Week 9 Lecture - Human Factors in Civil and Transport Engineering PDF
Document Details
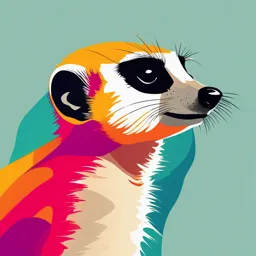
Uploaded by FinestPlatypus
UNSW Sydney
Dr Milad Haghani
Tags
Summary
This lecture covers mathematical modeling of road safety and statistical modeling methods in road safety research, focusing on accident severity analysis. It explores the complex interactions of vehicle, roadway, and human factors on resulting crash-injury severities.
Full Transcript
CVEN4405 Human Factors in Civil and Transport Engineering Week 9 Lecture Mathematical modelling of road safety Dr Milad Haghani School of Civil and Environmental Engineering Statistical modelling methods in road safety research Statistical modelling methods in accident severity analysis (micro-...
CVEN4405 Human Factors in Civil and Transport Engineering Week 9 Lecture Mathematical modelling of road safety Dr Milad Haghani School of Civil and Environmental Engineering Statistical modelling methods in road safety research Statistical modelling methods in accident severity analysis (micro-scale applications) Statistical modelling methods in accident severity analysis (micro-scale applications) • While progress in reducing the severity of accident injuries can be simply measured by the reduction in injury levels over time, insights into the effectiveness of injury-reduction technologies, policies, and regulations require a more detailed empirical assessment of the complex interactions that vehicle, roadway, and human factors have on resulting crash-injury severities. • Researchers have used a wide range of methodological tools to assess the impact of such factors on disaggregate-level injury-severity data. • Over time, there has been a steady improvement in statistical methodologies that have enabled safety researchers to extract more information from crash databases to guide a wide array of safety design and policy improvements. • In spite of this progress, quality and availability of data sources remain a major barrier. • Despite the limitations of traditional crash data (such as police underreported data), these data and models have improved our understanding of the factors that influence the occurrence of crashes and the personal injuries the result. Statistical modelling methods in accident severity analysis • Most existing highway-accident studies have extracted their data from police crash reports. • These reports are used to establish the frequency of crashes at specific locations and the associated injury-severity of vehicle occupants and other involved in these crashes. • Injury severities are commonly assessed by police officers at the scene of the crash: no injury, possible injury, evident injury, disabling injury, fatality (within 30 days of the crash). • Police reported data also include information on time of day, age and gender of the occupant(s), road surface conditions, weather conditions, possible contributing factor to the crash, roadway type, roadway lighting, speed limits, basic roadway geometry (curve, grade etc), type of crash (rollover, rear end, etc), type of object(s) struck, driver sobriety, safety belt usage, airbag deployment, … (examples provided in the next two slides) • This information can be expanded further by linking the data with government-provided roadway information such as traffic volumes, pavement friction, detailed roadway geometric characteristics, traffic signal details … • While these datasets have been commonly used, the inaccuracies associated with police data have also been well documented. • For example, less severe crashes are less likely to be reported to police and this less likely to appear in police databases. Also, there are often discrepancies between severity levels reported by the police and the assessment made by medical staff. Methodological considerations Statistical modelling methods in accident severity analysis: Driver behavioural adaptation & risk compensation Statistical modelling methods in accident severity analysis: Crash frequency (count) models • Crash frequency models: The number of crashes occurring on a roadway entity over a specific time period • In such models, the dependent variable is a non-negative integer. • These models essentially aim to determine factors that influence crash frequencies so that effective crash mitigation strategies, designs and policies could be implemented. • • • • • • Poisson regression model Negative binomial model (Poisson-Gamma) (handles over-dispersed data) Zero-inflated Poisson & negative binomial regression (accounts for preponderance of zeros) Gamma model Conway-Maxwell-Poisson model Negative binomial-Lindley model • Other issue: Spatial and temporal correlation among crash data count, unobserved factors • Other approaches have looked at crashes not as count data per se, but instead as the duration of time between crashes, leading to the use of duration models (e.g., hazard-based models). Statistical modelling methods in accident severity analysis: Data and methodological issues Statistical modelling methods in accident severity analysis: Data and methodological issues • Over-dispersion occurs when the variance of the observed accident counts is greater than what is expected under a Poisson distribution. In a Poisson model, it is assumed that the variance is equal to the mean (i.e., variance = mean), which is often not the case for real-world data like road accident counts. Over-dispersion suggests that the data have more variability than the Poisson model can account for. This means that the model underestimates the extent of variability, and the model's standard errors can be biased. To address over-dispersion, you might consider using a negative binomial regression model or other generalized linear models (GLMs) that allow for extra variability in the data. The negative binomial model allows the variance to be greater than the mean, providing a more flexible fit to the data. • UndeUnder-dispersion, on the other hand, occurs when the observed accident counts have less variability than what is expected under a Poisson distribution. In this case, the Poisson model is too flexible and assumes more variability than there actually is in the data. This can lead to overly conservative estimates of the standard errors, making it appear as if the data are more precise than they really are. Statistical modelling methods in count analysis: Poisson regression model • The probability of roadway entity i having y crashes per some period of time. • Poisson regression models are estimated by specifying the Poisson parameter (lambda) as a function of explanatory variables X. Beta represents a vector of estimated parameters. *Parsimonious models: Given data limitations or the need to specify models with a few simplistic explanatory variables, parsimonious models are often estimated. An example would be estimating a model of crash frequency using only the volume of traffic as an explanatory variable. Clearly, many other factors affect the frequency of crashes such as environmental conditions, roadway geometric conditions, the vehicle mix of traffic, lane widths etc. This is also referred to as the issue of “omitted variable bias”. Statistical modelling methods in accident severity analysis: Multinomial logit, mixed logit, ordered logit • Multinomial logit • Mixed logit • Ordered logit Statistical modelling methods in accident severity analysis: Emerging data sources • Crash Outcomes Data Evaluation System (CODES) in the US. • Equipping cars to gather naturalistic driving data. • Vehicle’s Event Data Recorders (EDRs) (or “black boxes”). Statistical modelling methods in accident severity analysis: Examples of model estimation output Statistical modelling methods in accident severity analysis: Examples of model estimation output Statistical modelling methods in accident analysis: Macro-scale (aggregate) modelling • The model estimated by WHO, a regression model to estimate total road traffic deaths • A negative binomial regression model, appropriate for modeling non-negative integer count data (number of road traffic deaths) • N is the total road traffic deaths (for a country-year), C is a constant term, Xi are a set of explanatory covariates, Pop is the population for the country-year, and ε is the negative binomial error term. Population was used as exposure, making it possible to interpret the coefficients (βi) for the independent variables as effects on rates rather than a count. Statistical modelling methods in accident analysis: Macro-scale modelling Pedestrian crossing behaviour Pedestrian crossing behaviour A hybrid framework is proposed to analyse the pedestrians’ choice on how to cross an urban street. Stated preference experiments were presented to walkers in the zone, to evaluate their crossing behaviour in six different hypothetical choice scenarios. There were three crossing alternatives in each choice scenario • crosswalk at a signalized intersection (alternative 1) • crossing anywhere (alternative 2) • crossing over a pedestrian bridge (alternative 3) The decision process is modelled as a discrete choice model incorporating latent variables to consider perceptions and psychological factors. It was found that a longer walking distance to a pedestrian bridge or a signalized crosswalk increases the probability of direct crossing. Pedestrian crossing behaviour Pedestrian crossing behaviour • Development of pedestrian crossing choice models on the basis of road, traffic and human factors. • A field survey was carried out, in which a panel of 75 pedestrians were asked to take 8 short walking trips (each one corresponding to a different walking and crossing scenario) in the Athens city centre in Greece, allowing to record their crossing behaviour in different road and traffic conditions. • The same individuals were asked to fill in a questionnaire on their demographics, travel motivations, their mobility characteristics, selfassessment and identity, behaviour and compliance, their risk perceptions and preferences with respect to walking and road crossing, their opinion on drivers. • The modelling results showed that pedestrian crossing choices are significantly affected by road type, traffic flow and traffic control. Pedestrian perception of security Perception of security in public spaces Pedestrian perception of security • The fear of crime and perceptions of insecurity are among the most important issues with regard to a sustainability framework, relative to crime itself, in cities with low crime rates. • A safe environment has been widely accepted as one of the most important walkability predictors. However, the relation between perceived safety and walkability has not been well established. • Fear of crime and perceptions of insecurity can greatly concern the residents of a city. Pedestrian security perception in public urban areas • The use of econometric models to quantify perception. • The use of various behavioural data collections approaches to fine-tune and calibrate the model. • Factors such as neighborhood attributes, social presence, physical attributes of walkways etc. Our aim is to understand: • What determines perception of security? • How can the combined effect of those factors be integrated in a unified model? • How can perception be quantified using empirical data? Model formulation: Ordered logit model ∗ • 𝑈(𝐴𝐵−1) = 𝑉(𝐴𝐵−1) + 𝜀 𝐴𝐵−1 = 𝜷′ 𝒙(𝐴𝐵−1) + 𝜀(𝐴𝐵−1) = 𝛼1 𝑋1 + 𝛼2 𝑋2 + 𝛼3 𝑋3 + 𝛼4 𝑋4 + 𝛽1 𝑌1 +𝛽2 𝑌2 + 𝛽3 𝑌3 + 𝛽4 𝑌4 + 𝛾1 𝑍1 + ⋯ + 𝛾16 𝑍16 + 𝜀 𝑅𝑖 = 𝐼𝑛𝑠𝑒𝑐𝑢𝑟𝑒 𝑖𝑓 − ∞ < 𝑈𝑖∗ < 𝜇1 𝑅𝑖 = 𝑆𝑙𝑖𝑔ℎ𝑡𝑙𝑦 𝑖𝑛𝑠𝑒𝑐𝑢𝑟𝑒 𝑖𝑓 𝑅𝑖 = 𝑆𝑒𝑐𝑢𝑟𝑒 𝑖𝑓 𝜇1 < 𝑈𝑖∗ < 𝜇2 𝜇2 < 𝑈𝑖∗ <∞ Integration with a Digital Twin platform • Sourcing input data from the Digital Twin. • Integrating the data through Digital Twin and feeding the model. Integration with a Digital Twin platform • Implementation of the model in Digital Twin platform and visualisation at large urban scales. • Extension to all major Australian cities. • Make the platform time-dependant and dynamics (i.e., day vs night, weekend vs weekdays) • Make the platform demographic dependant (e.g., men vs women) • Turn the platform to a decision tool for problem identification (hotspot identification), scenario testing and evidence-based solution/intervention appraisal. Driving simulator sickness & experimental validity Simulator sickness • Simulator sickness is most likely caused by an incongruity of sensory input, with conflicting signals from simulated and actual motion. • Investigating the possible influence of simulator sickness on driving performance measures such as standard deviation of lateral position (SDLP),and the effect of alcohol on the degree of simulator sickness. • Twenty healthy male volunteers underwent three simulated driving trials of 1 h’s duration with a curvy rural road scenario and rated their degree of simulator sickness after each trial. • Subjects drove sober and with blood alcohol concentrations (BAC) of approx. 0.5 g/L and 0.9 g/L in a randomized order. • Simulator sickness score (SSS) did not influence the primary outcome measure SDLP. Higher SSS significantly predicted lower average speed and frequency of steering wheel reversals. • These effects seemed to be mitigated by alcohol. Higher BAC significantly predicted lower SSS, suggesting that alcohol inebriation alleviates simulator sickness. Simulator sickness • Overall, the results suggest no influence of simulator sickness on SDLP or several other driving performance measures. However, simulator sickness seems to cause test subjects to drive more carefully, with lower average speed and fewer steering wheel reversals, hampering the interpretation of these outcomes as measures of driving impairment and safety. • BAC may act as confounding variables by influencing the degree of simulator sickness in experimental studies.