Prospect Theory: Decision Weights & Rare Events PDF
Document Details
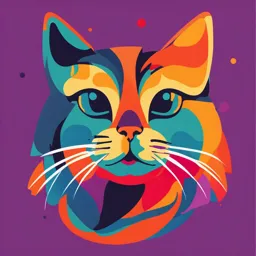
Uploaded by MercifulPiano5661
Universität Basel
Kahneman
Tags
Summary
This document discusses prospect theory, focusing on decision weights and the impact of rare events. It includes examples, like the decision to avoid buses during suicide bombings in Israel, and analyses different factors influencing decisions. It also addresses psychological biases in estimating probabilities and handling risk. This is suitable for an undergraduate studies level.
Full Transcript
S 12. Prospect Theory: Decision weights; Types of utility Kahneman Ch. 30 Rare Events Kahneman visited Israel several times during a period in which suicide bombings in buses were relatively common, though quite rare in absolute terms. For any traveller, the risks were tiny, but that was not how the...
S 12. Prospect Theory: Decision weights; Types of utility Kahneman Ch. 30 Rare Events Kahneman visited Israel several times during a period in which suicide bombings in buses were relatively common, though quite rare in absolute terms. For any traveller, the risks were tiny, but that was not how the public felt about it. People avoided buses as much as they could. Kahneman did not have much occasion to travel on buses, as he was driving a rented car, but he was chagrined to discover that his behavior was also affected. He found that he did not like to stop next to a bus at a red light, and he drove away more quickly than usual when the light changed. He knew that the risk was truly negligible, and that any effect at all on his actions would assign an inordinately high “decision weight” to a minuscule probability. In fact, he was more likely to be injured in a driving accident than by stopping near a bus. He was avoiding buses because he wanted to think of something else. This experience illustrates how terrorism works and why it is so effective: it induces an availability cascade. An extremely vivid image of death and damage, constantly reinforced by media attention and frequent conversations, becomes highly accessible, especially if it is associated with a specific situation such as the sight of a bus. ➔ System 2 may “know” that the probability is low, but this knowledge does not eliminate the self-generated discomfort and the wish to avoid it. ➔ System 1 cannot be turned off. Overweighting of unlikely outcomes is rooted in System 1. Overestimation and Overweighting 1. What is your judgment of the probability that the next president of the United States will be a third-party candidate? 2. How much will you pay for a bet in which you receive $1,000 if the next president of the United States is a third-party candidate, and no money otherwise? The two questions are different but obviously related. The first asks you to assess the probability of an unlikely event. The second invites you to put a decision weight on the same event, by placing a bet on it. How do people make the judgments and how do they assign decision weights? ➔ People overestimate the probabilities of unlikely events. ➔ People overweight unlikely events in their decisions. In overestimation and overweighting the same psychological mechanisms are involved: Focused attention, confirmation bias, and cognitive ease. Specific descriptions trigger the associative machinery of System 1. When you thought about the unlikely victory of a third-party candidate, your associative system worked in its usual confirmatory mode, selectively retrieving evidence, instances, and images that would make the statement true. You looked for a plausible scenario that conforms to the constraints of reality. Your judgment of probability was ultimately determined by the cognitive ease, or fluency, with which a plausible scenario came to mind. You do not always focus on the event you are asked to estimate. If the target event is very likely, you focus on its alternative. Example: What is the probability that a baby born in your local hospital will be released within three days? You were asked to estimate the probability of the baby going home, but you almost certainly focused on the events that might cause a baby not to be released within the normal period. You quickly realized that it is normal for babies in the United States to be released within two or three days of birth, so your attention turned to the abnormal alternative. The unlikely event became focal. The availability heuristic is likely to be evoked: your judgment was probably determined by the number of scenarios of medical problems you produced and by the ease with which they came to mind. Because you were in confirmatory mode, there is a good chance that your estimate of the frequency of problems was too high. The probability of a rare event is most likely to be overestimated when the alternative is not fully specified. Planning fallacy and other manifestations of optimism: The successful execution of a plan is specific and easy to imagine when one tries to forecast the outcome of a project. In contrast, the alternative of failure is diffuse, because there are innumerable ways for things to go wrong. Entrepreneurs and the investors who evaluate their prospects are prone both to overestimate their chances and to overweight their estimates. Vivid Outcomes In utility theory, decision weights and probabilities are the same. The decision weight of a sure thing is 100, and the weight that corresponds to a 90% chance is exactly 90, which is 9 times more than the decision weight for a 10% chance. In prospect theory, variations of probability have less effect on decision weights. An experiment found that the decision weight for a 90% chance was 71.2 and the decision weight for a 10% chance was 18.6. Psychologists at the University of Chicago published an article with the attractive title “Money, Kisses, and Electric Shocks: On the Affective Psychology of Risk.” Their finding was that the valuation of gambles was much less sensitive to probability when the fictitious outcomes were emotional (“meeting and kissing your favorite movie star” or “getting a painful, but not dangerous, electric shock”) than when the outcomes were gains or losses of cash. ➔ Rich and vivid representation of the outcome, whether or not it is emotional, reduces the role of probability in the evaluation of an uncertain prospect; adding irrelevant but vivid details to a monetary outcome also disrupts calculation. Example: 21% (or 84%) chance to receive $59 next Monday 21% (or 84%) chance to receive a large blue cardboard envelope containing $59 next Monday There will be less sensitivity to probability in the second case, because the blue envelope evokes a richer and more fluent representation than the abstract notion of a sum of money. You constructed the event in your mind, and the vivid image of the outcome exists there even if you know that its probability is low. Cognitive ease contributes to the certainty effect as well: when you hold a vivid image of an event, the possibility of its not occurring is also represented vividly, and overweighted. The combination of an enhanced possibility effect with an enhanced certainty effect leaves little room for decision weights to change between chances of 21% and 84%. Vivid Probabilities Urn A contains 10 marbles, of which 1 is red. Urn B contains 100 marbles, of which 8 are red. Which urn would you choose? The chances of winning are 10% in urn A and 8% in urn B, so making the right choice should be easy, but it is not: about 30%–40% of students choose the urn with the larger number of winning marbles, rather than the urn that provides a better chance of winning → Illustrates the superficial processing characteristic of System 1 The bias has been given several names; following Paul Slovic, Kahneman calls it denominator neglect. If your attention is drawn to the winning marbles, you do not assess the number of nonwinning marbles with the same care. Vivid imagery contributes to denominator neglect. The distinctive vividness of the winning marbles increases the decision weight of that event, enhancing the possibility effect. Of course, the same will be true of the certainty effect. If I have a 90% chance of winning a prize, the event of not winning will be more salient if 10 of 100 marbles are “losers” than if 1 of 10 marbles yields the same outcome. The idea of denominator neglect helps explain why different ways of communicating risks vary so much in their effects. You read that “a vaccine that protects children from a fatal disease carries a 0.001% risk of permanent disability.” The risk appears small. Now consider another description of the same risk: “One of 100,000 vaccinated children will be permanently disabled.” The second statement does something to your mind that the first does not: it calls up the image of an individual child who is permanently disabled by a vaccine; the 999,999 safely vaccinated children have faded into the background. ➔ As predicted by denominator neglect, low probability events are much more heavily weighted when described in terms of relative frequencies (how many) than when stated in more abstract terms of “chances,” “risk,” or “probability” (how likely). System 1 is much better at dealing with individuals than categories. The power of format (frequency or probability format) creates opportunities for manipulation. Decisions from Global Impressions The evidence suggests the hypothesis that focal attention and salience contribute to both the overestimation of unlikely events and the overweighting of unlikely outcomes (there are exceptions). Salience is enhanced by mere mention of an event, by its vividness, and by the format in which probability is described. Choice from description yields a possibility effect—rare outcomes are overweighted relative to their probability (Prospect Theory). In sharp contrast, overweighting is never observed in choice from experience, and underweighting is common. The interpretation of choice from experience is not yet settled. The probability of a rare event will (often, not always) be overestimated, because of the confirmatory bias of memory. Thinking about that event, you try to make it true in your mind. A rare event will be overweighted if it specifically attracts attention. Separate attention is effectively guaranteed when prospects are described explicitly (“99% chance to win $1,000, and 1% chance to win nothing”). When there is no overweighting, there will be neglect. When it comes to rare probabilities, our mind is not designed to get things quite right. Baron pp. 258 – 259: Experienced, predicted, and decision utility Experienced utility: Is what really matters. If you try two different kinds of beer, then the experience of drinking each beer is its true experienced utility. Predicted utility: The judgement you would make about each experience, how good it would be, possibly on the basis of memory of previous experience. Decision utility: Is inferred from your choice. Observe which one you choose. The three types of utility could conflict. Beer A might taste better (provide more experienced utility) than beer B, but you might predict the opposite. You might, for example, had a naive theory that a beer tastes better when you haven't had it for a while, and you might base your prediction on the fact that you haven't had B for a long time. Or, you might even predict that A would taste better, but you might choose B anyway because you follow a general heuristic of seeking variety, a rule that here could let you down, in terms of experienced utility. Part of the reason that we cannot predict our experiences well is that we cannot remember them well. Our memories of the quality of experiences are excessively influenced by their endings and by their best or worst points, and we tend to ignore their duration. Normative models are about experienced or, more generally, true utility. Ideally, your judgments and decisions should agree with your experienced utility. But they do not. Many of the demonstrations show that choices are inconsistent with other choices made by the same person. In such cases, both choices reflect "decision utility," but they cannot possibly both reflect true (or experienced) utility. The idea that utility is "revealed" in our choices — a common assumption in economics — is thus misleading because our choices reveal decision utility only. Our actual choices may not lead to the best outcomes. They may be subject to biases. Baron pp. 262 – 267: Prospect Theory It is important to remember that prospect theory is descriptive, not normative. It explains how and why our choices deviate from the normative model of expected-utility theory. Prospect theory applies directly to situations like the Allais paradox. Prospect theory, as a modification of expected-utility theory, has two main parts: one concerning probability and one concerning utility. The theory retains the basic idea that we make decisions as though we multiplied something like a subjective probability by something like a utility. The more probable a consequence is, the more heavily we weigh its utility in our decision. According to prospect theory however, we distort probabilities, and we think about utilities as changes from a reference point. The reference point is easily affected by irrelevant factors, and this fact leads us to make different decisions for the same problem, depending on how it is presented to us. Pi and the certainty effect: In essence, prospect theory begins with the premise that we do not treat the probabilities as they are stated. Instead, we distort them, according to a particular mathematical function that Kahneman and Tversky named the "pi function," using the Greek letter π instead of the usual p for probability. Instead of multiplying our utilities by p, the researchers proposed, people multiply by π. The function is graphed in Figure 11.1. More generally, we can describe the π function by saying that people are most sensitive to changes in probability near the natural boundaries of 0 (impossible) and 1 (certain). Sensitivity to changes diminishes as we move away from these boundaries. Thus, a 0.1 increase in the probability of winning a prize has a greater effect on decisions when it changes probability of winning from 0 to 0.1 (turning an impossibility into a possibility) or from 0.9 to 1 (turning a possibility into a certainty) than when it changes the probability from, say, 0.3 to 0.4, or 0.6 to 0.7 (turning a smaller possibility into a larger possibility). Certainty effect: An improvement from 95% to 100% is a qualitative change that has a large impact, the certainty effect. Outcomes that are almost certain are given less weight than their probability justifies. Principle of invariance: One’s choices ought to depend on the situation itself, not on the way it is described. In other words, when we can recognize two descriptions of a situation as equivalent, we ought to make the same choices for both descriptions. Subjects seem to violate this principle which is also called “framing effect” because the choice made is dependent on how the situation is presented or “framed”. Is the certainty effect rational? Why should we not weigh certain (sure) outcomes more than uncertain ones? 1.) It leads us to more inconsistent decisions, decisions that differ as a function of the way things are described to us (or the way we describe things to ourselves). 2.) Our feeling of "certainty" about an outcome is often, if not always, an illusion. For example, you may think of ($30) as a certain outcome: You get $30. Unless having money is your only goal in life, though, the $30 is really just a means to other ends. You might spend it on tickets to a football game, for example, and the game might be close, and so exciting that you tell your grandchildren about it or it might be a terrible game, with the rain pouring down, and you, without an umbrella, having to watch your team get slaughtered. In short, most, if not all "certain" outcomes can be analyzed further, and in doing so one finds, on close examination, that the outcomes are themselves gambles. The description of an outcome as certain is not certainty itself. Overweighting and underweigthing probabilities: Another property of the π function is the overweighing of very low probabilities and underweighing of very high probabilities. This may also contribute to the Allais paradox. When probabilities of some outcome are sufficiently small, we tend to disregard that outcome completely in our decisions. We behave as though we had a threshold below which probabilities are essentially zero. Schwalm and Slovic (1982), for example, found that only 10% of their subjects said they would wear seat belts when they were told that the probability of being killed in an automobile accident was about.00000025 per trip, but 39% said they would wear seat belts when they were told that the probability of being killed was about 0.01 over a lifetime of driving. The second probability is derived from the first, using the average number of trips per lifetime. People treat a probability of.00000025 as essentially zero, so it does not matter to them how many trips they take when the probability is so low. Kahneman Appendices A & B Over 40 pages to summarize………just read it lol