Week 8 lecture slides.pptx
Document Details
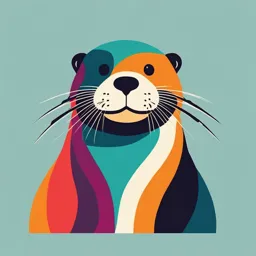
Uploaded by GroundbreakingEinsteinium6432
Taylor's University College
Tags
Full Transcript
Analyses in Psychological Testing PSY61204 Psychological Tests and Measurements Dr Michele Anne Overview Correlation Factor analysis Correlation Correlation Association between two variables or items Normality analysis must be carried out first to determine whether Pearson (normally di...
Analyses in Psychological Testing PSY61204 Psychological Tests and Measurements Dr Michele Anne Overview Correlation Factor analysis Correlation Correlation Association between two variables or items Normality analysis must be carried out first to determine whether Pearson (normally distributed) or Spearman (not normally distributed) correlation is more suitable Can be used for test-retest reliability, parallel forms reliability, criterion validity, and construct validity Analysis – Test of Normality Analyse Descriptive Statistics Explore Analysis – Test of Normality (cont.) Put all variables in “Dependent List” Click “Plots”. Tick “Normality plots with tests”. Click “continue”. Click “OK” Output Interpretation Kolmogorov-Smirnov test not significant (p >.05) data is normally distributed Use parametric test Kolmogorov-Smirnov test significant (p <.05) data is not normally distributed Use non-parametric test Analysis - Correlation Analyze Correlate Bivariate Analysis (cont.) Put variables in “Variables” Tick either “Pearson” or “Spearman” for “correlation coefficient” Click “OK” Output Interpretation Compare correlation coefficient to table below to get indication of strength of relation, and hence, reliability and validity Reliability analysis Reliability analysis can be carried out for internal consistency To get Cronbach’s alpha value Splits items in half and correlates them Analysis – Reliability analysis Analyze Scale Reliability analysis Analysis (cont.) Put items in “Items”. Keep “Model” as “Alpha” Tick either “Correlations” under “Inter-item” Click “OK” Output Interpretation Compare the Cronbach alpha value to the table below to determine the internal consistency reliability Factor analysis What is a Factor Analysis? Method of extracting factors which account for correlations between several variables (e.g., items on psychological scales, experimental tests) Uses patterns of test or sub-test correlations to provide support for theoretical constructs by locating correlational ‘clusters’ and identifying explanatory factors An approach which seeks to reduce a matrix of correlations between numerous items to a smaller set of ‘factors’ which best explain the variability amongst the original correlations. Exploratory vs Confirmatory Factor Analysis Exploratory factor analysis (EFA) Confirmatory factor analysis (CFA) Use of factor analysis to identify Factor analysis performed to latent (underlying) factors which support an already explain the variance in identified factor structure correlations Performed in a conceptual area Might be with a larger where there is as yet no known data set or on a different well-supported factor structure population from the original Principal Component Analysis Principal components analysis (PCA) is a variable reduction technique which maximizes the amount of variance accounted for in the observed variables by a smaller group of variables called COMPONENTS Can use PCA to reduce the number of questions by identifying and removing redundant questions The PCA process allows us to reduce the number of questions or variables down to their PRINCIPAL COMPONENTS Analysis – Factor analysis Analyze Dimension reduction Factor Analysis (cont.) Put items in “Variables” Under “Descriptives”, tick “Coefficients” and “KMO and Bartlett’s test of sphericity” Analysis (cont.) Under “Extraction”, select “Principle components” and tick “Correlation matrix”, “Unrotated factor solution” and “Scree Plot” Under “Rotation”, tick “Direct Oblimin” Analysis (cont.) Under “Options”, tick “Exclude cases pairwise”, “Sorted by size” and “Supress small coefficients”. Put in.3 for the “Absolute value below” Click “OK” Output Interpretation If KMO value is.6 and above and Barlett’s test of sphricity is significant, factor analysis is valid enough to be interpreted [KMO and Barlett’s test] Having most correlations of.3 and above between the items indicate that items are related sufficiently [Correlation matrix] Number of components with eigenvalues of 1 indicate number of factors of components present [Total variance explained]. This information can be further corroborated via the Screeplot [Screeplot] Interpretation (cont.) Where most items fall under suggest how many strong factors exist [Component matrix] Items should be included under factors where they have higher loadings. Only loadings of more than.3 will be shown – any less than that suggest the item doesn’t group together strongly in that factor [Pattern matrix] Can rerun the factor analysis is number of factors have been set Questions?