Thematic Analysis PDF
Document Details
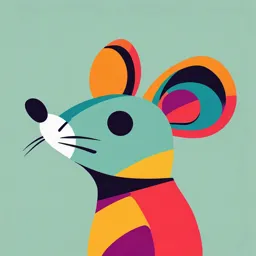
Uploaded by DashingPolonium5508
Queen Mary University of London
Gareth Terry et al.
Tags
Related
- The Textual-Visual Thematic Analysis: A Framework to Analyze the Conjunction and Interaction of Visual and Textual Data PDF
- Data Analysis in Qualitative Research PDF
- Successful Qualitative Research: A Practical Guide for Beginners PDF
- Qualitative Research Methods HE201
- Qualitative Data Analysis PDF
- Qualitative Data Analysis PDF
Summary
This document introduces thematic analysis (TA), a qualitative data analysis method. It discusses the history, context, and flexibility of TA, and illustrates its application with an example. The article also examines different approaches to TA and their theoretical underpinnings.
Full Transcript
[citations: alpha order] [spelling UK ize] [recto running head: Thematic analysis] 2 Thematic Analysis Gareth Terry, Nikki Hayfield, Victoria Clarke and Virginia Braun Introduction This chapter introduces themat...
[citations: alpha order] [spelling UK ize] [recto running head: Thematic analysis] 2 Thematic Analysis Gareth Terry, Nikki Hayfield, Victoria Clarke and Virginia Braun Introduction This chapter introduces thematic analysis (TA), a method that has become a widely-used tool for analysing qualitative data, both in psychology and beyond. We first outline the history and context of TA, and identify key issues that need to be considered when conducting TA. We discuss the flexibility TA can offer, and highlight the need for deliberate and careful research. This flexibility can apply to theoretical assumptions, research questions, data collection and analysis. We include a detailed worked demonstration of the processes and procedures of undertaking a TA, illustrated with examples from Nikki Hayfield, Victoria Clarke, Sonja Ellis and Gareth Terry’s research on the lived experiences of childfree women (see Box 2.1). Our discussion of how to complete a TA is based on a widely used version of TA – the approach developed by Virginia Braun and Victoria Clarke (2006). We conclude by considering the limitations and applications of TA, as well as future directions. [TS: Insert Box 2.1 about here] Box 2.1 Introducing the lived experience of childfree women (child -freedom) study Thematic analysis: History and context What is thematic analysis (TA)? This question invites many different answers. TA practitioner Joffe (2012) credits philosopher of science Gerald Holton with founding TA in his work on ‘themata’ in scientific thought (Holton, 1975), but the term does seem to pre-date Holton’s use of it. Since the early part of the twentieth century, if not earlier, the term ‘thematic analysis’ has been used to refer to a number of different things, including, but not limited to, data analysis techniques in the social sciences. Some earlier instances of the use of TA are similar to contemporary use – a method for identifying themes in qualitative data (e.g. Dapkus, 1985). It has also been used interchangeably with content analysis to refer to both qualitative (Baxter, 1991) and quantitative (Christ, 1970) content analysis, and some have claimed that TA developed from content analysis (Joffe, 2012). Procedures for using TA as a qualitative technique only began to be published in the 1990s (e.g. Aronson, 1994), but qualitative researchers have described their approach to analysis as ‘thematic’, without an explicit reference to a developed method, both pre- and post-specific procedural advice being published. This complexity is why, in 2006, Virginia Braun and Victoria Clarke described TA as ‘a poorly demarcated and rarely acknowledged, yet widely used qualitative analytic method’ (Braun and Clarke, 2006: 77). Since the publication of what became a landmark paper, TA as a ‘named and claimed’ method has gained hugely in popularity and has entered the qualitative canon as a recognisable and reputable method of analysis. Other notable accounts of TA procedures published prior to Braun and Clarke’s have also grown in popularity (e.g. Boyatzis, 1998). However, some confusion remains about what TA is, and indeed whether it is anything in particular. Our task in the remainder of this section is to map the terrain of TA, and identify some of the similarities and differences between various approaches to TA. This provides context for our subsequent discussion and demonstration of what has become the most widely used approach to TA – although claimed use does not always fit with the guidelines we have outlined. In seeking to clarify what TA is, our aim is not to suppress diversity and difference, but rather to acknowledge that the terrain of qualitative research is confusing and complex, and often unnecessarily so, because of poor practices in methodological writing – and particularly a failure among some methodological scholars to locate their stance. In our view, this complexity is a hindrance to high-quality qualitative research, and so our aims are for clarification, demystification, and contextualisation. One debate that continues among some psychologists is whether TA is a fully-fledged method in its own right, or whether it is simply a tool that underpins many different qualitative approaches (Willig, 2013). Some argue that TA is not a specific analytic approach, but rather a meta-analytic technique, and that most qualitative approaches involve themes in some way (Gibson and Brown, 2009); others maintain that TA is a specific analytic approach, but even if they do, it’s usually presented as a singular approach. For instance: In a thematic analysis three levels of codes are usually recognised … These are 1st, 2nd and 3rd level (or order) codes … Most people begin with a very basic descriptive level of coding and work upwards in a systematic manner towards a more interpretative level (Langridge, 2004: 267) These types of claims imply that qualitative researchers agree on what TA is, and how TA gets done. Few authors identity different versions of TA and when they do it is usually different versions of one underlying approach (for example, inductive and deductive versions, Fereday and Muir-Cochrane, 2006). In addition, many texts present more or less idiosyncratic TA procedures as definitive, or reduce the diversity of approaches – and, there are many different versions and varieties of TA – down to a singular method. For example: there are a few useful guides [on how to carry out TA], including Boyatzis (1998), Braun and Clarke (2006) and Joffe and Yardley (2004). This chapter moves to laying out the set of key steps involved in a TA. (Joffe, 2012: 215, our emphasis) Hence authors of methodological texts thus often fail to acknowledge diversity within TA. This is potentially confusing for qualitative beginners seeking clear guidance, but much more importantly, it obscures important theoretical and conceptual differences between different TA approaches. This diversity covers the overall conceptualisation of what TA is or offers, where it sits theoretically, and processes and procedures for (best practice) analysis. For a first broad categorisation, we find a distinction between ‘experiential’ and ‘critical’ orientations to qualitative research useful (Braun and Clarke, 2013; Reicher, 2000). Experiential orientations focus on what participants think, feel and do, and are underpinned by the theoretical assumption that language reflects reality (either a singular universal reality, or the perspectival reality of a particular participant). Critical orientations seek to interrogate dominant patterns of meaning and theoretically understand language as creating, rather than reflecting, reality. Some writers situate TA as only and always an experiential approach. Others describe TA as a theoretically independent – and thus flexible – approach, but still see it as particularly compatible with certain theoretical orientations, such a particular kind of phenomenology, or phenomenology in general (Guest et al., 2012; Joffe, 2012). It is rarely explained why TA is seen as particularly compatible with these approaches – and the claimed compatibility seems to rely on the assumption that TA is an experiential orientation. Moreover, any claimed theoretical independence is often circumscribed in two (related) ways. First, TA is often described as an approach that bridges a quantitative (positivist) and qualitative (interpretative) divide (Boyatzis, 1998). The idea that TA can bridge a divide between quantitative and qualitative research depends on a particular definition of qualitative research as offering techniques or tools for collecting (and analysing) qualitative data. With a conceptualisation of qualitative research as (only) about techniques and tools, TA is understood as offering a bridge over a divide, because it either provides qualitative techniques for use within a (post-)positivist paradigm, and/or allows for (post-)positivist standards like reliability to be utilised. However, this idea of what qualitative research offers is remarkably limited, and dominated by (post-)positivism, a framework that many qualitative researchers reject. An understanding of qualitative research as a paradigm (or multiple paradigms, Grant and Giddings, 2002) characterised by values and standards quite different from those espoused within (post-)positivist empiricist traditions dominates much qualitative scholarship. Therefore, any attempt to bridge qualitative and quantitative through TA therefore relies on limited conceptualisation of what qualitative research is (and can be). The second way the flexibility of TA is circumscribed stems from this point: critical orientations within qualitative research are rarely acknowledged. This absence results in a very limited account of what TA can offer. The approach to TA we have developed and that we expand on in this chapter offers full theoretical flexibility, potential for an experiential or critical orientation, and locates TA fully within a qualitative paradigm (e.g Braun and Clarke, 2006). The importance of these broader tensions is revealed through looking at the different procedures for conducting TA that are described. Despite variations across different versions, there seem to be two basic approaches: (1) an approach defined by an emphasis on coding reliability; (2) a more qualitative approach that advocates for a flexible approach to coding and theme development. Coding reliability approaches are often deductive, and echo the scientific method – moving from theory (deduction) to hypothesis/prediction (identifying themes), to evidence gathering/testing hypotheses (coding). This means analysis moves from familiarisation to some form of theme development then to coding. Themes are often at least partly determined in advance of full analysis, guided by existing theory and reflected in interview questions (in some instances, it is recommended that interview questions form the themes, Guest et al., 2012). The purpose of coding is to find the ‘evidence’ for the themes – but the distinction between codes and themes is often not very clear. You also find inductive (or data-driven) examples of ‘coding reliability’ modes of TA, but these seem to be less common (Boyatzis, 1998). What is key in ‘coding reliability’ versions of TA, is that the coding process is designed to allow the researcher to test and report on coding reliability – indeed, it is seen as essential for quality. One crucial aspect of determining coding reliability is the ‘code book’ or ‘coding frame’ – a tool that guides the coding process. A code book consists of a definitive list of codes – for each code, there is a label, a definition, instructions on how to identify the code/theme (a distinction that is not always clear), details of any exclusions, and examples. Codes are either determined in advance on basis of pre-existing theory (deductive coding), or inductively, based on familiarisation with the data, or sometimes a mix of both. The code book is then used by at least two independent coders to code all, or (more commonly) a sample of, the data. The level of agreement between the coders is then calculated to give an inter-rater reliability score (using Cohen’s Kappa; a Kappa of >.80 indicates a very good level of coding agreement and supposedly reliable coding, Yardley, 2008). This model is based in a thoroughly positivist conception of reliability – success is determined on the basis of different individuals achieving the same outcome (identical coding) through the administration of the same measure (the code book). In our view, this approach to coding is founded on a number of problematic assumptions: (1) that it is possible – and desirable – for qualitative coding to be ‘accurate’ and ‘objective’; (2) that your findings already exist in the data, waiting to be discovered; (3) that researcher subjectivity is flawed; and (4) that minimising the influence of researcher subjectivity leads to better analysis. A qualitative paradigm troubles these assumptions. Within a qualitative paradigm, there is no one right way to analyse data, because there is no single truth. Therefore, we argue that, at best, inter- rater reliability can only show that two coders have been trained to code the data in the same way, not that the coding is somehow ‘accurate’ (Braun and Clarke, 2013). In contrast, in more qualitative versions of TA such as our own (e.g. Braun and Clarke, 2006, 2013, Clarke, Braun and Hayfield, 2015a, Braun, Clarke and Terry, 2015), the subjectivity of the researcher is seen as integral to the process of analysis. Within such approaches, an inductive approach to coding and theme development is more common. Analysis once again starts with familiarisation, but close similarities with ‘coding reliability’ approaches to TA end there. Coding is treated as an organic and flexible process, where good coding requires a detailed engagement with the data. The assumption is that coding ‘gets better’ (i.e. develops depth and moves beyond the obvious surface level) through immersion in, or repeated engagement with, the data – something unlikely to be achieved with a code- book approach. Themes are developed from coding and working with the data and codes, rather than pre-existing the coding process. They are the outcome of the analytic process, rather than a starting point. They are not imagined or anticipated early on, and do not drive analytic direction. Coding and theme development are assumed to be subjective and interpretative processes. This means the outcomes of these processes can be stronger or weaker, but they cannot be right or wrong in any objective sense. The analysis is seen as something created by the researcher, at the intersection of the data, their theoretical and conceptual frameworks, disciplinary knowledge, and research skills and experience; it is not seen as something waiting ‘in’ the data to be found. Quality remains a vital concern, but quality-assurance strategies, such as a review of candidate themes (Braun and Clarke, 2006), are focused on encouraging reflection, rigour, a systematic and thorough approach, and even greater depth of engagement, rather than focusing on coding ‘accuracy’. Considering the differences among published existing versions of TA, we think they can be divided into two broad ‘schools’: (1) ‘Small q’ TA that retains a foothold in positivist research (e.g. Boyatzis, 1998; Guest et al., 2012, Joffe, 2012) and is concerned with establishing coding reliability; (2) a ‘Big Q’ approach to TA, that operates within a qualitative paradigm and is characterised by (genuine) theoretical independence and flexibility, and organic processes of coding and theme development (e.g. Braun and Clarke, 2006; Langridge, 2004). For readers unfamiliar with the small q/Big Q distinction, small q qualitative research describes the use of qualitative tools and techniques, particularly around data generation, within a positivist framework; Big Q refers to the use of these tools and techniques within the qualitative paradigm (Kidder and Fine, 1987). As our discussion above has illustrated, this distinction is important with regard to TA, because small q and Big Q approaches are underpinned by very different conceptualisations of knowledge, research, and the researcher. In small q TA, the researcher is like an archaeologist sifting through soil to discover buried treasures. Analysis is a process of discovering themes that already exist within a dataset, or finding evidence for themes that pre-exist the data. In Big Q TA, the researcher is more like a sculptor, chipping away at a block of marble. The sculpture is the product of an interaction between the sculptor, their skills and the raw materials. Analysis becomes a creative rather than technical process, a result of the researcher’s engagement with the dataset and the application of their analytic skills and experiences, and personal and conceptual standpoints. This section has highlighted that TA is far from the singular, homogeneous approach it is often treated as being, and the diversity within TA is consequential for research. Researchers need to both understand, and then locate their use of TA in relation to, this diversity – we often see authors stating they are doing TA, then referencing two different and contradictory approaches. We advocate an approach that is theoretically independent and flexible but clearly situated in practice, through a number of choices the researcher makes, and positionings to which they align. The next section highlights the ways TA can be flexibly applied across a number of theoretical frameworks. The flexibility of thematic analysis The approach to TA we have developed is characterised by independence from any particular epistemological and ontological base – and this ‘flexibility’ is partly what makes it distinct from other qualitative analyses (as well as many other versions of TA). Many approaches to qualitative analysis are better described as methodologies, as they are situated within particular theoretical frameworks, which inform the methods of data collection and analyses that can be used within that approach (see Clarke et al., 2015a). Unlike approaches such as interpretative phenomenological analysis (IPA) (e.g. Langridge, 2004; Smith, 1996), grounded theory (e.g. Charmaz, 2000; Glaser and Strauss, 1967), and discourse analysis (e.g. Potter and Wetherell, 1987; Wetherell, 1998), TA can be used within most theoretical frameworks. This flexibility, alongside its accessibility, makes TA particularly suitable for those new to qualitative research. Theoretical flexibility and research questions TA can be conducted within various ontological frameworks, which in turn will relate to epistemological approaches to data (see Table 2.1). This does not mean that TA is atheoretical! TA needs a theoretical underpinning, and researchers need to be clear about what this is. Indeed, it is precisely because of the theoretical independence of (our version of TA) that it is particularly important for researchers to make their theoretical approach explicit to the reader – it does not come ‘inbuilt’. Due to this theoretical independence, TA can be used to address a really wide range of research questions – which stem from, or require, quite different theoretical frameworks. Table 2.1 offers definitions of three broad ontological orientations typical within TA, and what the research is then assumed to capture, as well as offering some example questions. [TS: Insert Table 2.1 about here] Table 2.1 Ontologies and research questions Methods of data collection and sample size The flexibility of TA means it is suitable to analyse a wide range of data types: TA can be used to analyse data from ‘traditional’ face-to-face data collection methods such as interviews (e.g. Niland et al., 2014) and focus groups (e.g. Neville et al., 2015). It can also be used with textual data from qualitative surveys (e.g. Hayfield, 2013; Terry and Braun, 2016), diaries (e.g. Leeming et al., 2013), story based methods such as vignettes and story completion tasks (e.g. Clarke et al., 2015b), as well as online discussion forums (e.g. Bennett and Gough, 2013), and other media sources (e.g. Frith, 2015). The most important aspect of data type or mode of collection is quality of the data. Rich and complex data on a given topic are the crown jewels of qualitative research, allowing us deep and nuanced insights. Quantity (e.g. sample size) is also a consideration, but should not be conflated with quality. Key in thinking about sample size in TA is to recognise that it produces accounts of patterns across the dataset (this is not intended as a case-study approach, although some researchers are using TA in case studies, see Cedervall and Åberg, 2010). Sample size is a fraught, contentious, and debated topic in qualitative research. We offer some broad indicative size recommendations across TA projects of different scale for reference in Table 2.2 – linked to student projects. However, what is deemed ‘publishable’ is an entirely separate, and also fraught, issue, often linked to an editor’s view, but not necessarily shared by all qualitative scholars. [TS: Insert Table 2.2 about here] Table 2.2 Project sample size recommendations (adapted from Braun and Clarke, 2013) Possibilities for coding The flexibility of TA applies also to the analysis, where the researcher again needs to make some deliberate choices about their approach to data and analysis. One consideration is theoretical stance (as outlined above). Another is whether to approach the data inductively or deductively – either exclusively, or as a primary mode of engagement. Inductive coding and theme development involves working ‘bottom up’ from the data, and developing codes (and ultimately themes) using what is in the data as the starting point; the data provide the bedrock for identifying meaning and interpreting data. Of course, there is some fallacy in this idea, as the researcher is never a blank slate, and inevitably brings their own social position and theoretical lens to the analysis, but an inductive orientation signals a data-led analysis. In a deductive approach, the analytic starting point is more ‘top down’ – the researcher brings in existing theoretical concepts or theories that provide a foundation for ‘seeing’ the data, for what ‘meanings’ are coded, and for how codes are clustered to develop themes; it also provides the basis for interpretation of the data (Braun et al., 2015). A deductive orientation is less bound by the semantic meaning in the data than an inductive orientation. Whether to focus semantically or latently (again, exclusively, or primarily) is a second analytic choice. In semantic coding, codes capture explicit meaning; they are identified at the surface level of the data. In latent coding, the codes capture implicit meaning, such as ideas, meanings, concepts, assumptions which are not explicitly stated; a ‘deeper’ level of analysis is required to code in this way (see Box 2.2). In the early stages of coding, particularly for those new to qualitative analysis, the analysis is often more semantic/surface. However, with ‘immersing yourself in’ the data – and/or becoming more experienced – analysis can develop towards a more latent orientation. The appropriateness of each approach needs to fit with research question, and overall theoretical framework too: on the whole, more experiential and realist approaches align with inductive and semantic approaches to coding and theme development; more critical and relativist research often requires more deductive and latent analysis. But we are not suggesting that one approach is inherently superior to another – what is crucial is that the approach to coding is appropriate to the analytic purpose and research question. [TS: Insert Box 2.2 about here] Box 2.2 Semantic versus latent codes Doing TA: description and worked example of phases of analysis Our version of TA involves a six-phase analytic process. We use the term ‘phase’ to highlight that TA, like most approaches to qualitative analysis, is not a strictly linear process. Instead, it is iterative and recursive: the researcher often moves back and forth between the different phases. The first phase of TA, familiarising with the data, is a process that can begin during data collection. The second phase involves generating codes to immerse the researcher more deeply in the data and create the building blocks of analysis. It is likely that as coding progresses, the researcher starts to see similarities and notice patterns across the data. However, it is important to stay focused on coding the entire dataset before moving from coding to constructing themes in the third phase. The themes the researcher develops at this point are like draft versions of a piece of writing – not fixed, and flexibly open to change – with the fourth phase involving reviewing potential themes. There are a number of techniques and questions to guide progress to defining and naming themes, and then finally developing the entire analysis during the sixth and final phase, producing the report. Writing the report offers the final opportunity to make changes that strengthen the analysis and effectively communicate the analyst’s story of the data. Familiarisation and coding (phases 1–2) Familiarisation, a process common across many qualitative analytic approaches, is the bedrock for doing good TA. Familiarisation provides the researcher with an entry point into analysis – it’s a way of engaging with, and gaining insight into, what can sometimes appear to be an overwhelming mass of data. When done poorly, or not at all, the rest of the analysis often suffers. So as tempting may it be, skipping over familiarisation, or only doing it once over lightly, does not provide the best launching pad for a high quality TA. Familiarisation is the researcher’s first opportunity for what’s referred to as immersion in the dataset. While the term evokes a very passive, and possibly terrifying-sounding, process, like floating in a tank of water, it is nothing like that. Familiarisation is about intimately knowing the dataset – this facilitates a deep engagement with the data. It requires the researcher to get into a mode of reading that actively engages with the data as data – this means being observant, noticing patterns or quirks, starting to ask questions, and so on, rather than just absorbing the information therein, as when reading a good crime novel. In practice, this means reading and re-reading all textual data, making casual observational notes. It might involve (re)listening or (re)watching, if the dataset is audio or video. This first phase is about generating very early and provisional analytic ideas, and this requires being curious, and asking questions of the data. The sorts of questions vary by form of TA, combined with the research question: they could be about the way participants orient themselves to questions; about assumptions they make; about worldviews they drawing from; about the implications of their accounts for themselves and those around them; about (more semantically) the different emotional responses to the research topic; and so on (but keeping the general research question in mind). Familiarisation involves moving through the entire dataset. Keeping notes (e.g. in transcript margins; in a separate notebook) ensures these early analytic observations are remembered and can be referred back to. To make the most of this process, the researcher can synthesise observations and notes into ideas or insights related to the dataset as a whole, related to the research focus. Box 2.3 provides examples of familiarisation notes from the child-freedom study – related to one single participant and then across all transcripts. [TS: Insert Box 2.3 about here. TS Note that in the Box text file the author requests that the ‘handwritten’ typeface is retained] Box 2.3 Familiarisation from one interview and the entire dataset In the child-freedom study, the four researchers – Nikki Hayfield, Victoria Clarke, Sonja Ellis and Gareth Terry – each independently familiarised themselves with some or all the transcripts, and a research team meeting was held to discuss the insights generated. As Box 2.3 shows, one of the notes from across the dataset was about the precariousness of many of the women’s accounts. When reading the transcripts, Gareth noted early on that many of the women spoke about the various points in their lives at which they might have had children, or stages where, if they had made different choices – such as when partners put them under pressure – it may have resulted in rethinking their identity as childfree. This stood out to him, as in his previous research with vasectomised childfree men (see Terry and Braun, 2012), the participants instead emphasised a distinct, lifelong, unyielding resistance to children, a much more fixed identity. This demonstrates the way research is a subjective process (this is illustrated further in Box 2.4, where Nikki and Gareth reflect on what they brought to the project, and how it impacted on their analytic process). An overenthusiastic researcher might take a familiarisation noticing like this, and attempt turn it into a theme early on, before it has been identified across the data. There are two risks of a ‘fast and loose’ approach like that, which impacts quality: (1) the risk of ‘cherry picking’, or selectively choosing data to suit an argument; and (2) not providing the best explanation for the data as a whole, the best answer to the research question. This approach does not provide the evidence needed for a quality TA. [TS: Insert Box 2.4 about here] Box 2.4 What you see in the data (to some extent) reflects who you are Having developed a sense of the overall dataset, the researcher now begins generating codes. Where familiarisation was a process of making casual observational notes, coding is the systematic and thorough creation of meaningful labels attached to specific segments of the dataset – segments that have meaning relevant to the research question. Coding involves identifying these relevant data within each data item, and then ‘tagging’ them with a few words or a pithy phrase (e.g. hates the idea of pregnancy’) that captures the meaning of that data segment to the researcher. As noted above, codes vary in what they capture or highlight, from the semantic obvious meaning through to more latent or conceptual ideas (see Box 2.2 for examples). Good coding is open and inclusive, identifying and labelling all segments of interest and relevance within the dataset, and everything that is of relevance within those segments. Sometimes a data segment might be tagged with more than one code; other segments might not be coded at all, as they have no relevance to the research question. This latter point is particularly important: TA does not require the researcher to code every line of data (some other approaches do advocate this). There are no ‘right’ or ‘wrong’ codes: codes generated need to be meaningful to the researcher, capturing their interpretations of the data, in relation to their research question. However, it is good to remember that coding is a process both of data reduction (a way to reduce down and start to synthesise a mass of data), and a way of starting to organise the data and researcher observations of it into patterns. So a good code (label) ideally contains enough information about the content of that data extract, and sometimes analytic interpretation, that it is meaningful without needing to refer back to the data. We’ve called this the ‘take away the data’ test (Braun and Clarke, 2013). This is might seem annoyingly pedantic, but it becomes particularly important later in the process, when developing themes from codes. [TS: Insert Table 2.3 about here.] [TS: Note that entries in column 2 must keep position relative to text in column 1, as shown in text file] Table 2.3 Example of coding in P17 (‘Millers’) Table 2.3 gives an example of a coded data extract for the child-freedom project. The broadly semantic codes (e.g. quality of life would be impacted) reflect what the participant (‘Millers’) explicitly said about, and the meanings she ascribed to, being childfree. More latent codes capture ideas or concepts embedded within, or underpinning, the explicit content (e.g, resistance to engaging in superwoman/supermum position). Millers did not talk explicitly about a supermum/superwoman discourse – the notion that women should be able to be primary caregivers of their children, hold down a full time job, and still do both with high levels of competence (e.g. Sasaki and Hazen, 2010). However, this concept was useful for making sense of her logic when she talked about ‘spreading herself thin’ – an idea that is part of the discourse. The coding process is iterative and flexible, and code revision and development is part of this. Codes developed later in the process might capture a particular concept more clearly than earlier ones, and researchers tend to refine and revise codes throughout the process – it pays not to get too attached too early on (this is a bit of a mantra for doing our version of TA). The researcher often circles back through data items to clarify, or modify, earlier coding, which also helps with coding consistency – avoiding having hundreds or even thousands of unique codes with lots of overlap. Coding is there to help the analyst make sense of the data, develop insight, and provide a rigorous and thorough foundation for the analysis (it can also help to tighten or modify a research question). In terms of the practicalities of coding, we recommend researchers use whatever method works best for them: write codes in the margins of hard copies of the data items; use Microsoft Word’s comment function; use computer software designed for qualitative coding (see Chapter 23 to tag and collate data. People also use file cards, or cut and paste (either physically or digitally) data segments into new files or onto clean pages. Recently, we have seen people start to claim that computer programs provide the best way to code. We definitely do not agree with this sentiment as a generic position. Any researcher needs to identify the right tools for them, in the context of their particular project. For instance, software might facilitate code sharing and development in a large team project; a low-tech researcher working on a small individual project may find file cards work best for them. Coding is a process not a technology, and the same quality can be achieved through various means. Poor quality coding is thin, with limited interpretative work, and/or sloppy – inconsistent and partial; good quality coding is the opposite, deep, consistent and thorough. After coding all data items thoroughly, this phase ends with the production of a compiled list of codes that adequately identify both patterning and diversity of relevant meaning within the dataset. Collating associated tagged data segments is the last task before moving on to theme development. Theme development (phase 3) Establishing a deep understanding of the dataset through familiarisation and coding sets up the researcher well to begin constructing themes. Rather than describing themes as ‘emerging’ (like Mr Darcy from a murky pond in the BBC version of Pride & Prejudice), we think of this phase as a very active process of pattern formation and identification. The researcher now builds on earlier engagement to shape a first version of salient patterning in the data. The research question acts as a guide for this, as it helps determine what is, and what is not, relevant in terms of potential clusters of patterned meaning. It provides a foundation for the researcher, as they make choices about what data segments are relevant, and what is important to say about them, ensuring the themes tell a coherent and relevant story about the data. As qualitative data are often rich and enticing, it is easy to get lost in analysis; keeping oriented to the research question helps keep the analysis relevant. Theme development first involves examining codes (and associated data), and combining, clustering or collapsing codes together into bigger or more meaningful patterns. Sometimes, this is as simple as identifying a rich and complex code that potentially captures a number of other codes within its boundaries – such codes can be ‘promoted’ into a provisional theme (Charmaz, 2000). More commonly, thinking and effort is required to identify features of similarity and relationship across a range of different codes that means they can be clustered together into a possible theme. In this process, the researcher needs to identify a central organising concept – a ‘clear core idea or concept that underpins a theme’ (Braun et al., 2015, p. 102) – that is shared across the range of codes. This central organising concept helps the researcher determine what a theme is all about, and whether or not any particular code fits within it. This is not because of some essence or hidden value that the researcher uncovers; rather it helps the researcher gain clarity about what sense they are making of the codes and the data. Key to remember at this stage is that patterning has to be identified across your dataset – not just within a single data item. At this point in the analysis, it is really easy to get attached. But it is extremely rare that first attempts at theme development will produce a final thematic mapping. If themes emerged preformed, this might be an understandable way of looking at the process. However, as we view themes as constructed or generated through a productive, iterative, reflective process of data-engagement, it makes more sense to treat each clustering of codes as possibilities. At this stage, they are provisional or ‘candidate’ themes – imagining them as candidate themes gives the researcher the opportunity to discard them, to explore other possibilities, before eventually settling on a final set of themes. In order to facilitate this process of shifting mapping of various patterns, we encourage researchers to make use of visual aids, such as thematic maps (see Figure 2.1) (see also, Braun and Clarke, 2013; Braun et al., 2015) or tables (see Table 2.4). As with coding, such (visual) mapping aids are tools that enhance the researcher’s ability to identify and understand potential themes in relation to each other, and the overall dataset. Such tools provide a way of identifying what the boundaries of, and the relationships between, each theme might be, as well as how different themes work together to tell an overall story about the data. Good quality themes should be distinctive, with little ‘bleeding’ of codes between themes; themes should also be linked to, and work alongside, the other themes in the analysis – and each needs to have its own distinct central organising concept. Which mapping tools the researcher uses to construct themes, how they work with those tools, and whether they ever present the maps or tables in a research write-up, is dependent on what works best for them – and the context of the study (we, for instance, would be unlikely to report thematic maps, unless we were writing a methodological piece, like this one, but encourage undergraduate and postgraduate students to do so in the appendices of their projects). [TS: Insert Figure 2.1 about here] Figure 2.1 An early thematic map [TS: Insert Table 2.4 about here] Table 2.4 Four candidate themes from the child -freedom study, with example codes In the child-freedom study, the idea of a ‘precarious identity’ noted earlier continued to appear across the dataset. Women spoke of the decision to be childfree as one that wasn’t always straightforward, linear, or even at times, coherent. One of the women, Mary, spoke of this ‘precariousness’ in terms of percentages: ‘We might have a really nice interaction with a child and you’re like “ooh I’m seventy-five percent today” or “sixty percent today”’ (see Box 2.6 below). Thus, following a thorough coding, we did have evidence of a prevalent pattern across the data (a large number of data extracts across different interviews), and therefore a potential candidate theme – this addressed an important aspect of the lived experiences of childfree women. Two other candidate themes we generated also related to the lived experiences of childfree women: first, that children would interfere with the freedoms and quality of life the women enjoyed; second, that living in a pronatalist society meant they experienced marginalisation, but simultaneously, they would often deny any explicit stigma. All three themes spoke, centrally, to our research question, which was to ‘explore the lived experience of voluntary childlessness across the life course for a diverse group of women’. A fourth candidate theme ‘not a maternal bone in my body” (see Table 2.4) captured a prevalent meaning, but proved not to be of value to the research question, because it related more to women’s initial reasons for not having children, rather than to their current lived experiences of being childfree. This discrepancy was addressed as we reviewed and defined our (candidate) themes. Reviewing and defining themes (phases 4–5) With codes collated and a table or thematic map of candidate themes developed, the researcher can be tempted to draw the conclusion that they’ve got their themes, and all that’s required is a little polishing. However, the analysis so far has only developed candidate themes – the next phases of reviewing and defining are a vital a part of the TA process, as the themes are further shaped, clarified, or even rejected. The reviewing phase is like a quality control exercise, to ensure that the themes work well in relation to the coded data, the dataset, and the research question. In some instances, the review of themes alongside the dataset confirms that they actually do work well and tell a distinctive and meaningful story that answers the research question. If so, fantastic. But more commonly, and particularly for novice analysts, or those working with larger datasets, the review process leads to adjustments to the candidate themes and/or thematic map, or even considerable further analytic work – sometimes at this stage, the researcher even starts theme development afresh. The first stage of review involves checking whether your candidate themes capture the meaning in the collated, coded data segments. Does the analysis work at this level? For each candidate theme, this means that all the data extracts that this theme represents are clearly related to the central organising concept of the theme, and that the diversity of meaning around this central organising concept is captured. This applies for each candidate theme, and across all the candidate themes. Basically, each theme need to ‘account for’ the important things captured by the coded data relevant to the central organising concept, and the range of those important meanings, and each theme needs to be distinctive. Mismatch between what is contained in data extracts, and what the researcher claims those extracts demonstrate (which could reflect poor coding, or poor theme development), either produces a poor quality analysis, or a headache for the researcher later, when they have to redo the analysis. This reviewing phase also requires the researcher to check that their candidate themes work well across the whole dataset – so going back to the entire dataset, rather than just working with the collated coded segments. The analysis has moved on since coding; rereading the dataset helps ensure nothing has been missed, and that as the analysis has been developed, it has not moved too far away from the key (often most prevalent) stories in the data. It asks the researcher to evaluate whether their themes meaningfully and usefully capture what the dataset itself (Braun and Clarke, 2006). There is a balance between making sure that themes are distinct from each other, and ensuring that they relate to each other. The research builds an overall story about the data through the themes they present. If themes are distinctive, most of the codes will only be allocated to one theme. If many are allocated to more than one theme, they risk blurriness – note that this is not the same as having the same segment of data coded in different ways. Reviewing analysis involves making choices about the best and sharpest boundaries for inclusion and exclusion. In our analysis of the child-freedom data, we could have included the code 'no biological urge' in the 'precarious identity' theme, as the data (partly) related to women's discussions of certainty about being childfree. However with review, there were enough data and diversity around this code to develop it as a theme in its own right – it became ‘not a maternal bone in my body’. This example demonstrates the utility of the central organising concept in theme review: the central organising concept of ‘not a maternal bone in my body’ is a ‘fundamental’ lack of desire for children, which was often articulated as something biological. This central concept is quite distinct from that of the ‘precarious identity’ theme (discussed above). Our finalised thematic map (Figure 2.2) visually captures this development in our TA from the earlier map, based on our review process. [TS: Insert Figure 2.2 about here] Figure 2.2 A final thematic map The final review consideration is whether the story told through the themes answers the research question. If the analysis is strong and captures the meaning in the data well, but does not quite fit the research question, this may mean tweaking the research question, rather than restarting the analysis. How is that not cheating? The open and unanticipated nature of data in qualitative research means the researcher’s imagining of the data they will get – which is captured by the research question – often falls somewhat off the mark. The stories the researcher hears from participants, for instance, can show that the original research questions were limited, or did not quite provide the best question to capture the data. This openness and flexibility to shift focus is one of the joys of qualitative research, and one of the reasons why researchers value it over more fixed or closed response modes common in quantitative research. The reviewing process is continued as the researcher moves into defining and naming themes. By this point in the analysis, the researcher should have started to move away from a summative position (thinking about these themes as lists of codes and collated data) to an interpretative orientation. This involves telling a story that is based on, and about, the data, that makes sense of the patterning and diversity of meaning. This involves writing the analysis – the analytic narrative that encases the presented data extracts. This phase is about ensuring clarity, cohesion, precision and quality of the developing TA. If themes are still not clear, the practice of writing short theme definitions can help determine clarity and scope (content) for each theme, and provide a stronger sense of each ‘chapter’ of the analytic story, and is also a good time to double check that each theme coheres tightly around a central organising concept. Theme definitions are short summaries of the core idea and meaning of each theme – like an abstract for each theme (see Box 2.5). The process of writing definitions can confirm whether there is enough depth and detail for each theme to stand alone as a key chapter in the analytic story. If a theme is too thin, there will not be much to say. If it’s a bit like listening to one music track over and over, rather than a playlist of great songs, the theme probably is not working as it should. A theme, since it captures richness and diversity around the core meaning, should provide lots to write about (as the example in Box 2.5 shows), rather than a line or two. A thin theme might need to be dropped from the analysis, unless it can be expanded and enriched by going back to the coded data/dataset without being forced. Another question is whether it might work as a subtheme. A subtheme captures a distinct aspect of a theme, but shares the same central organising concept – hence it is not a standalone theme. The theme definition exercise might also reveal the opposite: too much to say about the data, which may mean the meanings contained are too complex, or they lack coherence and a unifying thread. If so, revision might involve splitting a theme, developing clear subthemes, or discarding it. The quality checklist for good TA (see Table 2.5 below) offers useful pointers around this. [TS: Insert Box 2.5 about here] Box 2.5 An example of theme definition for ‘childfree as a precarious identity’ Most researchers will have given their themes working titles – titles that are far more engaging than ‘theme 1’, ‘theme 2’. We suggest (again) keeping these as ‘working titles’ until this final stage. Some of us really like engaging, sometimes witty, theme names. But coming up with these early on, means we can find it hard to let go of them, even when they do not work well with the finalised themes. Researchers should ask whether another – or a refined – name better captures each theme. In the child-freedom study, the theme ‘a precarious identity’ was originally called ‘ambiguous identity’. As we developed our analysis and defined the theme more clearly, it became apparent that the women did not feel ambiguous about their decision per se; they were clear and confident in their decision not to have children in the present time. Rather, at other points in the interview, many seemed to be open to reconsidering their decision if circumstances changed in the future (see Box 2.5). The term ‘precarious’ better suited the content of the data than the word ‘ambiguous’, because although the identity was clear now, it wasn’t necessarily fixed for eternity. Theme names need to give a clear indication of the content within the theme, and draw the reader into the analysis. Given our noted predilection for fun names, we like incorporating data quotations, or using alliterations or plays on words in theme names. But straightforward descriptive theme names work better than a ‘fun’ name that does not capture the theme. Producing the report (phase 6) At the point the researcher gets to produce the report, they will have already done a lot of writing – qualitative analysis involves writing from early on. Familiarisation notes, codes, theme definitions, and a broad range of other writing, all help in the development of the final analysis, be it a journal article, short report, or dissertation/thesis. The reason we discuss this as a separate phase for TA is that there is a distinct final period of focus and refinement, where the researcher weaves together data, analysis, and connections to scholarly (and other) literature into a singular output that answers their research question(s). Here, the researcher moves from a ‘purely’ analytic point in the research process, coming back to the bigger picture of the overall project. A key decision – which often is effectively made earlier in the analytic process – is how any quoted data extracts are treated. Broadly, there are two styles for writing around data in TA: illustrative and analytic. If data extracts are used primarily as examples within the analytic narrative, evidence from the data that illustrates key elements of the story, they are being used illustratively. Writing analytically about the data is quite different: particulars of extracts are discussed by the researcher, with specific aspects or features forming the basis for analytic claims. Many analyses contain both styles, and there is often some blurring between the two, such as some analytic commentary about extracts otherwise used illustratively. Our example in Box 2.6 illustrates both style in relation to the ‘precarious identity’ theme. We treat the first two extracts illustratively: Mary and Annie’s words provide examples of the points made in our analytic write-up. We treat Louise’s account initially in an illustrative way, but we then shift to an analytic style, making analytic points about specific details of her data extract, and building connections to literature. A simple test illustrates the difference between these styles: can you remove an extract and have the narrative still make sense? If yes, the style is illustrative; if no, analytic. Mary and Annie’s extracts in Box 2.6 could be removed and the reader would still follow the argument; the analytic writing following Louise’s extract only makes sense if the reader can see her quotation. [TS: Insert Box 2.6 about here] Box 2.6 Excerpt from the write-up of the ‘precariousness’ theme showing data extracts used illustratively and analytically Quality When supervising students and looking at published examples of TA, we often identify problems – many of which we have discussed above. One of the most common problems is inconsistency between the theoretical approach claimed by the researcher and the analysis that is actually presented. For example, new-to-qualitative researchers might claim their research has been informed by a social constructionist perspective, yet the way they analyse their participants’ words treats language as though it is a direct conduit to the participants’ experience (i.e. a realist perspective). For people new to such theoretical frameworks, a way to tackle this kind of problem is to spend time reading peer-reviewed literature from that position – it can also be good to look at the counter-position as well. This helps identify the ways writers describe their participants’ words and the claims they make from them. We have also noticed the use of combinations of different versions of TA, which are theoretically and/or methodologically incompatible (e.g. discussing a code book when the author’s claims to be doing TA according to Braun and Clarke, 2006). Another issue we see, from particularly novice analysts, is ‘themes’ which are actually the questions participants have been asked about – such ‘themes’ usually contain quite diverse or even contradictory answers to the questions, rather than reporting a shared, cohesive meaning across the dataset. Another common problem is when features of the data, or data domains, are presented as themes (Connelly and Peltzer, 2016; Sandelowski and Leeman, 2012). For example, talk about biology was a feature of the child-freedom data, but without additional content related to how biological explanations were used to frame childfree positions, ‘biology’ on its own was not particularly useful. More analysis is needed in such instances to draw out what about these features may be interesting and important, and to develop a theme around a clear central organising concept. Our checklist for good TA (see Table 2.5) is a useful shorthand guide to ensure an analysis that is rigorous and robust (Braun and Clarke, 2006). [TS: Insert Table 2.5 about here] Table 2.5 15-point checklist for a good TA (Braun and Clarke, 2006, p. 96) Critical appraisal What are the strength and weaknesses of TA? As noted previously, TA has been critiqued for not really being a particular or distinctive method, but as simply referring to a process for identifying patterns, something common to many qualitative approaches. Such critique relies on the conflation of method and methodology, where the former refers to tools for data analysis (as is the case for TA), and the latter refers to broader frameworks for research, which more or less predetermine factors like theoretical frameworks, orientation to data, modes of data collection, and so on. As we have discussed elsewhere (Braun and Clarke, 2013), most approaches to qualitative data analysis are really methodologies, and many of those oriented to identifying patterning in data do, indeed, share similarities in process with TA. But we do feel that TA offers qualitative researchers something specific (if not entirely unique) – a process for ensuring rigorous and systematic engagement with data, to develop a robust and defensible analysis, that is independent of any predetermined particular theoretical framework or cluster of other design considerations. A bigger – but not unrelated – limitation relates not to what TA is, but to how it is sometimes used. In describing TA as a tool, we do not advocate its use outside of theory, or outside of interpretation – in purely descriptive, or in effectively summative, ways. But we see this happening. We fear that the idea that (good quality) qualitative research can proceed without a theory of what language is, and where meaning resides, and, indeed, what the relationship between the two is, stems from a paucity of engagement with qualitative research in learning environments. It may also be related to a failure to interrogate the commonsense idea that language is simply of mode of communication, reflective of reality (objective or subjective) and experience. We believe that we need to do better than that as a community of scholars, and ensure the theoretical foundations of our work are explicated – even if only briefly. However, by thinking about theory as a co-requisite, rather than a pre-requisite, TA can provide an easy entry-point into the wonderfully diverse world of qualitative researching. The clear set of steps our version of TA provides are also particularly helpful for the beginner: like the handrails around the edge of an ice rink, they provide a reassuringly firm grip when the world feels like it’s slipping away in someone’s first attempts on the ice. With experience, there is less need to cling to them in the same way, but they provide a foundation and guide that bounds good practice. Applications TA has been used in almost every conceivable field of scholarship in the social and health sciences, and suits any subdiscipline and area where general qualitative research questions about experience, understanding, social processes, and human practices and behaviour make sense. We have noticed, in particular, a widespread uptake of TA in some of the applied areas of psychology – where researchers potentially have more realist-oriented research questions, and where qualitative research is often part of a larger mixed-method project, or directly intersects with policy development. It is rewarding to see that TA has clear value for such researchers, and offers a tool useful to practical application. But a more critical perspective is, however, often overlooked – possibly because, as noted at the start of the chapter, others writing around TA often situate it as a realist method. This is a shame, as the approach as we have articulated it works just as well within critical fields of scholarship – such as critical health and social psychology, or critical sexuality studies. Indeed, our own use of the approach predominantly falls within this camp (e.g. Adams et al., 2013; Braun, 2008; Braun and Clarke, 2006; Braun and Clarke, 2012; Braun et al., 2015; Braun et al., 2009; Braun et al., 2013; Clarke et al., 2015b; Clarke et al., 2015b; Clarke and Smith, 2015; Hayfield, 2013; Opperman et al., 2014; Terry and Braun, 2011, 6). Future directions Fast-becoming one of the most widely used methods of analysing qualitative data, we do not see a future where TA disappears. However, we anticipate clearer articulation of different versions of TA, and clarification of how approaches differ, and what similarities they share. We have also come across TA being used to conduct literature reviews, or for qualitative synthesis and systematic reviews (e.g. Cruzes and Dybå, 2011; Thomas and Harden, 2008), and for case study research (e.g. Cedervall and Åberg, 2010), and combining TA with other method (such as narrative analysis (Ronkainen et al., 2016) and visual analysis (Ponnam and Dawra, 2013)), and these are areas that need further reflection. We are not sure case studies and qualitative synthesis really count as TA, and the theoretical and conceptual basis for identifying patterned meaning within both academic scholarship, and single cases, is somewhat different to identifying and interpreting patterned meaning in and across data. So, despite some questions to be resolved, including around conceptual muddiness and confusion, we see the future of TA as bright. References Adams, J., McCreanor, T., and Braun, V. (2013). Gay men’s explanations of health and how to improve it. Qualitative Health Research, 23(7), 887–899. doi:10.1177/1049732313484196 Basten, S. (2009). Voluntary childlessness and being Childfree. The Future of Human Reproduction: Working Paper, 5, 1-23. Baxter, L. (1991). Content analysis. In B. Montgomery and S. Duck (Eds.), Studying interpersonal interaction (pp. 239–254). New York: The Guilford Press. Bennett, E., and Gough, B. (2013). In pursuit of leanness: The management of appearance, affect and masculinities within a men’s weight loss forum. Health, 17(3), 284–299. doi:10.1177/1363459312454149 Blackstone, A., and Stewart, M. D. (2012). Choosing to be childfree: Research on the decision not to parent. Sociology Compass, 6(9), 718-727. Boyatzis, R. (1998). Transforming qualitative information: Thematic analysis and code development. Thousand Oaks, CA: Sage. Braun, V. (2008). ‘She’ll be right’? Lay attributions of cause around sexual health in Aotearoa/New Zealand. Social Science & Medicine, 67(11), 1817–1825. Braun, V., and Clarke, V. (2006). Using thematic analysis in psychology. Qualitative Research in Psychology, 3(2), 77. doi:10.1191/1478088706qp063oa Braun, V., and Clarke, V. (2012). Thematic analysis. In H. Cooper (Ed.), APA handbook of research methods in psychology (Vol. 2, pp. 57–71). Washington, DC: American Psychological Association. Braun, V., and Clarke, V. (2013). Successful qualitative research: A practical guide for beginners. London: Sage. Braun, V., Clarke, V., and Terry, G. (2015). Thematic analysis. In P. Rohleder and A. Lyons (Eds.), Qualitative research in clinical and health psychology (pp. 95–113). Basingstoke: Palgrave MacMillan. Braun, V., Terry, G., Gavey, N., and Fenaughty, J. (2009). ‘Risk’ and sexual coercion among gay and bisexual men in Aotearoa/New Zealand-key informant accounts. Culture, Health & Sexuality, 11(2), 111–124 Braun, V., Tricklebank, G., and Clarke, V. (2013). ‘It shouldn't stick out from your bikini at the beach’: Meaning, gender, and the hairy/hairless body. Psychology of Women Quarterly, 37(4), 478–493. doi:10.1177/0361684313492950 Cedervall, Y., and Åberg, A. C. (2010). Physical activity and implications on well-being in mild Alzheimer's disease: A qualitative case study on two men with dementia and their spouses. Physiotherapy theory and practice, 26(4), 226-239. doi: 10.3109/09593980903423012 Charmaz, K. (2000). Grounded theory methodology: Objectivist and constructivist qualitative methods. In N. Denzin and Y. Lincoln (Eds.), SAGE handbook of qualitative psychology (2nd Ed.) (pp. 509–535). Thousand Oaks, CA: Sage. Christ, T. (1970). A thematic analysis of the American business creed. Social Forces, 49(2), 239–245. doi:10.1093/sf/49.2.239 Clarke, V., and Smith, M. (2015). ‘Not hiding, not shouting, just me’: Gay men negotiate their visual identities. Journal of Homosexuality 62(1), 4–32. Clarke, V., Braun, V., and Hayfield, N. (2015a). Thematic analysis. In J. Smith (Ed.), Qualitative psychology: A practical guide to research methods (3rd ed.) (pp. 222–248). London: Sage. Clarke, V., Braun, V., and Wooles, K. (2015b). Thou shalt not covet another man? Exploring constructions of same-sex and different-sex infidelity using story completion. Journal of Community & Applied Social Psychology, 25(2), 153–166. doi:10.1002/casp.2204 Connelly, L. M., and Peltzer, J. N. (2016). Underdeveloped themes in qualitative research: Relationship with interviews and analysis. Clinical Nurse Specialist (January/February) 30(1), 51–57. Cruzes, D. S., and Dybå, T. (2011). Recommended steps for thematic synthesis in software engineering. International Symposium on Empirical Software Engineering and Measurement (pp. 275–284). doi. 10.1109/ESEM.2011.36 Dapkus, M. (1985). A thematic analysis of the experience of time. Journal of Personality and Social Psychology, 49(2), 408–419. Fereday, J., and Muir-Cochrane, E. (2006). Demonstrating rigor using thematic analysis: A hybrid approach of inductive and deductive coding and theme development. International Journal of Qualitative Methods, 5(1), 80–92. Frith, H. (2015). Sexercising to orgasm: Embodied pedagogy and sexual labour in women’s magazines. Sexualities, 18(3), 310–328. doi: 10.1177/1363460714550912 Gibson, W., and Brown, A. (2009). Working with qualitative data. London: Sage. Giles, D., Shaw, R. L., and Morgan, W. (2009). Representations of voluntary childlessness in the UK press, 1990–2008. Journal of Health Psychology, 14(8), 1218–1228. doi:10.1177/1359105309346341 Glaser, B., and Strauss, A. (1967). The discovery of grounded theory. London: Weidenfeld and Nicholson. Grant, B. M., and Giddings, L. S. (2002), Making sense of methodologies: A paradigm framework for the novice researcher. Contemporary Nurse, 13(1), 10–28. Guest, G., MacQueen, K., and Namey, E. (2012). Applied thematic analysis. Thousand Oaks, CA: Sage. Hayfield, N. (2013). ‘Never judge a book by its cover?’: Students’ understandings of lesbian, gay and bisexual appearance. Psychology & Sexuality, 4(1), 16–24. doi:10.1080/19419899.2013.748261 Holton, G. (1975). On the role of themata in scientific thought. Science, New Series, 188(4186), 328–334. Joffe, H. (2012). Thematic analysis. In D. Harper and A. Thompson (Eds.), Qualitative methods in mental health and psychotherapy: A guide for students and practitioners (pp. 209–223). Chichester: Wiley. Kidder, L., and Fine, M. (1987). Qualitative and quantitative methods: When stories converge. New Directions for Program Evaluation, 1987(35), 57–75. doi:10.1002/ev.1459 Langridge, D. (2004). Introduction to research methods and data analysis in psychology. Harlow: Pearson. Leeming, D., Williamson, I., Lyttle, S., and Johnson, S. (2013). Socially sensitive lactation: Exploring the social context of breastfeeding. Psychology & Health, 28(4), 450–468. Moller, N., and Vossler, A. (2015). Defining infidelity in research and couple counseling: A qualitative study. Journal of Sex & Marital Therapy, 41(5), 487–497. Neville, S., Adams, J., Bellamy, G., Boyd, M., and George, N. (2015). Perceptions towards lesbian, gay and bisexual people in residential care facilities: A qualitative study. International Journal Of Older People Nursing, 10(1), 73–81. Niland, P., Lyons, A., Goodwin, I., and Hutton, F. (2014). ‘See it doesn’t look pretty does it?’: Young adults’ airbrushed drinking practices on Facebook. Psychology & Health, 29(8), 877–895. Opperman, E., Braun, V., Clarke, V., and Rogers, C. (2014). ‘It feels so good it almost hurts’: Young adults’ experiences of orgasm and sexual pleasure. The Journal of Sex Research, 51(5), 503–515. Peterson, H., and Engwall, K. (2013). Silent bodies: Childfree women’s gendered and embodied experiences. European Journal of Women's Studies, 20(4), 376-389. doi: 10.1177/1350506812471338 Ponnam, A., and Dawra, J. (2013). Discerning product benefit through visual thematic analysis. Journal of Product & Brand Management, 22(1), 30–39. Potter, J., and Wetherell, M. (1987). Discourse and Social Psychology: Beyond Attitudes and Behaviour. London: SAGE Publications. Retrieved from http://books.google.co.uk/books?id=oHFIN4xiHW8C Reicher, S. (2000). Against methodolatry: Some comments on Elliott, Fischer, and Rennie. British Journal of Clinical Psychology, 39(1), 1–6. doi:10.1348/014466500163031 Ronkainen, N. J., Watkins, I. and Ryba, T. V. (2016). What can gender tell us about the pre- retirement experiences of elite distance runners in Finland? A thematic narrative analysis. Psychology of Sport and Exercise, 22, 37–54. Sandelowski, M., and Leeman, J. (2012). Writing usable qualitative health research findings. Qualitative Health Research, 22(10), 1404–1413. Sasaki, T., and Hazen, N. (2010). The supermom trap: Do involved dads erode moms’ self- competence? Personal Relationships, 17(1), 71–79. Shaw, R. (2010). Embedding reflexivity within experiential qualitative psychology. Qualitative Research in Psychology, 7(3), 233-243. doi:10.1080/14780880802699092 Smith, J. (1996). Beyond the divide between cognition and discourse: Using interpretative phenomenological analysis in health psychology. Psychology & Health, 11(2), 261–271. Terry, G., and Braun, V. (2011). ‘It’s kind of me taking responsibility for these things’: Men, vasectomy and ‘contraceptive economies’. Feminism & Psychology, 21(4), 477–495. doi:10.1177/0959353511419814 Terry, G., and Braun, V. (2012). ‘Sticking my finger up at evolution’: Unconventionality, selfishness, resistance and choice in the talk of men who have had ‘pre-emptive’ vasectomies. Men and Masculinities, 15(3), 207–229. Terry, G., and Braun, V. (2016). ‘I think gorilla-like back effusions of hair are rather a turn- off’ – men, body hair removal and the concept of ‘excessive’ hair. Body Image, 17, 14– 24.doi: 10.1016/j.bodyim.2016.01.006 Thomas, J., and Harden, A. (2008). Methods for the thematic synthesis of qualitative research in systematic reviews. BMC Medical Research Methodology, 8(45), http://www.biomedcentral.com/1471-2288/8/45 Wetherell, M. (1998). Positioning and interpretative repertoires: conversation analysis and post-structuralism in dialogue. Discourse & Society, 9(3), 387–412. Willig, C. (2013). Introducing qualitative research in psychology (3rd Edition). Maidenhead: Open University Press. Yardley, L. (2008). Demonstrating validity in qualitative psychology. In J. Smith (Ed.), Qualitative psychology: A practical guide to research methods (2nd Ed.) (Vol. 2, pp. 235– 251). London: Sage. Box 2.1 Introducing the lived experiences of childfree women (child-freedom) study Women’s choice to be childfree has recently become a focus of both academic and social interest in Western contexts. Researchers have identified a motherhood ‘imperative’ or ‘mandate’ in the wider culture (Giles, Shaw and Morgan, 2009), which creates an expectation that all women want, and have, children (Basten, 2009). The pervasiveness of the motherhood imperative is such that women who remain voluntarily without children are often perceived as deviant, maladjusted, emotionally unstable, unfeminine, unnatural, unhappy, immature, selfish and self-centred, and less sensitive or loving than women with children (Blackstone and Stewart, 2012). However, minimal research has explored women's experiences of being childfree (Peterson and Engwell, 2013). Little is understood of how childfree women negotiate and make sense of their identities and life course in relation to a supposedly stigmatised status and in a strongly pronatalist socio-cultural context. This project aimed to offer such insight. Our research team (Nikki Hayfield, Victoria Clarke, Sonja Ellis and Gareth Terry) interviewed 23 UK-based women across a number of social categories (e.g. sexuality, class, country of origin, age), to help understand their diverse and shared experiences and lives as childfree women. Data were analysed using TA within a critical realist framework, and meaning and experience were examined at both semantic and latent levels. Box 2.2 Semantic versus latent codes Semantic (or descriptive) codes identify and summarise the content of the data. They are built around what participants say, mirroring their meanings. They capture the surface meanings of the data, but that does not need to equate to a superficial or purely descriptive reading. In the selection of codes in Table 2.3, semantic codes include ‘having children limits capacity’ and ‘invested in being competent at job’. Latent (or interpretative) codes go beyond participant-expressed meanings, to the underlying patterns/stories in the data. They tend to bring the analyst’s theoretical frameworks to bear on the data, and are built around concepts that help explain the data, and thus require more interpretation or insight. In Table 2.3, some latent codes including ‘still engaging in features of the feminine role’ and ‘reluctance to engage in superwoman/supermum position’. Box 2.3 Familiarisation notes from one interview and the entire dataset Examples of familiarisation notes from interview with P17 (Pseudonym ‘Millers’) -Hold strong opinion that pregnancy will ruin your life. -She loves working with children, but does NOT want them at home. -Sleep, silence, and space are all-important. -Speaks a lot about her independence and unconventionality, a lot of examples deployed. -Demands excellence from herself – couldn’t live current life with children without something giving way. -Views children as innately good, but would only settle for a great one. Gamble is too much. -Being grown up is about responsibility, putting yourself second. -Sees others who have had children, lives not ruined, but different expectations of self. Examples of familiarisation notes for the entire dataset -Often quite individualised, neo-liberal rhetoric, being childfree framed simply as a choice/lifestyle choice. -Elsewhere there is contradictory framing: Born that way/essential ontological state (non-accountable, no agency). -Precariousness of the stories, not a central identity, not a master status, not an identity category. -Being childfree gives freedom, flexibility and control (but really unclear what this is from or for). -Criticisms of contemporary parenting culture. -Deterioration of children’s behaviour in recent decades – children are spoilt and over- indulged (and this makes children unlikeable/this is why I don’t like children), all children are a constant noise and relentless, children keep coming back, you can’t get rid of them (out of the house) these days. -Not stigma in an obvious sense of being abused or harassed, but a sense of childlessness being stigmatised/unspeakable. -Stories of old people’s homes and people not being visited by children, expecting a fully competent and able old age. Box 2.4 What you see in the data (to some extent) reflects who you are Reflexivity is an important element of qualitative research because it enables researchers to consider the (inevitable) impact they have on data collection and analysis (Shaw, 2010). In order to be reflexive, it is useful to consider personal interest in the topic. Nikki: I do not want children, and have been intrigued by the contentiousness of childlessness. I have encountered strong responses to my decision that have consisted of two diametrically opposed reactions. Some have dismissed my decision and seemed to want to convince me that I would (and should) change my mind. Others, usually those who also did not want children, were particularly interested in my decision and keen to talk about the topic. My own personal experiences sensitised me to particularly noticing accounts in the dataset where participants reported that others had dismissed and challenged their decision to be childfree, because I had similar experiences. This was helpful because it provided me with insight into the data. On the other hand, women’s accounts of being childfree that were very different to my own also stood out. I became particularly interested in some of these women negotiating their ongoing relationships when their partners wanted children - this was not an issue I had encountered, but one I would find difficult. What I noticed then, was partly informed by my own experiences and whether participants’ accounts were resonant or discordant to mine. My position was a strength in that I had some particular insights that the rest of the team may not have had. However, were I working alone, or not being thorough, this could become a weakness, as there could be a risk that I overlooked some aspects of the dataset that were less striking in relation to my own experiences. As a research team we all noticed some patterns in the data, and all contributed our own additional ‘noticings’, informed by our own perspectives and our wider academic knowledge of the topic. Gareth: My partner and I have been working through the decision not to have children for some time. As a small part of this process, I included men who had had ‘pre-emptive’ vasectomies in my PhD research (see Terry and Braun, 2012), to try and academically 'unpick' why other men had made a decision I was personally interested in. Due to this academic and other personal experience, I had a number of ideas about ‘childfree’ as a category coming into the project. But these ideas were not always reflected in the data we were generating. One of these idea ‘conflicts’ was surprising to me: the identities of the women seemed more fluid, or they felt more ambiguous about their decision, than the men that I had interviewed in the previous project. I started to see this everywhere, and was sure it would end up being a theme. This had the potential to be a trap, and one that new players (and even experienced researchers pressed for time!) can easily fall into. Being part of a diverse team of people, with various levels of experience, helped here, as we were able to put the brakes on and examine what was going on together, bringing the insights of our experience and various social positions (mine being heavily marked as privileged: white, ostensibly middle class, heterosexual, and male) to the data. Although the kernel of the idea I was seeing was prevalent, it was only a very small part of the overall picture. Through the systematic work of coding and bringing our broader theoretical understandings to the data we were able to identify a meaningful pattern with greater explanatory power. Box 2.5 An example of theme definition for ‘childfree as a precarious identity’ Our participants self-identified as childfree by choice and at some points in the interviews they were clear and confident about the absolute certainty of their decision to be childfree. However, in other parts of the interviews, it became clear that they were open to possible change in the future – they were ‘keeping their options open’. For example, some women talked about not being willing for they, or their partners, to be sterilised. They also articulated the relevance of their partner, or future partners, in their decision to be childfree. They made clear that their relationships were of great importance to them, such that a compromise about children may become necessary to maintain the relationship. It was clear that their childfree identity existed within the wider context of a pronatalist society. Women with children, and others including authoritative figures such as doctors, reiterated to them the possibility that they could (and should) change their minds. This meant that while they articulated their decision as permanent, they also reflected on their childfree identities, and described them (also) as potentially negotiable. Hence their position as childfree was provisionally open to possible change, and not as fixed as it may have first seemed at the beginning of the interview. There was liminality to their childfree identity; it was an identity category that was worked, reworked, and constantly in negotiation, and in this way, it seemed precarious rather than entrenched. Box 2.6 Excerpt from the write-up of the ‘precariousness’ theme showing data extracts used illustratively and analytically Many of the women spoke about their childfree identity as something that began forming at a very early age, or even something they had been ‘born with’. This bears some similarity to the accounts of men in Terry and Braun’s (2012) study, who argued their vasectomies were simply a physical manifestation of a longstanding (and complete) rejection of fatherhood. However, in contrast to these men, almost all of the female participants in our study discussed moments of precariousness in their childfree identity. For instance, Mary and her male partner would speak about their ‘sureness’ in terms of percentages: Mary: We might have a really nice interaction with a child and you’re like ‘ooh I’m seventy-five percent today’ or ‘sixty percent today’ (36, white, middle class, heterosexual). In many cases, the significance of these moments of precariousness would be minimised, as if they were simply brief moments, easily moved past. Annie spoke of a desire for children as like a ‘radio signal’ that would sometimes come into range, but would disappear just as quickly: Annie: [S]ometimes when I’ve been in the supermarket and I see a gorgeous lit- I mean just today I was I saw a little gorgeous little girl going all mushy with her mother and I thought ‘how sweet’ and I just, and then it I just moved on to think of something else. It’s like that thing of ‘ooh I fancy some ice cream’ and five minutes later you’ve completely forgotten about it (58, Jewish, middle class, heterosexual). For others though, it was a little more complicated. Even when they had no strong interest in children, external factors, such as a partner desiring children, would create a precariousness that was harder to dismiss. For instance: Louise: I don’t regret my decision though Int: Yeah but it it’s been a pretty constant thing? Louise: It’s been a constant thing. I mean at one point I was in a relationship with another woman who really wanted a child and so I was trying to help her get pregnant and I felt very, very ambivalent through that process because I knew she wanted me to co-parent and I wasn’t that comfortable with it (59, white, middle class, lesbian). Here, Louise describes her internal state, her own orientation toward being childfree, as ‘constant’. Elsewhere in the interview, she pinpointed a moment at twelve years old as her first point of awareness of not wanting children. In this account though, the desire of her partner to have children created conflict with her ‘internal’ state. Despite strong assertions of the constant nature of her childfree identity, she describes her co-parenting in minimally resistant terms (‘I wasn’t that comfortable with it’). Such ‘moments’ can be understood in terms of the dominance of the ‘motherhood mandate’ (Giles, Shaw and Morgan, 2009), and the potential this can have to override earlier decision making and identity processes, especially when a partner is involved. Table 2.1 Ontologies and research questions Type Definition What the research question Aims/research questions from an captures example study Realist/essential Reality is ‘out there’ and An understanding that Moller and Vossler’s (2015) research ist discoverable through the language captures participants’ question of ‘how infidelity is defined by research process; people’s experiences of reality. practitioners, and how they experience words provide direct access to the ways their clients understand and reality. define infidelity’ fits with their (‘tempered’, p. 489) realist approach to analysing their interview data. Critical Reality is ‘out there’ but access An understanding of Adams, McCreanor, and Braun (2013) realist/contextu to it is always mediated by participants’ experiences as used a critical realist perspective to alist socio-cultural meanings, and, lived realities that are explore gay men’s ‘explanations of health in the case of qualitative produced, and exist, within and well-being’ including ‘their accounts analysis, the participants’ and broader social contexts. of how to improve it’ within ‘(dominant) the researcher’s interpretative individualistic framings and the resources (so direct access to (alternative) social/community framings reality is never possible). of gay men’s health and well-being People’s words provide access to their particular version of evident in these data’ (pp. 888–889). reality; research produces interpretations of this reality. Relativist/constr There is no external reality An understanding that Frith’s (2015) examination of the ways uctionist discoverable through the language does not simply that ‘women are instructed that the body research process. Instead mirror a world ‘out there’, but needs to be trained to meet the versions of reality are created instead is used to construct requirements of a (multi) orgasmic in and through research. The realities, and taken for granted sexual subject’ (p. 318) and how orgasms researcher cannot look knowledge can – should! – be are constructed, in Cosmopolitan through people’s words to find queried. magazine. evidence of the psychological or social reality that sits behind them. Rather, people’s words become the focus of research, and the researcher interprets how these words produce particular realities within the speaker’s and hearer’s culture. Table 2.2 Project sample size recommendations (adapted from Braun & Clarke, 2013) Interviews Focus groups Qualitative Story completion Media texts surveys tasks Undergraduate or 6–10 2–3 (4–8 20–30 20–40 1–100 Honours project participants in each group) Masters or Professional 6–15 3–6 30–100 40–100 1–200 Doctorate project PhD/larger project (TA 15–20 3–6 50+ 100+ 4–400 data as only a part of the whole project) PhD/larger project (TA 30+ 10+ 200+ 400+ 4–400+ data as whole project) Table 2.3 Example of coding of P17 (‘Millers’) Data Code I could do a different job but I wouldn’t Having children limits capacity be able to do the job that I do and enjoy Invested in being competent at job and have a child. There just isn’t enough Reluctance to engage in in me to do that, or to do either well. I superwoman/supermum position think you know I would be spreading myself really thin in that respect, and also Quality of life would be impacted that would mean giving up my salary and I Loss of lifestyle more than gain from earn a decent wage and I enjoy the having a child trappings that that allows me. You know if we want to go on holiday we can go on holiday, we can afford a nice car, we can Acceptance of ‘selfless parent’ discourse afford a nice flat. I wouldn’t want to trade Questioning selfish/selfless binary any of those in for having a child and I Resists pronatalist argument think (pause) people automatically become (pause) more selfless when they Putting partner before self and relationship have a child and something that I’ve highly valued always taken great issue is people saying how not having children is selfish. I’ve always thought that’s a really odd one and (laughs) that selfish implies that somebody is missing out on something, Still engaging in features of feminine role surely I’m the only one that’s missing out Having children negatively affects intimacy on anything if I’m missing out at all but you know, and that sense that I can put Relationship focus changes from partner focus to children focus myself and my boyfriend first. He is my priority, he’s my priority over myself and I really cherish that relationship, and from Good partnerships means quality, the outside looking into it, it would feel like there’s no part of that that I would uninterrupted time want changed and I’ve seen couples who Primacy of the dyadic relationship have children and what their relationship like was before and what it’s like after, and in some ways they’re closer, but in other ways, you know, they don’t have time for each other, they don’t have patience for each other, they don’t have that kind of quiet intimacy. You know of just sitting reading the papers in silence for three hours Transcription notation: underline: participant emphasis; (pause): pause in speech; (laughs): laughter from speaker Table 2.4 Four candidate themes from the child-freedom study, with example Theme 1: A Theme 2: A Theme 3: Childfree Theme 4: ‘Not a ‘precarious ‘perfectly good life’ position as maternal bone identity’ marginalised in my body’ codes Precarious Children as self- Organising holidays Hard to identity sacrifice is more expensive fathom why position ‘Perfectly good life’ (package holidays anyone would as free of children are for families or want children Keeping Children ruin your the old) ‘Lacks’ the options open life Has to tolerate ingredients to (not getting Life cannot remain children’s spaces for do such a sterilised) the same sake of relationships difficult task Can’t make Inevitable with friends Not an decision for upending change Childfree people identity, a older self Anger over ‘unfair’ expected to adapt to state Percentages responsibility – others’ children Sisters have for/against looking after even though chosen ‘something’ having children siblings when a not to have children she does not Not always child ‘Oh you’ll change External sure Unrestricted your mind’ ‘reminders’ to Risk to freedom/time/care Trouble getting have kids (i.e. relationship er options sterilised friends means Unmitigated Explicit denial of getting rethinking freedom to be self stigma pregnant) – childfree status Time consuming Booked holidays not internal Twinges of hobbies open to ‘usurped’ by No biological regret, but childfree workmate with urge never enough Children are children to want expensive by children Not fitting in with default Wants friends whose grandchildren Choosing jobs identity is now easier as less ties without the focused on hard work of Children negatively parenting parenting affect intimacy Being asked to take Balancing Loss of lifestyle holidays at a freedom of not more than gain having from having a child different time to children/family accommodate those with isolation with children of not having family/children Table 2.5 15-point checklist for a good TA (Braun & Clarke, 2006, p. 96) Process No. Criteria Transcription 1 The data have been transcribed to an appropriate level of detail, and the transcripts have been checked against the tapes for ‘accuracy’ Coding 2 Each data item has been given equal attention in the coding process 3 Themes have not been generated from a few vivid examples (an anecdotal approach), but instead the coding process has been thorough, inclusive and comprehensive 4 All relevant extracts for all each theme have been collated 5 Themes have been checked against each other and back to the original dataset 6 Themes are internally coherent, consistent, and distinctive Analysis 7 Data have been analysed – interpreted, made sense of - rather than just paraphrased or described 8 Analysis and data match each other – the extracts illustrate the analytic claims 9 Analysis tells a convincing and well-organised story about the data and topic 10 A good balance between analytic narrative and illustrative extracts is provided Overall 11 Enough time has been allocated to complete all phases of the analysis adequately, without rushing a phase or giving it a once-over-lightly Written 12 The assumptions about, and specific approach to, thematic analysis report are clearly explicated 13 There is a good fit between what you claim you do, and what you show you have done – i.e. described method and reported analysis are consistent 14 The language and concepts used in the report are consistent with the epistemological position of the analysis 15 The researcher is positioned as active in the research process; themes do not just ‘emerge’ Figure 2.1 An early thematic map Figure 2.2 A final thematic map 56