistent with positive patient experiences? Quality and performance targets are a necessary accompaniment to the problem definition. HCOs cannot possibly improve every process, eliminate every inefficiency, and reduce every risk at once; otherwise, chaos will ensue and *nothing* will improve. Quality and performance targets define what the current priorities of the HCO are, and help to focus the efforts of quality improvement and analytics teams. The quality goals represent the most pressing problems that have been identified by stakeholders in the organization, highlight what most needs to improve, and indicate the desired or target performance levels. An analytics strategy needs to include the most relevant and important quality goals. This is because an HCO needs to communicate these critical goals to all relevant programs, departments, and units that will be held accountable for their performance. **Stakeholders and Users** From a project management perspective, stakeholders are "individuals and organizations that are actively involved in the project, or whose interests may be positively or negatively affected as a result of project execution" and "may also exert influence over the project and its results."2 Likewise, an analytics stakeholder is a person or group of persons who are impacted by, will be users of, or otherwise have a concern or interest in the development and deployment of analytical solutions throughout the HCO. In a modern HCO there are few people who are *not* impacted in some way by the use of analytics to improve quality and performance, and there are fewer yet whose roles could not be enhanced through the innovative and effective use of analytics. When developing an analytics strategy, it is important to elicit and document what each of the stakeholders will require, and develop approaches to ensure that their information needs are being met. There are many stakeholder groups within an HCO; analytics stakeholders typical within an HCO are summarized in Table 3.1. Stakeholder Description Patient The person whose health and healthcare experience we're trying to improve with the use of analytics. Sponsor The person who supports and provides financial resources for the development and implementation of the analytics infrastructure. Influencer A person who may not be directly involved in the development or use of analytics within the HCO, but who holds considerable influence (positive *or* negative) over the support of analytics initiatives. Customer/user A person within the HCO who accesses analytical tools, or uses the output of analytical tools, to support decision making and to drive action. **Patient.** The most important analytics stakeholder within an HCO is the patient. The patient is the reason healthcare exists, and is whom we are trying to care for in safer, more efficient ways through the use of analytics. Most pertinent quality improvement methodologies implore quality improvement practitioners not to lose sight of what is the best for the patient. Although it is possible to forget this fact when not working on the front line, analytics professionals must always remember that they are building analytics to directly support the teams that improve the health and in-hospital experience of the patient. **Sponsor.** The sponsor may be one of the most critical stakeholders in the successful implementation and application of analytics within the HCO. The project sponsor is "the individual or group within or external to the performing organization that provides the financial resources, in cash or in kind, for the project."3 This is the individual (or group of individuals) within the organization at a corporate level who approves, or provides a very strong recommendation to approve, the financial resources necessary to implement a viable analytics infrastructure. In many HCOs, the sponsor may be the same executive who recommends and/or approves funding for other IT initiatives. Keep in mind, though, that analytics efforts cross the boundary between IT and the business so there are likely to be clinical, business, and/or technical sponsors for analytics initiatives. **Customer/user.** From a project perspective, the customer is the individual or group that makes use of a project's product.4 Although the customers and users are often synonymous, within a large organization the customer is often the one who *pays* for the product or work, and the users are the ones who make direct use of the product. The customers and/or users are the individuals within the HCO who require and use the information and insight available with analytics. It is important not only to know *who* these analytics users and customers are, but *how* they intend to interact with the analytics tools and resultant data. For example, will the results of analytics be used to influence clinical decision making, financial planning, quality/process improvement, or for other reasons? **Influencers.** Influencers are people who, though not directly involved in the development or use of analytics within an organization, wield significant influence over it. Influencers can be found at almost every layer of an organization. It is important that influencers be informed of and understand the benefits of analytics within an HCO. Without the support of influencers at all levels of the HCO, important analytics initiatives may suffer or even be shut down. Nothing is worse for analytics within an HCO than apathy---the thinking that the "same old" data and information is good enough when it clearly is not. An obvious subset of customers are the "traditional" users of analytics--- the decision makers, analysts, and quality improvement facilitators. It is not uncommon, however, to see more frontline staff, including physicians and nurses, receive information regarding their performance. In addition, analytics tools are making their way to the point of care as they become embedded in clinical applications, which in turn provides critical decision support evidence and insight to frontline providers when and where it is needed most. Stakeholders classified as users are likely to be the most diverse, and will vary on several important dimensions. Table 3.2 lists several typical customers or users of analytics, as well as a few high-level analytics use cases for each user that are indicative of how analytics will be applied. Customer Sample Analytics Use Cases Physician Use real-time analytics for improving diagnostic accuracy. Use personalized performance report to adjust care practices. Unit manager Determine which patients are likely to exceed length-of-stay targets. Quality improvement team Identify bottlenecks in patient flow. Evaluate outcomes of quality improvement initiatives. Executive Evaluate and monitor overall performance of the organization. When developing the analytics strategy, it is a good idea to document analytics use cases, or how stakeholders intend to use analytics to make decisions and guide quality and performance improvement projects. Analytics use cases, in combination with goals and objectives of the organization, identify what data elements are most important, what indicators will be necessary to calculate, and what types of accessibility and usability factors (such as dashboard design, configuration of automated alerts, and mobile access) need to be considered. Information elicited from stakeholders to develop analytics use cases should include: Specific problems being addressed by the HCO. Decisions for which analytics insight is required. Actions that are triggered by analytics indicators. Risks that analytics identifies and/or helps to mitigate. What key processes need to be monitored and/or improved. What indicators are required to monitor quality and performance. Obtaining as much information as possible about the possible uses of analytics will help to identify any gaps in analytics capabilities and reduce the likelihood that critical analytics needs will be missed. Strategies for Working Well with Stakeholders Analytics initiatives are most likely to succeed when stakeholders are involved throughout all phases of a project. Here are a few strategies for working well with stakeholders. Identify key members of each of the stakeholder groups. Understand the needs of each stakeholder group, and the needs of members within each stakeholder group. Listen to, acknowledge, and act on the input of stakeholders. Keep stakeholders informed of progress. Deliver on promises made to stakeholders and demonstrate the value of analytics in addressing the stakeholders' needs. **Processes and Data** Accurate, timely, and readily available data is the backbone of all analytics used for decision making, especially in quality and performance improvement projects. Without data, it is impossible to determine baseline performance, use a verifiable decision-making process to decide on improvement opportunities, or evaluate outcomes. Modern computerized clinical systems, such as EMRs, contain dozens if not hundreds of individual data elements; with multiple systems online within HCOs, the potential exists for thousands of possible data items from which to choose. Even if every data item captured from available computerized systems within an HCO is made available via an enterprise data warehouse or other data store, most of this data would require additional processing and analysis to be useful. To make data useful, an analytics strategy must address: How to determine which data is most important for quality and performance improvement. How the data is managed and its quality assured. How the data links back to business processes for necessary context. See Table 3.3 for a summary of strategy components relating to data and processes. **Processes and Data** Accurate, timely, and readily available data is the backbone of all analytics used for decision making, especially in quality and performance improvement projects. Without data, it is impossible to determine baseline performance, use a verifiable decision-making process to decide on improvement opportunities, or evaluate outcomes. Modern computerized clinical systems, such as EMRs, contain dozens if not hundreds of individual data elements; with multiple systems online within HCOs, the potential exists for thousands of possible data items from which to choose. Even if every data item captured from available computerized systems within an HCO is made available via an enterprise data warehouse or other data store, most of this data would require additional processing and analysis to be useful. To make data useful, an analytics strategy must address: How to determine which data is most important for quality and performance improvement. How the data is managed and its quality assured. How the data links back to business processes for necessary context. See Table 3.3 for a summary of strategy components relating to data and processes. Data management and governance Who is responsible for data management, governance, and stewardship? What policies and procedures exist for data governance and management? Business processes What business processes and workflows align with important quality and performance issues of the organization? What data is available for measuring performance and quality of key processes? If no data exists, what proxy measures are necessary or available? What additional processing and analysis is required on available data? **DATA QUALITY, MANAGEMENT, AND GOVERNANCE** Before any analytics are possible, the relevant and necessary data must be understood and made available. Given the many possible sources of data within an HCO, one challenge is integrating data from these source systems into a manageable and accessible framework from which data can be drawn for analytics. These multiple data sources must all be managed to ensure suitability and usability for analytics purposes. Tip Data from source systems must be inventoried, analyzed, documented, and aligned with business processes. Successful execution of an analytics strategy requires relevant data to be identified, documented, processed, and made available to appropriate analytics users and applications. It may not be possible, feasible, or even necessary to account for every available data source. When initiating, or improving, the use of analytics within an HCO, focus on ensuring access to data that is related to the organization's major quality goals and key business objectives. Trying to encompass too much will only serve to water down the strategy document and risk sullying the insight and information required by stakeholders. Remember that a goal of the analytics strategy is to focus efforts on achieving the most important quality and performance objectives of the HCO. As the organization's priorities evolve, so, too, can the strategy document remain aligned with the priorities of the organization. At this point, new business problems and additional data can, and should, be considered. Tip Remember that the goal of the analytics strategy is to focus efforts on achieving the most important quality and performance objectives of the HCO. Tip Remember that the goal of the analytics strategy is to focus efforts on achieving the most important quality and performance objectives of the HCO. techniques, plus their acquisition strategy (build versus buy), need to be outlined in the strategy. Selection of appropriate software, statistics, or models is necessary to ensure that the "right kind" of analytics can be performed to address stakeholder needs and the HCO's business problems. Inappropriate tools and misaligned capabilities can lead to issues as basic as providing an inappropriate summarization to using a predictive model that does not work with the data available or is inappropriate for the use an HCO was intending. For example, if an HCO is looking to determine its geographic catchment area based on ZIP codes to fine-tune a marketing campaign, that information might be best presented visually using some sort of geographical representation rather than a table of numbers and ZIP codes. Not having the tools to properly visualize data in meaningful ways for decision makers would be a capability gap. Another example relates to advanced analysis; many reporting tools do not include anything other than basic statistics (such as mean, median, etc.). Yet sometimes an analysis needs to look beyond these simple statistics to determine correlation or to implement more complex statistical models. Because there are many ways in which analytics can be used, there are many different types of analytics tools. Several of the most common types of analytics tools include: **Statistical.** Statistical tools are used for deeper statistical analysis that is not available in most "standard" BI or reporting packages, including correlation and regression tests, ANOVA and *t*-tests, nonparametric tests, and statistical process control chart capabilities. **Visualization.** Beyond the static charts and graphs typical of almost all spreadsheet and business analysis software, some analytics users are looking for advanced visualization tools that allow them to interact visually with and explore data that is dynamic (that is, the visualizations update as the data is updated). **Data profiling and quality.** Because the volume of healthcare data is growing, HCOs are increasingly relying on software to identify and highlight patterns of good and poor data within a data set, and to help fix and prevent instances of poor-quality data. If an HCO has invested significantly in a BI infrastructure, there may not be much money available for analytics-related capabilities beyond what comes with the BI suite. Adding new and specialized tools to the analytics tool belt can become cost-prohibitive (especially when expensive "value-add" modules of already expensive base software are required). The good news is that there are very good open-source tools such as R (www.r-project.org) that can provide significant analytical horsepower without a prohibitively high price tag. For More Information on Tools and Techniques The landscape is constantly changing regarding the available tools for analytics. For an up-to-date summary of the most important tools and most recent developments, please visit this book's companion web site, http://HealthcareAnalyticsBook.com. **Team and Training** Analytics is a very quickly evolving field, and it is impossible for one person to be an expert in all aspects of BI and healthcare analytics. Training and professional development are key to ensuring that a knowledge gap does not become a gap in analytics capability within an organization. Professional development can (and most definitely should) involve both autodidactic and instructor-led instruction on the use of existing tools, the introduction to new tools, and education on new innovations in analytics (e.g., predictive analytics) and related technologies (e.g., new database formats for "big data"). Investing in analytics training is one of the smartest choices an HCO can make from the perspective of using available information to the maximum extent possible to enable evidence-informed decision making and smarter quality and performance improvement activities. Analytics teams, especially those working closely with quality improvement teams, are not composed solely of "analytics professionals" (that is, expert developers, analysts, and/or statisticians). HCOs are creating more interdisciplinary teams to tackle quality performance improvement issues. For example, it is common to have MBAs and engineers working alongside nurses and physicians to tackle various issues facing an HCO. So, too, are analytics teams becoming more interdisciplinary. In fact, it benefits an entire HCO to ensure that stakeholders who rely on analytics have some degree of knowledge about tools, techniques, and technologies available for analytics. The information gathered in the "stakeholders" section of the strategy document can be used to identify what type of knowledge is required of all analytics users, and to develop a plan to ensure necessary information and/or training is made available. There are many skills at which a healthcare analytics professional must be proficient. In general, healthcare analytics teams require broad knowledge in several key areas---the business of healthcare (both clinical operations and finance-related); technology (such as data warehouses, BI and analytics systems, and source systems such as EMRs); analytical techniques (including data and statistical modeling); and communications.6 See Table 3.4 for a summary of the common types of skills required of healthcare analytics professionals. Communications Analytics professionals must be effective communicators, both in listening and explaining. They must be able to listen to end users and subject matter experts to understand what information they need and how they intend to use it. They must also be able to explain analytics to those same people in a way that gets the point across. Effective, clear, and accurate writing Data graphing and visualization Requirements elicitation Technical Analytics can be a highly technical field, therefore analytics professionals need to be competent in several key areas in which healthcare analytics intersects with other technology disciplines. Intermediate programming and computation skills Database query skills Clinical Healthcare analytics professionals must know enough about the business of healthcare, from both a clinical operations and a financial perspective, so that they are aware of the context from which the data used is drawn. Basic healthcare processes Basic healthcare financing models Quality improvement One of the primary uses of healthcare analytics is for quality and performance improvement, and therefore healthcare analytics professionals must be familiar with at least the major approaches and methodologies in use within their HCO. They may not need to be Six Sigma Black Belts, but should be able to converse with the practitioners of quality improvement methodologies. Lean, Six Sigma, or other improvement methodology Process mapping Team and group facilitation Analytical Needless to say, healthcare analytics professionals must be analytical and curious in nature. The toughest of all challenges in healthcare analytics is identifying the root of a problem--- and this requires more than simply going through the motions of applying statistical tests and building data models. Ability to think critically and analytically Data centered; obsession with evidence-based problem resolution Familiarity with and ability to use scientific principles in addressing quality and performance problems In addition to bringing the right mix of people onto the analytics team, keeping their skills up to date and relevant is another important aspect of managing analytics teams. There are always new tools, approaches, and knowledge in the field of analytics. Regular training is required to keep analytics users' and developers' skills up to date, and they should be encouraged to maintain their own professional development. In this regard, there are certifications available in the field of analytics, and professional organizations, which enable analytics professionals to remain up to date. It is unlikely that a single person can be proficient at all the necessary skill sets required for effective analytics within an HCO. However, there may be strong generalists who are good at several of the key areas. It is important to recognize what skills are required to develop, implement, and utilize the particular types of analytics your organization requires, what gaps may exist, and what to do to address any gaps. Finding the Right People It is absolutely critical to have the right mix of people on an analytics team. Look for people who are naturally analytical, curious, and creative, and who will mesh well within a team environment **Technology and Infrastructure** *Note: HIT infrastructure is a very large and important topic. It is impossible* *to do it justice in one small section of a book. The purpose of this section is to* *provide a high-level overview of how business and analytical considerations* *should drive technical requirements, not to delve deeply into all aspects of* *technical infrastructure.* Interestingly, technology and infrastructure are often the first stops along the analytics path taken by IT professionals. There are numerous reasons for this. Many analytics professionals reside in the IT departments within HCOs, and IT departments are naturally drawn to think about servers, networks, and other infrastructure requirements. Analytics must remain very business-focused because of the information and insight needed by healthcare leaders, quality improvement teams, and other decision makers. The technology required to enable analytics, however, can be complex especially in larger organizations with numerous data sources and many stakeholders. Because of the volumes of data that may be available, the variety of sources supplying data, the sophisticated algorithms that can be applied to the data, and the speed at which decisions need to be made, analytics is growing beyond what can be accomplished by a single analyst running statistical software on a stand-alone computer. Instead, a highly connected and reliable communications and data infrastructure to enable the sharing of what information analytics produces is necessary to drive evidence-informed decision making in most modern healthcare environments. Aligning the Needs of the Business and IT Ideally, the analytical needs of an organization and the technological requirements to supply those needs figure prominently in the organization's IT infrastructure deployment strategy. An HCO's data infrastructure is the backbone of analytics. The three key elements of the infrastructure include the *network* (that provides connectivity between all elements of IT infrastructure), *servers* (on which applications are run and data is made available), and *physical storage* (the devices on which data are stored),7 and now cloud computing (in which case the data used for analytics may not be housed by the HCO at all). All these components are typically managed by an HCO's IT department, and they must be chosen and scaled appropriately to support the many applications and services required by an HCO. Closely related to these more physical elements of infrastructure is what is sometimes called a *knowledge and discovery layer*. This layer of infrastructure is where integration of various data sources occurs. For example, the data resulting from a hospital visit by a single patient may span multiple source systems, such as admission/discharge/transfer, electronic patient record, lab information system, and radiology information system. For analytics, it is best if this information is integrated into a single view and the knowledge and discovery layer helps to logically link these various independent sources of data into a cohesive, comprehensive, and cross-silo representation of a patient's visit. Although there are many different applications and tools that fit within this layer of infrastructure, the tools that are most pertinent to analytics and the most common in HCOs include Extraction/Transformation/Load services that copy information from source systems, transform it (into a form suitable for a data warehouse), and load it into a data store so that it is accessible for analytics. Newer approaches to integration include loose coupling of data so that a unified view of data spans multiple sources of data without necessarily needing to copy it all into a completely different data store; in other words, through a modified data schema, a "virtual" database can access data from source systems directly. One challenge of developing an infrastructure to support analytics is that analytics requirements will undoubtedly expand as more data sources are added, new problems and issues confront the HCO, new analytical capabilities are required, and new hardware and software systems, optimized for analytical performance, emerge on the marketplace. Scalability, which "allows us to maintain a consistent level of performance regardless of changes and growth,"8 must be built into an analytics infrastructure so that the HCO has spare capacity to grow into as the amount and types of data, as well as analytics needs of stakeholders, continue to evolve and expand. Although the analytics strategy may not necessarily state what technical infrastructure should be acquired, the strategy should make it clear what the near- and long-term analytics needs of the business are going to be. The decisions made regarding hardware selection and infrastructure design and configuration essentially set the boundaries for what analytics will be capable of within the organization. The analytics strategy can be a very important input to the HCO's overall technical strategy---the sooner that analytical requirements can be incorporated into an HCO's IT development plan, the less likely it is that technology will be purchased that is not appropriate (either insufficient or complete overkill) for the analytical requirements of the organization. **Developing an Analytics Strategy** Developing an analytics strategy is critical to ensuring that the analytical needs of an HCO are being met. Most HCOs will not be starting from square one, however. In all likelihood, there are many pockets of analytical knowhow throughout every HCO, suffering through some inadequacy in analytics capabilities and not living up to an analytics potential. For example, some of these analytical pockets may be using outdated or inadequate tools for data management or analysis, some may be reaching the limits of poorly designed data warehouses, and others might simply be so overwhelmed with report requests that they are unable to perform any "real" analytics. An analytics strategy is the starting point to help organizations achieve maximum benefit from their data. A completed strategy will help an organization identify what it does well, what it needs to do better, where it can consolidate, and where it needs to invest. The three main steps in creating an analytics strategy are: 1\. **Document the current state.** Review the six main components of strategy discussed above, and speak with stakeholders who are current (and potential users) of analytics to identify how analytics is currently used and what capability is required but does not yet exist, as well as what exists now but can be improved. **Identify gaps.** Documentation of the current state will reveal a laundry list of things that are needed. Some of these gaps will be in infrastructure, some will be in software/tools, some will be in knowledge/ training of the team, and some will be in knowledge of what is possible with analytics. 3\. **Execute strategy.** Once the gaps are identified, identify which gaps are a priority to address and which can be addressed quickly and affordably, and develop a plan to implement the strategy's recommendations. Many organizations in all industries spend a significant effort on developing a strategy but in the end fail miserably at executing on the strategy and achieving any of the goals and objects that the strategy was to enable. The bottom line is that developing a strategy is a wasted effort without a true intention and/or capability to execute on it. Having a strategy is simply not enough; organizations must find ways to actually execute what is set out in the strategy, otherwise it will become another piece of "shelfware." An analytics strategy is not set in stone; it needs to evolve as the analytics needs of the organization and its stakeholders evolve, as technology becomes better and/or less expensive, and as the state of the art in analytics itself changes. An organization should not be afraid to revisit the strategy frequently to ensure that it is up to date and that its execution is successfully meeting all stated requirements. 4 **Defining Healthcare** **Quality and Value** *Quality means doing it right when no one is looking.* ---Henry Ford Improving safety, quality, and value are the cornerstones of healthcare transformation. Although there are many ways in which quality and value are defined and measured, healthcare organizations (HCOs) m