Super Thinking: Understanding Inertia and Momentum PDF
Document Details
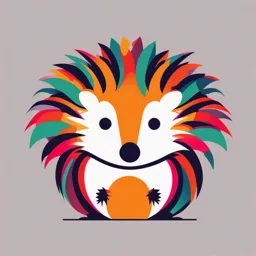
Uploaded by scrollinondubs
Stanford School of Medicine
Tags
Summary
This document discusses the concept of inertia, resistance to change in beliefs, organizations, and markets. It explains the Lindy effect, where the longer something has existed, the longer it will likely continue. The author also touches upon the concept of momentum, the rate at which something changes, in relation to inertia. Finally, the document examines how to leverage momentum and avoid resistance to change.
Full Transcript
At some point you have likely heard someone paraphrase Isaac Newton’s rst law of motion, often referred to as the law of inertia: “An object at rest stays at rest and an object in motion stays in motion with the same speed and in the same direction unless acted upon by an unbalanced force.” Ine...
At some point you have likely heard someone paraphrase Isaac Newton’s rst law of motion, often referred to as the law of inertia: “An object at rest stays at rest and an object in motion stays in motion with the same speed and in the same direction unless acted upon by an unbalanced force.” Inertia is a physical object’s resistance to changing its current state of motion. The meme above illustrates this concept in practice. As a metaphor, inertia can describe any resistance to a change in direction. In Chapter 1, we explained how you tend to have signi cant inertia in your beliefs because of con rmation bias and related models. This adherence to your beliefs can hamper your adaptability. By questioning your assumptions, you can adapt to new ways of thinking and overcome this personal inertia. Inertia can increase the longer you hold on to your beliefs. If you’re like most people, many of your core political, religious, and social beliefs can be traced to the family and geographic culture in which you were raised as a child. Have you reevaluated these views recently? If not, you are likely clinging to many beliefs that are in con ict with other beliefs you came to hold later, or that you never properly questioned. The more inertia you have, the more resistant you will be to changing these beliefs, and the less likely you will be to adapt your thinking when you need to. Think about how scienti c theories change over time, but how old “facts” still persist. When our parents were in school, they weren’t taught about how an asteroid led to the extinction of the dinosaurs, because that theory wasn’t put forth until 1980. And now, forty years later, this widely accepted asteroid theory has come under increased scrutiny in terms of how large a role it actually played in causing the mass extinction. Something di erent may very likely be in textbooks decades from now. Have you heard that the latest research indicates that Tyrannosaurus rex had a form of feathers over parts of its body? Or that the war on saturated fat and dietary cholesterol that loomed large when we were kids in the 1980s has been completely revised, and now whole milk and eggs are thought to be part of a healthy diet? It can be hard to change old habits and beliefs once they are so ingrained, even if you now know them to be awed. We are of course aware of both of these revisions, but still the image that comes to mind when we hear about T. rex is not that of a feathered dinosaur, and we still take pause at the idea of eating eggs every day. Organizations face a similar danger because of inertia. A long-term commitment to an organizational strategy creates a lot of inertia toward that strategy. This inertia can lead to suboptimal decisions, referred to as a strategy tax. For example, most people would like to reduce their online footprint and be tracked less by advertisers. As a result, web browsers have incorporated more privacy features. For example, in 2017, Apple’s Safari browser introduced a feature called Intelligent Tracking Prevention, which attempts to prevent ads from following you around the internet. However, we expect that Google will not add a feature like this to its Chrome browser, because Google itself is the company tracking you on most sites, since its long-term strategy is to dominate online advertising. Since Google tracks you, it can sell advertisers the ability to follow you around the internet with its ads. Google’s strategy of being the world’s biggest advertising company requires it to pay the tax of not adding signi cant anti-tracking features to its browser, since doing so would counteract that strategy. Apple does not have to pay this tax since it does not have such a strategy. Politicians and political parties create strategy tax when locking themselves into a long-term position. For example, the U.S. Republican Party has staked out a position that opposes climate change mitigation, with many politicians denying that man-made climate change is even taking place. As the negative e ects of man-made climate change are becoming clearer through increased catastrophic weather incidents, this strategy tax may start to cost politically. Reversing course once a strategy tax is established can be even more costly. In 1988, George H. W. Bush delivered this famous line at the Republican Party’s national convention: “Read my lips: no new taxes.” Later, this commitment caused signi cant problems for him when he faced a recession as president. Ultimately, Bush decided he had to break his pledge and raise taxes, and it cost him reelection. The lesson here is that you should, as much as possible, avoid locking yourself into rigid long-term strategies, as circumstances can rapidly change. What strategy taxes are you currently paying? A model related to the strategy tax is the Shirky principle, named after economics writer Clay Shirky. The Shirky principle states, Institutions will try to preserve the problem to which they are the solution. An illustrative example is TurboTax, a U.S. company that makes ling taxes easier, but also lobbies against ideas that would make it easier to le taxes directly with the government. For example, “return-free ling,” a system in which the government would send you a pre- lled form based on information it already has available, would work well for most people. It is already a reality in some countries, saving time and money for millions. Yet TurboTax ghts against the adoption of such a program because it wants tax ling to continue to be complex, since it is the solution to that problem. Sometimes a person or department will try to preserve an ine cient process, even when a new idea or technology comes around that can make things easier. Think of the stodgy person at your o ce or school who is always talking about the “way it’s always been done,” constantly anxious about change and new technology. That person embodies the Shirky principle. You do not want to be that person. Inertia in beliefs and behaviors allows entrenched ideas and organizations to persist for long periods of time. The Lindy e ect is the name of this phenomenon. It was popularized by Nassim Taleb in his book Antifragile, which we mentioned in Chapter 1. Taleb explains: If a book has been in print for forty years, I can expect it to be in print for another forty years. But, and that is the main di erence, if it survives another decade, then it will be expected to be in print another fty years. This, simply, as a rule, tells you why things that have been around for a long time are not “aging” like persons, but “aging” in reverse. Every year that passes without extinction doubles the additional life expectancy. This is an indicator of some robustness. The robustness of an item is proportional to its life! The Lindy e ect applies to technologies, ideas, organizations, and other nonperishable things. Assuming that the thing in question is not falling out of favor, the longer it endures, the longer you can expect it to further endure. The Lindy e ect explains the continued relevance of Shakespeare and the Beatles. Since they show no signs of falling out of favor, the Lindy e ect tells us we can expect Shakespeare plays to be performed for at least another four hundred years, and Beatles songs to be heard for at least another fty. Of course, things can and do eventually become unpopular, and there is another mental model to describe the point at which something’s relevance begins to decline. This model is peak, as in peak sexism, peak Facebook. This concept was actually popularized with oil, as peak oil is usually de ned as the point in time when the maximum amount of oil is being extracted from Earth. After peak oil, the decline may be a slow one, but it will have begun, with oil production falling each year instead of rising. People have predicted peak oil many times. As far back as 1919, David White, the chief geologist of the U.S. Geological Survey, predicted in “The Unmined Supply of Petroleum in the United States” that the U.S. “peak of production will soon be passed, possibly within three years.” Many similar predictions have come and gone, and peak oil has still not occurred. What has happened instead is that increased demand has driven innovation in how to get more oil out of the ground, continually increasing yearly production. Now, though, a better argument for peak oil is starting to form as the oil market’s underlying structure is proving to be unhealthy. The e ects of climate change are looming. Solar energy is quickly becoming cost- competitive with oil on a global scale. Increasing cost-competitiveness of electric cars and the advent of autonomous vehicles and ride-sharing services are threatening to collapse the car and truck markets as we know them. All of these have the potential to create lasting e ects on the oil market. Whether you are a market observer or a market participant, these structural changes are worth considering when you’re thinking about a possible new reality for the oil market. Should your next car be electric? Should you buy another car at all? More generally, the Lindy e ect and peak concepts can help you assess any idea or market opportunity and better predict how it might unfold. Is the market healthy? Has it already reached its peak? How long has it been around? Remember, markets that have been around a long time have more inertia. And the healthier the market is, the more di cult it will be to change. In fact, something with a lot of inertia, even after its peak, can take an extremely long time to decline. Over the past decade, consumers have read fewer physical newspapers and have been “cutting the cord” with cable, yet plenty of newspapers and cable subscriptions are still sold and will continue to be sold for decades to come. Similarly, fax machines, video rental stores, and dial-up internet feel like relics of the nineties, but people still send plenty of faxes, and, as of the time of this writing, in late 2018, there still exists a Blockbuster video store in Bend, Oregon, and more than a million people still use AOL dial-up! As Samuel Clemens (aka Mark Twain) said, “The report of my death was an exaggeration.” U.S. Newspaper Circulation Momentum is a model that can help you understand how things change. Momentum and inertia are related concepts. In physics, momentum is the product (multiplication) of mass and velocity, whereas inertia is just a function of mass. That means a heavy object at rest has a lot of inertia since it is hard to move, but it has no momentum since its velocity is zero. However, a heavy object gets momentum quickly once it starts moving. The faster an object goes, the more momentum it has. However, its inertia remains the same (since its mass remains the same), and it is still similarly di cult to change its velocity. To relate the concept to a real-world example, sending faxes is continually losing momentum. However, the act still has a lot of inertia since the technology is entrenched in many business processes. As a result, the momentum behind sending faxes decreases very slowly. In your life, you can take advantage of this concept by seeking out things that are rapidly gaining momentum. For example, you could join an organization that is just starting to take o or start a new organization that leverages an innovative technology or idea that is beginning to go mainstream. In Chapter 3, we discussed the bene ts of focusing on high- leverage activities to make the most out of your time. Activities associated with organizations or ideas with high and increasing momentum are very often high-leverage, because your e orts get ampli ed by the momentum. Similarly, creating inertia by entrenching beliefs and processes in others is also a high-leverage activity. Once they are established, those beliefs or processes will be di cult to unwind, persisting for a long time. Think about those beliefs from childhood that we spoke about earlier and how hard they are to let go. In an organizational context, establishing such beliefs and norms is the act of creating culture, which we will explore more fully in Chapter 8. Here, though, consider the relation of culture to inertia embodied in this saying: Culture eats strategy for breakfast. It is a warning that if you embark on a strategy that is in opposition to your organization’s culture, which has much more inertia than even its strategy, it is very unlikely to succeed. For example, in 2013 the U.S. government embarked on a strategy to create a website where citizens could directly apply for healthcare coverage. The website, HealthCare.gov, was to be made available on October 1, 2013, though unlike major technology rms, the U.S. government does not have a culture attuned to creating mainstream websites on a deadline. This culture/strategy mismatch was obvious when the government botched the launch of HealthCare.gov. In the rst week of operations, only a small percentage of those interested were able to enroll. A strike team was assembled to x the website, lled with top talent from major tech rms, a group steeped in a culture better matched to this strategy. Quite simply, to be successful you need your organization’s culture to align with its strategy. As an organizational leader, you must recognize if there is a mismatch and act accordingly. As the U.S. government eventually did, you could create a new team with a di erent culture more t for the strategy. You could abandon the strategy or pursue a modi ed strategy more aligned with the existing culture. Or you could try to change the culture over time, steering it toward the desired long-term strategy, recognizing that it may be a slow and challenging process. In new organizations, you have the opportunity to mold the culture toward long-term strategic directions. However, consider that the world can rapidly change around you. It follows, then, that you eventually may have to rapidly change your organization’s strategy as well. Consequently, the best organizational culture in many situations is one that is highly adaptable, just as it is recommended for people themselves to be highly adaptable. That is, you likely want to craft an organizational culture that can readily accept new strategies or processes. A culture like this is agile, willing to experiment with new ideas, not tied down to existing processes. The good news is that if you can establish inertia and momentum in an adaptable culture, or in any context really, it can have staying power. A mental model that captures this process well is the ywheel, a rotating physical disk that is used to store energy. Flywheels are still used in many industrial applications, though a more relatable example to get the concept is a children’s merry-go-round. It takes a lot of e ort to get a merry-go- round to start spinning, but once it is spinning, it takes little e ort to keep it spinning. Flywheel Nonpro t marketing expert Tom Peterson credits the ywheel model for the growth of the global anti-poverty nonpro t Heifer International from $3 million in revenue in 1992 to $90 million in 2008. In the 1970s, Heifer International created its gift catalog fundraising concept, encouraging people to give a gift like a goat or water bu alo to a family in need in order to make them more self-reliant. With Peterson’s help, Heifer improved the catalog each year, running dozens of experiments through changes to its look, contents, production, distribution, and publicity. This constant testing and experimentation helped the company’s revenue grow a little bit each year, never slowing down. It has continued to sustain higher and higher levels through today. In his book Good to Great, Jim Collins relates many similar examples, using the ywheel metaphor to summarize how companies systematically and incrementally go from good to great. The ywheel image captures the overall feel of what it was like inside the companies as they went from good to great. No matter how dramatic the end result, the good-to-great transformations never happened in one fell swoop. There was no single de ning action, no grand program, no one killer innovation, no solitary lucky break, no wrenching revolution. Good to great comes about by a cumulative process—step by step, action by action, decision, turn by turn of the ywheel—that adds up to sustained and spectacular results. An example of a ywheel in everyday life is how it takes a lot of time and practice to become an expert on a topic, but once you are an expert it takes only minimal e ort to remain on top of new developments in the eld. On a shorter time scale, any personal or professional project can be viewed from the perspective of a ywheel. It is slow when you get started on the project, but once you gain some momentum, it seems easier to make progress. And as we discussed in Chapter 3, when we multitask, we never get enough momentum on any one task for it to start to feel easier. Instead, we are constantly spending energy starting and restarting the wheel rather than taking advantage of its momentum once we get it to start spinning. The ywheel model tells you your e orts will have long-term bene ts and will compound on top of previous e orts by yourself and others. It’s the tactical way to apply the concepts of momentum and inertia to your advantage. On the other hand, trying to change something that has a lot of inertia is challenging because of the outsized e ort required. That doesn’t mean it isn’t worth the e ort, but you should go in with eyes wide open, knowing that it can be di cult and time-consuming. If you do decide to try enacting such a change, there are several useful models to aid you in your quest. First, from biology, there is homeostasis, which describes a situation in which an organism constantly regulates itself around a speci c target, such as body temperature. When you get too cold, you shiver to warm up; when it’s too hot, you sweat to cool o. In both cases, your body is trying to revert to its normal temperature. That’s helpful, but the same e ect also prevents change from the status quo when you want it to occur. In general, societies, organizations, families, and individuals usually exhibit homeostasis around a set of core cultural values or metrics that relate to the status of the group. Doing so ensures their self-preservation (or so they think). For example, in the U.S., attempts at campaign nance reform have continually failed as lobbying groups have found new and innovative ways to react to regulations, and they continue to infuse their money into politics. On a more mundane scale, within organizations or communities, people often naturally resist change, regularly responding with the mantra “If it ain’t broke, don’t x it,” “Don’t rock the boat,” or “That’s how we do things around here.” This reaction can be rationalized and justi ed because change can be disruptive, even if the proposed end state has attractive bene ts. In our kids’ school district right now, there is a debate about changing the school start times based on solid research showing that teenagers do better with later start times. However, moving school times causes a decent amount of disruption to various pockets of the community, and would require many families and teachers to adjust their schedules and arrangements, such as for childcare. If the goal is to achieve the best educational outcome for the students, the data shows that start times should be moved, though the reaction to protect the status quo is nevertheless understandable. Unfortunately, as a result of these types of homeostatic reactions, we stay in suboptimal arrangements for too long, from toxic personal relationships to poor organizational processes, all the way up to ine ective government policies. When you ght homeostasis—in yourself or in others —look out for the underlying mechanisms that are working against your e orts to make changes. A good example would be exercising more to lose weight only to have your increased exercise lead to an increased appetite. Anticipating this reaction, some people eat protein after working out to mitigate the homeostatic e ect, because certain types of slow-digesting proteins help you feel full for longer. What is the “eating protein” equivalent in whatever situation you’re dealing with? Finding the answer can help you overcome the status quo. One common approach is to get data that support the desired change, and then use that data to counteract objections to it. In the school start-times example, some people claim that making the start time later will just cause teenagers to stay up later, negating the e ect. But studies from actual school districts that have already made the change show that is not the case, and that teenagers do in fact sleep more on average with later school times. This concept of trying hard not to deviate from the status quo reminds us of a toy that is generically called a roly-poly toy (in the U.S., Playskool had a branded version called a Weeble—“Weebles wobble, but they don’t fall down”), which rights itself when pushed over. These toys work using two useful concepts that are also metaphorical mental models that help you when enacting change: potential energy and center of gravity. Potential energy is the stored energy of an object, which has the potential to be released. Center of gravity is the center point in an object or system around which its mass is balanced. Potential Energy Any time a roly-poly is tilted, its potential energy increases, as it takes in the energy used to tilt the toy. When released, this energy gets translated into a wobble around its center of gravity. Potential energy like this comes in many physical forms: gravitational, such as any object lifted up; elastic, such as a taut bowstring or spring; chemical, such as the energy locked up in food or fuel; etc. Metaphorically, we talk about people and organizations having pent-up energy, energy waiting to be unlocked, released from its stored state, and unleashed on the world. Hidden potential energy is another thing you can look for when seeking change. Think of people in your organization who are motivated to make the change happen. They may be willing to help you. Talking to a diverse set of potential stakeholders can help you discover these hidden pockets of potential energy. The term center of gravity is used notably in military strategy to describe the heart of an operation. Knowing an opponent’s center of gravity tells you where to attack to in ict the most damage or what pieces of their infrastructure they will defend more than others. The closer to their center of gravity, the more damage you will cause, and the more they will risk defending it. As applied tactically to enact change, if you can identify the center of gravity of an idea, market, or process—anything—then you might e ect change faster by acting on that speci c point. For example, you might convince a central in uencer, someone other people or organizations look to for direction, that an idea is worthwhile. Businesses often take advantage of this concept by seeking endorsements from celebrities, in uencers, press, or marquee clients. One endorsement can have a cascading e ect, as your idea is able to spread because you convinced the right person. In this context, it’s a type of pressure point: press it, and you can move the whole system. So far in this section we’ve discussed the power of inertia (strategy tax, Shirky principle), how to assess it (peak, Lindy e ect), how to take advantage of it ( ywheel), and how to think about reversing it through tactical models (homeostasis, potential energy, center of gravity). A couple other chemistry concepts will also be helpful to you tactically: activation energy and catalyst. Activation energy is the minimum amount of energy needed to activate a chemical reaction between two or more reactants. Consider striking a match to ignite it: the friction from striking the match supplies the activation energy needed for it to ignite. A catalyst decreases the activation energy needed to start a chemical reaction. Think of how it is easier for a wild re to start on a hot and dry day, with increased temperature and decreased moisture serving as catalysts. More generally, activation energy can refer to the amount of e ort it would take to start to change something, and catalyst to anything that would decrease this e ort. When you are settled into the corner of the couch, it requires a lot of activation energy to get up. However, knowing there is ice cream in the freezer is a catalyst that lowers this activation energy. When attempting change, you want to understand the activation energy required and look for catalysts to make change easier. In 2017, the U.S. saw both a rapid takedown of statues commemorating Confederate leaders and the accelerating takedown of sexual predators via the #MeToo movement. In both cases it seems that once there was enough activation energy, the movements pushed forward very quickly. It turns out there was a lot of potential energy waiting to be unleashed once those rst steps were taken. Furthermore, social media posts and reporting by journalists were catalysts, serving as both a blueprint and an outlet for others to publicize these causes. In Chapter 3 we described how commitment can help you overcome present bias; it can also serve as a great catalyst, or forcing function, to reach the activation energy required for a personal or organizational change. It usually takes the form of a prescheduled event, or function, that facilitates, or forces, you to take a desired action. A common example of a forcing function is the standing meeting, such as one-on-one meetings with a manager or coach, or a regular team meeting. These are set times, built into the calendar, when you can repeatedly bring up topics that can lead to change. You can similarly build additional forcing functions directly into your personal or company culture. For instance, you can set the expectation of producing weekly project updates, which serve as catalysts to think critically about project status and communicate progress to stakeholders. A more personal forcing function would be a regular appointment with a trainer at a gym, or a weekly family meeting or budget review. These set blocks of time will grease the wheels for change. The title of this section is Don’t Fight Nature. You should be wary of ghting high-inertia systems blindly. Instead, you want to look at things more deeply, understand their underlying dynamics, and try to craft a high- leverage path to change that is more likely to succeed in a timely manner. HARNESSING A CHAIN REACTION Now we will discuss what often creates the underlying momentum behind new ideas as they permeate society: critical mass. As we noted in the Introduction, in physics critical mass is the mass of nuclear material needed to create a nuclear chain reaction, where the by-products of one reaction are used as the inputs for the next, chaining them together in a self- perpetuating fashion. Nuclear Chain Reaction This piece of knowledge was essential for the creation of the atomic bomb. Below the critical mass, nuclear elements are relatively harmless; above, and you have enough material to drive an atomic explosion. In 1944 in Los Alamos, New Mexico, Austrian-British physicist Otto Frisch was tasked with determining how much enriched uranium was required to create the critical mass for the rst atomic bomb. Believe it or not, Frisch gured out the critical mass in part by physically stacking three- centimeter uranium bars, continually measuring their radioactive output as the stack grew larger. One day he almost caused a runaway reaction, the rst known criticality accident, by simply leaning over the stack with his body. Some of the radiation re ected o his body and back into the stack, already near the critical mass, causing the radiation-detecting red lamps in the vicinity to shine continuously instead of ickering intermittently as usual. Noticing the lamps, Frisch scattered some of the bars quickly with his hand, and later wrote in his memoir, What Little I Remember, that if he “had hesitated for another two seconds before removing the material... the dose would have been fatal.” Critical mass as a super model applies to any system in which an accumulation can reach a threshold amount that causes a major change in the system. The point at which the system starts changing dramatically, rapidly gaining momentum, is often referred to as a tipping point. For example, a party needs to reach a critical mass of people before it feels like a party, and the arrival of the nal person needed for the party to reach the critical number tips the party into high gear. Sometimes this point is also referred to as an in ection point, where the growth curve bends, or in ects. However, note that mathematically the in ection point actually refers to a di erent point on the curve, when it changes from concave to convex, or vice versa. Most popular technologies and ideas have had tipping points that propelled them further into the mainstream. If you graph their adoption curves, as in the chart below, you can plainly see these points. Technology Adoption Curves When thinking about engaging with new ideas and technologies, you want to examine where they are along their adoption curves, paying special attention to tipping points. Did a tipping point just happen? Will one ever happen? What could be a catalyst? Being an expert in an area that is about to hit a tipping point is an advantageous position, since your expertise has increasing leverage as the idea or technology takes o. Conversely, specializing in an area that is a decade away from hitting a tipping point is a much lower-leverage situation. The spreading, or di usion, of an idea or technology is known as the technology adoption life cycle. In his 1962 book Di usion of Innovation, sociologist Everett Rogers theorized that people belong to one of ve groups based on how and when they adopt new things: Innovators (about 2.5 percent of the population) have the desire and nancial wherewithal to take risks and are closely connected to the emerging eld, usually because they are speci cally interested in trying new things within it. Early adopters (13.5 percent) are willing to try out new things once they are a bit more eshed out. Early adopters do not require social proof to use a product or idea. They are often the in uencers that help push an idea past a tipping point, thus making it more broadly known. The early majority (34 percent) are willing to adopt new things once the value proposition has been clearly established by the early adopters. This group is not interested in wasting their time or money. The late majority (34 percent) are generally skeptical of new things. They will wait until something has permeated through the majority of people before adopting it. When they get on board, it is often at a lower cost. Laggards (16 percent) are the very last group to adopt something new, and they do so only because they feel it is necessity. Technology Adoption Life Cycle Consider the adoption of the cellphone, which, as you can see from the Technology Adoption gure, progressed in several stages. The initial users— the innovators and early adopters—were rich tinkerers or professionals (e.g., doctors) who were able and willing to pay the high expense because it helped them do their jobs better. Later, as the price came down and new use cases emerged (e.g., text messaging), the early and late majority adopted. And nally, when they felt left behind, the laggards bought cellphones. The smartphone has followed a similar pattern, albeit more quickly. Do you still know people who use ip phones? They are the laggards in the smartphone adoption lifecycle. The curves that emerge from the technology adoption life cycle are known as S curves because they resemble an S shape. The bottom part of the S is the pace of initial slower adoption; then adoption kicks into high gear; and nally, adoption slows as the market saturates, creating the top part of the S. S Curve While developed as a theory about technological innovation, the concept of an adoption life cycle also applies to social innovations, including ideas of tolerance and social equality. In the past decades acceptance of same-sex marriage has swept through the early majority in the U.S., and even into the late majority among Independents and Democrats (see chart on next page). Reaching a critical mass is a common proximate cause (see Chapter 1) of a tipping point. But the root cause of why a tipping point has been reached is often found in network e ects, where the value of a network grows with each addition to it (the e ect). Think of a social network—each person who joins makes the service more enticing because there are then more people to reach. U.S. Same-Sex Marriage Support The concept of a network is wider, however, encompassing any system where things (often referred to as nodes) can interact. For example, you need enough uranium atoms (“nodes”) in the “network” of a nuclear bomb such that when one decays, it can rapidly interact with another, instead of dissipating harmlessly. To use another example from everyday life, the telephone isn’t a useful device if there is no one else to call. But as each person gets a phone, the number of possible connections grows proportionally to the square of the number of phones (nodes). Two phones can make only one connection, ve can make ten, and twelve can make sixty-six. Network E ects 2 phones = 1 connection 5 phones = 10 connections 12 phones = 66 connections This relationship, known as Metcalfe’s law, is named after Robert Metcalfe, the co-inventor of the networking technology Ethernet. It describes the nonlinear growth in network value when nodes are connected to one another. His law oversimpli es reality since it assumes that every node (or telephone in this case) has the same value to the network and that every node may want to contact every other, but nevertheless it serves as a decent model. Having a million telephones on the phone network is much more than twice as valuable as having ve hundred thousand. And knowing that everyone is connected is extremely valuable, which explains why Facebook has such a strong network e ect. Critical mass occurs when there are enough nodes present to make a network useful. Amazingly, the fax machine was invented in the 1840s, but people didn’t regularly use it until the 1970s, when there were enough fax machines to reach critical mass. The modern equivalent is internet messaging services: they need to reach critical mass within a community to be useful. Once they pass this tipping point, they can rapidly make their way into the mainstream. Network e ects have value beyond communication, however. Many modern systems gain network e ects by simply being able to process more data. For example, speech recognition improves when more voices are added. Other systems gain advantages by being able to provide more liquidity or selection based on the volume or breadth of participants. Think of how more goods are available on Etsy and eBay when more people are participating on those sites. Network e ects apply to person-to-person connections within a community as well. Being part of the right alumni network can help you nd the right job or get you answers quickly to esoteric questions. Any time you have nodes in a system participating in some kind of exchange, such as for information or currency, you have the potential for network e ects. Once an idea or technology reaches critical mass, whether through network e ects or otherwise, it has gained a lot of inertia, and often has a lot of momentum as well. In the fax example, after a hundred years of struggling for adoption, once fax technology passed the critical mass point, it became embedded in society for the long term. The lesson here is, when you know that the concept of critical mass applies to your endeavor, you want to pay special attention to it. Just as we suggested questions to ask about tipping points, there are similar questions you can ask about critical mass and network e ects: What is the critical mass point for this idea or technology? What needs to happen for it to reach critical mass? Are there network e ects or other catalysts that can make reaching critical mass happen sooner? Can I reorganize the system so that critical mass can be reached in a sub-community sooner? It’s important to note that these critical mass models apply in both positive and negative scenarios. Harmful ideas and technologies can also reach critical mass and spread quickly through societies. Historical examples abound, from fascism to institutional racism and other forms of discrimination. For negative or positive, modern communication systems have made it much easier for ideas to reach critical mass. In Chapter 1 we explored how people are in echo chambers online, which make it easier for insular points of view to persist. In addition, ad targeting can nd the individuals most susceptible to a message, both by targeting the people who are most inclined to believe it and by experimenting with di erent ad variations until the most manipulative method is discovered. In this manner, conspiracy theories and scams can thrive. When discovering the atomic critical mass, Otto Frisch narrowly avoided a catastrophic chain reaction, known more generally as a cascading failure, where a failure in one piece of a system can trigger a chain reaction of failure that cascades through the entire system. Major blackouts on our electric grid are usually the result of cascading failure: overload in one area triggers overload in adjacent areas, triggering further overload in more adjacent areas, and so on. The 2007/2008 nancial crisis is another example of a cascading failure, where a failure in subprime mortgages ultimately led to failures in major nancial institutions. In biological systems, the decimation of one species can lead to the decimation of others, as their absence cascades through the food chain. This occurs often when one species almost exclusively feeds on another, such as pandas and bamboo or koalas and eucalyptus leaves. Or think about how many species depend on coral reefs for their survival: when the reef disappears, so do most of the organisms that rely on it. It’s not all bad, though; these are natural laws that can be used for good or bad. The nuclear critical mass can be used for relatively safe, essentially unlimited nuclear energy, or the nuclear critical mass could be the delivery mechanism of a catastrophic nuclear winter. In any case, these mental models are playing an increasing role in society as we get more and more connected. As technologies and ideas spread, you will be better prepared for them if you can spot and analyze these models—how S curves unfold, where tipping points occur, how network e ects are utilized. And if you are trying to gain mainstream adoption and long-term inertia for a new idea or technology, you will want to understand how these models directly relate to your strategy. ORDER OUT OF CHAOS Many global systems, including the economy and weather, are known as chaotic systems. That means that while you can guess which way they are trending, it’s impossible to precisely predict their overall long-term state. You can’t know how a particular company or person in the economy will fare over time or exactly when and where an extreme weather event will occur. You can only say that it seems like the unemployment rate is moving down or that hurricane season is coming up. Mathematician Edward Lorenz is famous for studying such chaotic systems, pioneering a branch of mathematics called chaos theory. He introduced a metaphor known as the butter y e ect to explain the concept that chaotic systems are extremely sensitive to small perturbations or changes in initial conditions. He illustrated this concept by saying that the path of a tornado could be a ected by a butter y apping its wings weeks before, sending air particles on a slightly di erent path than they would have otherwise traveled, which then gets ampli ed over time and ultimately results in a di erent path for the tornado. This metaphor has been popularized in many forms of entertainment, including by Je Goldblum’s character in the 1993 movie Jurassic Park and in the 2004 movie The Butter y E ect, starring Ashton Kutcher. THE BUTTERFLY EFFECT The fact that you are surrounded by chaotic systems is a key reason why adaptability is so important to your success. While it is a good idea to plan ahead, you cannot accurately predict the circumstances you will face. No one plans to lose their spouse at a young age, or to graduate from college during an economic downturn. You must continuously adapt to what life throws at you. Unlike an air particle, though, you have free will and can actively navigate the world. This means you have the potential to increase the probability of a successful outcome for yourself. You can at least attempt to turn lemons into lemonade by using these chaotic systems to your advantage. For example, some studies show that businesses started during a recession actually do better over time, and research by the Kau man Foundation, summarized in “The Economic Future Just Happened” in 2009, found that the majority of Fortune 500 companies were started during tough economic times. We’re sure you can point to times in your history when a small change led to a big e ect in your life. It’s the “what if” game. What if you hadn’t gone to that event that led to meeting your spouse? What if you had moved into that other apartment? What if you had struck up a relationship with a di erent teacher or mentor? That’s the butter y e ect at the most personal level. One way to more systematically take advantage of the butter y e ect is using the super model of luck surface area, coined by entrepreneur Jason Roberts. You may recall from geometry that the surface area of an object is how much area the surface of an object covers. In the same way that it is a lot easier to catch a sh if you cast a wide net, your personal luck surface area will increase as you interact with more people in more diverse situations. If you want greater luck surface area, you need to relax your rules for how you engage with the world. For example, you might put yourself in more unfamiliar situations: instead of spending the bulk of your time in your house or o ce, you might socialize more or take a class. As a result, you will make your own luck by meeting more people and nding more opportunities. Thinking of the butter y e ect, you are increasing your chances of in uencing a tornado, such as forming a new partnership that ultimately blossoms into a large, positive outcome. You obviously have to be judicious about which events to attend, or you will constantly be running to di erent places without getting any focused work done. However, saying no to everything also has a negative consequence—it reduces your luck surface area too much. A happy medium has you attending occasional events that expose you to people who can help you advance your goals. Say no often so you can say yes when you might make some new meaningful connections. Your luck surface area relates to the natural concept of entropy, which measures the amount of disorder in a system. In a clean room where there is a rule for where everything goes—socks in the sock drawer, shirts on hangers, etc.—there are not many possible con gurations for everything in the room because of these strict rules. The maximum amount of entropy in this arrangement is small. If you relax those rules, for example by allowing clothes to go on the oor, there are suddenly many more possible con gurations for everything in the room. The amount of possible disorderliness, the maximum entropy level possible for the room, has gone up signi cantly. In this context, increasing your luck surface area means increasing your personal maximum entropy, by increasing the possible number of situations you put yourself in. Your life will be a bit less orderly, but disorder in moderation can be a good thing. Of course, as we have seen so far, too much of a good thing can also be a bad thing. Too much entropy is just chaos. We refer to our kids as entropy machines because they create disorder very quickly. They don’t follow rules for where their belongings go in their rooms, so the maximum possible entropy for their rooms is very high. Almost anything can go almost anywhere and ultimately their rooms can get pretty close to this maximum, resulting in a big mess. As entropy increases, things become more randomly arranged. If left to continue forever, this eventually leads to an evenly distributed system, a completely randomly arranged system—clothes and toys anywhere and everywhere! In a closed system, like our kids’ rooms, entropy doesn’t just decrease on its own. Russian playwright Anton Chekhov put it like this: “Only entropy comes easy.” If our kids don’t make an e ort to clean up, the room just gets messier and messier. The natural increase of entropy over time in a closed system is known as the second law of thermodynamics. Thermodynamics is the study of heat. If you consider our universe as the biggest closed system, this law leads to a plausible end state of our universe as a homogenous gas, evenly distributed everywhere, commonly known as the heat death of the universe. On a more practical level, the second law serves as a reminder that orderliness needs to be maintained, lest it be slowly chipped away by disorder. This natural progression is based on the reality that most orderliness doesn’t happen naturally. Broken eggs don’t spontaneously mend themselves. In boiling water, an ice cube melts and never re-forms as ice. If you take a puzzle apart and shake up the pieces, it isn’t going to miraculously put itself back together again. You must continually put energy back into systems to maintain their desired orderly states. If you never put energy into straightening up your workspace, it will get ever messier. The same is true for relationships. To keep the same level of trust with people, you need to keep building on it. In Chapter 3, we discussed ways to proactively organize your time in order to spend this limited resource wisely, such as by using the Eisenhower Decision Matrix. Seen through the lens of entropy, your time, if left unmanaged, will start to go to random, largely reactionary activities. You will get pulled into the chaotic systems that surround you. Instead, you need to manage your time so that it is in a state of lower entropy. When you are able to make time for important activities, you are more easily able to adapt to your changing environment because you have the ability to allocate time to a particular important activity when needed. To apply the Eisenhower Decision Matrix usefully, though, you need to assess properly what is an important activity and what is not. Given the butter y e ect and the fact that you must interact with chaotic systems like the economy, making these determinations can be challenging. This is especially true when deciding how and when to pursue new ideas, where an unexpected encounter can reveal new and important information. To make these determinations, you must therefore seek to understand and simplify chaotic systems like the economy so that you can successfully navigate them. All the mental models in this book are in service of that general goal. You can also develop your own models, such as by making your own 2 × 2 matrices like the Eisenhower Decision Matrix. Below is one we made up relating speci cally to helping you determine what events you might want to attend. Low-cost events High-cost events High-impact events Attend Maybe attend Low-impact events Maybe attend Ignore You can use this 2 × 2 matrix to help you categorize events as either high or low impact and high or low cost (time, money, etc.). You want to attend high-impact, low-cost events, and ignore low-impact, high-cost events. The other two quadrants are more nuanced. If there is a high- impact, high-cost event, such as a conference far from where you live, it may be worth going to depending on the speci cs of the event and your particular situation: do you have the time and money to go? Similarly, if there is a low-impact event down the hall that will take only an hour of your time, it might be worth attending because the cost is so low. These 2 × 2 matrices draw on a concept from physics called polarity, which describes a feature that has only two possible values. A magnet has a north and south pole. An electric charge can be positive or negative. Polarity is useful because it helps you categorize things into one of two states: good or bad, useful or not useful, high-leverage or low-leverage, etc. When you mix two groupings together, you get the 2 × 2 matrix. These visualizations are powerful because you can distill complicated ideas into a simple diagram and gain insights in the process. While 2 × 2 matrices can be illuminating, they can also be misleading because most things don’t fall squarely into binary or even discrete states. Instead they fall along a continuum. For example, if you’re considering ways to make extra money across a set of possible activities, you don’t just want to know if you will make any money with each; you want to know how much, and how di cult it will be to generate new income from each activity. Winning the lottery will be signi cantly di erent from nding money on the ground or getting a part-time job. One simple way visually to introduce this type of complexity is through a scatter plot on top of a 2 × 2 matrix, which visualizes the relative values of what you are analyzing. While polarity can be useful, when making comparisons you must be careful to avoid the black-and-white fallacy—thinking that things fall neatly into two groups when they do not. When making decisions, you usually have more than two options. It’s not all black and white. Practically, whenever you are presented with a decision with two options, try to think of more. People are susceptible to the black-and-white fallacy because of the natural tendency to create us versus them framings, thinking that the only two options are ones that either bene t themselves at the expense of “others,” or vice versa. This tendency arises because you often associate identity and self-esteem with group membership, thereafter creating in- group favoritism and, conversely, out-group bias. Social psychologists Henri Tajfel and John Turner established research in this area, published as “The Social Identity Theory of Intergroup Behavior” in Political Psychology in 2013, that has since been corroborated many times. It showed that with the tiniest of associations, even completely arbitrary ones (like de ning groups based on coin tosses), people will favor their “group.” Outside the lab, this tendency toward in-group favoritism often fosters false beliefs that transactions are zero-sum, meaning that if your group gains, then the other group must lose, so that the sum of gains and losses is zero. However, most situations, including most negotiations, are not zero- sum. Instead, most have the potential to be win-win situations, where both parties can actually end up better o , or win. How is this possible? It’s because most negotiations don’t include just one term, such as price, but instead involve many terms, such as quality, respect, timing, control, risk, and on and on. In other words, there are usually several dimensions underlying a negotiation, and each party will value these dimensions di erently. This opens up the possibility for a give-and-take where you give things you value less and take things you value more. As a result, both parties can end up better than they were before, getting things they wanted more and giving things they wanted less. In fact, this give-and-take is the basis for most economic transactions! Otherwise, without misinformation, misunderstanding, or duress, people wouldn’t make all these transactions. Zero-sum is the exception, not the rule. Black-and-white and zero-sum thinking simply do not provide enough possible options, just having two. Recognizing that there are more options and dimensions can be desirable in many situations, such as business deals. The more terms of the deal you consider, the more possible arrangements of deal terms there are. If managed properly, this increases the likelihood of a successful deal for both sides, of nding that win-win state. You can go too far, though. For example, in a complex business negotiation, you cannot discuss every word in the contract or else discussions will take forever, and you’ll never get a deal done. You must instead choose thoughtfully which words are worth discussing and which are not. More generally, you must continually try to strike the right balance between order and chaos as you interact with your environment. If you let the chaos subsume you, then you will not make progress in any particular direction. But if you are too ordered, then you will not be able to adapt to changing circumstances and will not have enough luck surface area to improve your chances of success. You want to be somewhere in the middle of order and chaos, where you are intentionally raising your personal entropy enough to expose yourself to interesting opportunities and you are exible and resilient enough to react to new conditions and paradigms that emerge. If you study the biographies of successful people, you will notice a pattern: luck plays a signi cant role in success. However, if you look deeper, you will notice that most also had a broad luck surface area. Yes, they were in the right place at the right time, but they made the e ort to be in a right place. If it wasn’t that particular place and time, there might have been another. Maybe it wouldn’t have resulted in the same degree of success, but they probably would have still been successful. Another pattern: many of the most in uential gures (Bill Gates, Martin Luther King Jr., etc.) were at the center of major adoptions of ideas or technologies that swept through society via the critical mass models described earlier. In some cases, they created the new idea or technology, but more often they were the ones who brought the ideas or technologies into the mainstream. They created momentum and ultimately inertia by guiding the ideas and technologies through the technology adoption life cycle. With deeper understanding of these models, you should be able to more easily adapt to the major changes that will come in your lifetime. You should also be able to spot them coming from afar and participate in them, as if you were catching a wave and having it glide you safely to shore. Being adaptable like this helps you in good times and bad. On the positive side, you can make better decisions with your life and career; on the negative side, you can be more resilient when setbacks and unfortunate events occur, and even help limit their negative e ects. KEY TAKEAWAYS Adopt an experimental mindset, looking for opportunities to run experiments and apply the scienti c method wherever possible. Respect inertia: create or join healthy ywheels; avoid strategy taxes and trying to enact change in high-inertia situations unless you have a tactical advantage such as discovery of a catalyst and a lot of potential energy. When enacting change, think deeply about how to reach critical mass and how you will navigate the technology adoption life cycle. Use forcing functions to grease the wheels for change. Actively cultivate your luck surface area and put in work needed to not be subsumed by entropy. When faced with what appears to be a zero-sum or black-and- white situation, look for additional options and ultimately for a win-win. 5 Lies, Damned Lies, and Statistics DATA, NUMBERS, AND STATISTICS now have an everyday role in most professional careers, not just in engineering and science. Increasingly, organizations of all kinds are making data-driven decisions. Every eld has people studying ways to do it better. Consider K–12 education: What is the most e ective way to teach kids to read? How much homework should students be getting? What time of day should school start? The same is increasingly true in everyday life: What is the best diet? How much exercise is good enough? How safe is this car compared with that one? Unfortunately, there often aren’t straightforward answers to these types of questions. Instead, there are usually con icting messages on almost every topic: for nutrition, medicine, government policy (environmental regulation, healthcare, etc.), and the list goes on and on. For any issue, you can nd people on both sides with “numbers” to back up their position. This leads many people to feel that data can be too easily manipulated to support whatever story someone wants to tell, hence the title of this chapter. Similarly, even if people aren’t intentionally trying to mislead you, study results are often accidentally misinterpreted, or the studies themselves can su er from design aws. However, the answer is not to dismiss all statistics or data-driven evidence as nonsense, leaving you to base decisions solely on opinions and guesses. Instead, you must use mental models to a get a deeper understanding of an issue, including its underlying research, enabling you to determine what information is credible. You can also use data from your life and business to derive new insights. Insights based on true patterns, such as those found in market trends, customer behavior, and natural occurrences, can form the basis for major companies and scienti c breakthroughs. They can also provide insight in everyday life. As an example, consider being a rst-time parent. Lucky parents have a baby who goes to sleep easily and sleeps through the night at one month old. The rest of us have to hear all the advice: use a rocker, swaddle them, let them cry it out, don’t let them cry it out, co-sleep, change the baby’s diet, change the mother’s diet, and on and on. Our older son never wanted to be put down, but our pediatrician nevertheless advised us to put him down when he was sleepy but still awake. That always led to him screaming the minute he was set down. If he wasn’t deeply asleep, he would just rouse himself and start crying. The rst few nights of this were harrowing, with each of us taking turns staying awake and holding him while he slept; he may have slept on his own for an hour a night. We had to nd another way. Through experimentation and collecting our own data over the rst few weeks (see scienti c method in Chapter 4), we discovered that our son liked a tight swaddle and would fall asleep in an electric swing, preferably on the highest setting. When he grew out of the swaddle, we feared that we were going back to square one. Luckily, he quickly adapted, and before he turned one, he could easily be put down and sleep straight through the night. When we had our second son, we thought of ourselves as baby-care professionals. We had our magic swing and we thought we were all set. And then, per Murphy’s law (see Chapter 2), baby number two hated the swing. We circled back through all the advice, and after a few days, we tried to set him down when he was sleepy but awake (per our pediatrician’s original advice). Lo and behold, he put himself to sleep! Like babies and their sleep procedures, many aspects of life have inherent variability and cannot be predicted with certainty. Will it rain today? Which funds should you invest your retirement money in? Who are the best players to draft for your fantasy football team? Despite this uncertainty, you still have to make a lot of choices, from decisions about your health to deciding whom to vote for to taking a risk with a new project at work. This chapter is about helping you think about wading through such uncertainty in the context of decision making. What advice should you listen to and why? Probability and statistics are the branches of mathematics that give us the most useful mental models for these tasks. As French mathematician Pierre-Simon Laplace wrote in his 1812 book Théorie Analytique des Probabilités: “The most important questions of life are indeed, for the most part, really only problems of probability.” We will discuss the useful mental models from the elds of probability and statistics along with common traps to avoid. While many of the basic concepts of probability are fairly intuitive, your intuition often fails you (as we’ve seen throughout this book). Yes, that means some of this chapter is a bit mathematical. However, we believe that an understanding of these concepts is needed for you to understand the statistical claims that you encounter on a daily basis, and to start to make your own. We’ve tried to include only the level of detail that is really needed to start to appreciate these concepts. And, as always, we’ve included plenty of examples to help you grasp them. TO BELIEVE OR NOT BELIEVE It is human nature to use past experience and observation to guide decision making, and evolutionarily this makes sense. If you watched someone get sick after they ate a certain food or get hurt by behaving a certain way around an animal, it follows that you should not copy that behavior. Unfortunately, this shortcut doesn’t always result in good thinking. For example: We had a big snowstorm this year; so much for global warming. My grandfather lived to his eighties and smoked a pack a day for his whole life, so I don’t believe that smoking causes cancer. I have heard several news reports about children being harmed. It is so much more dangerous to be a child these days. I got a runny nose and cough after I took the u vaccine, and I think it was caused by the vaccine. These are all examples of drawing incorrect conclusions using anecdotal evidence, informally collected evidence from personal anecdotes. You run into trouble when you make generalizations based on anecdotal evidence or weigh it more heavily than scienti c evidence. Unfortunately, as Michael Shermer, founder of the Skeptics Society, points out in his 2011 book The Believing Brain, “Anecdotal thinking comes naturally, science requires training.” One issue with anecdotal evidence is that it is often not representative of a full range of experiences. People are more inclined to share out-of-the- ordinary stories. For instance, people are more likely to write a review when they had a terrible experience or an amazing experience. As a result, the only takeaway from an anecdote is that a single event may have occurred. If you hear an anecdote about someone who smoked and escaped lung cancer, that only proves you are not guaranteed to get lung cancer if you smoke. However, based solely on this anecdote, you cannot draw a conclusion on the chances that an average smoker will get cancer or the relative likelihood of smokers getting lung cancer compared with nonsmokers. If everyone who ever smoked got lung cancer and everyone who didn’t smoke never got lung cancer, the data would be a lot more convincing. Unfortunately, the real world is rarely that simple. You may have heard anecdotes about people who happened to get cold and u symptoms around the time that they got the u vaccine and blame their illness on the vaccine. Just because two events happened in succession, or are correlated, doesn’t mean that the rst actually caused the second. Statisticians use the phrase correlation does not imply causation to describe this fallacy. What is often overlooked when this fallacy arises is a confounding factor, a third, possibly non-obvious factor that in uences both the assumed cause and the observed e ect, confounding the ability to draw a correct conclusion. In the case of the u vaccine, the cold and u season is that confounding factor. People get the u vaccine during the time of year when they are more likely to get sick, whether they have received the vaccine or not. Most likely the symptoms people are experiencing are from a common cold, which the u vaccine does not protect against. In other instances, a correlation can occur by random chance. It’s easier than ever to test the correlation between all sorts of information, so many spurious correlations are bound to be discovered. In fact, there is a hilarious site (and book) called Spurious Correlations, chock-full of these silly results. The graph below shows one such correlation, between cheese consumption and deaths due to bedsheet tanglings. Correlation Does Not Imply Causation One time when Lauren was in high school, she started feeling like a cold was coming on, and her dad told her to drink plenty of uids to help her get better. She proceeded to drink half a case of raspberry Snapple that day, and, surprisingly, the next day she felt a lot better! Was this clear evidence that raspberry Snapple is a miracle cure for the common cold? No. She probably just experienced a coincidental recovery due to the body’s natural healing ability after also drinking a whole bunch of raspberry Snapple. Or maybe she wasn’t sick at all; maybe she was just randomly having a bad day, followed by a more regular day. Many purveyors of homeopathic “treatments” include similar anecdotal reports of coincidental recoveries in advertisements for their products. What is not mentioned is what would have happened if there were no “treatment.” After all, even when you are sick, your symptoms will vary day by day. You should require more credible data, such as a thorough scienti c experiment, before you believe any medical claims on behalf of a product. If you set out to collect or evaluate scienti c evidence based on an experiment, the rst step is to de ne or understand its hypothesis, the proposed explanation for the e ect being studied (e.g., drinking Snapple can reduce the length of the common cold). De ning a hypothesis up front helps to avoid the Texas sharpshooter fallacy. This model is named after a joke about a person who comes upon a barn with targets drawn on the side and bullet holes in the middle of each target. He is amazed at the shooter’s accuracy, only to nd that the targets were drawn around the bullet holes after the shots were red. A similar concept is the moving target, where the goal of an experiment is changed to support a desired outcome after seeing the results. One method to consider, often referred to as the gold standard in experimental design, is the randomized controlled experiment, where participants are randomly assigned to two groups, and then results from the experimental group (who receive a treatment) are compared with the results from the control group (who do not). This setup isn’t limited to medicine; it can be used in elds such as advertising and product development. (We will walk through a detailed example in a later section.) A popular version of this experimental design is A/B testing, where user behavior is compared between version A (the experimental group) and version B (the control group) of a site or product, which may di er in page ow, wording, imagery, colors, etc. Such experiments must be carefully designed to isolate the one factor you are studying. The simplest way to do this is to change just one thing between the two groups. Ideally, experiments are also blinded, so that participants don’t know which group they are in, preventing their conscious and unconscious bias from in uencing the results. The classic example is a blind taste test, which ensures that people’s brand a nities don’t in uence their choice. To take the idea of blinding one step further, the people administering the experiment or analyzing the experiment can also remain unaware of which group the participants are in. This additional blinding helps reduce the impact of observer-expectancy bias (also called experimenter bias), where the cognitive biases of the researchers, or observers, may cause them to in uence the outcome in the direction they expected. Unfortunately, experimenter blinding doesn’t completely prevent observer-expectancy bias, because researchers can still bias results in the preparation and analysis of a study, such as by engaging in selective background reading, choosing hypotheses based on preconceived notions, and selectively reporting results. In medicine, researchers go to great lengths to achieve properly blinded trials. In 2014, the British Medical Journal (BMJ) published a review by Karolina Wartolowska et al. of fifty-three studies that compared an actual surgical intervention with a “sham” surgery, “including the scenario when a scope was inserted and nothing was done but patients were sedated or under general anesthesia and could not distinguish whether or not they underwent the actual surgery.” These fake surgeries are an example of a placebo, something that the control participants receive that looks and feels like what the experimental participants receive, but in reality is supposed to have no e ect. Interestingly, just the act of receiving something that you expect to have a positive e ect can actually create one, called the placebo e ect. While placebos have little e ect on some things, like healing a broken bone, the placebo e ect can bring about observed bene ts for numerous ailments. The BMJ review reported that in 74 percent of the trials, patients receiving the fake surgeries saw some improvement in their symptoms, and in 51 percent of the trials, they improved about as much as the recipients of actual surgeries. For some conditions, there is even evidence to suggest that the placebo e ect isn’t purely a gment of the imagination. As an example, placebo “pain relievers” can produce brain activity consistent with the activity produced by actual pain-relieving drugs. For all the parents out there, this is why “kissing a boo-boo” actually can help make it better. Similarly, anticipation of side e ects can also result in real negative e ects, even with fake treatments, a phenomenon known as the nocebo e ect. One of the hardest things about designing a solid experiment is de ning its endpoint, the metric that is used to evaluate the hypothesis. Ideally, the endpoint is an objective metric, something that can be easily measured and consistently interpreted. Some examples of objective metrics include whether someone bought a product, is still alive, or clicked a button on a website. However, when the concept that researchers are interested in studying isn’t clearly observable or measurable, they must use a proxy endpoint (also called a surrogate endpoint or marker), a measure expected to be closely correlated to the endpoint they would measure if they could. A proxy essentially means a stand-in for something else. Other uses of this mental model include the proxy vote (e.g., absentee ballot) and proxy war (e.g., current con icts in Yemen and Syria are a proxy war between Iran and Saudi Arabia). While there is no one objective measure of the quality of a university, every year U.S. News and World Report tries to rank schools against one another using a proxy metric that is a composite of objective measures, such as graduation rates and admission data, along with more subjective measures, such as academic reputation. Other examples of common proxy metrics include the body mass index (BMI), used to measure obesity, and IQ, used to measure intelligence. Proxy metrics are more prone to criticism because they are indirect measures, and all three of these examples have been criticized signi cantly. As an example of why this criticism can be valid, consider abnormal heart rhythms (ventricular arrhythmias) that can cause sudden death. Anti- arrhythmic drugs have been developed that prevent ventricular arrhythmias, and so it would seem obvious that these drugs would be expected to prevent sudden death in the patients who take them. But use of these drugs actually leads to a signi cant increase in sudden death in patients with asymptomatic ventricular arrhythmias after a heart attack. For these patients, the reduced post-treatment rate of ventricular arrhythmias is not indicative of improved survival and is therefore not a good proxy metric. However, despite the complications that arise when conducting well-run experiments, collecting real scienti c evidence beats anecdotal evidence hands down because you can draw believable conclusions. Yes, you have to watch out for spurious correlations and subtle biases (more on that in the next section), but in the end you have results that can really advance your thinking. HIDDEN BIAS In the last section, we mentioned a few things to watch out for when reviewing or conducting experiments, such as observer-expectancy bias and confounding factors. There are a few more of these subtle concepts to be wary of. First, sometimes it is not ethical or practical to randomly assign people to di erent experimental groups. For example, if researchers wanted to study the e ect of smoking during pregnancy, it wouldn’t be right to make nonsmoking pregnant women start smoking. The smokers in the study would therefore be those who selected to continue smoking, which can introduce a bias called selection bias. With selection bias, there is no guarantee that the study has isolated smoking to be the only di erence between these groups. So if there is a di erence detected at the end of the study, it cannot be easily determined how much smoking contributed to this di erence. For instance, women who choose to continue smoking during their pregnancy against the advice of doctors may similarly make other medically questionable choices, which could drive adverse outcomes. Selection bias can also occur when a sample is selected that is not representative of the broader population of interest, as with online reviews. If the group studied isn’t representative, then the results may not be applicable overall. Essentially, you must be really careful when drawing conclusions based on nonrandom experiments. The Dilbert cartoon above pokes fun at the selection bias inherent in a lot of the studies reported in the news. A similar selection bias occurs with parents and school choice for their kids. Parents understandably want to give their kids a leg up and will often move or pay to send their kids to “better schools.” However, is the school better because there are better teachers or because the students are better prepared due to their parents’ nancial means and interest in education? Selection bias likely explains some signi cant portion of these schools’ better test scores and college admissions. Another type of selection bias, common to surveys, is nonresponse bias, which occurs when a subset of people don’t participate in an experiment after they are selected for it, e.g., they fail to respond to the survey. If the reason for not responding is related to the topic of the survey, the results will end up biased. For instance, let’s suppose your company wants to understand whether it has a problem with employee motivation. Like many companies, you might choose to study this potential problem via an employee engagement survey. Employees missing the survey due to a scheduled vacation would be random and not likely to introduce bias, but employees not lling it out due to apathy would be nonrandom and would likely bias the results. That’s because the latter group is made up of disengaged employees, and by not participating, their disengagement is not being captured. Surveys like this also do not usually account for the opinions of former employees, which can create another bias in the results called survivorship bias. Unhappy employees may have chosen to leave the company, but you cannot capture their opinions when you survey only current employees. Results are therefore biased based on measuring just the population that survived, in this case the employees remaining at the company. Do these biases invalidate this survey methodology? Not necessarily. Almost every methodology has drawbacks, and bias of one form or another is often unavoidable. You should just be aware of all the potential issues in a study and consider them when drawing conclusions. For example, knowing about the survivorship bias in remaining employees, you could examine the data from exit interviews to see whether motivation issues were mentioned by departing employees. You could even try to survey them too. A few other examples can further illustrate how subtle survivorship bias can be. In World War II, naval researchers conducted a study of damaged aircraft that returned from missions, so that they could make suggestions as to how to bolster aircraft defenses for future missions. Looking at where these planes had been hit, they concluded that areas where they had taken the most damage should receive extra armor. However, statistician Abraham Wald noted that the study sampled only planes that had survived missions, and not the many planes that had been shot down. He therefore theorized the opposite conclusion, which turned out to be correct: that the areas with holes represented areas where aircraft could be shot and still return safely, whereas the areas without holes probably contained areas that, if hit, would cause the planes to go down. Similarly, if you look at tech CEOs like Bill Gates and Mark Zuckerberg, you might conclude that dropping out of school to pursue your dreams is a ne idea. However, you’d be thinking only of the people that “survived.” You’re missing all the dropouts who did not make it to the top. Architecture presents a more everyday example: Old buildings generally seem to be more beautiful than their modern counterparts. Those buildings, though, are the ones that have survived the ages; there were slews of ugly ones from those time periods that have already been torn down. Survivorship Bias When you critically evaluate a study (or conduct one yourself), you need to ask yourself: Who is missing from the sample population? What could be making this sample population nonrandom relative to the underlying population? For example, if you want to grow your company’s customer base, you shouldn’t just sample existing customers; that sample doesn’t account for the probably much larger population of potential customers. This much larger potential customer base may behave very di erently from your existing customer base (as is the case with early adopters versus the early majority, which we described in Chapter 4). One more type of bias that can be inadvertently introduced is response bias. While nonresponse bias is introduced when certain types of people do not respond, for those who do respond, various cognitive biases can cause them to deviate from accurate or truthful responses. For example, in the employee engagement survey, people may lie (by omission or otherwise) for fear of reprisal. In general, survey results can be in uenced by response bias in a number of ways, including the following: How questions are worded, e.g., leading or loaded questions The order of questions, where earlier questions can in uence later ones Poor or inaccurate memory of respondents Di culty representing feelings in a number, such as one-to-ten ratings Respondents reporting things that re ect well on themselves It’s worth trying to account for all of these subtle biases (selection bias, nonresponse bias, response bias, survivorship bias), because after you do so, you can be even more sure of your conclusions. BE WARY OF THE “LAW” OF SMALL NUMBERS When you interpret data, you should watch out for a basic mistake that causes all sorts of trouble: overstating results from a sample that is too small. Even in a well-run experiment (like a political poll), you cannot expect to get a good estimate based on a small sample. This fallacy is sometimes referred to as the law of small numbers, and this section explores it in more detail. The name is derived from a valid statistical concept called the law of large numbers, which states that the larger the sample, the closer your average result is expected to be to the true average. The gure below shows this in action. Each line represents a di erent series of coin ips and shows how the percentage of heads changes from the rst to the ve hundredth ip for each series. Note how the curves may deviate quite a bit from the 50 percent mark in the beginning, but start converging closer and closer toward 50 percent as the number of ips increases. But even out to ve hundred ips, some of the values are still a fair bit away from 50 percent. Law of Large Numbers The speed of convergence for a given experiment depends on the situation. We will explain in a later section how you know when you have a large enough sample. For now, we want to focus on what can go wrong if your sample is too small. First, consider the gambler’s fallacy, named after roulette players who believe that a streak of reds or blacks from a roulette wheel is more likely to end than to continue with the next spin. Suppose you see ten blacks in a row. Those who fall victim to this fallacy expect the next spin to have a higher chance of coming up red, when in fact the underlying probability of each spin hasn’t changed. For this fallacy to be true, there would have to be some kind of corrective force in the roulette wheel that is bringing the results closer to parity. That’s simply not the case. It’s sometimes called the Monte Carlo fallacy because in a widely cited case in August 18, 1913, a casino in Monte Carlo had an improbable run of twenty-six blacks! There is only a 1 in 137 million chance of this happening in any twenty-six-ball sequence. However, all other twenty-six-spin sequences are equally rare; they just aren’t all as memorable. The gambler’s fallacy applies anywhere there is a sequence of decisions, including those by judges, loan o cers, and even baseball umpires. In a University of Chicago review of refugee asylum cases from 1985 to 2013, published in the Quarterly Journal of Economics as “Decision-Making Under the Gambler’s Fallacy: Evidence from Asylum Judges, Loan O cers, and Baseball Umpires,” judges were less likely to approve an asylum case if they had approved the last two. It also explains that uncomfortable feeling you might have gotten as a student when you saw that you had chosen answer B four times in a row on a multiple-choice test. Random data often contains streaks and clusters. Are you surprised to learn that there is a 50 percent chance of getting a run of four heads in a row during any twenty- ip sequence? Streaks like this are often erroneously interpreted as evidence of nonrandom behavior, a failure of intuition called the clustering illusion. Look at the pair of pictures on the next page. Which is randomly generated? These pictures come from psychologist Steven Pinker’s book The Better Angels of Our Nature. The left picture—the one with the obvious clusters—is actually the one that is truly random. The right picture—the one that intuitively seems more random—is not; it is a depiction of the positions of glowworms on the ceiling of a cave in Waitomo, New Zealand. The glowworms intentionally space themselves apart from one another in the competition for food. Clustering Illusion In World War II, Londoners sought to nd a pattern to the bombings of their city by the Germans. Some became convinced that certain areas were being targeted and others were being spared, leading to conspiracy theories about German sympathizers in certain neighborhoods that didn’t get hit. However, statistical analysis showed that there was no evidence to support claims that the bombings were nonrandom. The improbable should not be confused with the impossible. If enough chances are taken, even rare events are expected to happen. Some people do win the lottery and some people do get struck by lightning. A one-in-a- million event happens quite frequently on a planet with seven billion people. In the U.S., public health o cials are asked to investigate more than one thousand suspected cancer clusters each year. While historically there have been notable cancer clusters caused by exposure to industrial toxins, the vast majority of the cases reported are due to random chance. There are more than 400,000 businesses with fty or more employees; that’s a lot of opportunities for a handful of people to receive the same unfortunate diagnosis. Knowing the gambler’s fallacy, you shouldn’t always expect short-term results to match long-term expectations. The inverse is also true: you shouldn’t base long-term expectations on a small set of short-term results. You might be familiar with the phrase sophomore slump, which describes scenarios such as when a band gets rave reviews for their rst album and the second one isn’t as well received, or when a baseball player has a fantastic rookie season but the next year his batting average is not that impressive. In these situations, you may assume there must be some psychological explanation, such as caving under the pressure of success. But in most cases, the true cause is purely mathematical, explained through a model called regression to the mean. Mean is just another word for average, and regression to the mean explains why extreme events are usually followed by something more typical, regressing closer to the expected mean. For instance, a runner is not expected to follow a record-breaking race with another record-breaking time; a slightly less impressive performance would be expected. That’s because a repeat of a rare result is equally as rare as its rst occurrence, such that it shouldn’t be expected the next time. The takeaway is that you should never assume that a result based on a small set of observations is typical. It may not be representative of either another small set of observations or a much larger set of observations. Like anecdotal evidence, a small sample tells you very little beyond that what happened was within the range of possible outcomes. While rst impressions can be accurate, you should treat them with skepticism. More data will help you distinguish what is likely from what is an anomaly. THE BELL CURVE When you are dealing with a lot of data, you can use graphs and summary statistics to combat the feeling of information overload (see Chapter 2). The term statistics is actually just the name for numbers used to summarize a dataset. (It also refers to the mathematical process by which those numbers are generated.) Graphs and summary statistics succinctly communicate facts about the dataset. You use summary statistics all the time without even realizing it. If someone asked you, “What is the temperature of a healthy person?” you’d likely say it was 98.6 degrees Fahrenheit or 37 degrees Celsius. That’s actually a summary statistic called the mean, which, as we just explained, is another word for average. You probably don’t even remember when you rst learned that fact, and it’s even more likely you have no idea where that number comes from. A nineteenth-century German physician, Dr. Carl Wunderlich, diligently collected and analyzed more than a million armpit temperatures from twenty- ve thousand patients to calculate that statistic (yes, that’s a lot of armpits). Yet 98.6 degrees Fahrenheit isn’t some magical temperature. First of all, more recent data indicates a lower mean, closer to 98.2 degrees. Second, you may have noticed from taking your own temperature or that of a family member that “normal” temperatures vary from this mean. In fact, women are slightly warmer than men on average, and temperatures of up to 99.9°F (37.7°C) are still considered normal. Third, people’s temperatures also naturally change throughout the day, moving up on average by 0.9°F (0.5°C) from morning to night. Just saying a healthy temperature is 98.6°F doesn’t account for all of this nuance. That’s why a range of summary statistics and graphs are often used on a case-by-case basis to summarize data. The mean (average or expected value) measures central tendency, or where the values tend to be centered. Two other popular summary statistics that measure central tendency are the median (middle value that splits the data into two halves) and the mode (the most frequent result). These statistics help describe what a “typical” number might look like for a given set of data. For body temperature, though, just reporting the central tendency, such as the mean, can at times be too simplistic. This brings us to the second common set of summary statistics, those that measure dispersion, or how far the data is spread out. The simplest dispersion statistics report ranges. For body temperature, that could be specifying the range of values considered normal, e.g., minimum to maximum reported values from healthy people, as in the graph below (called a histogram). Histogram The graph on the previous page depicts the frequencies of 130 di erent body temperatures derived from a study of healthy adults. A histogram like this one is a simple way that you can summarize data visually: group the values into buckets, count how many data points are in each bucket, and make a vertical bar graph of the buckets. Before reporting a range, you might rst look for outliers, those data points that don’t seem to t with the rest of the data. These are the data points set apart in the histogram, such as the one at 100.8°F. Perhaps a sick person sneaked into the dataset. As a result, you might report a normal temperature range of 96.3°F to 100.0°F. Of course, with more data, you could produce a more accurate range. In this dataset, central tendency statistics are quite similar because the distribution of the data is fairly symmetric, with just one peak in the middle. As a result, the mean is 98.25°F, the median is 98.3°F, and the mode is 98°F. In other scenarios, though, these three summary statistics may be quite di erent. To illustrate this, consider another histogram, below, showing the distribution of U.S. household income in 2016. This dataset also has one peak, at $20,000–$24,999, but it is asymmetric, skewing to the right. (All incomes above $200,000 are grouped into one bar; had this not been the case, the graph would have a long tail stretching much farther to the right.) Unlike for the body temperatures, the median income of $59,039 is very di erent from the mean income of $83,143. Whenever the data is skewed in one direction like this, the mean gets pulled away from the median and toward the skew, swayed by the extreme values. Distribution of U.S. Household Income (2016) Also, a minimum–maximum range is less informative here. A better summary of the dispersion in this case might be an interquartile range specifying the 25th percentile to the 75th percentile of the data, which captures the middle 50 percent of incomes, from $27,300 to $102,350. The most common statistical measures of dispersion, though, are the variance and the standard deviation (the latter usually denoted by the Greek letter σ, sigma). They are both measures of how far the numbers in a dataset tend to vary from its mean. The following gure shows how you calculate them for a set of data. Variance & Standard Deviation Number of observations: n = 5 Observations: 5, 10, 15, 20, 25 Sample mean: (5+10+15+20+25)/5 = 75/5 = 15 Data point deviations from sample mean, squared: (5-15)2 = (-10)2 = 100 (10-15)2 = (-5)2 = 25 (15-15)2 = (0)2 = 0 (20-15)2 = (5)2 = 25 (25-15)2 = (10)2 = 100 Sample variance: (100+25+0+25+100)/(n-1) = 250/(5-1) = 250/4 = 62.5 Sample standard deviation (δ): √(variance) = √(62.5) = 7.9 Because the standard deviation is just the square root of the variance, if you know one, then you can easily calculate the other. Higher values for each indicate that it is more common to see data points further from the mean, as shown in the targets below. Variance Low Variance High Variance The body temperature dataset depicted earlier has a standard deviation of 0.73°F. Slightly more than two-thirds of its values fall within one standard deviation from its mean (97.52°F to 98.98°F) and 95 percent within two standard deviations (96.79°F to 99.71°F). As you’ll see, this pattern is commonplace for many datasets consisting of measurements (e.g., heights, blood pressure, standardized tests). Histograms of these types of datasets have similar bell-curve shapes with a cluster of values in the middle, close to the mean, and fewer and fewer results as you go further away from the mean. When a set of data has this type of shape, it is often suggested that it comes from a normal distribution. The normal distribution is a special type of probability distribution, a mathematical function that describes how the probabilities for all possible outcomes of a random phenomenon are distributed. For example, if you take a random person’s temperature, getting any particular temperature has a certain probability, with the mean of 98.2°F being the most probable and values further away being less and less probable. Given that a probability distribution describes all the possible outcomes, all probabilities in a given distribution add up to 100 percent (or 1). To understand this better, let’s consider another example. As mentioned above, people’s heights also roughly follow a normal distribution. Below is a graphical representation of the distribution of men’s and women’s heights based on data from the U.S. Centers for Disease Control and Prevention. The distributions both have the typical bell-curve shape, even though the men’s and women’s heights have di erent means. Normal Distribution In normal distributions like these (and as we saw with the body temperatures), approximately 68 percent of all values should fall within one standard deviation of the mean, about 95 percent within two, and nearly all (99.7 percent) within three. In this manner, a normal distribution can be uniquely described by just its mean and standard deviation. Because so many phenomena can be described by the normal distribution, knowing these facts is particularly useful. Normal Distribution Standard Deviations So, if you stopped a random woman on the street, you could use these facts to form a likely guess for her height. A guess of around ve feet four inches (162 centimeters) would be best, as that’s the mean. Additionally, you could, with about two-to-one odds, guess that she will have a height between ve feet one inch and ve feet seven inches. That’s because the standard deviation of women’s heights is slightly less than three inches, so about two-thirds of women’s heights will fall within that range (within one standard deviation of the mean). By contrast, women shorter than four feet ten inches or taller than ve feet ten inches make up less than about 5 percent of all women (outside two standard deviations from the mean). Probability Distributions Log-normal distribution Applies to phenomena that follow a power law relationship, such as wealth, the size of cities, and insurance losses. Poisson distribution Applies to independent and random events that occur in an interval of time or space, such as lightning strikes or numbers of murders in a city. Exponential distribution Applies to the timing of events, such as the survival of people and products, service times, and radioactive particle decay. There are many other common probability distributions besides the normal distribution that are useful across a variety of circumstances. A few are depicted in the gure on the previous page. We called this section “The Bell Curve,” however, because the normal distribution is especially useful due to one of the handiest results in all of statistics, called the central limit theorem. This theorem states that when numbers are drawn from the same distribution and then are averaged, this resulting average approximately follows a normal distribution. This is the case even if the numbers originally came from a completely di erent distribution. To appreciate what this theorem means and why it is so useful, consider the familiar opinion poll that determines an approval rating, such as for the U.S. Congress. Each person is asked whether they approve of Congress or not. That means the individual data points are each just a yes or a no. This type of data looks nothing like a normal distribution, as each data point can take only one of two possible values. Binary data like this is often analyzed using a di erent probability distribution, called the Bernoulli distribution, which represents the result of a single yes/no-type experiment or question, such as from a survey or poll. This distribution is useful in a wide variety of situations, such as analyzing advertising campaigns (whether someone purchased or not), clinical trials (responded to treatment or not), and A/B testing (clicked or not). The estimated approval rating is just an average of all of the di erent individual answers (1 for approval and 0 otherwise). F