Integration of Generative AI into Pharmacy Practice PDF
Document Details
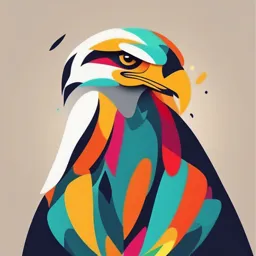
Uploaded by MiraculousMeteor
Creighton University Medical Center
Tags
Summary
This document explores the integration of generative artificial intelligence (AI) into pharmacy practice. It outlines the potential benefits, such as enhancing the quality, efficiency, and safety of pharmaceutical care, and improving patient outcomes. However, it also acknowledges potential risks, including the generation of incorrect or outdated recommendations, and challenges, such as maintaining clinical judgment in the face of AI assistance.
Full Transcript
Integration of Generative Artificial Intelligence into Pharmacy Practice Objectives: Define generative AI and its relevance to the pharmacy profession. Identify different ways in which generative AI is used from drug discovery to pharmacy practice. Discuss the ethica...
Integration of Generative Artificial Intelligence into Pharmacy Practice Objectives: Define generative AI and its relevance to the pharmacy profession. Identify different ways in which generative AI is used from drug discovery to pharmacy practice. Discuss the ethical and legal risks raised with generative AI use in pharmacy practice. Describe how teaching and learning about generative AI is integrated into pharmacy education. Introduction: The integration of generative artificial intelligence (AI) into pharmacy practice has begun to revolutionize the way that pharmaceutical services are delivered, enhancing the quality, efficiency, and safety of pharmaceutical care. The leveraging of AI models, especially those designed specifically for healthcare practice, has the potential to augment and improve the way tasks are carried out within pharmacy in many different realms, including community and health system settings, education, and within research and industry settings. Utilization of these technological advancements available, specifically generative AI, can not only streamline workflow for pharmacy employees, including technicians, pharmacist interns, and pharmacists, but also help improve patient outcomes, making the healthcare system as a whole more responsive and adaptable to patient needs, especially in the new digital age of this 21st century. While generative AI has many benefits to offer to pharmacy practice, including in caring for patients, many concerns are also raised on its implementation and use. Key issues and considerations include AI generation of wrong or out-of-date recommendations, replacement of the clinical judgement of a healthcare professional, and privacy concerns relating back to the handling of sensitive private health information. Despite these potential concerns raised, the integration of generative AI tools into pharmacy has the potential to transform not only the field of pharmacy but also the role of pharmacists, positioning them to be valuable members of the healthcare team in a more data-driven, patient-centered healthcare practice environment. Formal Definition: Generative AI can be formally defined as a form of technological machine learning that can create new content or re-design old ideas into new forms, including conversations, written materials, images, and videos. It uses input such as data or text and applies statistical methods and automated technology to create a new output 4. This specific branch of AI science aims to replicate human intelligence and cognitive processes through different computational processes and algorithms 5. Key Terms: Large Language Model A semantic component of generative AI that helps interpret and give context to text that is input into the AI system, in order to simplify output or present the output data in a more streamlined way2. In AI systems with built- in ambient listening, this also allows the technology to differentiate between different phrases and match the spoken word with a phrase the system recognizes3. Natural Language Processing A component of generative AI that allows the system to process human language it is given and assign meaning to it, which may also include identifying relationships between data or groups of sentences4. In the field of healthcare, this type of technology can use clinical notes, such as from patient encounters, to then convert this information into medical terminology, or certain diagnosis or treatment codes3. AI Hallucinations Can occur when generative AI tools produce seemingly credible but inaccurate answers by inventing non-existent references or sources13. Cluster Detection Refers to the process of identifying and grouping similar data points or patterns within larger groups of data, then organizing by a common variable14. This strategy is often applied in research and patient clinical care in order to group similar disease states and courses of action for treatment2. Automated Response Technology A feature that allows a generative AI system to use large language models and natural language processing to draft real-time responses to patient inquiries, questions, or messages within an EMR, based on the input and context contained within a patient chart. The most known example of this is Epic’s MyChart In-Basket10. Ambient Voice and Listening A feature embedded into the EHR that allows the AI to Technology capture conversations then transform them into a clinical note. This technology is often compared to a scribe that helps providers with their documentation during visits2. Machine Learning A branch of generative AI where the software is run by algorithms and statistical models which help to predict results (output) based on data that is entered by a human mind7. These models are adaptable, in which continuous use of the program helps provide reinforcement feedback, like human neuronal pathways8. Background: The use of generative AI in pharmacy, and healthcare in general, is just a small part of the digital age that we see in today’s world. While the term artificial intelligence seems relatively new in the most recent century, it has actually been around for much longer than what may initially appear. AI made its initial debut in healthcare in the 1970s, with a computer consultation system called MYCIN, which was a program designed to recognize treatments for blood infections4. In the last half century, AI use in healthcare has significantly evolved, especially in realms such as managing patient medical records, data for large hospital networks, and inventory stock and usage. On a more patient-centered level, generative AI technology has recently begun to facilitate the development of automated machines and software applications, such as diagnostic tools and treatment algorithm programs. It is important to note, however, that the forms of automation technology provided by generative AI are much different than other earlier technologies, such as automated robotic systems. A key distinction is that robots are programmed to carry out repetitive tasks that do not change, depending on what the input variable is. Generative AI, on the other hand, aims to interact more uniquely when given the input variable, by applying human-like cognition and thought processes to the task at hand7. Examples Being Used in Practice Now: Before discussing specific applications to generative AI in pharmacy practice, let’s begin by going over some common generative AI programs that are used today, both in everyday life and in the healthcare field: ❖ Chat Generative Pretrained Transformer (ChatGPT) is a reinforcement learning model sponsored by OpenAI4. It interacts with users in a format similar to texting, where users can submit questions to the platform then receive written responses. It functions similar to everyday conversations6. ❖ Google Gemini is a form of generative AI embedded into Google browsers, based on a system of natural language processing, depending on what the user inputs into a Google search7. ❖ MedAware is a form of generative AI in which machine learning is embedded into a medication safety monitoring program. This program analyzes large data sets, whether historical or from patient records, to identify potential medication errors, help find areas that could be improved to prevent errors, and sends alerts of adverse drug interactions4. ❖ Google Deep Mind Health Project, another form of Generative AI from Google, uses built-in algorithms to analyze medical records in order to help optimize outcomes. Its software prorgrams are capable of providing both diagnostic and treatment plan insights. ❖ IBM Watson for Oncology is a generative AI system in the form of a computational decision support program that is used to help oncologists make treatment decisions regarding care of their patients. This program was trained and programmed using patient cases from Memorial Sloan Kettering Cancer Center, located in New York, and aims to provide specific recommendations to physicians, based on large-scale analysis of historical patient data from oncology wards/clinics in this hospital7. Applications in Pharmacy Practice: The application of generative AI into the field of pharmacy is revolutionizing not only how pharmacists practice, but also how they interact with patients. By leveraging advanced algorithms and human-like cognitive processes, generative AI is able to analyze vast amounts of data, to not only generative personalized recommendations for clinicians or patients but can also be used to predict outcomes on small or large scales, from individual patients to groups or populations. As generative AI continues to evolve, its integration into pharmacy practice may help to enhance efficiency, reduce errors, and ultimately lead to better health outcomes for patients and healthcare systems. We will be looking at the use of generative AI in pharmacy in 5 major sectors – clinical decision support, administrative tasks, patient education, academia/educational settings, and research. ❖ Clinical Decision Support in Practice: Generative AI can be utilized by pharmacists in many different applications to enhance the care they provide, by adding an extra element of clinical decision support. Integration into Reference Systems: Generative AI can be used for pharmacists making medication interventions when it is integrated into different patient and drug databases; it can also act as a supplement resource for medication therapy management (MTM) or medication reconciliation. On an individual level, this could mean being incorporated into electronic health records (EHRs) to summarize patient chart data, like patient history, disease states, or current treatment regimens. On a macro level, generative AI can be integrated into reference systems, such as Up-To-Date, as an augmented clinical decision support system (CDSS). In this way, the generative AI program helps to provide real- time, evidence-based guidelines that can help clinicians in recommending treatments. Furthermore, the integration of this AI technology with CDSS can assist providers in making safer, more optimized treatment plans, because AI can complement the cognitive thought process of the provider while gathering data simultaneously, to give insight into potential adverse effects of a treatment protocol or interventions that may need to be made, such as lab values to monitor4. Risk Stratification: Utilization of technology like generative AI can assist in applying predictive algorithms to analysis of patient charts, to allow pharmacists, and practitioners in general, to complete a risk-benefit assessment of patients and triage interventions that should/should not be made in order to optimize patient outcomes while prioritizing safety. At a more advanced level, AI systems can pinpoint specific clinical parameters or metrics to monitor, and even help hierarchically code certain conditions or severity of disease states to provide risk adjustment for future treatment3. In addition, hospital systems can also implement AI to identify patients at greater risk of certain disease states or poor prognosis/outcomes, to guide them on next steps for treatment or if any prophylactic measures should be taken preemptively 2. Ambulatory Care Settings: Generative AI can be implemented in ambulatory care clinics, where pharmacists may provide services to patients, to help with not only making recommendations on treatment but also on documentation. A study published in the Journal of the American Pharmacists Association titled “Effectiveness of ChatGPT in clinical pharmacy and the role of artificial intelligence in medication therapy management” looked at how ChatGPT, an AI tool, compares to that of a clinical pharmacist in making recommendations and formulating treatment plans. The study looked at 3 main components – 1.) interactions between drugs, diseases, substances and supplements; 2.) recommendation of alternative medications; and 3.) management plans. The study concluded that the responses of ChatGPT were all correct and valid (out of 39 mock patient cases)4. While tools like AI should not be used to replace pharmacist clinical judgement, they can be used to validate it and also increase efficiency. For example, tools like this can be used to help providers select more appropriate medication choices and avoid any trial-and-error selections or adverse events that may be able to be predicted by this cognitive algorithm tool. Generative AI tools can be used to provide personalized medications regimens in clinic by connecting dots between many variables that must be considered when selecting pharmacotherapy options, including a patient’s history, current health status and diagnoses, treatment goals, factors that affect adherence, and cost of therapy. In addition, tools embedded within certain generative AI systems, like augmented response technology and ambient voice and listening technology can help pharmacists to triage patient messages and requests in a more timely, effective manner, to devote more attention to seeing their patients and providing patient- centered care. For example, tools like Epic’s MyChart In-Basket, that automatically drafts responses to messages sent by patients to an office, can cut down over 30 seconds spent on each message by practitioners, which over time can add up and may help reduce burn-out that documentation causes10. Pharmacogenomics: Generative AI systems can be utilized to analyze large quantities of pharmacogenomic data, whether from real or simulated patients, to aid in recommending safer, more effective pharmacotherapeutic regimens for patients. Utilizing complex programs like this allows for evaluation of multiple factors and sources of patient data, in combination with existing pharmacokinetic data, that may be beyond what a human is capable of, in regards to quantitative- wise and timewise. One of these systems, Deep Genomics, retrieves genetic information from a patient’s chart and helps to identify how particular genetic mutations are linked to certain diseases and how this influences potential therapeutic treatment options or drug targets7. Another system, XGBoost, looks at genetic differences in patient’s drug-metabolizing enzymes, along with their individual lab values and therapeutic drug monitoring values; then, using simulated pharmacokinetic data profiles, applies the pharmacogenomic data to help predict area under the curve (AUC) in order to best optimize dosing and dosing intervals8. Public Health: Pharmacists in certain roles can use generative AI systems to contribute towards greater good of public health and health equity, by allowing them to locate specific targets for interventions via population data. Furthermore, as later discussed, AI can be a tool for carrying out administrative tasks in a more efficient way, such as in public health cases by aiding in distributing efforts and allocating resources to best help communities on a larger scale. For example, the technology can aid identifying disease outbreaks or drug usage patterns, based on analysis of patient charts, including factors like age, travel history, social or family history, and lifestyle habits5. One of these systems, Reverse Engineering and Forward Simulation, used by GNS healthcare, works to identify cause-and- effect relationships by indirectly transferring a large amount of data points between different systems – encompassing a wide range from clinical, genetic, laboratory, radiographic imaging, pharmacotherapy, geographical location, consumer lifestyle, mobile data, and proteomic information – to best explain public health trends7. ❖ Administrative Tasks: Generative AI can be very helpful in pharmacy practice by assisting with or helping to automate many different administrative tasks. This can help to free up time of not only pharmacists, but also technicians, in order to provide more attention to direct patient care. Triaging Services Provided to Patients: When integrated into systems, generative AI technologies can assist in triaging prescriptions that need filled, orders verified, or patient messages/requests that need a response or action completed. The use of Large Language Models within a program allows for the AI platform to assess the severity of a request then rank it accordingly 2. This can be useful for patients reaching out to pharmacies with refill requests, voicemails left, and even new scripts/orders (such as prioritizing urgent prescriptions or stat hospital orders), by allowing the pharmacy to direct their attention to most time-sensitive manners first. Inventory Management: Generative AI can be used to help optimize inventory management within different pharmacies and companies by predicting demand based on historical data, seasonal trends, and patient prescription refill patterns. Many pharmacy systems already have technology in place to alert staff of low inventory or even give suggestions for re-order quantities. However, AI has the power of being able to utilize algorithms behind-the-scenes to forecast demand based on previous activity and even predict optimal ordering time and quantity based on when medications may be expiring (based on if they were utilized or not). The use of these features, which AI is capable of, can help to not only reduce pharmacy stock-outs that may delay patient care, but also prevent overstocking which can be harmful to inventory and overall operations of the pharmacy 5. Furthermore, AI can also be applied in the same manner to over-the-counter drugs, where previous purchases can be analyzed to determine optimal stocking quantity and frequency 7. Automation and Scheduling: Generative AI can be used to help automate reminders and provide scheduling – both from a standpoint of pharmacy employees and patients. From a standpoint of pharmacy employees like technicians and pharmacists, this generative technology can help analyze workflow and metrics to predict optimal staffing metrics, at both one location or on an aggregate in a particular area. While many companies may have a particular designated employee in charge of scheduling, the advantage of AI is that it is capable of working 24/7, does not call in sick, and also is able to interconnect a myriad of factors at once to create an output 11. This same software can be applied to the patient front also, by delivering automated messages to patients when they are due for refills. While many systems already do this, the technological advancement and integration of AI within systems may allow for automatic ordering based on patient responses, which further enhances inventory management and delivery of timely patient care, as previously discussed, because pharmacies are better able to predict patient needs 7. Machine learning algorithms built into the generative AI also allow for continuous adaptation and perpetual system improvement 5. ❖ Patient Education: While on the surface generative AI may seem to make delivery of pharmaceutical care to patients more depersonalized, it may actually help do the opposite. Generative AI can be used to draft educational materials for patients and also write out counseling points in a manner easy for patients to understand. Furthermore, the conversational and prompted nature of some programs, such as ChatGPT, may help practitioners in preparing to have more difficult conversations with patients. Counseling: An integral part of a community pharmacist’s role is providing patient counseling to patients, on not only prescription drugs but also over- the-counter products. Generative AI can be utilized to help better summarize key counseling points to patients, not only verbally but also in the form of patient education materials such as handouts. One study published in the Journal of the American Pharmacists Association, titled “Unlocking the future of patient Education: ChatGPT vs. Lexicomp as sources of patient education materials” compared premade resources in a common tertiary resource database to generative AI created-materials. The study found that generative AI helped to create less wordy materials for patients that utilized less passive, and more active language to empower patient action 6. Generative AI can also help augment pharmacist counseling points, by ensuring sufficient information is included, such as in relation to analyzing drug-drug interactions with other medications on a patient’s profile or even adding in any drug-food interactions. Tailor Materials to Certain Reading Levels: Within pharmacy practice and medicine in general, it is recommended that materials be designed at a fifth grade reading level, and also account for varying levels of health literacy, in order to ensure adequate patient understanding. Generative AI can be used in a couple ways to assist with this. Firstly, patients or providers can query a prompt to say “Please provide these instructions or material at a fifth grade reading level”; this allows the large language model itself to design material with the same context and meaning as the input. Secondly, generative AI can be utilized by pharmacists or groups designing patient education material to double-check that what the individual themselves wrote is at an optimal reading level for patient understanding, then revise the material accordingly 6. Personalized Assistance to Patients: Generative AI can be used in a couple different ways to help provide more personalized care to patients, even when they are not at the pharmacy. Based on patient preference and needs and along with its ability to sense future events based on algorithms and previous occurrence, the AI technology can help send reminders to patients to either refill medications or administer them 1. For example, generative AI can be used to design personalized messages to patients who may need to administer a weekly injection they have at home, then programmed to send these messages at a particular time or interval; this would help to improve overall patient adherence to their personal pharmacotherapeutic regimens. In a different way, generative AI can be used by patients directly when they have questions by acting as an individual they can reach out to with questions. One study published in the Journal of the American Pharmacists Association demonstrated how patients are beginning to use generative AI technologies to ask questions regarding health-related information 6. However, the downfall with this is that systems used by the general public are not tailed to an individual’s own medical history. Integration of generative AI with pharmacy systems, though, could help provide more personalized answers to patients questions usually answered by a pharmacist or provider, while also freeing up time that the provider uses to answer these questions via using generative AI to make educated recommendations with the access they have to patient charts via system interoperability 1. Assistance in Having Difficult Conversations: Generative AI can help assist pharmacists in having difficult or sensitive conversations with patients, whether in community, hospital, or ambulatory care settings. Many of these AI language models work by responding to a question they are prompted with, which means in advance of anticipated difficult conversations, pharmacists can better prepare for the interaction 1. In the same token, the conversational nature of many AI platforms can also help pharmacists to simulate conversations with patients in a back-and-forth cyclic nature 2. Outside of simulating conversations, generative AI can help provide pre-emptive, passive guidance to pharmacists by suggesting more empathetic ways to phrase things and strategies to use to help support the patient and also include them in decision-making. As previously mentioned, AI technologies could even be used to create patient education materials, to help aid in patients not only understanding but accepting difficult information. All of these strategies discussed can help enhance pharmacist’s confidence in being able to navigate difficult conversations with patients in a professional, yet empathetic tone. ❖ Education: Generative AI has the potential to be a very powerful tool within pharmacy educational settings, for both students and faculty, to empower the delivery of better curriculum and learning materials. It can be used not only to foster academic collaboration, but also to enhance individual growth and learning. While it will never replace the mind of student or teacher, it can be used to generate practice material, present information in different ways, and also develop case-based scenarios. While some individuals, especially those trained in a different generation that did not utilize digital technology as a foundation, may question the reasoning and ethics behind using AI, the future of education will likely incorporate it for the greater good of teaching students. Furthermore, universities or regulatory bodies may consider revising curriculum to include generative AI as a topic of learning/discussion in the future. Currently the Accreditation Council for Pharmacy Education (ACPE) does not address AI or generative AI in its standards. However, a report featured in Academic Medicine does provide guidance regarding AI use by healthcare professionals in general. This report features 6 general competency statements, including those related to what AI is and how it can be applied to healthcare; the influence of social, economic, and politic factors in regard to the ethics of AI use; integrating AI and information it provides into patient encounters; evaluating AI for quality, accuracy, and bias, especially in regards to providing care for different population groups of patients; implementing AI technologies into interprofessional healthcare teams; and incorporating AI into continued professional development activities 12. Keeping these general proficiencies in mind regarding AI use in healthcare and pharmacy, we can begin to see how generative AI may become more prevalent and valuable in educational settings, both by students and academia faculty. Students: There are many different ways in which generative AI may be used by pharmacy students to enhance their learning of new material that will later be applied in practice. To begin, AI tools can help summarize textbook chapters or also explain complex materials in a simpler, more concise manner. For example, students could ask AI platforms to explain drug mechanisms of action in the form of a story or real-life example, or to create mnemonics that help them remember different drug side effects. Students can also use generative AI to assist them in making study materials, such as flashcards or practice questions for upcoming exams. One study out of the University of Colorado surveyed PharmD students regarding their opinion on the use of generative AI to supplement their studies, and many students found that platforms such as ChatGPT helped make them more engaged and motivated to learn material, especially in a way that was more personalized to their learning style and material being studied 12. Generative AI tools may also be used by students in the future to assist them in reviewing available literature and research. An article published in the American Journal of Pharmaceutical Education explained how it may not be long until chatbots or generative AI are integrated into drug information databases; this could mean that students would be able to ask questions like they do to Google or another search engine, but instead while directly within a reference material. Furthermore, this article also went on to explain that generative AI tools like Large Language Models may be incorporated into search tools like PubMed in order to create literature reviews and analyses of all pieces published within the research database 11. In these ways, generative AI has the potential to not only help students study to better understand and retain knowledge but also be able to stay better informed on new pharmaceutical advancements and treatment guidelines. It must be emphasized, however, that generative AI is in no way a replacement for students to learn clinical knowledge. Instead, it is an adjunct to the process of developing clinical skills. More specifically, students who choose to utilize generative AI must use their own judgement to ensure AI output is of a particular quality and standard. In addition, generative AI requires students to ask specific and relevant questions (input) in order to receive the information they are looking for (output) 11. Faculty: In addition to use by students, generative AI has many potential applications that pharmacy faculty working in academia can utilize. Generative AI can be a supplement to faculty evaluation of students on projects or grading of assignments. For example, faculty could compare an AI-generated response to an open-ended/higher order thinking question to a student’s response, or even have AI platforms evaluate student responses via copy/paste by the faculty. In this manner, generative AI can be used as a supplement to faculty judgment and possibly improve efficiency of some academic or more administrative-type tasks, such as grading 11. Generative AI can also be used by faculty to help write curriculum (such as objectives), homework, exam questions, or case-based scenarios, including Objective Structured Clinical Exams (OSCEs) 5. This specific application of generative AI may be particularly useful for concepts that are more mathematical in nature, such as Pharmaceutical Calculations or Pharmacokinetics, because when given a certain type of word-based problem, generative AI can be used to generate similar problems with different values. Professors can then use this to provide extra reinforcement and practice for students, whether for group learning or independent studying by students. ❖ Research: Generative AI can be very useful in the realm of research, as it combines pharmaceutical science with a technological basis. Integration of AI into pharmaceutical research can help to improve the innovation, efficiency, and precision of not only drug discovery but also computational pharmaceutics. Drug Discovery: Generative AI can be applied to a few different facets of drug discovery within the practice of pharmacy. Algorithms created by AI may be used to analyze chemical databases or systems in order to identify molecular structures that may be ideal for a specific drug target – such as molecular weight, solubility, and binding properties; this can help to reduce trial-and-error common with use of just pure human cognition, which allows more time to devote to drug development steps and overall helps to acclerate the drug development process 8. In the same token, generative AI can be used during clinical trials to help identify eligible patients or even potentially other indications for current drugs in use, through its ability to analyze data from multiple sources at once 7. AI will not necessarily replace the skills and minds of pharmaceutical researchers, but will instead enhance their ability to evaluate sources and available data, especially from a time perspective. While it may be a little while before generative AI is intertwined with the process of pharmaceutical drug discovery, there are currently a few trademarked AI systems in use now by specific companies or institutions, including: ▪ AtomNet: This is an AI super-network designed by Atomwise company that utilizes a database of molecular structures to help find connections between structure and diseases, in order to narrow down a therapeutic treatment. The system is also able to account for patient- specific biological factors. It was especially utilized during the Ebola virus outbreak, to look at existing drugs that may help target the specific virus 7. ▪ Machine Learning for Pharmaceutical Discovery and Synthesis Consortium: This is a program that was designed by Massachusetts Institute of Technology (MIT), in partnership with Novartis and Pfizer. The system is designed to predict chemical stability and reactivity of certain chemicals in order to predict how they may be used for various disease targets if re-formulated into a drug molecule. In addition, this machine learning form of AI is also capable of looking at how drugs may be re-purposed for different indications or patient populations 8. ▪ Read Across Structure Activities Relationship (RASAR): This program, which originated in Europe, uses a combination of machine learning and natural language processing AI technologies. It encompasses a database of chemical structures (close to one million structures), which the built-in generative AI technologies are able to determine their specific toxicity profiles (including to specific organ systems) and any predicted health hazards if formulated into a drug. The RASAR AI system was found to be of comparable accuracy as to animal reproducibility tests, in up to 96% of cases 17. Computational Pharmaceutics: Generative AI can be used within the field of computational pharmaceutics in the form of models and simulation techniques, to help optimize drug formulation, bioavailability, polymorphic forms, and overall pharmacokinetics of a certain drug and its dosage form. Many of these models have the capability to be able to predict pharmacokinetics of different drugs, by illustrating their absorption, distribution, and clearance within the body. Utilization of these AI-models would be very beneficial in drug optimization and precision medicine, as it would allow for dosage forms to be tailored towards patient-specific factors, such as the influence of disease states on various pharmacokinetic parameters5. In addition to pharmacokinetic simulations, some generative AI platforms are already capable of running mock scenarios, similar to trials by running simulations on a large group of samples meant to represent patient population groups. One example of this is the Generative Adversarial Networks (GAN) platform, created by Insilco Medicine. This specific AI technology uses two different types of neural networks, a generator and discriminator, controlled by a reinforcement learning algorithm, that aims to give an output of potential new chemical structures which may have a connection with biological factors of particularly diseases that have already been identified7. Potential Risks: While the integration of generative AI into pharmacy practice presents many different opportunities to enhance delivery of patient care by pharmacists and efficiency of operations, it also has the potential to introduce a few significant risks that must be evaluated before implementation occurs. As technology continues to evolve within the field of pharmacy and healthcare in general, it is imperative that we consider any unintended consequences of implementation before proceeding forward. It is important to acknowledge any risks that may exist while also evaluating their individual and combined impact on delivery of services, to best ensure that these generative AI tools are used effectively and responsibly, without compromising the integrity of pharmacy practice, care of patients, or trust from patients themselves. At the forefront of this is remembering that generative AI is in no way meant to replace pharmacist clinical judgement, but is instead there to supplement it, and that pharmacists must still maintain a high level of professional responsibility accountability when performing their roles. In this section we will be looking at potential risks that generative AI use within pharmacy practice may pose, including what some recent research has discovered regarding these specific risks. ❖ Misinformation: Using generative AI in pharmacy practice, or healthcare in general, has the potential to create misinformation by producing inaccurate, deceiving, or false information output, especially when taken out of context of clinical practice or an individualized patient case by this form of technology. Depending on how individual generative AI models are trained, the output data or recommendations may not be consistent or be able to give as clear of or transparent recommendations. In this same manner, it is difficult to trace where AI technologies pull their information from; some generative AI platforms may not be able to evaluate and retrieve clinical information or research findings from databases that are blocked by firewalls or paid access. For example, ChatGPT, a well-known platform, has been trained on some internet medical literature, including PubMed, MEDLINE, Embase, and Cochrane Library, along with some drug databases like DrugBank and RxNorm. However, it still lacks access to major tertiary references like LexiComp, UpToDate, and Micromedex and also does not have access to textbooks or some treatment guidelines. Not having complete access to literature and being unable to trace where AI receives their evidence-based guidelines from has the potential to lead to misinformation in the output given. One study published in the Journal of the American Pharmacists Associated compared how responses of ChatGPT compared to those of clinical pharmacists on patient medication therapy management cases of varying complexity. The study concluded that the accuracy of ChatGPT responses declined as the complexity of cases increased. While the study cited that the AI technology still identified drug, disease, and supplement interactions over 70% of the time and formulated general treatment plans with complex cases, the accuracy and specificity of these recommendations decreased with complexity, and often follow- up questions were needed to clarify the AI recommendations 4. This is one of the key challenges with AI is that it will always give you an output result, though this information may not be able to be truly seen, understood, or proven 1. However, it is imperative that when caring for patients, recommendations are evidence-based, relevant, and individualized, thus clinical and professional judgement must always be exercised when using generative AI technologies. ❖ Access to Most Recent Information: In the same way that it is important to know where generative AI receives its information from, it is also critical to ensure that these platforms are trained on the most up-to-date and recent information. As previously discussed, AI models are trained on versions of previous information and they may not have full accessibility to information that is hidden behind firewalls, within a subscription or that has been recently updated. Poor quality or inconsistency of training information, especially between different generative AI platforms, is shown to impact both the accuracy and reliability of its output recommendations 5. Furthermore, medical information is constantly evolving as new research comes out, and it can be a challenge to trace back how recently AI platforms have been trained or updated on new literature. To provide a different perspective, when new treatments or drugs are being rolled out, the standard procedure is to conduct clinical trials. In the same way, if generative AI tools are going to be more widely used in healthcare, it may be necessary to have their platforms and algorithms undergo a similar FDA approval process, in order to ensure a patient’s safety and best interest are upheld in utilization of new technology 2. ❖ Concern for Replacement of Professional and Clinical Judgement: In addition to misinformation and not giving up-to-date information, one of the concerns of using generative AI in pharmacy practice is that it may slowly replace the professional and clinical judgement of pharmacists (or other healthcare professionals). This is a valid risk to be raised as training an AI platform from other resources/literature or machine learning via previous decisions/patient cases should not be the final say for patient care; in this way, real patients should not risk harm or consequences due to generative AI or a form of a computer formulating their care plans, instead of a trained professional 14. Especially if using a text-based system like Google Gemini or ChatGPT, it is very challenging to communicate all of a patient’s needs and medical history in this way to capture the full story, and this is where clinical judgement and evaluation of patients is a key role of a pharmacist. In order to mitigate this risk, it is important to ensure that we do not become over-reliant on generative AI, and instead use it as an augmentation to practice, not replacement. For example, in a previously referenced study that compared plans of a clinical pharmacist with ChatGPT, the generative AI platform did not recommend specific dosages or treatment plans, only general recommendations for management 4. In the same way, generative AI cannot fully replace the face-to-face interactions pharmacists have with their patients or the human judgement that comes with years of practice within the field 2. With these aforementioned reasons, generative AI should and could not 100% replace the skills and knowledge base of a practicing pharmacist, and clinical and professional judgement should remain as essential components to providing safe, effective patient care. ❖ AI Hallucinations: One risk of using generative AI is the potential of receiving AI hallucinations as an output. These AI hallucinations are different than misinformation in that the responses may seem credible but are actually inaccurate and made based on sources that do not exist. Furthermore, AI hallucinations are a major contributor to why there can be inconsistencies in response or output between different large language models used 13. Traditionally, when an algorithm, such as one within a medical device or program, is approved by the FDA it is in the form of a locked algorithm. Locked algorithms are unique in that whenever the same input is applied to a system, the same output is given each time. However, the occurrence of AI hallucinations and the fact that some generative AI programs are adaptive over time or can train themselves, means that the systems act different than locked algorithms and may require special regulation by the FDA, if these platforms do require FDA approval within the future of healthcare practice15. The risk of having seemingly plausible but wrong recommendations given and potentially used in patient care is why generative AI cannot and should not completely take over clinical and professional decision-making in pharmacy practice, as decisions should be based on evidence and individualized patient history. ❖ Bias: There are multiple areas in which bias may be introduced within generative AI, including within training of the models and in the generation of output results. As previously mentioned, inconsistencies within responses given as an output to the same input question is termed “automation bias” 4. In addition, sometimes when inputs are entered, AI responses may not account for patient specific factors that can influence both diagnostics and treatment of disease, including gender, race, socioeconomic status, genetics, lifestyle and/or health literacy. While large language models used in generative AI may be trained on multiple different reference sources or publishers of medical literature, oftentimes these resources also reflect the normal, common clinical presentation of disease state or treatment present in the majority population, thus failing to account for diversities or impacts of certain factors on patient care 3. Beyond the resource with which AI platforms pull data from, the models themselves may also contain historical or systemic biases, depending on how the algorithms function. If any of this trained data includes under-representation of particular patient populations, genders, or disease states, then AI output recommendations could potentially be less accurate or equitable for individual patients, due to lack of diversity. On a large scale, this may result in unequal access to medical treatment for some patient populations, further potentiating pre-existing inequities already present within the healthcare system 5. In order for bias like this to be minimized with use of generative AI, additional diagnostic or screening filters would need to be present within these AI platforms, to account for nuances in patient history and apply this to care provided 3. This is another reason why it is essential that pharmacists clinically and professionally assess any AI output results to ensure their recommendations are fair, inclusive and specific to a given patient case. ❖ Legal and Security Risks: Before beginning to use generative AI technologies within pharmacy practice, it is critical to ensure the technology itself is compliant with federal and state laws. Much of this begins with the training and design of generative AI systems, especially if they are dedicated strictly to healthcare. This includes ensuring not only that information the AI is trained on is accurate, but also ensures anonymity and de- identification of any PHI. One way this may be accomplished is by using large-scale, decentralized data sets to train models in order to protect privacy of sensitive content; these federated, decentralized models allow for utilization of large data sets, with minimal risk of it being transferred to any outside or centralized servers 2. Privacy concerns arise from the handling of sensitive patient data by AI systems, where these AI systems themselves may be vulnerable to breaches or misuse. For example, large language models that have been previously trained may potentially be subjected to data leakages2. Just how healthcare records are protected, AI technologies trained on or fed patient data must also be kept anonymized, encrypted, and under strict access with audit policies in place to verify appropriate use. Ensuring the maintaining of patient trust, along with confidentiality, is at the forefront of rolling out new technologies, before leveraging the features and benefits it has to offer. ❖ Pattern Recognition: There is concern that generative AI systems, especially over time, may be operating under pattern recognition, instead of being able to provide individualized and personalized patient care recommendations. This would take away from individualized patient care by allowing technology to potentially overshadow the role of clinical judgement, potentially decreasing not only quality of care but also patient outcomes. Especially when adaptive algorithms used in AI platforms, the technology may learn from dataset patterns then apply these trends to give an output regarding a situation that has not been seen before 14. This is not ideal for patient care as all patients may present differently, even if they have the same disease or treatment plan. Furthermore, generative AI runs the risk of not only functioning on pattern recognition but also in a more one-dimensional aspect. AI platforms respond only by the input with which they are fed; the limitation with this technology is it may not be able to understand the context of a situation, read non-verbal cues happening during a clinical encounter, choose to run further lab testing, or conduct a physical exam. The communication is often one- dimensional in nature because it runs not only on pattern recognition, but also must be prompted in the form of follow-up questions 4. While generative AI may be helpful for giving generalized patient recommendations, it may miss over key nuances in patient history or rare conditions due to often functioning by pattern identification via its trained algorithm(s). ❖ Interoperability: While AI can be very beneficial in assisting with making clinical decisions or completing documentation, there may be limitations when it comes to ensuring the output data of AI large language models is interoperable and compatible with electronic medical records systems in terms of coding, billing, and overall documentation of patient information and care provided by healthcare professionals. Unfortunately, most generative AI platforms currently around operate in isolation, meaning they do not seamlessly integrate with electronic health records and/or pharmacy systems. In regards to clinical platforms, lack of interoperability with generative AI may potentially lead to gaps or inconsistencies in patient medical information, reduced accuracy, and difficulties in continuity and coordination of care. From a different aspect, generative AI may also not understand medical language or coding used within these clinical platforms, such as ICD or CPT codes. This creates an added barrier for large language models and natural language processing tools within AI platforms to be able to decipher information in order to properly apply it to an output result. With time, especially if some generative AI platforms are tailored specifically to healthcare or pharmacy practice, clinical data relevancy engines such as the Fast Healthcare Interoperability Resource (FHIR) may become more commonly integrated within these systems to assist in interoperability and exchange of information, regardless of its format3. Ensuring that AI tools are transparent, accurate, and secure is crucial to mitigating these aforementioned risks and preserving trust not only in pharmacy services provided but also in the professionals that provide this care to patients. Just as some academic journals have started requiring authors to disclose if AI-generated materials were used to compose manuscripts, it may also become more commonplace in healthcare and pharmacy practice to disclose to patients if AI was used at all during the process of providing patient care 5. Takeaways: The integration of generative AI into pharmacy practice has the potential to not only transform pharmacy practice, but also act as a transformative shift in how pharmacists approach their clinical and administrative roles. As this form of technology continues to evolve, it offers unique opportunities to enhance clinical decision support, administrative tasks, patient education, pharmacy education, and the field of research. By leveraging the capabilities of generative AI, pharmacists can work to not only enhance the care provided to patients but also streamline the efficiency of some pharmacy processes. However, it is vital to keep in mind that generative AI is not a replacement for a pharmacist’s professional or clinical knowledge and judgement. Successful integration of generative AI into pharmacy practice requires balancing its advantages with the potential risks of this technology; this includes acknowledging that AI has the power to be a valuable tool but is not a replacement for any tasks within pharmacy practice. Looking at the pharmacy sector as a whole, AI will not replace pharmacists or even technicians; however, it is possible with time that individuals who choose to utilize AI may replace those who do not 11. Just as the profession of pharmacy has evolved over time, looking forward to the future of pharmacy, it is likely that the use of generative AI may also evolve in the profession. Over time pharmacy has transformed to being dispensing-focused, as in an apothecary, to very cognitive and problem-solving in nature, embracing the role of the pharmacist as a medication experts. By augmenting human thought processes, AI can help to accentuate these clinical and educational aspects 11.