Week 9 600-2023 Linkage, Genotype-Phenotype Interactions, and GWAS.pptx
Document Details
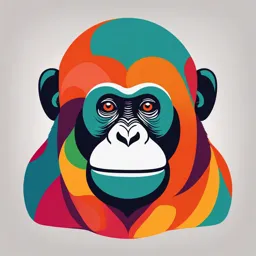
Uploaded by ReliableMookaite1890
Full Transcript
Linkage Disequilibrium, GenotypePhenotype Interactions, and GWAS Dr. Jan E. Janecka [email protected] 236 Mellon Hall Goals of Today • • • • Linkage disequilibrium & Haplotype blocks Genotype-Phenotype Associations Inheritance Models Genome Wide Association Studies (GWAS) Lesson 1 • Linkage dis...
Linkage Disequilibrium, GenotypePhenotype Interactions, and GWAS Dr. Jan E. Janecka [email protected] 236 Mellon Hall Goals of Today • • • • Linkage disequilibrium & Haplotype blocks Genotype-Phenotype Associations Inheritance Models Genome Wide Association Studies (GWAS) Lesson 1 • Linkage disequilibrium & Haplotype blocks • Genotype-Phenotype Associations • Inheritance Models Modeling genotype-phenotype interactions has many important applications… Easton et al. 2005 FGFR2 Linkage Disequilibrium (LD) Linkage Disequilibrium: Nonrandom association of alleles at two or more loci Coefficient of Linkage Disequilibrium, D: Difference between the frequency of gametes carrying the pair of alleles A and B at two loci (pAB) and the product of the frequencies of those alleles (pA and pB). D = pAB – pApB See the posted Slatkin 2008 review Coefficient of Linkage Disequilibrium : Measures to what extent two alleles on DIFFERENT LOCI are associated together, relative to that expected by chance Where does LD on chromosomes come from? The closer two loci (locus A and locus B) are on a chromosome: • the less likely there will be a cross over between them • the more likely their alleles will occur together on a chromosome The ramification: If two loci are very close to each other on a chromosome, then they will nearly always have their alleles inherited together, forming what are called “Haplotype” Linkage Block • Most chromosomes are divided into segments with strong LD called linkage blocks. • Within a linkage block, alleles on all loci are highly correlated • A linkage block with a unique set of alleles all ways together is referred to as a haplotype • For each linkage block, there are relatively small numbers of haplotypes in one population Haplotype Blocks Most genomes are partitioned into block-like Three linkage blocks, of eachLD with very low patterns • recombination rates • • Alleles on the loci within each linkage block are associated together forming haplotypes Human chromosomes have linkage blocks from 100s to 100,000s bp long Class II MHC complex region in Drosophila, See Slatkin 2008 Linkage Block • Haplotypes are identified by genotyping single nucleotide polymorphisms (SNPs) • A few representative TAG SNPs can be genotyped to capture the common haplotypes present in populations • TAG SNP – single nucleotide polymorphism correlated with all variants in that linkage block Linkage blocks Haplotype 1 Tag SNPs A, B, C, D a B A b C C d d Haplotype 2 Linkage Block • Genetic linkage is the association of alleles from different loci because they are on same linkage block How can you go from association between loci (different genes) to the between genes and physical traits? Genotype-Phenotype Association Genotype-Phenotype Association Case 1 This allele causes the phenotype, so if you genotype it, there will be a direct association Case 2 This allele does not cause the disease, but it is close to three alleles that do, and so if you genotype it there will be an indirect association Linkage block Hirschhorn & Daly 2005 Indirect association caused by linkage disequilibrium Inheritance Patterns and Associations • What else will affect how strong an association there is between the genotype and phenotype? example: Locus affecting size If “a” is recessive: AA – normal Aa – normal aa - big A – wild type allele, normal size a – mutant allele, big size If “a” is dominant: AA – normal Aa – big aa - big If “a” is incomplete dominant: AA – normal Aa – medium aa - big Heritability of will affect the expectations for testing the significance of associations between the genotype and phenotype Inheritance Models • Penetrance = risk of disease in a given individual ( ) • For GWAS, models are needed to specify the expected relationship between genotype and phenotype Inheritance Models Assuming genetic penetrance parameter and biallelic locus with alleles “A” and “a” • Multiplicative model • Disease risk is increased -fold with each additional A allele • Additive model • Risk of disease is increased -fold for genotype a/A and by 2 -fold for genotype A/A • Recessive model • Two copies of allele are required for -fold increase in disease risk • Common dominant model • Either one or two copies of allele A are required for a -fold increase in disease risk • Polygenic model (complex traits) • Numerous alleles and genes and contribute small amounts to disease risk Inheritance Models Expressed Mathematically Shown are disease penetrance functions for genotypes a/a, A/a and A/A and associated relative risks for genotypes A/a and A/a compared with baseline genotype a/a for standard disease models when baseline disease penetrance associated with genotype a/a is f0 = 0 and genetic penetrance parameter is γ> 19. Two Common Approaches to Find Gene • Linkage Mapping • Genes mapped by typing markers in families with diseases/trait values within pedigrees • In any family, disease alleles will be within 1020 cM of marker (cM % recombination) • Markers are spaced every 10 cM (10 Mb) • Mendelian inherited trait – a carrier passes on disease to half of his/her offspring • GWAS • Variants are genotyped across genome and compared to phenotype information • Correlations between alleles and disease are “associations” • A genetic map is used to then identify causal genes in LD with significant SNPs in region Genome Wide Association Studies (GWAS) • Goal is to map causal mutation(s) to chromosomes • What is the genetic contribution to the phenotype? • For many complex phenotypes and diseases any one locus has only a modest contribution • Quantitative traits – cumulative action of many genes and the environment • There are many challenges • • • • Power Comprehensiveness Interpretation Analysis Activity 6 – GWAS in Medical News 18 Lesson 2 • Genome Wide Association Studies (GWAS) • Study design • Odds ratios and significance • Minimizing false positives & negatives • Follow up studies • Case study on cancer GWAS GWAS: An association study that surveys most of the genome for causal genetic variants Advantages: • Can be applied to quantitative and complex traits • Very dense SNP assays now available that covers entire genome (mostly) • Relatively inexpensive because you still take advantage of linkage so do not need to sequence all variants Do not need prior information on pathways/genes that affect phenotype GWAS Became feasible in last 10 years • In 2005 dbSNP already had 9 million SNPs in human populations, out of an estimated 11 million • LD pattern described during human HapMap project enabled selection of most informative markers in linkage blocks Relatively inexpensive - Do not need to genotype all variants because of linkage Haplotype Blocks Haplotypes blocks used to find genes affecting phenotypes • Not necessary to sample all loci in each haplotype block to find significant associations • Enables large-scale Genome Wide Associations Studies (GWAS) Sequence Identify Identify SNPs in SNPs in haplotype haplotype blocks blocks Sample Sample SNPs representative representative SNPs in affected and in affectedgroups and unaffected unaffected groups Sequence genes in that genes in that haplotype haplotype block to find block to find causal causal mutation mutation Study Design • SNP Assays • Currently there are human SNP chips that assay >1 million SNPs • Need to include some low frequency SNPs (1-5%) as these are the ones that likely contribute proportionately more to diseases • Population samples • Need to have SNP assay developed for the population you are testing • Important to repeat GWAS with another set of samples • Subset can be retested with a denser SNP assay Challenges: Study Design • Genes with modest effects require genotyping of thousands of individuals • Must correct for multiple-hypothesis testing because each SNP is an independent test − For 1 million SNP assay, if you use p = 0.05, then there will be 50,000 significant SNPs! Bonferroni correction n = # of independent tests pcorrected = 1 – (1-puncorrected)n simplifies to: pcorrected = (puncorrected)/n What would be the correct P-value for a GWAS test with 1 million SNPs? P = 0.05/106 = 5 x 10 -8 Study Design • Need to genotype lots of individuals to have power Example: • allele frequency = 15% • odds ratio = 1.25 (similar to PPARG for type 2 diabetes) For 80% statistical power using 500,000 SNP assay need 6,000 cases and 6,000 controls for pcorrected = 1.0 x 10-7 A multistage approach can reduce genotyping while maintaining power Bonferroni punitively conservative Permutation to find more appropriate pvalue threshold Odds Ratio • Measure of effect size, or the strength of association odds = P / (1 - P) where P = probability of event Example: • Probability of Pens winning is 50% odds = 0.5 / (1 – 0.5) = 1 (that is 50:50 or “even money”) • Probability of Pens winning given Sydney Crosby starts is 75% odds = 0.75 / (1 – 0.75) = 3 odd ratios = odds ratio for Pens win with Crosby, compared to without him odds(event | exposure) odds(event | lack of exposure) odds (win | Crosby) odds 3 = = 3 = (win | no Crosby) 1 Disease Example: Odds Ratio • 80% of people with “A” genotype have disease odds = 0.8 / (1 – 0.8) = 4 • 20% of people with “T” genotype have disease odds = 0.2 / (1 – 0.2) = 0.4 odds ratio for disease given “A” compared to “T” genotype odds (D | A genotype) 4 = 16 = = odds (D| T genotype) 0.4 Disease risk in population is 25% What is the odds ratio of “A” individuals relative to the general population? Odds (D| general pop) = 0.25/(1-0.25) = 0.25/0.75 = 1/3 odds ratio for disease given “A” compared to general pop odds (D| A genotype) 4 = = 12 = odds (D| general pop) 1/3 A multi-stage approach can reduce genotyping cost while maintaining power Permutation to find more appropriate pvalue threshold Avoiding False Positives • Random effects that result in false low p-values • Can be reduced by: • Multi-stage population analysis • Permutation testing to find best p-value threshold Other sources of error: • Systematic bias in study design • Population stratification due to admixture • Technical artifacts • Cases and controls not genotyped together • Missing data if particular genotypes such as heterozygotes are more likely not to be scored Population Stratification • Different ethnic groups have different disease prevalence and allele frequencies • Grouping ethnic subgroups in one population creates a stratified population via admixture • Admixture – combining two or more populations with different alleles frequencies into one group • If one ethnic group has greater incidence of a disease, and stratification is not taken into account, all alleles that have higher frequencies in that population will be in association with the disease Critical to take population structure into account when doing GWAS! Population Stratification • Many more false positives with greater population structure = index of divergence between subgroups Finding the Causal Mutation • Most often the rational is that missense mutations that disrupt proteins are the causal mutations • This is more often the case in severe, and rare, Mendelian inherited disease • Most complex diseases have numerous subtle mutations that only slightly change the protein or expression • Regulatory changes can be just as important Example: Autoimmune disease • Missense variant Thr17Ala in CTLA4 association with disease • Non-coding variant in regulatory region Genomics Revolution and GWAS • Hirschorn & Daly 2005 Nature Genetics Review page 105: “No truly genome-wide association study has yet been carried out..” 2 years later... Easton et al. 2007 ... “we conducted a two-stage genome-wide association study in 4,398 breast cancer cases and 4,316 controls, followed by a third stage in which 30 single nucleotide polymorphisms (SNPs) were tested for confirmation in 21,860 cases and 22,578 controls from 22 studies...” Genotyped total of 227,876 SNPs GWAS for novel genes linked to breast cancer • Easton et al. 2007 266,722 TAG SNPs genotyped in 408 cases and 400 controls 12,711 SNPs in 3,990 cases and 3,916 controls 30 SNPs in 21,860 cases and 22,578 controls for 22 different case-control studies GWAS for novel genes linked to breast cancer Note that Odds ratio of 1.25 is only about 25% relative difference in getting the disease… if 1 in 2,500 get it then it is the difference 0.0005% vs 0.0004% GWAS for novel genes linked to breast cancer FGFR2 SNP Finding the Causal Mutation(s) Fibroblast growth factor receptor 2 • Re-sequenced region in linkage block that contained the FGFR2 for 45 individuals • Additional variants analyzed in Asian and European populations Fine resolution mapping found linkage block with exon 2 and intron 2 that had likely causal mutations Original significant SNP FGFR2 Finding the Causal Mutation(s) • All likely causative mutations (red) are in introns Original • 4 SNPs in region conserved across significant mammals suggesting regulatory effects SNP Genomics Revolution and GWAS • Hirschorn & Daly Nature Genetics Review page 106 “note that the most comprehensive approach towards understanding complex disease would be complete genome resequencing in a large population of cases and controls carried out ... Unfortunately, this approach is not close to becoming feasible.” 12 years later... Nik-Zainal et al. Today… 2016 ... “We analyzed whole-genome sequences of 560 breast cancers to advance understanding of the driver mutations conferring clonal advantage and the mutational processes generating somatic mutation...” Review – Week 9 Linkage Disequilibrium, GenotypePhenotype Interactions, and GWAS Main Concepts Lesson 1 • The way linkage disequilibrium leads to haplotype blocks • How and why phenotypes are associated with genotypes • Modeling inheritance patterns Lesson 2 • How GWAS studies performed and interpreted • Ways to decrease error in GWAS studies • Follow up studies to understand function • Cancer case study (Easton et al. 2007) Review – Week 9 Linkage Disequilibrium, GenotypePhenotype Interactions, and GWAS Main Terms Lesson 1 • Linkage map, cM, Linkage Disequilibrium, Haplotype block, Coefficient of Linkage Disequilibrium (D), TAG SNP • Genotype-Phenotype Association • Ineritance models (Multiplicative, Additive, Recessive, Common dominant, Polygenic) Lesson 2 • GWAS, quantitative traits • Bonferroni correction, Power, Odds ratio • Multi-stage approach, permutation, false positives • Population stratification, admixture