Week 8 Lecture - Part 1 PDF
Document Details
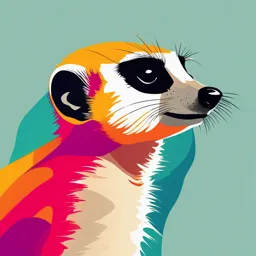
Uploaded by FinestPlatypus
UNSW Sydney
Milad Haghani
Tags
Summary
This lecture details human factors in civil and transport engineering, specifically focusing on built environments, crowds, and emergencies. It covers various aspects of road safety, including economic, cognitive, mathematical, clinical, neuro-cognitive, social science, social psychological, and philosophical aspects, along with micro-mobility.
Full Transcript
CVEN4405 Human Factors in Civil and Transport Engineering Week 8 Lecture – Part 1 Human factors in built environments, crowds and emergencies Dr Milad Haghani School of Civil and Environmental Engineering Economic aspects of road safety Cognitive aspects of road safety Mathematical modelling of...
CVEN4405 Human Factors in Civil and Transport Engineering Week 8 Lecture – Part 1 Human factors in built environments, crowds and emergencies Dr Milad Haghani School of Civil and Environmental Engineering Economic aspects of road safety Cognitive aspects of road safety Mathematical modelling of road safety Clinical and epidemiological aspects of road safety Neuro-cognitive aspects of road safety Social science of road safety Social psychological aspects of road safety Philosophical aspects of road safety Micro-mobility aspects of road safety Week 8: Human factors in built environments, crowds and emergencies Why do we study crowd safety Just in the past 20 (up until 2019) years alone around 8000 people have been killed in crowd accidents and more than 15,000 have been injured. https://newsroom.unsw.edu.au/news/science-tech/travelling-overseas-map-shows-hot-spot-areas-deadly-crowd-accidents https://www.theweek.in/news/sci-tech/2023/05/18/india-increasingly-becoming-hot-spot-for-crowd-accidents--study.html https://www.linkedin.com/pulse/road-vision-zero-crowd-accidents-goes-through-low-milad-haghani%3FtrackingId=nvpOdh1TSH2XML3xac5iMA%253D%253D/?trackingId=nvpOdh1TSH2XML3xac5iMA%3D%3D https://www.linkedin.com/pulse/superstar-drawn-spontaneous-crowds-lesser-explored-crowd-haghani%3FtrackingId=m1pxqPSxQQaBEkzzzNcpfw%253D%253D/?trackingId=m1pxqPSxQQaBEkzzzNcpfw%3D%3D https://www.linkedin.com/pulse/safeguarding-mass-gatherings-era-severe-weather-events-milad-haghani%3FtrackingId=dGdCHZlyRVCMM8at0oRMxQ%253D%253D/?trackingId=dGdCHZlyRVCMM8at0oRMxQ%3D%3D https://www.linkedin.com/pulse/quantifying-cost-safety-pedestrian-heavy-urban-spaces-milad-haghani%3FtrackingId=hOcOCbeaQvyaPGMU8FU58g%253D%253D/?trackingId=hOcOCbeaQvyaPGMU8FU58g%3D%3D Knowledge gaps in scholarly science on terrorism prevention and response Risks to crowd safety Intrinsic Risks Extrinsic Risks Overcrowding & pushing Environmental hazards Crowd crushes Extreme weather Stampede Fire Crowd violence Terror attacks False alarm (fireworks) Yorkdale Mall, Canada NYE gate breach London, UK False alarm (suicide) La Defense Mall, Paris NYE gate breach London, UK False alarm (suicide) La Defense Mall, Paris Pitch invasion Melbourne, Australia Fire in a Bar Birmingham, UK Crowd stampede/crush Religious event, India FIFA WC Live Screening Melbourne, Australia Bomb attack in street mall Istanbul, Turkey NYE gate breach London, UK Rock concert Pedestrian Dynamics as a research domain Why laboratory experiments? Economic Experiment A Health Economics Experiment Decision-making or Social Interactions Psychology Experiment Developmental Psychology Experiment Driving Simulator Experiment Why study crowd behaviour in controlled laboratory settings? An empirically informed modelling framework Decision-making layers Econometric models Locomotion layers Models from statistical mechanics, physics and transportation The conceptual framework Behavioural experiments Human response prediction Intervention & optimisation Cox-proportional Weibull Hazard duration model for pre-evacuation time i • When an evacuation signal is perceived, not all individuals react to it at the same time. • The time between the onset of an evacuation and the decisive movement of an evacuee is called pre-evacuation or pre-movement or reaction time. • Probabilistic Hazard duration models are suitable for this purpose. • Empirical evidence shows that reaction time is correlated with the spatial position of the evacuee. ln 𝑢 𝑇𝑖 = − 𝜆 exp 𝜇 × (𝑀𝑖𝑛_𝐸𝑥𝑖𝑡_𝐷𝐼𝑆𝑇𝒊 No need to memorise any equation! Calibration parameters 1/𝜈 Hazard duration models 𝑇 = 𝐻0−1 (− ln 𝑢 exp( − 𝜷′ 𝒙)) Distribution Characteristics Exponential Weibull Scale parameter 𝜆 > 0 Parameter Scale parameter 𝜆 > 0 Range [0, +∞) [0, +∞) Hazard function ℎ0 (𝑡) = 𝜆 ℎ0 (𝑡) = 𝜆𝜈𝑡 𝜈 −1 Cumulative hazard function 𝐻0 (𝑡) = 𝜆𝑡 𝐻0 (𝑡) = 𝜆𝑡 𝜈 Inverse cumulative hazard function 𝐻0−1 (𝑡) = 𝜆−1 𝑡 𝐻0−1 (𝑡) = (𝜆−1 𝑡)1/𝜈 Density function 𝑓0 (𝑡) = 𝜆 exp(−𝜆𝑡) 𝑓0 (𝑡) = 𝜆𝜈𝑡 𝜈−1 exp(−𝜆𝑡 𝜈 ) Survival function 𝑆0 (𝑡) = exp(−𝜆𝑡) 𝑆0 (𝑡) = exp(−𝜆𝑡 𝜈 ) Mean 𝜆−1 Variance 𝜆−2 Shape parameter 𝜈 > 0 𝜆 𝜆 2 − 𝜈 Γ 1 − 𝜈 1 Γ( + 1) ν 2 1 + 1 − Γ2 + 1 𝜈 𝜈 Model Characteristics Cox-exponential Survival time Hazard function 𝑇=− ln 𝑢 𝜆 exp(𝜷′ 𝒙) ℎ(𝑡 𝒙) = 𝜆 exp(𝜷′ 𝒙) Cox-Weibull ln 𝑢 𝑇= − 𝜆 exp(𝜷′ 𝒙) 1/𝜈 ℎ(𝑡 𝒙) = 𝜆 exp(𝜷′ 𝒙) 𝜈𝑡 𝜈 −1 Reaction time & speed heterogeneity in a real-life situation https://twitter.com/Milad_Haghani/status/1591924578917711872?s=20&t=_M0MADNIbXl5YUv9-RRnkA https://twitter.com/Milad_Haghani/status/1591917514052997120?s=20&t=_M0MADNIbXl5YUv9-RRnkA https://twitter.com/Milad_Haghani/status/1591914942403588097?s=20&t=_M0MADNIbXl5YUv9-RRnkA https://twitter.com/Milad_Haghani/status/1591911944596115456?s=20&t=_M0MADNIbXl5YUv9-RRnkA An empirically informed modelling framework Decision-making layers Econometric models Locomotion layers Models from statistical mechanics, physics and transportation An empirically informed modelling framework – Utility functions VExit i = β1· Distance + β2· Congestion + β3· Flow · Visibility + β4· Flow · (1-Visibility) + β5· Visibility – Probability of choosing an exit i PExit i = exp (VExit i ) exp (V ) Exit j j Hypothetical choice experiments (stated choice survey) Remember these surveys from the Value of Life lecture? Field type (lab-in-the-field) experiments Field type (lab-in-the-field) experiments Field type (lab-in-the-field) experiments Field type (lab-in-the-field) experiments Field type (lab-in-the-field) experiments Field type (lab-in-the-field) experiments Field type (lab-in-the-field) experiments How a choice data set looks like How do we estimate model parameters? The concept of (log-)likelihood function optimisation 𝐿 = 𝑃1𝑗1 × 𝑃2𝑗2 × ⋯ × 𝑃𝑛𝑗𝑛 × ⋯ × 𝑃𝑁𝑗𝑁 exp 𝑉𝑗1 𝐿= σ𝑖 exp 𝑉𝑖 exp 𝑉𝑗2 × σ𝑖 exp 𝑉𝑖 exp 𝑉𝑗𝑛 ×⋯× σ𝑖 exp 𝑉𝑖 Sample exp 𝑉𝑗𝑁 ×⋯× σ𝑖 exp 𝑉𝑖 How do we estimate model parameters? The concept of (log-)likelihood function optimisation 𝐿 = 𝑃1𝑗1 × 𝑃2𝑗2 × ⋯ × 𝑃𝑛𝑗𝑛 × ⋯ × 𝑃𝑁𝑗𝑁 exp 𝑉𝑗1 𝐿= σ𝑖 exp 𝑉𝑖 exp 𝑉𝑗2 × σ𝑖 exp 𝑉𝑖 exp 𝑉𝑗𝑛 ×⋯× σ𝑖 exp 𝑉𝑖 exp 𝑉𝑗𝑁 ×⋯× σ𝑖 exp 𝑉𝑖 Estimated exit choice model Interpreting the coefficient estimates The stated choice survey The revealed choice experiment Can we learn crowd behaviour from surveys? Are experimental results generalisable? Other lab-in-the-field experiments How people make exit decisions How people make decisions within social groups (reaction decision, exit choice etc) How people make a decision to change their decision Other lab-in-the-field experiments How people decisionmaking is affected by observing peer behaviour How people decisionmaking is affected by elevated level of stress/urgency Other lab-in-the-field experiments How crowd behaves at bottlenecks How people resolve conflicts while on the move An empirically informed modelling framework Decision-making layers Econometric models Locomotion layers Models from statistical mechanics, physics and transportation Exit choice changing Exit choice changing model Exit choice changing model 𝑈𝑐ℎ𝑎𝑛𝑔𝑒 = 𝑉𝑐ℎ𝑎𝑛𝑔𝑒 + 𝜀𝑐ℎ𝑎𝑛𝑔𝑒 = 𝐼𝑛𝑒𝑟𝑡𝑖𝑎 + 𝛽1 𝐶𝑂𝑁𝐺𝑛 − 𝐶𝑂𝑁𝐺𝑚𝑖𝑛 + 𝛽2 𝑉𝐼𝑆 + 𝛽3 𝑆𝑜𝑐𝐼𝑛𝑓 + 𝜀𝑐ℎ𝑎𝑛𝑔𝑒 𝐶𝑂𝑁𝐺𝑛 =𝐶𝑜𝑛𝑔𝑅𝑎𝑡𝑖𝑜 𝑃𝑐ℎ𝑎𝑛𝑔𝑒 = 1 1 + 𝑒 −𝑉𝑐ℎ𝑎𝑛𝑔𝑒 Let’s practice the model 𝑈𝑐ℎ𝑎𝑛𝑔𝑒 = 𝑉𝑐ℎ𝑎𝑛𝑔𝑒 + 𝜀𝑐ℎ𝑎𝑛𝑔𝑒 = 𝐼𝑛𝑒𝑟𝑡𝑖𝑎 + 𝛽1 𝐶𝑂𝑁𝐺𝑛 − 𝐶𝑂𝑁𝐺𝑚𝑖𝑛 + 𝛽2 𝑉𝐼𝑆 + 𝛽3 𝑆𝑜𝑐𝐼𝑛𝑓 + 𝜀𝑐ℎ𝑎𝑛𝑔𝑒 𝐶𝑂𝑁𝐺𝑛 =𝐶𝑜𝑛𝑔𝑅𝑎𝑡𝑖𝑜 𝑃𝑐ℎ𝑎𝑛𝑔𝑒 = 1 1 + 𝑒 −𝑉𝑐ℎ𝑎𝑛𝑔𝑒 Calibrating the exit choice changing model Exit choice changing experiment 1 2 3 4 Decision change observation Calibrating the exit choice changing model Estimation outcome Table 6 Binary logit estimates for exit choice change models. The (***) and (**) signs indicate statistical significance at 99% and 95% confidence levels respectively. Exit choice change model I Parameter estimates Inertia CongRatio SocInf Vis Goodness-of-fit measures Log-likelihood at estimates Initial Log-likelihood value Log-likelihood ratio No. of observations Estimates Standard T-stat Error P value 95% confidence interval -3.382*** 2.144*** 1.419*** 0.127 0.343 0.790 0.355 0.423 0.000 0.007 0.000 0.764 -4.054 0.595 0.723 -0.701 Standard T-stat Error P value 95% confidence interval 0.304 0.716 1.368 0.356 0.000 0.001 0.339 0.000 -3.931 0.999 -1.373 0.713 -2.709 3.694 2.116 0.955 -116.12 -282.80 0.589 408 Exit choice change model II Estimates Parameter estimates Inertia CongRatio_Vis CongRatio_Invis SocInf Goodness-of-fit measures Log-likelihood at estimates Initial Log-likelihood value Log-likelihood ratio No. of observations -9.850 2.710 3.990 0.300 -3.336*** 2.403*** 1.308 1.411*** -115.87 -282.80 0.590 408 -10.990 3.350 0.960 3.960 -2.741 3.807 3.989 2.109