Sustainable Reverse Logistics Implementation with Triple Bottom Line Approach PDF
Document Details
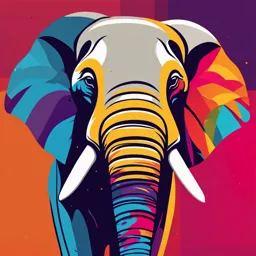
Uploaded by SnazzyMossAgate8932
Sepuluh Nopember Institute of Technology (ITS)
2023
Fahri Anwar, Erwin Widodo, Iwan Vanany
Tags
Summary
This paper investigates sustainable reverse logistics implementation in the Indonesian food and beverage industry, focusing on the effects of reverse logistics activity on triple bottom line (TBL) performance as well as operational performance. The research utilizes Structural Equation Modeling (SEM-PLS) and data from 123 respondents to assess the significance of various factors. Key findings reveal that external factor capability positively affects reverse logistics effectiveness, while internal capabilities do not. Furthermore, the effectiveness of reverse logistics significantly impacts economic, environmental, and social performance, but not operational performance. The research concludes by offering insights for companies to improve their reverse logistics implementation.
Full Transcript
Proceedings of the International Conference on Industrial Engineering and Operations Management Manila, Philippines, March 7-9, 2023 Sustainable Reverse Logistics Implementation with Triple Bottom Line Approach Fahri Anwar, Erwin Widodo, Iwan Vanany....
Proceedings of the International Conference on Industrial Engineering and Operations Management Manila, Philippines, March 7-9, 2023 Sustainable Reverse Logistics Implementation with Triple Bottom Line Approach Fahri Anwar, Erwin Widodo, Iwan Vanany. Industrial and Systems Engineering Sepuluh Nopember Institute of Technology (ITS) Surabaya, 60111, Indonesian Email: [email protected], [email protected], [email protected] Abstract To explore reverse logistics in the context of the Indonesian food and beverage manufacturing industry and examine the effect of the effectiveness of reverse logistics activity on TBL. Performance and operational performance. By applying the method of Structural Equation Modeling based on Partial Least Square (SEM-PLS). Recommendations are made to improve the implementation of sustainable reverse logistics. The hypothesis related to the effectiveness of reverse logistics activity, TBL performance, and operational performance is developed. Based on data collection, as many as 123 respondents were involved in collecting data by distributing online questionnaires to company stakeholders. Findings based on the results obtained show that internal factor capability has no significant effect on the effectiveness of reverse logistics activity, while external factor capability has a significant effect on the effectiveness of reverse logistics activity. The effectiveness of reverse logistics activity has a significant impact on economic performance, environmental performance and social performance. In contrast, the effectiveness of reverse logistics activity does not have a significant impact on operational performance. However, economic performance and environmental performance have a significant influence on operational performance. Therefore, the implications of the research results can be input for companies to continue to improve the implementation of reverse logistics in companies. 1. Introduction Producers are parties that have activities to produce output, both in goods and services, but in the process and finished goods will generate waste in packaging, therefore producers have a big responsibility in solving packaging waste made of plastic they produce as stated in the regulation. Reverse logistics as a related process, starting from the point of consumption to the point of origin with the aim of creating value or proper disposal of products/goods in a cost- effective manner includes planning, implementing and controlling efficiently the flow of raw materials, goods in process, finished goods, and information. Environmental concerns form the basis for legal pressures, and environmental regulations that steer companies towards sustainable reverse logistics management. But it requires practices that are environmentally and socially sensitive. Moreover, environmental applications include investment in pollution control and prevention as well as the implementation of environmental management systems. Previous researchers said to address this increasing global concern, Gaustad, (2018) that economic policy can be considered as an effective solution that allows by leveraging a circular economy consisting of end-of-life management of a product only so that the product can be brought back into the supply chain by a mechanism that once it has completed its functional life, instead of being considered waste. This research also considers the factor of reverse logistics activities as a main objective, which analyzes what is interfering with the wider adoption of reverse logistics among manufacturers in the Nigerian manufacturing sector (U-Dominic et al. 2021). Research in Bangladesh that reviews perspectives and barriers to adopting green supply chain management practices in the textile context (Tumpa et al. 2019). Meanwhile in Thailand addressing performance bottlenecks by focusing on identifying and ranking solutions on reverse logistics adoption in the palm oil industry using a fuzzy multi-criteria decision making method (Phochanikorn et al. 2020). Research aimed at implementing reverse logistics in finding solutions for the Thai electronics industry using structural equation modeling (SEM) (P Sirisawat 2019). SEM is also used to measure the effects of the three pillars of the triple bottom line (TBL) which include: economic, environmental and social by identifying the antecedents of sustainable product innovation performance (Muñoz-Pascual et al. 2019). © IEOM Society International 260 Proceedings of the International Conference on Industrial Engineering and Operations Management Manila, Philippines, March 7-9, 2023 Previous researchers Saurabh Agrawal & Singh (2019) have modeled external and internal factors considered in disposition decisions affect the effectiveness of disposition decisions on TBL performance. Agarwal et al. (2018) tested internal and external factors for their impact on the adoption of green supply chain management (GSCM). Afum et al. (2021) in his research aims to determine the effect of the relationship between external factors on the adoption of reverse logistics in the pharmaceutical industry. Chileshe et al. (2018) measured the effect of known drivers on reverse logistics , thereby identifying action items with the greatest potential to positively enhance reverse logistics outcomes by hypothesizing a positive relationship between economic, environmental, and social drives on reverse logistics implementation. Saurabh Agrawal & Singh (2020) conducted a test between the economic, environmental and social performance of outsourcing and reverse logistics. Meanwhile, according to Masa'deh et al. (2017) by developing a hypothetical model that aims to determine the effect of the relationship between economic performance and operational performance in green implementation supply chain management on hotels. Permana & Soediantono (2022) also developed a hypothetical model that aims to determine the effect of the relationship between environment performance and operational performance in the industrial supply chain management sector in Indonesia. Based on some of the literature, it has strengthened several contexts, decisions in the effectiveness of reverse logistics encountered from several cases. Therefore, to obtain the expected model in Indonesia by identifying the effectiveness of implementing reverse logistics activities in the food and beverage industry that uses plastic-based packaging on the relationship with TBL performance and operational performance. 2. Literature Reviews 2.1 Reverse Logistics In The Plastics Industries Research has been carried out to increase understanding of plastic recycling such as by designing a sustainable reverse logistics network for efficient plastic recycling, through the development of a reverse logistics network model. Bing (2014) made a choice between source separation, post-separation collecting polyethylene terephthalate (PET) bottles separately or not and if separately, whether or not to include PET bottles of all sizes in this channel. Regarding reverse logistics in the plastics sector, there have been many studies conducted, including by analyzing the results of an exploratory study on reverse logistics practices in the plastics sector in Colombia (Halabi 2013). While in Iran the researcher chose the PET bottle reverse logistics network , a brief review published in the last decade according to which some of the models under consideration are solved using numerical examples whereas other models use real case studies and solve real world problems (Paydar 2018). This research proves that reverse logistics plays an important role in dealing with environmental issues, especially plastic by evaluating studies related to plastic waste recycling and analyzing the available frameworks for using reverse logistics as a strategic tool (Tesfaye 2020). Meanwhile, in Indonesia, several have conducted ongoing research, especially discussing the reverse logistics of plastic waste. Suryana (2017) in the reverse logistics system in Indonesia is one of the best alternatives that can be considered to reduce the limited resources of raw materials, so conduct research that aims to develop a plastic waste recycling model, especially Low Density Polyethylene (LDPE) and High Density plastics. Polyethylene (HDPE). 2.2 Hypotheses Development a. Internal and External Factor Adoption of green supply chain management (GSCM) is broader in scope than reverse logistics. According to Agarwal et al. (2018) in addition to regulatory, market, and supplier drivers, we suggest that internal drive, indicating internal organizational motivation and managerial commitment to environmental sustainability, is the main driver of GSCM adoption. Furthermore, external forces have also been studied for their impact on effectiveness by using reverse logistics with the auto-parts industry in Thailand (Charoensuk, 2018). Research conducted by Afum et al. (2021) aims to determine the effect of the relationship between external factors on the adoption of reverse logistics in the pharmaceutical industry. The findings reveal that all external variables including stakeholder (top management support) and customer pressure, except environmental regulations, have a strong influence on adoption. This means that RL activities shorten lead times and reduce procurement of new raw materials needed for production. Thus, with the implementation of RL, companies can rely on product returns as a secondary source of materials rather than a source of new raw materials. This ultimately leads to reduced operating costs, increased company competitiveness and increased profits. H1 : internal factor capability does not have a significant effect on the effectiveness of reverse logistics activity © IEOM Society International 261 Proceedings of the International Conference on Industrial Engineering and Operations Management Manila, Philippines, March 7-9, 2023 The external pressures referred to include: regulations, markets and suppliers related to higher adoption of GSCM (Agarwal et al. 2018). External and internal factors are also used in research, Agrawal & Singh (2019) which considers each disposition decision to influence the effectiveness of disposition decisions in reverse logistics activities by analyzing the relationship between internal and external factors, and their impact on the effectiveness of disposition decisions, and their relationship to performance economic, environmental, and social in reverse logistics , including indicators including internal factors: environmental mission, quality of returned products, reverse logistics costs , and the volume of returned products. While the external factors consist of: consumer behavior, business environment , and government rules and regulations. Another indicator that is included in internal barriers is support for reverse logistics practices (Govindan & Soleimani 2017; Prakash et al. 2015; Pornwasil Sirisawat & Kiatcharoenpol 2019). This can run according to the procedure if it is supported by commitment from top management (S Agrawal 2019; Piyachat, 2017; Waqas et al., 2018). Meanwhile, external barriers require an efficient and effective system to monitor returns and withdrawals (Jindal & Sangwan 2011; Kiatcharoenpol 2020). With infrastructure facilities to support the implementation of reverse logistics (S Agrawal 2019; Kiatcharoenpol 2020). Research conducted by Charoensuk (2018) aims to determine the effect of external factor relationships on the effectiveness of reverse logistics in the auto parts industry. Based on this research, it results that if a company can manage external barriers, reverse logistics management will be successful. When a company is successful with reverse logistics management , their costs and time are reduced and they can gain more reliability. H2 : external factor capability has a significant influence on the effectiveness of reverse logistics activity b. Reverse Logistics Activities According to Hazen et al. (2012) extrapolated the components of dispositional decisions in determining what alternative to decide on from reverse logistics activities , the aim is to identify the critical components of the decision- making process and suggest alternative decision frameworks that can guide future investigations and practices. According to Roger (2002); Saurabh Agrawal et al. (2015); Saurabh Agrawal & Singh (2019); Hazen et al. (2012) in the alternative decisions of reverse logistics activities most frequently used in the literature there are four including: reuse, material recovery, product improvement, and waste management. So that in measuring the effectiveness of reverse logiatics management of industrial companies using three dimensions, the results reveal the factors of cost assignment, time assignment, and reliability assignment. Where the variables that represent costs include customer service costs, transportation costs, remanufacturing, recycling costs and inventory carrying costs (Saurabh Agrawal & Singh 2019; Charoensuk, 2018; Hazen et al. 2012). According to Afum et al. (2021) explained that these activities include return, salvage, stockpiling, remanufacturing, disposal, reuse, recycling, repair and repackaging. Agrawal & Singh (2019) , proposed a model of the mechanism of influence of internal factors and internal factors on TBL performance through mediating effectiveness of disposition decisions in reverse logistics activities which include: reuse, repair, remanufacturing and recycling. Aparecida et al. (2020) , determine the effect of the relationship between reverse logistics competence on economic performance and environmental performance in the manufacturing sector in Brazil. positively, namely economic and environmental performance. H3 : The effectiveness of reverse logistics activity has a significant influence on economic performance H4 : effectiveness of reverse logistics activity does not have a significant effect on operational performance H5 : The effectiveness of reverse logistics activity has a significant influence on environmental performance H6 : The effectiveness of reverse logistics activity has a significant influence on social performance c. Economies performances Economic performance is the company's ability to reduce the cost of purchasing goods, waste disposal, waste treatment, energy consumption, and fines for environmental accidents. According to Jindal & Sangwan (2011) the implementation obstacles faced in implementing reverse logistics will outweigh the economic benefits because apart from the benefits that can be obtained by implementing reverse logistics, many organizations are still reluctant to implement reverse logistics in a simple way. high investments and less return-on-investments are the reasons companies are reluctant to implement reverse logistics in an economic context (Saurabh Agrawal & Singh 2019; Lau & Wang 2009; Tan & Hosie 2010; Waqas et al. 2018). Meanwhile, research that is constrained by the high cost of © IEOM Society International 262 Proceedings of the International Conference on Industrial Engineering and Operations Management Manila, Philippines, March 7-9, 2023 reverse logistics is an obstacle that many companies experience (MD Abdulrahman 2014; Jindal & Sangwan 2011; Sarkis 2010; Waqas et al. 2018). Company efficiency in carrying out recycling processes with cost optimization activities (Saurabh Agrawal & Singh, 2019). Higher production costs are one reason for remanufacturing or low value products are generally preferred for material recovery in recycling, but product value is an important factor in the decision. Consumers choose recycled products or may prefer new products so that they are used as indicators of annual sales of remanufactured products (Saurabh Agrawal & Singh 2019; Chung & Wee 2011; Sharma et al. 2016). The obstacle to the sustainability of reverse logistics in economic factors is due to the lack of realization of economic benefits (Saurabh Agrawal & Singh 2019; Jindal & Sangwan 2011; Waqas et al. 2018). So it requires a positive impact on operational performance. Masa'deh et al. (2017) in this study developed a hypothetical model that aims to determine the effect of the relationship between economic performance and operational performance in the supply chain management sector on hotels. H7 : economics performance has a significant influence on operational performance d. Environmental Performances The industry is generally not aware of reverse logistics practices, as support and knowledge that cares for the environment while increasing the company's economy. Therefore it requires awareness from the company or the consumer itself. Many researchers and practitioners have used key indicators as parameters to improve or analyze barriers including environmental law awareness indicators due to the company's lack of concern for the environment caused by producers (Bouzon et al. 2016, 2018; Govindan et al. 2014; Mudgal et al. 2009; Waqas et al. 2018). Waste management and waste reduction are waste management practices, where companies are responsible for or reduce the impact caused by their products (Muhammad D Abdulrahman et al. 2014; Saurabh Agrawal & Singh 2019; Bouzon et al. 2018). Furthermore a lack of Eco-literacy among members of the Industry's supply chain (Govindan et al., 2014; Mudgal et al. 2009). So that the number of actions that are less effective on environmental performance. Manufacturers also sometimes neglect the use of efficient packaging both from the ease of packaging damage and the efficiency of use, resulting in performance to reduce packaging usage (Saurabh Agrawal & Singh 2019). The complexity in measuring and monitoring suppliers still applies environmental practices (Govindan et al. 2014; Waqas et al. 2018). Monitoring and end-of-life management are important for companies to pay attention to ( de Campos et al. 2021). So researchers want to prove that environmental performance can have a significant effect on operational performance. Based on research conducted by (Fernando & Tew, 2016) who developed a hypothetical model that aims to determine the effect of the relationship between reverse logistics activity, economics performance on operational performance in the Malaysian manufacturing industry supply chain management sector. H8 : environment performance has a significant influence on operational performance 3. Methods 3.1 Research Design This research uses descriptive research because the researcher wants to examine the phenomenon that occurs with the relationship between the variables that the researcher has determined. In terms of data collection, this research includes a cross-sectional design because researchers collect data in only one group, namely, departmental representatives related to reverse logistics from companies that produce and distribute food and beverage products that use plastic packaging. 3.2 Conceptual Models According to the literature review, there are 2 independent constructs namely, internal factor capability (IFC) and external factor capability (EFC). Then 5 dependent constructs namely, effectiveness reverse logistics activity (ERL), economics performance (ECP), environment performance (EVP), social performance (SCP) and operational performance have been used to build the SEM model can be seen in Figure 1 depicting the SEM conceptual model that used in this study showed a variety of predictors and outcome variables. The conceptual model built in this study works to examine the relationship between the seven variables. Thus, the following seven hypotheses (H1, H2, H3, H4, H5, H6, H7 and H8) are also proposed to test the degree of association between various variables in food and beverage companies that use plastic packaging. Hypothesis testing consists of four groups that are directly and indirectly related. © IEOM Society International 263 Proceedings of the International Conference on Industrial Engineering and Operations Management Manila, Philippines, March 7-9, 2023 Figure 1. Conceptual model Based on the framework and population in this study, it is limited to beverage and food companies that use plastic packaging in South Sulawesi, Indonesia. The hypothesis is tested after accessing the suitability of the data through a measurement model. The complexity of the relationship is studied using the Partial Least Squares Path Modeling (PLSPM) approach, a structural equation model that takes into account the causal relationship between latent variables. PLSPM was used in this study because it can solve a sample with a small size. Although PLSPM is an old technique it has been used in the field of logistics management by researchers such as. Sampling This Sampling Frame consists of a series of instructions to identify the target population Malhotra and Birks (2007). Respondents who participated in the survey represented various levels of management including top positions for mid- level and executive employees and most belonged to the logistics, marketing & sales, corporate strategy, quality control and human resources departments. Based on the company that includes reverse logistics include: manufacturing, third party logistics, and wholesalers. In this study, researchers used a non-probability sampling technique with a judgmental sampling method because respondents were selected according to the criteria, namely, respondents who have criteria as stakeholders in beverage and food companies and are related to forward and reverse logistics operations Determining sample size by Hair et al. (2017) in PLS-SEM using the minimum R-squared method Furthermore, detecting the minimum value of 𝑅𝑅 - square in the previous literature model is 0.327 (Saurabh Agrawal & Singh, 2019). Based on the r-square value, it is determined by the closest value and the range in the table (Hair et al. 2016), which is a minimum R-square of 0.25, with a 5% probability of error. the minimum R-square method is used to determine the number of samples that must be obtained for analysis using the SEM -PLS technique using the smartPLS application. So that the minimum number of samples that must be obtained is 84 data. So, the amount of data needed for processing is more than enough. The designed questionnaire is based on the variables that have been used in the research Stages testing PLS- SEM After the data is collected and meets the minimum amount, the next step is to test the model that has been made. Testing was carried out using the SEM-PLS method by using software called SMART-PLS. Tests were carried out to find out whether the model created was consistent and matched the data collected. According to , Hair et al. (2017) there are stages in testing PLS-SEM includes: measurement mode and structural model. The test uses several criteria If the model does not match the data, it is necessary to modify the model in order to obtain a better match. Testing on SEM consists of testing the measurement model and structural model as follows a. Model Testing Measurement ( OuterModel ) _ © IEOM Society International 264 Proceedings of the International Conference on Industrial Engineering and Operations Management Manila, Philippines, March 7-9, 2023 Model _ measuring is a model that defines how an indicator can relate to latent variables. In measure or interpret measurement models, by looking at convergent validity, discriminant validity , and reliability. Stages in Hair et al. (2017) testing the measurement model is as follows. 1. Convergent validity useful for measuring the amount of outer loading and average variance extracted (AVE) for every variable latent. Mark outer loading Which recommended that is above 0.7 but the value of 0.6 is still understandable if the model is in the development stage. The recommended dam average variance extracted is AVE > 0.5 2. Discriminant Validity ) is useful for assessing whether a variable has sufficient discriminant validity. To find out the discriminant validity value i have sufficient ie with compare correlation indicator with construct Which must aim more big If compared with the correlation with the construct other. To find out the correlation value between other constructs can be seen by value fornell-lacker criterion. By looking at the fornell-lacker value criteria or AVE must be higher than the correlation between each latent construct 3. reliability indicates consistency from every indicator in variable latent Which measure these variables. The value when the variable has a good level of consistency can be measured with mark cronbach's alpha > 0.6 - 0-7, composite reliability , And rho_A accepted is > 0.7 b. Model Testing Structural ( InnerModel ) _ The structural model is a model that serves to examine the relationship between latent variables. The inner model can show that there are direct effects, indirect effects, and moderating effects of the variables studied. According to Ghozali (20 08 ) for the structural model begins by looking at the R-square value for each latent variable, then the f- square, q-square and t-statistic values as predictive power. Stages in Hair et al. (2017) testing the structural model is as follows: 1. R 2 (coefficient of determination) is the outcome measure of the modification of the coefficient of determination that calculates the number of independent variables Which enter into the similarities and sizes sample. It means R 2 states how much influence the independent variable has on the dependent variable. 2. Q 2 (cross validated redundancy) According to Ghozali (20 08 ) Q 2 is a means to assess the predictive relevance of the inner model. 3. f 2 ( effect size ) is the effect size for each path determined by calculating f 2. Based on literature how to calculate f 2 is to see the change in R 2 when the construct is removed from the model. The level of effect will be high when exogenous variables contribute to endogenous variables. f value 2 divided into three power tests in cohen (1998) 4. T-Statistics serves to test how significant the hypothesis is in research by means of a bootstrapping process. This study uses an alpha level of 5% which makes the critical value for this research is 1.65. If obtain mark on prone to -1.65 < t-sta tics so test which done stated No significant. If mark t-statistics is at on vulnerable < -1.65 or > 1.65 so test Which done stated significant. In addition, by looking at the p-value 50 years 0 0.00% Education Strata 2 (S2) 3 2.44% Undergraduate (S1) 112 91.06% Diploma (D3) 6 4.88% Senior Hih school 2 1.63% Position manager 14 11.38% Supervisors 22 17.89% head unit 22 17.89% staff 65 52.85% Company Manufacturing Units 36 29.27% © IEOM Society International 265 Proceedings of the International Conference on Industrial Engineering and Operations Management Manila, Philippines, March 7-9, 2023 third party logistics 18 14.63% wholesalers 69 56.10% Furthermore, based on the companies involved, it includes the supply chain, namely: manufacturing, third party logistics, and wholesalers with food and beverage products. Data collection begins on the 3rd October 2022 to 10 December 2022. This response can be seen from how the respondents replied to the email sent by the researcher. Delivery process via direct message and whatsapp to the respondents. During that period, 131 data were obtained response received. Then 8 were eliminated because they did not meet the criteria or were incomplete so that there were 123 respondents. 5. Results and Discussion 5.1 Evaluation of Measurement Models In the PLSPM technique of the measurement equation model, all relationships between constructs related to testing the hypothesis of the developed model are analyzed. The model is analyzed using the PLSPM approach with the help of Smart-PLS software. The relationship between internal and external factors, as well as their impact on the effectiveness of disposition decisions, and their relationship to the economic, environmental and social performance of RLs are considered in the model. The individual reliability of each item is accessed by standard factor loading. As shown in Table 1, the factor loadings for all items ranged from 0.618 to 0.931, which is greater than the threshold value of 0.7, suggested by Hair et al. except for the 6th external factor which is EF6: Government rules and regulations. The value of this factor is 0.24 which is smaller than the threshold value. Therefore, these factors were removed from the study and again factor weights were calculated. The final loading values are shown in Table 1 which meet the minimum threshold criteria for individual reliability of each construct. Internal consistency for each construct is accessed by the CR Value, which must have a minimum value of 0.7 for acceptance (Nunnally, 1978). CR values for all constructs are greater than the minimum threshold values as shown in Table 1. AVE analyzes convergent validity for all constructs. The values for all constructs are higher than the minimum threshold value of 0.5 which meets the requirements of convergent validity. The correlation between the constructs and the AVE square root for each construct was analyzed to access the discriminant validity of the model. Table 2. Statistical results of the measurement model Latent Variable Indicator Loading Cronbach's Composite AVE factor alpha reliability Internal factor Compliance rate volume of products returned 0.802 0.785 0.861 0.61 capability (IFC) Corporate environmental mission level 0.735 The importance of the business advantages of reverse logistics 0.756 Budget value allocated by top management/Top management 0.549 commitment External factor Level of fulfillment/requirements for environmentally friendly 0.672 0.75 0.856 0.666 capability (ECP) products Government pressure and regulation 0.806 Level of environmental friendly technology mastery from 0.785 suppliers green strategy comparison for the same product 0.786 Effectiveness of Product packaging reuse rate 0.8 0.746 0.855 0.663 reverse logistics Packaging waste processing level 0.622 (ERL) The effectiveness of recycled products 0.824 Product remanufacturing/repackaging/repair effectiveness 0.716 Appropriate rate of disposal of returned product 0.56 Economic Rate of return on Investment from returned products 0.836 0.732 0.832 0.553 performance Sales levels of remanufactured/repackaged products 0.852 (ECP) Reverse logistics cost optimization 0.708 total cost of disposal of waste ( disposal cost ) 0.717 Environmental Percentage reduction in consumption of rare materials/non- 0.75 0.731 0.848 0.65 Performance renewable energy (EVP) Reduction rate of packaging used 0.722 Waste reduction rate with the use of recycled materials 0.793 © IEOM Society International 266 Proceedings of the International Conference on Industrial Engineering and Operations Management Manila, Philippines, March 7-9, 2023 Number of infrastructure facilities to support RL 0.708 implementation Social Level of consumer complaints 0.557 0.825 0.613 0.613 Performance Consumer health and safety level 0.779 (SCP) Number of environmental certifications/awards achieved 0.775 Job stability level 0.624 operational Responsiveness is significant in the lead time of reverse 0.578 0.838 0.72 0.72 performance logistics operations (OPP) Level of capacity utilization and supply chain integration 0.726 Significant product quality improvement 0.736 scarp rate is decreasing 0.836 The results shown in table 3 show that the square root of the AVE value in all cases is greater than the corresponding off-diagonal value in the correlation matrix which supports the discriminatory validity condition. Discriminant validity testing relates that the gauges (manifest variables) are different and should not be highly correlated. Includes how to compare the AVE square root for each latent variable with the correlation value in the model (Fornell Larcker, 1981; Chin., 2010; Hair et al., 2019). Table 3. Discriminant Validity via Fronell-Larcker Latent Variable ECP EFC ERL EVP IFC OPP SCP Economics performance 0.781 External factor capability 0.75 0.816 Effectiveness of reverse logistics 0.642 0.589 0814 Environmental Performance 0.688 0.603 0.576 0.744 internal factor capability 0.492 0.476 0.62 0.486 0.806 operational performance 0.724 0.64 0.674 0.693 0.575 0.783 Social Performance 0.515 0.442 0.541 0.511 0.349 0.584 0849 5.2 Structural Model Evaluation Evaluation of the structural model (inner model) is used to evaluate the influence relationship between latent variables. The size used to evaluate the structural model in this study is to use the R-square ( 𝑅𝑅 2 ) and Q-square ( 𝑄𝑄 2 ) values, Table 4. R-square ( 𝑅𝑅2 ) and Q-square ( 𝑞𝑞 2 ) values hypothesis R-square q-square Effectiveness of reverse logistics 0.367 0.317 Economics performance 0.562 0.356 Environmental Performance 0.364 0.283 Social Performance 0.195 0.187 operational performance 0.607 0.36 The ability of exogenous latent variables can explain variations in exogenous variables. It was found that the R-square of the endogenous ERL latent variable was 36.7%. This means that the variation can be explained by the IFC and EFC latent variables , while the other 63.3% is influenced by other variables outside the model. The R-square value on the ECP latent variable is 56.2%, the EVP latent variable is 36.4%, and the SCP is 19.5%. which means that it can be explained by the ERL, while for the percentage difference in the ECP latent variable of 43.8%, EVP of 63.7%, and SCP of 80.5% the other variables are influenced by other variables outside the model. Finally, the R-square value for the OPP latent variable is 60.7%, which means that it can be explained by the ECP and EVP variables. while the other 39.3% is explained by other variables outside the model. Based on table 4.21 above, it shows that the Q-square value of each ERL endogenous latent variable is worth 31.7%, meaning it has a moderate effect, ECP is 35.6%, it means it has a strong effect , EVP is 28.3%, it has a moderate effect , SCP is 18.7% , it has a moderate effect, and OPP is 36. % has a strong influence. The values 𝑓𝑓 2 shown in Table 3 are within the limits as discussed in the method section regarding testing the structural model by looking at reference f2. Shows the value 𝑓𝑓 2 of each latent variable IFC on ERL is worth 0.032> 0.02 meaning that the effect is weak , EFC on ERL is worth 0.221 > 0.15 meaning the effect is moderate , ERL on ECP is worth 1.286 > 0.35 meaning it has a very strong effect , ERL on OPP is worth 0.025 > 0.02 weak effect , ERL on EVP is © IEOM Society International 267 Proceedings of the International Conference on Industrial Engineering and Operations Management Manila, Philippines, March 7-9, 2023 worth 0. 572> 0.35 strong influence , ERL on SCP is worth 0.243 > 0.15 moderate effect , and ECP on OPP has value 0.123 > 0.02 weak effect. EVP against OPP is worth 0.155 > 0.15, meaning that it has a moderate effect. Hypotheses Testing The next step is to look at the path coefficient. The value of the path coefficient or path model shows the level of significance in testing the hypothesis in this study. Hypothesis (H1) predicts that IFC affects ERL and Hypothesis (H2) EFC affects ERL in RL. The results obtained from the Smart-PLS software are shown in Table 5 and Figure 1. The path coefficient for the internal factor is 0.18 and the t-statistics value of the IFC latent variable on ERL is 1.330 which is smaller than the t-table which is 1.96. So it can be concluded that these variables have no significant effect. External factors show that the path coefficient is 0.18 with the t-statistics value of the EFC latent variable on ERL having a value of 3.194 which is greater than the t-table which is 1.96. So it can be concluded that the effect is significant or EFC has a positive effect on ERL. Table 5. Statistical results of the measurement model hypothesis Latent variable original samples T-statistics P-values f-square Information H1 IFC -> ERLs 0.18 1.33 0.184 0.032 Rejected H2 EFC -> ERLs 0.477 3,194 0.001 0.221 Accepted H3 ERL -> ECP 0.75 15,443 0 1,286 Accepted H4 ERL -> OPP 0.153 1,578 0.115 0.572 Rejected H5 ERL -> EVP 0.603 8,483 0 0.025 Accepted H6 ERL -> SCP 0.442 4,355 0 0.243 Accepted H7 ECP->OPP 0.371 3,531 0 0.123 Accepted H8 EVP->OPP 0.346 4.145 0 0.155 Accepted Hypothesis (H3) has a t-statistics value of the ERL latent variable to ECP of 15,443 which is greater than the t-table, which is 1.96. So it can be concluded that the effect is significant or ERL has a positive effect on ECP and the magnitude of the effect is 0.750. Hypothesis (H4) The t-statistics value of the ERL latent variable on OPP is 1,578 which is smaller than the t-table, which is 1.96. So it can be concluded that this variable has no significant effect. Hypothesis (H5) the t-statistics value of the ERL latent variable on EVP is 8,483 which is greater than the t-table, which is 1.96. So it can be concluded that the effect is significant or ERL has a positive effect on EVP and the magnitude of the effect is 0.603. Hypothesis (H5) The t-statistics value of the ERL latent variable on SCP is 4,355 which is greater than the t-table, which is 1.96. So it can be concluded that the effect is significant or ERL has a positive effect on SCP and the magnitude of the effect is 0.442. Hypothesis (H7) The t-statistics value of the ECP latent variable on OPP is 3,531 which is greater than the t-table, which is 1.96. So it can be concluded that the effect is significant or EVP has a positive effect on OPP and the magnitude of the effect is 0.371. Hypothesis (H8) the t- statistics value of the latent variable EVP to OPP is 4.145 which is greater than the t-table which is 1.96. So it can be concluded that the effect is significant or EVP has a positive effect on OPP and the magnitude of the effect is 0.346. This study analyzes the factors that affect ERL and its relationship with TBL and OPP performance. Hypothesis (H1) in predicting internal factors has no influence on the effectiveness of dispositional decisions in RL. In contrast, Agarwal's research (2018 ) states that with greater managerial motivation for green supply chain management ( GSCM ) is translated into a strong internal drive because the company's mission statement, corporate strategy, and organizational policies are a reflection of the beliefs and values managers in seeing the impact on the company. This can happen when suppliers are more concerned with the affordability of the manufacturing process in order to maintain the quality of the company's products. Chileshe et al. (2018) stated that the affordability of original products without remanufacturing processes is still more competitive than recycled materials. While based on the results of the hypothesis (H2) it can be concluded that EFC can affect ERL in companies. That is, with the encouragement of the level of technological mastery regarding reverse logistics from suppliers or third parties, the level of fulfillment of customer pressure and competitor pressure related to the company's green strategy regarding its products will have a significant effect on ERL. The hypotheses (H3, H4, H5 & H6) show a significant relationship to the ECP, EVP and SCP hypotheses except OPP. It is clear that ERL is significantly influenced by EFC and not significantly by IFC in reverse logistics activities that can effectively increase OPP reverse logistics through mediation or the intervention of EVP and ECP variables. Based on table 6, this shows that there is no direct relationship to OPP variables. Further studies analyzing Fernando & Tew (2016) Increase in OPP should also be considered in supply chain performance. Thus, this study has achieved its © IEOM Society International 268 Proceedings of the International Conference on Industrial Engineering and Operations Management Manila, Philippines, March 7-9, 2023 objectives and provided empirical justification for a positive and significant relationship between EVP and OPP, with ERL mediating the relationship in manufacturing companies. In this study it was found that a significant relationship between EVP and OPP obtained a higher t-value than significant relationship between ERL and OPP. Yu et al. ( 2018) in Pakistani context research and found that the ECP of companies can be improved by adopting recycling, remanufacturing activities, which are part of the reverse logistics operations. Huang et al. (2010) stated that in addition companies can increase their profitability in terms of ECP and productivity by reducing costs and investing in inventory. The company also avoids the pressure of caring for the environment and environmental regulations. Because the essence of reverse logistics is to reduce harmful effects on the environment and use recycling and remanufacturing techniques, which not only reduces solid waste but also increases process efficiency Fleischmann et al. (2000) the relationship to social performance is pressure to handle product returns at the end of their useful life due to government rules and regulations, environmental concerns, and corporate social responsibility. One way to deal with both types of returns is to adapt and implement reverse logistics practices (Agrawal 2019). Hypotheses (H7, & H8) show a significant relationship to the hypothesis of economic performance and environmental performance to operational performance. This means that the more companies manage waste or manufacturing scrap or repairs produced by the company will have an impact on operational performance. But operational responsiveness in lead time and capacity utilization decreases when implementing reverse logistics. Masa'deh et al. (2017) stated that ECP significant effect on OPP. This is associated with several context-specific factors that are very important for reverse logistics , namely labor costs, disposal costs, the market value of the goods or products salvaged. Based on these results, it can be concluded that. This study found variables with high relative importance but performance scores below other variables in the model. Previous studies had the same conditions as these indicators (Agarwal et al. 2018; Fernando & Tew, 2016; Yu et al. 2018). 6. Conclusion Based on the data processing and analysis that has been done previously, the conclusions that can be drawn are in accordance with the research objectives set at the beginning that the research aims to test the hypothesis. In the research model developed using 7 variables, namely: IFC , EFC , ERL , ECP , EVP, SCP, and OPPs. The 7 variables of the research model produce the 8 research hypotheses that are tested. In this study, recommendations were obtained as an effort to improve companies that can be carried out by companies by increasing the company's reward system for implementing reverse logistics. This study explores that EFC heavily influences ERL. Factor This identified will help managers and academics in decision making. This study relates the TBL aspect where ERL has the highest influence on ECP after EVP and SCP. OPP is not significantly influenced by ERL variables so it must be mediated by ECP variables and EVP variables. It can be concluded that the variables that can drive operational performance in implementing reverse logistics in companies are ECP and EVP. Based on an analysis of reverse logistics activities using the TBL approach, researchers found that there were hypotheses that were not significantly different from previous studies. Researchers found that the IFC hypothesis on ERL has no significant relationship. In contrast to previous studies stating that IFC has a significant relationship to ERL. Researchers found that the ERL hypothesis against OPP has no significant relationship. In contrast to previous studies stating that the ERL variable has a significant relationship to OPP which can be a driving force for improving reverse logistics in companies. This study incorporates the conceptual model from previous studies. But there is no conceptual model or hypothesis directly or indirectly linking IFC and EFC to OPP. Based on the results of the study, it has indirectly linked EFC and IFC to OPP. The results, researchers found that there was a significant indirect relationship to OPP mediated by the ERL and ECP and EVP latent variables. While IFC does not have a significant relationship indirectly. References Abdulrahman, M D., Critical barriers in implementing reverse logistics in the Chinese manufacturing sectors. International Journal of Production Economics, 147, 460–471,2014. https://doi.org/10.1016/j.ijpe.2012.08.003 Abdulrahman, Muhammad D, Gunasekaran, A., & Subramanian, N., Int. J. Production Economics Critical barriers in implementing reverse logistics in the Chinese manufacturing sectors. Intern. Journal of Production Economics, 147, 460–471, 2014. https://doi.org/10.1016/j.ijpe.2012.08.003 Afum, E., Sun, B. Z., & Kusi, C. L. Y., Reverse Logistics , Stakeholder Influence and Supply Chain Performance in Ghanaian Manufacturing Sector. February, 2021. Agarwal, A., Giraud-Carrier, F. C., & Li, Y., A mediation model of green supply chain management adoption: The © IEOM Society International 269 Proceedings of the International Conference on Industrial Engineering and Operations Management Manila, Philippines, March 7-9, 2023 role of internal impetus. International Journal of Production Economics, 205(June), 342–358, 2018. https://doi.org/10.1016/j.ijpe.2018.09.011 Agrawal, S., , 2020 Analyzing disposition decisions for sustainable reverse logistics: Triple Bottom Line approach. Resources, Conservation and Recycling, 150,2019. https://doi.org/10.1016/j.resconrec.2019.104448 Agrawal, Saurabh, & Singh, R. K. (2019a). Analyzing disposition decisions for sustainable reverse logistics: Triple Bottom Line approach. Resources, Conservation and Recycling, 150. https://doi.org/10.1016/j.resconrec.2019.104448 Agrawal, Saurabh, & Singh, R. K. (2019b). Analyzing disposition decisions for sustainable reverse logistics: Triple Bottom Line approach. Resources, Conservation and Recycling, 150(August), 104448. https://doi.org/10.1016/j.resconrec.2019.104448 Agrawal, Saurabh, & Singh, R. K. (2020). Outsourcing and reverse supply chain performance: a triple bottom line approach. Benchmarking, 28(4), 1146–1163. https://doi.org/10.1108/BIJ-09-2020-0498 Aparecida, E., Campos, R. De, Carísio, I., Paula, D., Schwengber, C., Carlos, A., Maçada, G., Marôco, J., & Klarmann, P. (2020). The e ff ect of collaboration and IT competency on reverse logistics competency - Evidence from Brazilian supply chain executives. Environmental Impact Assessment Review, 84(March), 106433. https://doi.org/10.1016/j.eiar.2020.106433 Bing, X. (2014). Sustainable reverse logistics network design for household plastic waste. Flexible Services and Manufacturing Journal, 26(1), 119–142. https://doi.org/10.1007/s10696-012-9149-0 Bouzon, M., Govindan, K., & Rodriguez, C. M. T. (2018). Evaluating barriers for reverse logistics implementation under a multiple stakeholders’ perspective analysis using grey decision making approach. Resources, Conservation and Recycling, 128, 315–335. https://doi.org/10.1016/j.resconrec.2016.11.022 Charoensuk, A. (2018). External barriers of energy management effectiveness by using reverse logistics with auto- parts industry in Thailand. Proceedings of 2018 5th International Conference on Business and Industrial Research: Smart Technology for Next Generation of Information, Engineering, Business and Social Science, ICBIR 2018, 314–318. https://doi.org/10.1109/ICBIR.2018.8391213 Chileshe, N., Rameezdeen, R., Hosseini, M. R., Martek, I., & Xian, H. (2018). Factors driving the implementation of reverse logistics : A quantified model for the construction industry. Waste Management, 79, 48–57. https://doi.org/10.1016/j.wasman.2018.07.013 Chung, C., & Wee, H. (2011). Int. J. Production Economics Short life-cycle deteriorating product remanufacturing in a green supply chain inventory control system. 129, 195–203. https://doi.org/10.1016/j.ijpe.2010.09.033 Damayanti, E. E. (2020). PERANCANGAN VENDOR MANAGED INVENTORY (VMI) UNTUK MENURUNKAN SHORTAGE COMPONENTS PADA PRODUCT LV DI PT. SCHNEIDER …. repository.president.ac.id. http://repository.president.ac.id/handle/123456789/3580 Fernando, Y., & Tew, M. M., Reverse logistics in manufacturing waste management: The missing link between environmental commitment and operational performance. International Journal of Integrated Supply Management, 10(3–4), 264–282,2016. https://doi.org/10.1504/IJISM.2016.081273 Govindan, K., Kaliyan, M., Kannan, D., & Haq, A. N. (2014). Barriers analysis for green supply chain management implementation in Indian industries using analytic hierarchy process. International Journal of Production Economics, 147(PART B), 555–568. https://doi.org/10.1016/j.ijpe.2013.08.018 Govindan, K., & Soleimani, H. (2017). A review of reverse logistics and closed-loop supply chains : a Journal of Cleaner Production focus. Journal of Cleaner Production, 142, 371–384. https://doi.org/10.1016/j.jclepro.2016.03.126 Hair, J.F., Hult, G.T.M., Ringle, C.M. and Sarstedt, M. (2017a), A Primer on Partial Least Squares Structural Equation Modeling (PLS-SEM), Sage, Thousand Oaks, CA. Halabi, A. X. (2013). A modelling framework of reverse logistics practices in the Colombian plastic sector. International Journal of Industrial and Systems Engineering, 13(3), 364–387. https://doi.org/10.1504/IJISE.2013.052282 Hazen, B. T., Hall, D. J., & Hanna, J. B., Reverse logistics disposition decision-making: Developing a decision framework via content analysis. International Journal of Physical Distribution and Logistics Management, 42(3), 244–274,2012. https://doi.org/10.1108/09600031211225954 Jindal, A., & Sangwan, K. S. (2011). Development of an Interpretive Structural Model of Barriers to Reverse Logistics Implementation in Indian Industry. 448–453. https://doi.org/10.1007/978-3-642-19692-8 Kiatcharoenpol, T., A selection of barrier factors affecting reverse logistics performance of thai electronic industry. International Journal of Intelligent Engineering and Systems, 13(2), 117–126,2020. https://doi.org/10.22266/ijies2020.0430.12 Lau, K. H., & Wang, Y., Reverse logistics in the electronic industry of China: A case study. Supply Chain © IEOM Society International 270 Proceedings of the International Conference on Industrial Engineering and Operations Management Manila, Philippines, March 7-9, 2023 Management, 14(6), 447–465,2009. https://doi.org/10.1108/13598540910995228 Masa’deh, R., Alananzeh, O., Algiatheen, N., Ryati, R., Albayyari, R., & Tarhini, A. (2017). The impact of employee’s perception of implementing green supply chain management on hotel’s economic and operational performance. Journal of Hospitality and Tourism Technology, 8(3), 395–416. https://doi.org/10.1108/JHTT-02-2017-0011 Melamed, B., Rogers, D. S., & Tibben-lembke, R. (n.d.). AN EXAMINATION OF REVERSE Related papers by. Mudgal, R. K., Shankar, R., Talib, P., & Raj, T. , Greening the supply chain practices: an Indian perspective of enablers’ relationships. International Journal of Advanced Operations Management, 1(2/3), 151,2009. https://doi.org/10.1504/ijaom.2009.030671 Muñoz-Pascual, L., Curado, C., & Galende, J. (2019). The triple bottom line on sustainable product innovation performance in SMEs: A mixed methods approach. Sustainability (Switzerland), 11(6). https://doi.org/10.3390/su11061689 Paydar, M., Designing and solving a reverse logistics network for polyethylene terephthalate bottles. Journal of Cleaner Production, 195, 605–617,2018. https://doi.org/10.1016/j.jclepro.2018.05.218 Permana, A. I., & Soediantono, D. (2022). The Role of Eco Supply Chain on Environment and Operational Performance of Indonesian Defense Industry. Journal of Industrial Engineering & …, 3(3), 73–84. https://jiemar.org/index.php/jiemar/article/view/284%0Ahttps://jiemar.org/index.php/jiemar/article/download/ 284/209 Phochanikorn, P., Tan, C., & Chen, W. , Barriers analysis for reverse logistics in Thailand’s palm oil industry using fuzzy multi-criteria decision-making method for prioritizing the solutions. Granular Computing, 5(4), 419– 436,2020. https://doi.org/10.1007/s41066-019-00155-9 Piyachat, B. (2017). The relationships among resources’ commitment reverse logistics innovation reverse logistics performance and reverse logistics cost savings: Manufacturing vs service industry. Journal of Administrative and Business Studies, 3(3), 122–135. https://doi.org/10.20474/jabs-3.3.2 Prakash, C., Barua, M. K., & Pandya, K. V., Barriers Analysis for Reverse Logistics Implementation in Indian Electronics Industry using Fuzzy Analytic Hierarchy Process. Procedia - Social and Behavioral Sciences, 189, 91–102,2015. https://doi.org/10.1016/j.sbspro.2015.03.203 Sarkis, J. (2010). Barriers to the Implementation of Environmentally Oriented Reverse Logistics : Evidence from the Automotive Industry Sector. 21, 889–904. https://doi.org/10.1111/j.1467-8551.2009.00655.x Sharma, V., Garg, S. K., & Sharma, P. B. (2016). Identi fi cation of major drivers and roadblocks for remanufacturing in India. Journal of Cleaner Production, 112, 1882–1892. https://doi.org/10.1016/j.jclepro.2014.11.082 Sirisawat, P. (2019). Correlation of Reverse Logistics Performance to Solutions Using Structural Equation Modeling. Journal of Advanced Manufacturing Systems, 18(4), 511–525. https://doi.org/10.1142/S0219686719500276 Sirisawat, Pornwasil, & Kiatcharoenpol, T. (2019). Developing Reverse Logistics Practices Solutions Model Using Structural Equation Modeling. Proceedings - 2018 3rd International Conference on Information Systems Engineering, ICISE 2018, 122–126. https://doi.org/10.1109/ICISE.2018.00030 Suryana, H. (2017). Model development of reverse logistics in the distribution and transportation network (case study of plastic waste management in Indonesia). Journal of Engineering and Applied Sciences, 12, 6649–6654,2017. https://doi.org/10.3923/jeasci.2017.6649-6654 Tan, A. W. K., & Hosie, P., Reverse logistics operations in Singapore to support Asia Pacific regions. 4(2), 196– 208,2010. Tesfaye, W. , Conceptualizing reverse logistics to plastics recycling system. In Social Responsibility Journal (Vol. 17, Issue 5, pp. 686–702,2020). https://doi.org/10.1108/SRJ-12-2019-0411 Tumpa, T. J., Ali, S. M., Rahman, M. H., Paul, S. K., Chowdhury, P., & Rehman Khan, S. A. (2019). Barriers to green supply chain management: An emerging economy context. Journal of Cleaner Production, 236. https://doi.org/10.1016/j.jclepro.2019.117617 U-Dominic, C. M., Orji, I. J., & Okwu, M., Analyzing the barriers to reverse logistics (Rl) implementation: A hybrid model based on if-dematel-edas. Sustainability (Switzerland), 13(19),2021. https://doi.org/10.3390/su131910876 Waqas, M., Dong, Q. L., Ahmad, N., Zhu, Y., & Nadeem, M., Critical barriers to implementation of reverse logistics in the manufacturing industry: A case study of a developing country. Sustainability (Switzerland), 10(11), 1– 25,2018. https://doi.org/10.3390/su10114202 © IEOM Society International 271