Week 13 Test for Significance Epidemiology PDF
Document Details
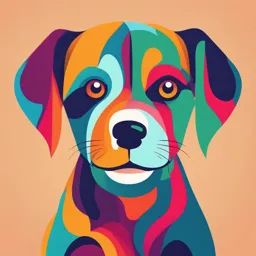
Uploaded by AdjustableZinnia
University of Namibia
2024
N. Kakehongo
Tags
Summary
This document provides a detailed overview of epidemiology concepts including testing for significance, population and sample considerations, and hypothesis testing. The document features various illustrative examples and tables.
Full Transcript
Course : Epidemiology Topic : Testing for significance N. Kakehongo April, 2024 Learning outcome After completing this session, the student should be able to: Describe test of significance Describe steps of significance testing 1st an overview of...
Course : Epidemiology Topic : Testing for significance N. Kakehongo April, 2024 Learning outcome After completing this session, the student should be able to: Describe test of significance Describe steps of significance testing 1st an overview of Population & Sample Population & Sample Population: consists of the totality of the observations with which we are concerned. Sample: a subset of a population. Sample Population Parameter and Statistic Parameter: some measurable characteristic of a population, such as a mean or a variance Statistic: a measurable characteristic of the sample The idea of statistical inference Generalisation to the population uncertainty Conclusions based on the sample Study population Sample randomly drawn What is Statistical Inference? The process of drawing conclusions about population parameters based on a sample taken from the population. Is the attempt to reach a conclusion about a population based on observations from a sample. Population Statistics Sample What is Statistical Inference? What can I say about the value of a single population parameter based on a sample? Probability Data Truth Statistical Inference What is statistical testing? Also called “hypothesis testing” … the process of inferring from your data whether an observed difference is likely to represent chance variation or a real difference NB: Does NOT address bias, confounding, or investigator error! Influenced by: Size of difference in results between groups Number of subjects or observations in study Test for significance Answer an important question- is difference observed likely real or chance? A statistical procedure-compares the data of a sample with a hypothesis about the parameter of the population Results are expressed in terms of a probability First let us Understanding Hypothesis Testing Understanding Hypothesis Testing A person is on trial for a criminal offense and the judge needs to provide a verdict on his case. Now, there are four possible combinations in such a case: First Case: The person is innocent and the judge identifies the person as innocent Second Case: The person is innocent and the judge identifies the person as guilty Third Case: The person is guilty and the judge identifies the person as innocent Fourth Case The person is guilty and the judge identifies the person as guilty Understanding Hypothesis Testing Understanding Hypothesis Testing As you can clearly see, there can be two types of error in the judgment o Type 1 error, when the verdict is against the person while he was innocent o Type 2 error, when the verdict is in favor of person while he was guilty According to the presumption of innocence, the person is considered innocent until proven guilty. That means the judge must find the evidence which convinces him “beyond a reasonable doubt” This phenomenon of “Beyond a reasonable doubt” can be understood as Probability (Judge Decided Guilty | Person is Innocent) should be small The basic concepts of Hypothesis Testing are actually quite analogous to this situation. Understanding Hypothesis Testing We consider the Null Hypothesis to be true until we find strong evidence against it, then, we accept the Alternate Hypothesis We also determine the Significance Level (⍺) which can be understood as the Probability of (Judge Decided Guilty, Person is Innocent) in the previous example Thus, if ⍺ is smaller, it will require more evidence to reject the Null Hypothesis Hypothesis to be Tested “Any conjecture cast in a form that will allow it to be tested and refuted.” (Last, J. A Dictionary of Epidemiology) Example from Criminal Justice Hypothesis – “innocent until proven guilty” Prosecutor goal - refute hypothesis of innocence In statistical inference Hypothesis - “no real difference exists” Investigator – refute hypothesis of no difference Stating a hypothesis Idea: X exposure might be associated with Y out come State null hypothesis (H0): there is no association between X and Y (Innocent until proven guilty) Do the study, and perform the statistical test: ✓Find statistical association-’reject the null hypothesis, so accept alternative, that X is associated with Y ✓Find no statistical association-’fail to reject the null hypothesis, can not say that X is associated with Y Null Hypothesis (H0) A statement about a parameter in the population that is being tested by the test of significance worded so as to imply that there is no relationship, no effect, or no difference. Examples of H0 : The observed difference is not real, or the observed difference is the result of chance.” H0: The observed difference in HIV positivity between drug users and non-users is due to chance Alternative Hypothesis (HA) A statement of the “hoped for” effect Examples of HA: H0 is not true, i.e., the observed difference is not due to chance HA: The observed difference in HIV positivity between drug users and non-users is NOT due to chance Hypothesis test: Hypothesis test: Upper-tailed Lower-tailed Two-tailed The research hypothesis form depends on the investigator's belief about the parameter of interest The alternative hypothesis can take one of 3 forms Based on believing that the parameter has increased, decreased or changed Which results in these hypothesizes: H1 : μ > μ0 upper-tailed test H1 : μ < μ0 lower-tailed test H1 : μ ≠ μ 0 two-tailed test Upper-tailed, Lower-tailed, Two-tailed Tests Hypothesis test: Upper-tailed, Lower-tailed, Two-tailed Tests Hypothesis testing based on the population parameter of interest For example: The average weight of soccer players H0 : µ = 80.0kg HA: µ ≠ 80.0 kg HA: µ > 80.0kg or µ < = 80.0kg The hypothesis need to be tested Hypothesis testing Hypothesis testing seeks to determine whether there is enough evidence to reject the null hypothesis in favor of the alternative Test statistics: Test statistic is a number that summarizes the sample information Depends on: Type of measurements, Whether the data is normally distributed/skewed Small sample (t-test), bigger (z-test) Steps of Significance Testing There are steps to perform Hypothesis Testing: State the null hypothesis State the alternative hypothesis Choose a statistical test for testing the null hypothesis Specify a significance level, criterial for decision Perform the statistical test (and compute a P-value) based on the data Make a decision about the hypotheses 1. State the Null and Alternative Hypotheses Null hypothesis H0: The observed difference is not real, i.e., the observed difference is the result of chance Alternative hypothesis HA: H0 is not true, i.e., the observed difference is not due to chance Example: State H0 and HA – Wedding Cake Study Wedding attendees Attack rate, cake+ = 254 / 411 = 61.8% Attack rate, cake− = 33 / 223 = 14.8% H0: the attack rates in the two groups are the same (RR=1) HA: the attack rates in the two groups are not the same (RR ≠ 1), or HA: those who ate cake had higher attack rate (RR > 1) Investigation: Gastroenteritis after a Wedding Oswego Data Ill Not ill Total AR Ate Y 43 11 54 79.6% vanilla ice cream? N 3 18 21 14.3% 46 29 75 2. Choosing a Statistical Test Choice depends on: Study design measurement scale of the variables Study size (z or t) (In general, for small sample sizes (under 30) or when you don’t know the population standard deviation, use a t-score, otherwise, use a z-score) Test for comparison of 2 means: Student t-test Test for 2-x-2 table data: Chi-square test Types of Statistical Tests Parametric tests: Continuous data; summarized with means Sampled from normally distributed populations Nonparametric tests: Data is continuous but population is probably NOT normally distributed, and sample size < about 30; medians For samples of data where variables are NOT continuous (e.g. ranked data) Chi-square tests: For comparing proportions (e.g. attack rates) Dichotomous variables: ill/well, case/control, exposed/not 2. Choosing a Statistical Test (continued) Test Used for Mann-Whitney U Test Comparison of two medians (Wilcoxon rank-sum test) (ranks) Paired Student T Test Parametric comparison of two linked values Wilcoxon signed-rank test Nonparametric comparison of two linked values F Test Comparison of several means McNemar Test Matched 2-by-2 table Statistical Tests for a 2-by-2 Table Fisher Exact Test use when any expected value < 5 Chi-square Test use when all expected values > 5 4 variations – Uncorrected – Mantel-Haenszel uncorrected – Yates corrected – Mantel-Haenszel corrected Examples of Parametric Tests Name of Test Purpose of Test Underlying Probability Distribution One-sample t test Compare mean of one Normal Distribution group to a hypothetical value Unpaired t test Compare means of Normal Distribution two unpaired groups Paired t test Compare means of Normal Distribution two paired groups One-way ANOVA Compare 3 or more Normal Distribution unmatched groups Repeated-measures Compare 3 or more ANOVA matched groups (adapted from Intuitive Biostatistics) Choose a Nonparametric Test When Data are continuous but not normally distributed Outcome of interest is a rank or score, e.g. in ranking movies or restaurants using 1-4 stars (*, ****) Some values of a variable are “off the scale,” e.g. too low or too high to measure (assign arbitrary very low or very high values) If sample is large, nonparametric tests still work well even if data is normally distributed Use a Non-parametric Test When Data Is Not Normally Distributed Continuous variable Skewed Non-continuous variable Cannot assume a “normal” distribution Negative (Left) Skew Undefined distribution Tests to compare means cannot rely on normality Positive (Right) Skew Nonparametric Tests - Examples Name of Test Purpose of Test Based on rank or score, not the normal distribution Wilcoxon test Compare one group to a hypothetical value Mann-Whitney test Compare two unpaired groups Wilcoxon test Compare two paired groups Kruskal-Wallis test Compare 3 or more unmatched groups Friedman test Compare 3 or more matched groups (adapted from Intuitive Biostatistics) Challenging Situation Sample size is small (< 30), unknown whether population is normal Parametric tests may generate inaccurate P-values when distribution is not normal and therefore are not as robust as nonparametric tests Nonparametric tests lack statistical power when population is normally distributed and P-values tend to be too high More Significance Tests Name of Test Purpose of Test Underlying Probability Distribution Chi-Square Compare one group Binomial Distribution to a hypothetical value Fisher’s test Compare two Binomial unpaired groups Distribution McNemar’s test Compare two paired Binomial Distribution groups Chi-square test Compare 3 or more Binomial Distribution unmatched groups Cochrane Q Compare 3 or more Binomial matched groups Distribution (adapted from Intuitive Biostatistics) Questions to Ask What type of data have you collected? How large is your sample? What is your goal? Are we comparing continuous variables or binary variables? Are there two or more groups to compare? Independent groups or matched groups? Possible Goals Describe a group: mean, median, range, variance Compare (means/proportions) one group to a hypothetical value Compare two unpaired groups Compare two paired groups Compare three or more unmatched groups Compare three or more matched groups Quantify association between two variables Predict value from another measured variable Predict value from several measured or binomial variables from Intuitive Biostatistics Oswego Data Ill Not ill Total AR Ate Y 43 11 54 79.6% vanilla ice cream? N 3 18 21 14.3% 46 29 75 Chi-Square Test for Independence Test Statistic (“Uncorrected”) 2 (observed - expected) = 2 expected degrees of freedom = (rows−1) (columns −1) Chi-square test determines whether the deviations between observed and expected are too large to be attributed to chance. Finding Expected values Formula for finding Expected values equals: (Row total Multiplied by Column total) divided by Overall total i.e grand total I.e: (Row total x Column total)/ by Overall total Therefore, ill Not ill total Expected for cell a = 54 x 46/75 = 33.12 Ate yes 43 11 54 Expected for cell b: 54 x 29/75 = 20.88 vanilla Expected for cell c: = 21 x 46/75=12.88 NO 3 18 21 Expected for cell d: = 29 x 21/ 75 = 8.12 Total 46 29 75 3. Specify a Level of Significance Level of significance = an arbitrary cut-off, a small probability, for deciding whether to declare the null hypothesis untenable Also called alpha level Commonly, alpha set at 0.05 (5%) or 0.01 (1%) if our test score lies in the acceptance zone we fail to reject the Null Hypothesis If our test score lies in the critical zone, we reject the Null Hypothesis and in favour of the Alternate Hypothesis. rejection and acceptance region Critical values is the point which separate the tail from the rest of the curve Critical Value is the cut off value between acceptance zone and rejection zone We compare our test score to the critical value and if the test score is greater than the critical value, that means our test score lies in the rejection zone and we reject the Null Hypothesis On the opposite side, if the test score is less than the Critical Value, that means the test score lies in the acceptance zone and we fail to reject the null Hypothesis. Confidence intervals, level of significance and critical value Confidence interval (1-alpha) 100% Significant level Critical value 90% 0.10 ± 1.645 95% 0.05 ± 1.960 98% 0.02 ± 2.326 99% 0.01 ± 2.567 4. Perform the Statistical Test, Compute P -value Chi-square tests provide chi-square test statistic, which must be converted to P-value (use computer or look-up table) P-value = probability of observing a difference as great or greater than the observed difference, if the null hypothesis were true P-value influenced by: – size of difference / strength of association – size of the sample (number of subjects) Oswego: Observed vs. Expected X2=Sum of all cell (observed value – expected value) 2/ expected value (O-E)2 Observed Expected E Cell a 43 33.12 2.947 Cell b 11 20.88 4.675 Cell c 3 12.88 7.579 Cell d 18 8.12 12.021 Total 75 75.00 27.222 X2= 2.947 +4.675+ 7.579+ 12.021=27.222 Chi-Square Tests for 2-by-2 Tables Uncorrected (Pearson) Chi-square Test 𝑁 𝑎𝑑 − 𝑏𝑐 2 or 𝑋2 = 𝑎+𝑐 𝑏+𝑑 𝑎+𝑏 𝑐+𝑑 ill Not ill total (75)(43 18 − 11 3) 2 = 2 54 21 46 29 Ate yes 43 11 54 vanilla NO 3 18 21 = 27.222 2 Total 46 29 75 Converting a X2 to a P-Value To convert the X2 into a P-value by hand, use a special X2 table The bigger the X2, the smaller the P-value For data with 1 degree of freedom, i.e., data from a 2x2 table, the X2 value must be ≥ 3.84 to yield a P-value ≤ 0.05 Alternatively, let the computer do the conversion X2 test table Critical value column for 0.05 Find the alpha level used e.g. here is 0.05 Find the intersection of your alpha level with the d.f. (column on your left titled df) The intersect of d.f and the area in the tail (e.g. 0.05) is your Critical value Critical value at alpha level of 0.05 and 1d.f is 3.84 Epi-info Using Epi-info software Open epi-info Click statcalc Click two-by-two table Then enter the data in the two-by-two table Epi-info Open epi info Epi-info statcalc Epi-info Tables (2x2xN) Box Epi-info Enter your data in the 4 cells Epi-info output Here is Epi-Info output Here is Epi Info output of an outbreak of gastroenteritis. The P-value is well below 0.05 for all 3 of the Chi-square variations. Steps of Significance Testing But why do we need p-value when we can reject/fail to reject hypotheses based on test scores and critical value? p-value has the benefit that we only need one value to make a decision about the hypothesis We don’t need to compute two different values like critical value and test scores Another benefit of using p-value is that we can test at any desired level of significance by comparing this directly with the significance level. This way we don’t need to compute test scores and critical value for each significance level We can get the p-value and directly compare it with the significance level P-value P-value: The probability of getting the observed results or more extreme if the null hypothesis (Ho) is true We reject the Ho when the p-value is smaller than the pre- established significance level A smaller p-value shows a greater evidence against the null hypothesis What Influences a P-value? Strength of association / size of difference Number of subjects (size of sample) P- value and Strength of Association D+ D- AR RR E+ 10 10 20 50% 2.0 X2 = 2.67 E- 5 15 20 25% p = 0.10 D+ D- AR RR E+ 12 8 20 60% 2.4 X2 = 5.01 E- 5 15 20 25% p = 0.03 P- value and Size of Study D+ D- AR RR E+ 10 10 20 50% 2.0 X2 = 2.67 E- 5 15 20 25% p = 0.10 D+ D- AR RR E+ 20 20 40 50% 2.0 X2 = 5.33 E- 10 30 40 25% p = 0.02 Hypothetical Cohort Study Dead Alive Total % Dead X2 = 0.53 Diabetic 2 2 4 50.0% P = 0.47 Nondiabetic 1 3 4 25.0% Diabetic 10 10 20 50.0% X2 = 2.67 Nondiabetic 5 15 20 25.0% P = 0.10 Diabetic 20 20 40 50.0% X2 = 5.33 Nondiabetic 10 30 40 25.0% P = 0.02 5. Make Decision about Hypothesis Statement that indicate the circumstances for rejecting the null hypothesis The decision rule depends on 3 factors: ✓ The alternative hypothesis, (1 or 2 tailed test) ✓ The test statistic (critical value selected) ✓ The level of significance (α =0.05) The threshold beyond which the null hypothesis would be rejected The difference of alpha from 1, gives us the Confidence Interval level alpha of 0.05 correspond to the 95% Confidence Interval level For the Gastroenteritis example above, the test statistic is greater than the Alpha level of 0.05. Also the P-value is less than the alpha level, we conclude that is statistically significant 5. Make Decision about Hypothesis If computed P-value < alpha, reject H0, i.e., conclude that difference is unlikely to be due to chance* If computed P-value > alpha, do not reject H0, i.e., conclude that difference could be due to chance* You could be right or you could be wrong! You could commit an Error NB: these Errors will be discussed in the next topic Notes on Interpretation of Statistical Tests Statistical testing does not address bias! Statistical significance ≠ importance “A difference, to be a difference, has to make a difference.” – Carl Tyler Not significant ≠ no association “Absence of evidence should not be taken as evidence of absence.” – Sherlock Holmes Statistical significance ≠ causation Next Type I and Type II Errors - end-