1b Visualisation Tasks.pdf
Document Details
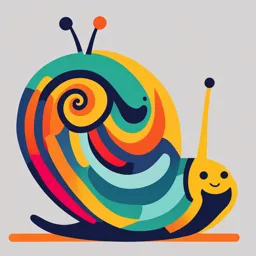
Uploaded by AffluentRisingAction9914
Tags
Full Transcript
Visualisation tasks 1 From “Visualisation Analysis & Design”, T. Munzner, CRC Press, 2015 (Chapter 3) 2 Three different actions Given a visualisation of a data set, a user can: – Analyse: consume or produce – Search:...
Visualisation tasks 1 From “Visualisation Analysis & Design”, T. Munzner, CRC Press, 2015 (Chapter 3) 2 Three different actions Given a visualisation of a data set, a user can: – Analyse: consume or produce – Search: location/target is known/unknown? – Query: find specific information 3 The Analyse action Consuming: user simply accesses the data using the visualisation to discover information not known before to present information to another person enjoy and find something interesting Producing: user actively creates something annotations of the data or the visualisation a persistent record of a visualisation (or aspects thereof) derive new data based on existing data 4 Running example: Analyse Discover: did anyone win both the TBHR and the Highland Fling in 2010? Present: here are the first Swandling club finishers for the TBHR in 2012 Enjoy: a racer, seeing how races have changed in many years, and looking for anyone they know Annotate: Mary Smith is the same person as Mary Bernados Record: this chart on my wall shows how much faster I have become in the Ben Lomond race over the past ten years Derive: calculate the percentage of active women in each club in each year 5 The Search action Locating target of interest in the visualisation Lookup: target known & location known (where and what) Browse: target unknown & location known (where) Locate: target known & location unknown (what) Explore: target unknown & location unknown 6 Position in TBHR 2015 45 40 35 30 25 20 15 10 5 0 AD AS BH BH BH CN DF DG EF ES ET FD GR GR HH HJ HR HT HY JE JT KU LI LY N H N T OI PL PP RG SB SE SE U K W BW HW S XC XD YS Imagine this was the race data that you were looking at… with position as the height of the bar, and the initials of the name of the runner as the label below each bar. There are many kinds of searches that a system could support, and we can map out examples of them here 7 Running example: Search Lookup: what position did John Thomas (JT) come in? (4) Browse: who won the race? (SB) Locate: did CG run this year? (no) Explore: is there any noticeable pattern? (no) Noting that it is the nature/form of the visualisation that will determine what is meant by ‘location’ – if it is location in the perceived visualisation (or simply a location in a data source) 8 The Query action Once you have found the data you are interested in, what will you do with it? – Identify: get all the information about it – Compare: differences between more than one data item – Summarise: produce an overview of more than one data item Summarise here means creating something new, in a semi-automatic way, from the raw data items in the set of query results. It might, for example, be a heat map or chart that compresses the set in some useful way, or a simple statistic based on that data set, or even representative sample. 9 Running example: Query Identify: What club was the TBHR 2015 winner from? Compare: Was ND faster than DF? Summarise: Of the first ten finishers, three were women 10 Targets Targets are the ‘things of interest’ in a visualisation Targets are not necessarily just the individual data points (although this is common) – for all data: trends, outliers, features – for attributes: distributions, dependencies, correlations, similarities – for network data: topology, paths – for spatial data: shape 11 Targets (things of interest) over all data Trends: – patterns: e.g. increase, decrease, plateau, etc. Outliers: – data points that don’t fit into an obvious pattern Features: – other structures of interest, depending on the domain 12 Running example: Targets over all data Trends: – JD’s finishing time in the TBHR decreased suddenly in the early 2010’s , but recovered later in the decade Outliers: – the winner’s time in 2015 was much slower than in all other years Features: – there are more females finishing in the first 25 places in the past four years than in the whole decade before that 13 Targets (things of interest) relating to Attributes For the values of one attribute: – distribution For the values of more than one attribute – dependency, correlation, similarity 14 Running example: one attribute target Distribution: the number of runners per age category Extremes: the number of runners over 70yrs Distribution of Age Categories, Ben Osmand, 2018 50 40 30 20 10 0 U18 U21 Open V40 V50 V60 V70 15 Many Attribute Targets Dependency: the value of one attribute can be determined directly by the value of another Correlation: there is a tendency for the value of one attribute to be linked to the value of another Similarity: attributes ranked according to their similarly (as defined by quantitative aggregates) 16 Running example: many Attribute Targets Dependency: a runner’s category (e.g. M40) is directly dependent on age & gender (e.g 42yrs, male) Correlation: there is a trend for a runner’s finishing time to relate to their weight Similarity: the average finish time for the Ben Osmand Race is closer to the average finish time for the Ben Styles Race than it is to the Ben Rinnes Race 17 Targets (things of interest) in Specific data sets For network data – topology (structure of the network) – paths (sequnces of connections between nodes) For spatial data – shape 18 Running example: Specific data set targets Network of run-buddies – topology: are there small groups of runners who always train with each other? If so, how many? – paths: if JH has training advice that he gives to the people he trains with, will that advice get to BK? A race where runners have to pass through a set of checkpoints – shape: what is the shape created by these checkpoints when they are connected by straight lines on a map? 19 Why is it useful to describe data types and visualisation tasks in such an abstract way? 20 Why is it useful to describe data types and visualisation tasks in such an abstract way? It is always good to pause and think about your data, and its use Decisions you make in your visualisation for one domain can be compared or used with those needed for another domain 21 Running example scenario I want to join a Hill Running Club that has an equal balance of gender membership, a wide distribution of members with different age categories, some very fast runners, and a dense network of run-buddies. A generic visualisation tool that supports a good variety of tasks should allow me to find this information easily This is to make us consider what should be in a generic system that supports the varied tasks described in this lecture 22 Looking for a data item (club) which is a categorical attribute of other data items (runners). Looking at the frequencies of an attribute (distribution of the genders of the members) for each of a set of data items in a table (clubs), and compare. Looking at the frequencies of an attribute (distribution of the age categories of the members) for each of a set of data items in a table (clubs), and compare. Deriving new data (calculating the average) of a quantitative attribute (finishing position) for each of a set of data items in a multi-dimensional table (runners in clubs), and compare. Looking at the structure of a set of networks (run-buddies) to identify information about them (the extent of connectivity), and compare. 23 A similar example I need to choose which companies to apply to for a job. I want a company that offers relatively high salaries for the sector and has strong social ties between its employees. I would like there to be an equal gender balance, and for there to be options for me to work in different countries. If I load the relevant data into the same visualisation system, will I be able to find this information easily? I’d hope so… as the high level tasks here are basically the same, even if the data and terminology are not the same 24 Summary Actions (verbs): things a user can do – analyse, search, query Targets (nouns): things a user can be interested in – all data trends, outliers, features – attributes distribution, dependency, correlation, similarity – networks topology, paths – spatial data shape 25 Visualisation tasks 26