W5 - Recommendation Systems.pdf
Document Details
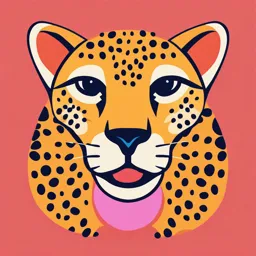
Uploaded by DexterousFern6890
National University of Singapore
Tags
Full Transcript
IS4242 INTELLIGENT SYSTEMS & TECHNIQUES L5 – Recommendation Systems Aditya Karanam © Copyright National Univer...
IS4242 INTELLIGENT SYSTEMS & TECHNIQUES L5 – Recommendation Systems Aditya Karanam © Copyright National University of Singapore. All Rights Reserved. Announcements ▸ Programming Assignment – 1: Due Tonight (September 10, 11:59 PM) ▸ Programming Assignment – 2 ‣ Will be released later in the evening ‣ Due: October 6, 11:59 PM IS4242 (Aditya Karanam) 2 In this Class … ▸ Relaying and Connecting ‣ Recommendation Systems and their hidden side effects ▸ Collaborative Filtering ‣ Singular Value Decomposition IS4242 (Aditya Karanam) 3 The Bottleneck is in Demand ▸ Firms face a monumental challenge due to the emergence of IT ‣ Products are pouring out faster than the market’s ability to absorb them ‣ The bottleneck is not in the value chain, resource scarcity, or manufacturing ‣ The bottleneck is in demand: the ability of consumers to understand make sense of and buy the products ▸ Solutions lie in the petabytes of information spewing out from the billions of consumer interactions, transactions, and other events ▸ We look at one prominent way in which this information can be used to create value for consumers: Relaying and Connecting IS4242 (Aditya Karanam) 4 Relaying and Connecting ▸ Refers to the scenario where firms learn from one customer and use that learning to help another customer ‣ A satellite with a large footprint: relay information from one location to other parts of the world, creating value for the receiver and perhaps also for the sender ▸ Firms act as a connector between two parties (E.g., buyers and sellers) that can benefit from knowing each other ▸ Simple idea but requires considerable skill to build such information arbitrage systematically ‣ Large and dispersed organizations are the best placed groups to implement this idea ‣ Any business with more than one customer or supplier can create value through this strategy IS4242 (Aditya Karanam) 5 Relaying and Connecting: Big Picture ▸ Relaying is possible due to the Big Picture advantage of the firm ‣ Firms have a view of the forest where customers can only see the trees ‣ With information across all customers, firms see ideas, products, or solutions that have been implemented or tried ‣ These solutions could be very valuable to customers in other locations, industries, or contexts ▸ Customers, however, have no means of accessing this knowledge or a view of the entire playing field ‣ They would have to reinvent the wheel to find a solution IS4242 (Aditya Karanam) 6 How Do You Relay Information? ▸ Face of it: Bringing information to a customer about what happened elsewhere seems simple enough ▸ But capturing value and building competitive advantage through relaying is difficult ‣ Requires more than a one-time transfer of information to build trust ‣ Customers value firms for their accurate and consistent relaying capabilities not just instances of relaying IS4242 (Aditya Karanam) 7 How Do You Relay Information? ▸ Requires firms to build systems to collect and collate information from a vast network, and turn that knowledge into viable customer solutions ▸ Relaying functions must be institutionalized, rather than left to the initiative of front-line (E.g., customer service) employees ▸ Such Information Systems are prominent in information-intensive online businesses with large numbers of customers ‣ Amazon, Netflix, TikTok, YouTube, Spotify, etc. IS4242 (Aditya Karanam) 8 Value through Relaying and Connecting: Amazon ▸ Amazon.com started as a bookstore ▸ Over time acquired several dedicated retailers in the online shopping space including Zappos (shoes), Diapers.com (baby products) ▸ In a decade and a half, Amazon.com has grown from zero to more than $65 billion in annual sales ‣ Revolutionized retailing challenging the world’s largest general merchandisers (Walmart) IS4242 (Aditya Karanam) 9 Value through Relaying and Connecting: Amazon ▸ How was an online store - Amazon.com, operating in an experience economy neutralize challenges from brick-and-mortar booksellers? ‣ Offline bookstores offer hot coffee, leather reading chairs, meet-the-author events, immediate product delivery, reading club meetings, etc. ▸ How did a bookstore grow big enough to buy the other category-focused retailers? ▸ Why didn’t online retailers come out of established offline general merchandise businesses? IS4242 (Aditya Karanam) 10 Value through Relaying and Connecting: Amazon ▸ Customers get much more than books at Amazon.com ‣ Additional value resides in relayed experience and connections with other readers and consumers who provide valuable information ‣ Amazon.com uses this information and builds individualized best-seller lists ▸ Readers can find out about other similar books and the books that are purchased together ‣ Books the customer might not otherwise have heard of or considered ▸ Readers don’t just learn what others are reading, they get an answer to a more personalized question: “What are others like me reading?” ‣ For repeat customers, based on their purchasing and browsing history, Amazon.com provides highly accurate and targeted recommendations IS4242 (Aditya Karanam) 11 Platforms for Relaying and Connecting ▸ Relaying makes the company occupy the central position in a network of customers and producers ‣ All information flows first to the firm and then from this central node to other nodes in the network ▸ The company acts as a platform connecting different parties that would not otherwise connect ‣ This makes the firm indispensable IS4242 (Aditya Karanam) 12 Platforms for Relaying and Connecting: Amazon ▸ Amazon.com not only connects readers but also connects thousands of store owners with consumers who visit Amazon ‣ Some of these store owners (especially, small stores) and consumers might never otherwise have connected ‣ Consumers are comfortable buying from unknown stores as they are on Amazon ▸ Amazon extracts rent from stores to gain access to its consumers, payment services, logistics, etc. ‣ Why isn’t Amazon charging consumers to have access to millions of stores? ▸ Similar strategies for other products, which made it buy other dedicated retailers ‣ Similar strategies are present in the iTunes store, App Store, etc. IS4242 (Aditya Karanam) 13 Competitive Advantage ▸ The relaying and connecting is not easy for competitors to replicate ▸ Once these functions attract a critical mass of users, they become almost insurmountable barriers to entry ▸ What do Amazon competitors need to deliver similar information to consumers with such accuracy and reliability? ‣ Hundreds of millions of customers and their experiences! ▸ Amazon’s competitive advantage resides literally with its customers! ‣ Amazon.com is customer-obsessed! IS4242 (Aditya Karanam) 14 Competitive Advantage: Amazon ▸ This is working rather well ‣ Amazon revenues were just over $10 billion in 2006 and have risen fourfold around the time of deepest recessions in the US ▸ Customers are happy too ‣ The company has consistently been one of the top two online retailers in Foresee’s annual retail satisfaction index since 2005 ‣ Another front-runner is Netflix! IS4242 (Aditya Karanam) 15 Relaying and Connecting: Summary ▸ Most valuable when customers are unable to learn from each other or from accessing other parts of the market ‣ Firms can help bridge the information gap ▸ Customers find this valuable ‣ Reduces customer’s cost of search, evaluation, comparison, and decision making ‣ Reduces customer’s risks of choosing the wrong product ▸ How do firms operationalize relaying and connecting strategies? ‣ Recommendation Systems IS4242 (Aditya Karanam) 16 Recommendation Systems: Amazon ▸ General merchandisers operated with the mindset of the offline world ‣ Sell more of the stuff they were already selling ▸ Amazon was building information channels (recommendation engines) to relay information ‣ Applicable to all products – shoes, baby products, furniture, etc. ▸ Recommendation systems bring a unique competitive advantage ‣ More customers in the platform, more informative the reviews, more accurate its recommendations, more value it adds to customers, and more business it does at a lower cost ‣ Barriers to entry escalate with a larger installed base of customers! ‣ Less likely that competitors can replicate these advantages IS4242 (Aditya Karanam) 17 Not Just Amazon ▸ Recommendation systems brought significance to the e-commerce industry! ▸ Netflix: 75% of the content watched by its subscribers was suggested by its recommendation system ‣ Netflix offered $ 1 Million to develop a collaborative filtering algorithm for its platform ‣ Popularized the idea of crowd-sourced idea generation (Kaggle cashed it) ▸ At Spotify, users listened to 2.3 billion hours of music from ‘discover weekly’ recommendations ▸ TikTok introduced personalized recommended feeds for users ‣ On average a user spends ~50 minutes on this feed ‣ YouTube, Instagram and others copied this feature IS4242 (Aditya Karanam) 18 Side Effects of Recommendation Systems ▸ Recommendations do more than just reflect consumer preferences – they shape them! ▸ Experiment with 169 consumers of music, where participants listened to songs and provided their willingness to pay for each song ‣ Each song was presented with manipulated recommendation system ratings (which participants did not know) ‣ Despite the manipulation, a 1-star increase in the rating created an average 12- 17% increase in the willingness to pay ▸ Regardless of the likelihood of actual fit, recommendation systems can decrease willingness to pay for some items and increase it for others ‣ Evokes unethical behavior of inflating recommendations artificially IS4242 (Aditya Karanam) 19 Side Effects of Recommendation Systems ▸ The advent of recommendation systems may leave us questioning our own taste ‣ Don’t need a system to tell how much we enjoyed a song we just heard ‣ We move from asking ourselves, Do I like this? ⇒ Should I like this? ▸ More important, these systems may create information bubbles in social media ‣ E.g., A republican voter may only get the positive news on Trump and only get the negative news on Biden IS4242 (Aditya Karanam) 20 Recommendation Systems © Copyright National University of Singapore. All Rights Reserved. 21 Recommendation Systems ▸ Content Based Recommendations ▸ Collaborative Filtering IS4242 (Aditya Karanam) 22 Content Based Recommendation System ▸ Users and items are associated with feature-based descriptions ‣ Textual description of items ‣ Ex: Summary of the book, title, etc. ‣ Explicit interests provided by users in a profile ‣ Ex: Book genres, etc. ▸ Obtain the similarity between users’ interests and items’ descriptions to make recommendations IS4242 (Aditya Karanam) 23 Collaborative Filtering ▸ Leverages user preferences in the form of ratings or buying behaviour in a collaborative way, for the benefit of all users ‣ Ratings, purchases, browsing, etc. represent preference or utility ▸ Utility matrix is used for providing recommendations ‣ If a relevant item is determined based on similarity between items ‣ Item-based collaborative filtering ‣ If a relevant item is determined based on user similarity ‣ User-based collaborative filtering IS4242 (Aditya Karanam) 24 Collaborative Filtering IS4242 (Aditya Karanam) 25 Collaborative Filtering IS4242 (Aditya Karanam) 26 Application: Book Recommendation System ▸ Data on books and user ratings ▸ Objective: Build a recommendation system that provides the next book to be read by the consumer ▸ Collaborative Filtering Algorithm IS4242 (Aditya Karanam) 27 Collaborative Filtering ▸ Given historical user preferences, predict (unknown) preference of a user Item1 Item2 … ItemM User1 1 0 … 1 User2 0 2 … 0 … … … … … Usern 1 ? … ? ▸ Can we just consider this as a missing value problem and use K-Nearest Neighbors? ‣ At higher dimensions, pairwise distances are concentrated at a higher value IS4242 (Aditya Karanam) 28 Singular Value Decomposition (SVD) ▸ SVD is more general than PCA ‣ PCA: Obtain low-dimensional representation of rows of data matrix ‣ SVD: Generalizes this idea to both the dimensions ▸ Decomposition is a Factorisation of the Matrix ‣ Generalizes factorization of scalars (e.g., 15 = 5 x 3) ‣ Expresses a given matrix as a product of two (or more) factor matrices ‣ 𝐴 = 𝐵𝐶 IS4242 (Aditya Karanam) 29 SVD ▸ Singular value decomposition factorizes any matrix, A ∈ ℝ𝑚×𝑛 as: A = 𝑈Σ𝑉 𝑇 ▸ 𝑈 ∈ ℝ𝑚×𝑚 and 𝑉 ∈ ℝ𝑛×𝑛 are orthonormal matrices ‣ Columns of U or rows of 𝑉 𝑇 are orthogonal, and they are unit vectors ‣ Vectors are orthogonal to each other if their dot product is zero ‣ A vector is a unit vector if its L2-norm is 1 ‣ Orthonormal matrix has the property that its transpose is its inverse. ‣ E.g., 𝑈𝑈 𝑇 = 𝑈 𝑇 𝑈 = 𝐼 IS4242 (Aditya Karanam) 30 SVD ▸ Singular value decomposition factorizes any matrix, A ∈ ℝ𝑚×𝑛 as: A = 𝑈Σ𝑉 𝑇 ▸ Σ is a diagonal matrix with values 𝜎1 , 𝜎2, 𝜎3, … , 𝜎min 𝑚,𝑛 ≥ 0 in its diagonal ‣ These values are the square root of the eigenvalues of matrix 𝐴𝐴𝑇 ‣ These are called singular values of matrix A ▸ SVD of a data matrix A can give us the principal components ordered by the eigenvalues ‣ Singular values are presented in a decreasing order in the matrix IS4242 (Aditya Karanam) 31 SVD: Geometric Interpretation ▸ U and V are new sets of axes in the m and n dimensional space ▸ Singular value 𝜎𝑖 amplifies the vectors in the 𝑣𝑖 axis in the n-dimensional space and maps them into the 𝑢𝑖 axis in the m-dimensional space IS4242 (Aditya Karanam) 32 SVD: Geometric Interpretation SVD identifies the most important directions (directions of the highest variance) in the n-dimensional and m-dimensional spaces and their relative importance IS4242 (Aditya Karanam) 33 SVD ▸ Efficient algorithms exist to compute SVD ▸ PCA is usually done by computing SVD ‣ In sci-kit learn as well ▸ SVD provides low-dimensional representations of both rows and columns ‣ a.k.a. Latent representations ‣ Varied Applications: Latent Semantic Analysis, Image Compression, etc. IS4242 (Aditya Karanam) 34 Truncated SVD ▸ Truncated SVD takes the first k columns of U and V and the main k-by-k sub matrix of Σ 𝐴𝑘 ≈ 𝑈𝑘 Σ𝑘 𝑉𝑘𝑇 ▸ Eliminating the lower variance components can also help in noise removal IS4242 (Aditya Karanam) 35 Factor Interpretations A = 𝑈Σ𝑉 𝑇 ▸ If two rows have similar values in a column of 𝑉 , corresponding columns (features) in A are similar ▸ If two rows have similar values a column of U, corresponding rows (observations) in A are similar IS4242 (Aditya Karanam) 36 Collaborative Filtering ▸ Large sparse ratings matrix R: ▸ SVD on R: 𝑅 ≈ 𝑈𝑘 Σ𝑘 𝑉𝑘𝑇 ‣ 𝐹𝑢𝑠𝑒𝑟 = 𝑈𝑘 Σ𝑘 = 𝑃𝑘 ‣ 𝐹𝑖𝑡𝑒𝑚 = 𝑉𝑘𝑇 = 𝑄𝑘𝑇 ▸ 𝑅 ≈ 𝑃𝑘 𝑄𝑘𝑇 ‣ P: Latent representations of users ‣ Q: Latent representations of items IS4242 (Aditya Karanam) 37 Collaborative Filtering: Latent Factors ▸ 𝑅𝑖𝑗 = σ𝑘𝑠=1 𝑃𝑖𝑠 𝑄𝑠𝑗 = σ𝑘𝑠=1 𝐴𝑓𝑓𝑖𝑛𝑖𝑡𝑦 𝑜𝑓 𝑢𝑠𝑒𝑟 𝑖 𝑡𝑜 𝑐𝑜𝑛𝑐𝑒𝑝𝑡 𝑠 ∗ (𝐴𝑓𝑓𝑖𝑛𝑖𝑡𝑦 𝑜𝑓 𝑖𝑡𝑒𝑚 𝑗 𝑡𝑜 𝑐𝑜𝑛𝑐𝑒𝑝𝑡 𝑠) ▸ What do latent factors/concepts mean? ‣ They characterise both users and items ‣ Sometimes they have meaningful interpretations, but not always IS4242 (Aditya Karanam) 38 Collaborative Filtering: Latent Factors ▸ K-Nearest Neighbours algorithm can be used in lower dimensions to find ‘neighbors’ ‣ Similar users with respect to their item-ratings ‣ Similar items with respect to their user-ratings IS4242 (Aditya Karanam) 39 Matrix Completion ▸ Once P and Q are learnt, they can be multiplied to ‘complete’ the ratings 𝑇 matrix: 𝑅 ≈ 𝑃𝑚×𝑘 𝑄𝑘×𝑛 ‣ For a given user the completed row can be ordered, and the highest valued items can be recommended ▸ What we have not covered: how can P and Q be learnt when there are missing values in R? ‣ Essentially reconstruct P and Q using only known values ‣ This was among the top 3 finalists in Netflix Prize ‣ Details: http://nicolas-hug.com/blog/matrix_facto_3 IS4242 (Aditya Karanam) 40 References ▸ Adomavicius et al. 2018 Effects of Online Recommendations on Consumers’ Willingness to Pay, Information Systems Research ▸ Understanding Complex Datasets: Data Mining with Matrix Decompositions, by David Skillicorn ‣ Chapters 2.1-2.3, 3.1-3.3 ▸ Data Mining: The Textbook, by Charu Aggarwal ‣ Chapter 2.4.3 IS4242 (Aditya Karanam) 41 Thank You © Copyright National University of Singapore. All Rights Reserved.