Temporal systems.docx
Document Details
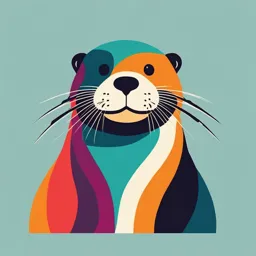
Uploaded by SeasonedGenius
Full Transcript
Temporal systems Systems Biology is like putting together puzzle pieces to understand how different parts of living things work together; instead of just studying individual pieces like genes or proteins, it looks at the big picture of how everything interacts using experiments, computer models, and...
Temporal systems Systems Biology is like putting together puzzle pieces to understand how different parts of living things work together; instead of just studying individual pieces like genes or proteins, it looks at the big picture of how everything interacts using experiments, computer models, and math. This helps us see the dynamic and interconnected nature of life, leading to insights that can help in medicine, technology, and overall understanding of how living systems function. The law of mass action is a crucial concept in deriving kinetic equations for chemical reactions, establishing a link between the macroscopic rate of a reaction and the microscopic events at the molecular level. This law states that the rate of a reaction is directly proportional to the product of the concentrations of the reactants, raised to the power of their respective stoichiometric coefficients. The derivation of kinetic equations involves using this law to formulate a differential rate equation, quantifying how reactant and product concentrations change over time. This process, integrating principles from chemical kinetics and reaction mechanisms, provides a quantitative framework to understand chemical reaction dynamics, predict rates under different conditions, and gain insights into molecular interactions within a system. The Michaelis-Menten equation, a crucial model in enzyme studies, is derived with a few assumptions. It assumes that the formation of the enzyme-substrate complex is the slowest part of the reaction. It also supposes that the concentration of this complex stays constant, forming a quasi-steady state where the creation equals the breakdown rate. The equation further assumes that the initial substrate concentration is much higher than the complex concentration during the reaction, justifying the use of initial velocity as a measure of enzyme activity. Lastly, it assumes the complex quickly reaches equilibrium compared to product formation. In cellular processes, feedback loops and feedforward loops are distinct regulatory mechanisms. Feedback loops involve the self-regulation of a pathway, with negative feedback maintaining stability by countering deviations and positive feedback potentially leading to rapid, irreversible transitions. On the other hand, feedforward loops regulate a pathway based on external signals, anticipating the system's needs. Coherent feedforward loops reinforce effects, while incoherent ones counteract them. Feedback loops contribute to stability and homeostasis, while feedforward loops enable rapid responses to changing conditions, acting as predictive mechanisms. The interplay between these regulatory mechanisms showcases the complexity and adaptability of biochemical networks in living organisms. In biochemical networks, coherent and incoherent three-node feedforward loops are distinct organizational structures. In coherent loops, an external signal influences both the target node and an intermediate node, aligning their effects in the same direction for a stronger and more sustained regulatory response. This enhances overall strength and robustness. In contrast, incoherent loops involve the external signal and the intermediate node acting in opposite directions on the target, leading to a more transient and precise regulatory response, often associated with pulse-like reactions. Understanding these differences provides insights into the dynamic behavior and functional consequences of regulatory motifs in complex biochemical networks. Extending concepts like feedback and feedforward loops to complex biological networks reveals intricate interactions. In complex networks, feedback loops can create nonlinear dynamics, leading to phenomena like sustained oscillations or biostability. Hierarchical feedback structures coordinate regulation at different levels. Similarly, feedforward loops in complex networks take diverse forms, allowing cells to process multiple signals simultaneously. Crosstalk between pathways adds complexity, enabling cells to adapt to changing environments. Studying these structures requires a systems biology approach, integrating experimental data, computational modeling, and advanced techniques. Understanding the hierarchical organization, crosstalk, and nonlinear dynamics in complex networks is crucial for deciphering regulatory strategies. Advances in computational methods and experimental technologies aid in unraveling biological complexities with applications in medicine, biotechnology, and synthetic biology.