Computational Molecular Microbiology (MBIO 4700) Lecture Notes PDF
Document Details
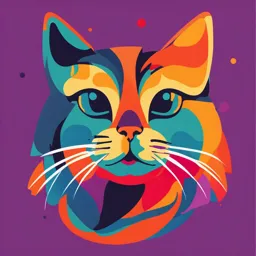
Uploaded by ArticulateBowenite6305
University of Manitoba
Abdullah Zubaer
Tags
Summary
These lecture notes discuss computational molecular microbiology, focusing on RNA and its various roles. It provides a comprehensive overview of different types of RNA molecules and their functions in biological processes, including regulatory roles.
Full Transcript
Computational Molecular Microbiology (MBIO 4700) ABDULLAH ZUBAER UNIVERSITY OF MANITOBA RNA RNA – work horse of the nucleic acids RNA folds - G – U viable H bonding ◦ Plus A – U and G – C ◦ 2nd and tertiary structures http://upload.wikimedia.org/wikipedia/commons/d/d3/RNA_secondary_structure....
Computational Molecular Microbiology (MBIO 4700) ABDULLAH ZUBAER UNIVERSITY OF MANITOBA RNA RNA – work horse of the nucleic acids RNA folds - G – U viable H bonding ◦ Plus A – U and G – C ◦ 2nd and tertiary structures http://upload.wikimedia.org/wikipedia/commons/d/d3/RNA_secondary_structure.png RNA folding WHY? RNA folding can have regulatory significance 1. Transcriptional and translational stops 2. Speed of translation and folding of peptides stabilizes rRNA 2nd and 3 D structures (ribosomes, ribozymes, rDNA ITS region, tRNAs etc.) 3. Riboswitches (involves metabolites) RNA foldback (translation); RNAi and miRNAs, siRNAs RNA thermometers (i.e. heat shock) attenuation (regulation); non-coding regulatory RNAs 4. Ribozymes – some enzymes are composed of RNA or some active sites are composed of RNA – ribozymes, snRNPs, RNaseP etc. Small non-coding RNAs miRNA siRNA piRNA i.e., RNA not only for translation of proteins. https://evolution.berkeley.edu/evolibrary/images/interviews/rnaworld2.gif RNA world? RNA the foundation supporting the DNA world? Why work on RNA? http://rfam.xfam.org/ Database for RNA families Classic RNAs mediating protein synthesis and “regulatory” RNAs mRNAs (messenger RNAs) Transcripts of protein-coding genes that act as templates for protein synthesis. rRNAs (ribosomal RNAs) RNA constituents of the ribonucleoprotein particles known as ribosomes, which mediate the decoding of mRNAs to the amino-acid sequences of proteins. tRNAs (transfer RNAs) Adapter molecules carrying individual amino acids to the site of protein synthesis that recognize specific codons in mRNA. (tmRNAs in Bacteria and some organelles; for rescuing stalled ribosomes) snoRNA – modify ribosomal RNAs snRNA – involved in splicing spliceosomal introns Modified from: http://www.nature.com/nature/journal/v451/n7177/full/451414a.html https://www.youtube.com/watch?v=FThA4Vxs3v4 Non-coding regulatory RNAs siRNAs (small interfering RNAs) Small RNAs (20–25 nucleotides in length) formed through cleavage of long double-stranded RNA molecules. siRNAs are particularly important for taming the activity of transposons and combating viral infection, but they can also regulate protein-coding genes. Synthetic siRNAs can also be artificially expressed for experimental purposes. miRNAs (microRNAs) Small RNAs (20–25 nucleotides in length) that are encoded by specific genes and function in repressing mRNA translation or in mRNA degradation in plants and animals. They are processed from long, single-stranded RNA sequences that fold into hairpin structures. piRNAs (or ~rasiRNA; Piwi-associated RNAs) Small RNAs (25–30 nucleotides in length) that are generated from long single-stranded precursors. They function in association with the Piwi subfamily of Argonaute proteins and are essential for the development of germ cells. Silencing repetitive regions and transposons. Long non-coding RNAs (lncRNAs) RNAs of 70 to thousands of nucleotides that participate in various cellular processes, miRNA attenuation, including mRNA splicing and ribosome biogenesis. circRNA (circular RNA) - can encode proteins and can have regulatory functions (can be biproducts of intron splicing) Modified from: http://www.nature.com/nature/journal/v451/n7177/full/451414a.html https://www.youtube.com/watch?v=FThA4Vxs3v4 Regulatory RNA – small noncoding RNAs siRNA – small interfering RNAs – derived from double stranded RNA usually transcribed from target gene (exons) (siRNA perfect match to mRNA or target RNA) miRNAs – encoded by miRNA genes -> TL suppression and mRNA decay; do not need to be a perfect match for mRNA (so one miRNA can target several mRNAs) PIWI piRNA (a type of rasiRNAs) – repeat associated small interfering RNAs -> RNA derived from repeat rich or transposon rich regions of the genome – suppression of gene expression in germ line tissues Rfam – RNA families Pevsner 2015 CRW back online: Comparative RNA website: https://crw-site.chemistry.gatech.edu/ Many tools: https://www.ebi.ac.uk/services https://www.ebi.ac.uk/services/all Significance in RNA folding: Can impact rate of translation, can influence the folding of the peptide during translation etc. RNA folds can be “targets” for viral nucleases OR other proteins etc. Example: Aaron S Mendez, Carolin Vogt, Jens Bohne, Britt A Glaunsinger; Site specific target binding controls RNA cleavage efficiency by the Kaposi's sarcomaassociated herpesvirus endonuclease SOX, Nucleic Acids Research, , gky932, https://doi.org/10.1093/nar/gky932 Nucleic Acids Research, Volume 46, Issue 22, 14 December 2018, Pages 11968–11979, mRNA features (RNA folds) allow certain viral nucleases to target host mRNAs. (site specific = means RNA fold – not actual sequence is targeted) Example of RNA molecules Ribozymes: RNA fold conserved (sequence conservation low) Group I introns Sethuraman et al. 2008 http://geohaus.wixsite.com/curriculum-vitaer/intron-structures- Group II intron (Secondary Structure) ORF “wheel with six “fingers” Some group II elements encode reverse transcriptase In the domain 4 region Note in domain 6 the A * → “A-2’OH . For more information on groupII introns: http://www.fp.ucalgary.ca/group2introns/ RNA folding method RNA sequence -> find base pairs along that string that are “correct” or “optimal” Strategy: -Maximize H-bonding (base pairs) -Minimize energy (optimal delta G) [mfold, ViennaRNA] Problem: will get many plausible structures (but are they biologically relevant? Need for manual adjustments. New generation of programs: (Incorporate the above PLUS) -Comparative analysis – computationally demanding ->need structural alignments [covariance analysis and Infernal] RNA folding tools RNAweasel RNAweasel (Lang et al., 2007; Trends in Genetics) Query – examined for RNA structures/features etc. (organellar genomes) http://megasun.bch.umontreal.ca/RNAweasel/ Output in Vienna notation: use ( ) above sequence to indicate pairings. Predicts: intron cores, rRNA, and tRNA folds. More RNA folding programs RNA structure (claims it can do pseudoknots) http://rna.urmc.rochester.edu/RNAstructureWeb/ Sfold - mostly for small RNAs but includes trans-splicing ribozymes http://sfold.wadsworth.org/cgi-bin/index.pl Vienna RNA package – general RNA folding package – rival to mfold http://www.tbi.univie.ac.at/RNA/ http://rna.tbi.univie.ac.at/#kinetics RNAfold web server http://rna.tbi.univie.ac.at/cgi-bin/RNAWebSuite/RNAfold.cgi MORE RNA folding … RNAsoft - Software for RNA/DNA secondary structure prediction and design http://www.rnasoft.ca/ The “list”: world according to wikipedia: http://en.wikipedia.org/wiki/List_of_RNA_structure_prediction_software Easy RNA Profile IdentificatioN* RNA motif - ERPIN http://rna.igmors.u-psud.fr/Software/erpin.php Need: alignment and secondary structure file *Gautheret D, Lambert A. (2001) Direct RNA Motif Definition and Identification from Multiple Sequence Alignments using Secondary Structure Profiles. J Mol Biol. 313:1003-11 Vienna notation for RNA folding: ((((((((.....)))))---))) 5’UCACGCAGCGCGCCUGCG---UGG3’ (consensus) ( ) base pairing ….. In loops ---- insertions/deletions (or “missing data”) Draft: Loop [ ] segment that is variable (bulge) 5’ 3’ Lorenz, R., Bernhart, S.H., Höner zu Siederdissen, C. et al. ViennaRNA Package 2.0. Algorithms Mol Biol 6, 26 (2011). https://doi.org/10.1186/1748-7188-6-26 Mfold - The UNAFold Web Server Mfold web server with a new and improved interface: http://www.unafold.org/mfold/applications/rna-folding-form.php M. Zuker Mfold web server for nucleic acid folding and hybridization prediction. Nucleic Acids Res. 2003. 31: 3406-3415. Warning: get a “mathematically predicted structure” may not be the actual biological structure - also you may get many structures that are thermodynamically possible. Also conditions such as temp., pH, and salt concentrations can be modified (in same advanced version of mfold) . Mfold NOW Part of “Unafold” http://www.unafold.org/mfold/applications/rna-folding-form.php features and assumptions mfold performs RNA and DNA secondary structure prediction using nearest neighbor thermodynamic rules -H-bonding -Van der Waals interactions -hydrophobic therefore: ----> nucleic acids assume helical configurations and like to be double stranded and base-pairs like to stack on top of each other (and find folds which minimize free energy – delta G) The UNAFold Web Server Mfold Mfold is a powerful “tool” that helps you model DNA and/or RNA molecules BUT YOU have to “drive it” based on pre-existing models set constraints), environmental conditions, and thermodynamic principles. HINT: analyze sequences in “small segments” (100 to 200 nt) and check the literature for structures, also be not afraid to fold by “hand”. Some long range interactions may be missed by the program (unless you “tell” the program). The UNAFold Web Server http://www.unafold.org/mfold/applic ations/rna-folding-form.php The UNAFold Web Server Mfold Mfold Leptographium wingfieldii strain WIN(M)1123 AY935608 1 catta x gcgag ttcacagtga ctcccaaccc gtgcaaacct taccgcatcc tttctgagag 61 agagcgcccg ttgcttcctg ccgggcggcg tgccctcctc cccccccctc tgcggggggg 121 ggggttggac gggcgcccgc ccgccggggg tgcggcgcgg ccgctccctc tcgccgcgaa 181 ccttctttgc agtataattg tatcgtctga gcaaaaccac agaatcgtt x a aaactttcaa 241 caacggatct cttggttctg gcatcgatga agaacgcagc gaactgcgat aagtaatgcg 301 aattgcagaa ttcagcgagc catcgaatct ttgaacgcac attgcgcccg ccagcattct 361 ggcgggcatg cctgtccgag cgtcattt y cc tccctcacgc agcgcgcctg cgtggtgttg 421 gggcgttctg cggccaggcc tgcgcccagc gcaggccgcc gcagcccccg aaagccagtg 481 gcgggccggc agcgggctcc gagcgcagta agcatcacgc cctcgctctg gacgctcccg 541 cctgcgccct gccccacaga ccggcagacg cgagtctgcc tccttctc y aa ggtt // xITS1x rDNA ITS regions [ITS1 5.8S gene ITS2] yITS2y http://www.unafold.org/mfold/applications/r na-folding-form.php Mfold Example > 1183ITS2 ribosomal internal transcribed spacer 1: CCTGTCCGAG CGTCATTTCC TCCCTCACGC AGCGCGCCTG CGTGGTGTTG 51: GGGCGTTCTG CGGCCAGGCC TGCGCCCAGC GCAGGCCGCC GCAGCCCCCG 101: AAAGCCAGTG GCGGGCCGGC AGCGGGCTCC GAGCGCAGTA AGCATCACGC 151: CCTCGCTCTG GACGCTCCCG CCTGCGCCCT GTCCCACAGA CCGGCAGACG 201: CGAGTCTGCC TCCTTCTCAA GGTTGACCTC GGATCAGG 238 The UNAFold Web Server http://www.unafold.org/mfold/applic ations/rna-folding-form.php The UNAFold Web Server Example > 1183ITS2 ribosomal internal transcribed spacer Mfold 1: CCTGTCCGAG CGTCATTTCC TCCCTCACGC AGCGCGCCTG CGTGGTGTTG 51: GGGCGTTCTG CGGCCAGGCC TGCGCCCAGC GCAGGCCGCC GCAGCCCCCG 101: AAAGCCAGTG GCGGGCCGGC AGCGGGCTCC GAGCGCAGTA AGCATCACGC 151: CCTCGCTCTG GACGCTCCCG CCTGCGCCCT GTCCCACAGA CCGGCAGACG 201: CGAGTCTGCC TCCTTCTCAA GGTTGACCTC GGATCAGG 238 mfold: 2 Forcing a string of consecutive base pairs. i.e. 1 goes with 238, 2-237, 3-236, 4-235 F 1 238 4 Constraints based on F 19 222 3 comparative analysis with F 25 45 3 other structures. F 58 94 7 (Mullineux 2008, 2009) Constraints based on comparative analysis with other structures. The UNAFold Web Server http://www.unafold.org/mfold/applications/ rna-folding-form.php The UNAFold Web Server png structure (format) http://www.unafold.org/mfold/applica tions/rna-folding-form.php Pseudoknots: -cannot be predicted with mfold http://en.wikipedia.org/wiki/Pseudoknot http://en.wikipedia.org/wiki/List_of_RNA_structure_prediction_software Why do we care about pseudoknots: Gene regulation (signals), Catalytic sites in ribozymes, Promote frame-shifting (ribosome skips one nucleotide); Staple, D. W., & Butcher, S. E. (2005). Pseudoknots: RNA structures with diverse functions. PLoS biology, 3(6), e213. https://doi.org/10.1371/journal.pbio.0030213 Integration of “tools” WHY? Study the evolution of ITS regions within a group of organisms. Observation: ◦ ITS1 and ITS2 “coevolve with regards to size” ◦ Conservation of structure supported by compensatory substitutions ◦ Phylogenetic context of ITS compaction Application: understand the function of ITS regions and assess the utility of ITS regions for molecular taxonomy. RNA structure logo: https://rth.dk/resources/slogo/ ----> Recent applications of sequence logos: “structural logos available” to show conservation of positions due to structural constraints. Requirement: code your structural element into the Vienna notation. Sequence Logo for ITS I sequences plus RNA fold "Vienna" bracket notation Helix 1 >1103 UCACGCAGCGCGCCUGCG---UGG ((((((((.....)))))---))) >1194 UCACGCAGCGCGCCUGCG---UGG ((((((((.....)))))---))) >OgalC1101 UCACGCGGCCCCCCCGCG---UGG ((((((((.....)))))---))) >984 UCACGCAGCACGCCUGCG---UGG ((((((((.....)))))---))) >1495 UCACGCGCCCCGGC-GCG---UGG ((((((((....))-)))---))) >1426 UCACGCGGC-CUCUCGCG---UGG ((((((((.-...)))))---))) >967 UCACGCAACGCGCCUGCG---UGG (((((((.......))))---))) >1380 UCACGCAGCGCGCCUGCGCGCUGG ((((((((.....)))))...))) >46 UCACGCAGCACGCCUGCG---UGG ((((((((.....)))))---))) >Obrev UCAUGCGGCCUUUC-GCG---UGG ((((((((....))-)))---))) --------------------------[[[((((( )) ))) ]]] (Mullineux 2008) Format for input data into structure logo program > ((((((((.....)))))---))) > UCACGCAGCGCGCCUGCG---UGG > UCACGCAGCGCGCCUGCG---UGG > UCACGCGGCCCCCCCGCG---UGG > UCACGCAGCACGCCUGCG---UGG > UCACGCGCCCCGGC-GCG---UGG > UCACGCGGC-CUCUCGCG---UGG > UCACGCAACGCGCCUGCG---UGG > UCACGCAGCGCGCCUGCGCGCUGG > UCACGCAGCACGCCUGCG---UGG > UCAUGCGGCCUUUC-GCG---UGG ( ) base pairing https://rth.dk/resources/slogo/ ….. In loops ---- insertions/deletions (or “missing data”) FORNA http://rna.tbi.univie.ac.at/forna/ Mutual information (MI) theory - Coevolution “Mutual dependence” between two variables. (can be linear OR non-linear dependence) Tries to quantify the "amount of information (bits)" obtained about one variable via observing the other variable. You see X what can you conclude about Y? M – mutual information ~ compensatory substitutions https://rth.dk/resources/slogo/ Covariance Model (CM) ▪ Stochastic context-free grammar “probabilistic models that account for long-range correlations along a sequence that occur because of base pairing of RNA sequences that is required to form appropriate secondary structure” ▪ Covariance model describes states M (including match states, insert states, and delete states), symbol emission probabilities Eddy and Durbin (1994) http://eddylab.org/software/infernal/ INFERNAL covariance a measure of "linear dependence" between the two random variables Structural alignment vs fold Use structural annotated RNA alignments to develop CMs (~ sequence profile) that can be applied to search genomes for RNA elements (based on recognizing RNA folds). For many non coding-RNAs – primary sequence conservation is low but there is conservation at the structural level. INFERNAL Invalid = no standard H bonding Tourasse, N. J., & Darfeuille, F. (2020). Structural Alignment and Covariation Analysis of RNA Sequences. Bio-protocol 10(3): e3511. DOI: 10.21769/BioProtoc.3511. Lai, D., Proctor, J. R., Zhu, J. Y., & Meyer, I. M. (2012). R-CHIE: a web server and R package for visualizing RNA secondary structures. Nucleic acids research, 40(12), e95. https://doi.org/10.1093/nar/gks241 StructRNAfinder (CM-based) StructRNAfinder (integrativebioinformatics.me) Web based server: Finding RNA families: input sequences in fasta format Arias-Carrasco, R., Vásquez-Morán, Y., Nakaya, H. I., & Maracaja-Coutinho, V. (2018). StructRNAfinder: an automated pipeline and web server for RNA families prediction. BMC Bioinformatics, 19(1), 55. For information: Grüning BA, Fallmann J, Yusuf D, Will S, Erxleben A, Eggenhofer F, Houwaart T, Batut B, Videm P, Bagnacani A, Wolfien M, Lott SC, Hoogstrate Y, Hess WR, Wolkenhauer O, Hoffmann S, Akalin A, Ohler U, Stadler PF, Backofen R. The RNA workbench: best practices for RNA and high-throughput sequencing bioinformatics in Galaxy. Nucleic Acids Res. 2017 Jun 5. doi: 10.1093/nar/gkx409. Protein / RNA interactions: 1. https://omictools.com/protein-rna-interactions-category 2. Mann et al. 2017: https://link.springer.com/protocol/10.1007/978-1-4939-6716-2_8 Promoters and regulatory sequences: http://www-bimas.cit.nih.gov/molbio/proscan/ Eukaryotic RNA pol 2: promoter scan (prediction only) http://wwwmgs.bionet.nsc.ru/mgs/programs/proga/ (RNA pol 2 promoters) http://www.fruitfly.org/seq_tools/promoter.html (also does prokaryotic promoters BUT ?) http://rna.tbi.univie.ac.at/#kinetics TurboFold Web Server Comparative Analysis! https://rna.urmc.rochester.edu/research.html Riboswitches: Nucleic Acids Res. 2004 July 1; 32(Web Server issue): W154–W159. Riboswitch finder—a tool for identification of riboswitch RNAs Peter Bengertand and Thomas Dandekar http://riboswitch.bioapps.biozentrum.uni-wuerzburg.de/server.html Nucleic Acids Res. 2003 Jul 1;31(13):3441-5. A software tool-box for analysis of regulatory RNA elements. Peter Bengertand and Thomas Dandekar Check out the list on this website: Online Analysis Tools - Promoters (molbiol-tools.ca) CMBL- PATLOC Pattern Locator . For a brief explanation how to use this program, go here. An application note about Pattern Locator has been published in Bioinformatics.. Additional functionalities have been added to this service, you may find an old version of Pattern Locator here.. Note: this is a free service provided without any warranty explicit or implied. www.cmbl.uga.edu CMBL- PATLOC (uga.edu) SoftBerry - cnnpromoter b The approach implemented in the program is described at: Solovyev V., Umarov R. (2016) Prediction of Prokaryotic and Eukaryotic Promoter s Using Convolutional Deep Learning Neural Networks. arXiv:1610.00121 [q-bio.GN] Genome2D (molgenrug.nl) Prokaryote Promoter Prediction MolgenRug Prokaryote Promoter Prediction Simple Prediction tool for prokaryote promoters. Note: If the input is too large, Time-out will occur after 5 minutes. genome2d.molgenru g.nl Online Analysis Tools - Promoters PROMOTERS & TERMINATORS. A. Bacterial SAPPHIRE Sequence Analyser for the Prediction of Prokaryote Homology Inferred Regulatory Elements - is a neural network based classifier for σ70 promoter prediction in Pseudomonas (Reference: Coppens L & Lavigne R (2020) BMC Bioinformatics 21(1): 415).. 70ProPred - is a predictor for discovering sigma70 promoters based on combining multiple features ... molbiol-tools.ca Infernal or non-infernal?