Baseball Salary Analysis (PDF)
Document Details
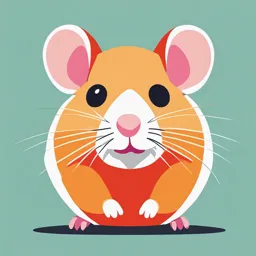
Uploaded by TruthfulRealism2101
Princess Nourah Bint Abdulrahman University
Tags
Summary
This document analyzes the relationship between baseball player salaries and on-base percentage and slugging percentage across multiple eras. It examines how the value of these statistics has been perceived in different time periods, potentially influenced by the "Moneyball" era, using regression analysis.
Full Transcript
Now we've created the functions we need. Let's run them to see what the results look like. The first thing we're going to do is define the three different eras we're going to look at in the data. We have 22 years of data. We're going to divide that into a Pre-Moneyball Era running from 1994 to 2000....
Now we've created the functions we need. Let's run them to see what the results look like. The first thing we're going to do is define the three different eras we're going to look at in the data. We have 22 years of data. We're going to divide that into a Pre-Moneyball Era running from 1994 to 2000. A Moneyball Era, which we're going to define is the period 2001-2007 and the Post Moneyball Era, which is going to be the final eight years, 2008-2015. We just define those using our Lm season command that we've already been working with, and we set the range of numbers of observations which are covered by this, the number of different functions, number of different regressions that we want to include. The first one is up to seven, then we run includes 7-14 and finally, we will include 14 up to the end. If we run that now you can see that these eras are defined here as the first seven years, the second seven years, and the last seven years. Now let's actually run the regressions to produce the tables. As before, we want the data to be produced in summary columns so that we can compare the results across years. We can define the summary columns based on the lm results for each of the groups of regressions that we've included. The first one here includes the summary columns for the regression zero up to regression 6. Those are the first seven in our list and they should be number 1994-2000. If we run this now, we will see indeed we get this output here. Now of course, what we're really interested in is the relationship between salary and on base percentage and slugging in this Pre-Moneyball Era. We can look at the other variables and I advise you to do that separately to see what you think about the patent of relationships, but the main thing to note here is that in this Pre-Moneyball Era, slugging percentage is consistently statistically significant and on base percentage is statistically significant in only one out of the seven years in 1998. Just a note to mention here, the statistical significance can be identified with the number of stars which are attached and note that the number of stars, one star means statistically significant at the 10 percent level. Two stars mean statistically significant at the 5 percent level, and three stars means statistically significant the 1 percent level. In fact, as a general rule, I would ignore statistical significance with one star of the 10 percent level. That's not really a very reliable indicator. I'm going to think of statistical significance in terms of two or more stars for the regressions, but you can see that slugging is statistically significant or by that standard in every season in this Pre-Moneyball era, whereas on base percentage is statistically significant early once out of seven years. Let's now look at the Moneyball era and see what we get in this period. Now we can see somewhat different results. In fact, we can see that as far as on base percentage is concerned, the actual statistical significance in most years has not changed at all. We can see in 2001 it's actually negative and statistically insignificant. That suggests that players with a higher on base percentage actually faced a salary penalty for having that high statistic, which is clearly something which contradicts basic expectation that better statistics should lead to higher salaries. Whereas slugging percentage continues to be statistically significant in five of the seven years, and only insignificant in 2003 and 2004. But what stands out, most of all from this table is the significance of on base percentage in 2004, the year of the Moneyball is published. In this year, on base percentage is way larger than it is in any other season. It is statistically significant and slugging percentage is not statistically significant. It's as if in the year following the publication of Moneyball, every team went out and bought players only on the basis of on base percentage and on no other criteria and whatever, but that after 2004, they went back to thinking about slugging percentage. That's one interpretation that you could make of this data. Now, it's also noticeable to say that if you look at the size of the coefficient of on base percentage after 2004, it is always larger than the size of the coefficient on slugging percentage. That suggest some changes going on about in the evaluation of on base percentage, but it's never statistically significant. Which suggests that there's a certain amount of uncertainty about the value of on-base percentage. Effectively, you might argue or one interpretation would be that scouts and general managers were not entirely convinced in this era, although, clearly some adjustments seems to be taking place. Now, let's look into the most recent era, the post Moneyball era, and see what the regression looks like from 2008 onwards. Now, we see in this period, again, a slightly different story. Now, in this era, neither on-base percentage nor slugging percentage seem to be consistently statistically significant. We can see on-base percentage is significant in 2009, 2010, and 2015 whereas slugging percentage is only statistically significant at the five percent level of better in 2011. It seems perhaps, it's the case that teams are looking at more complex statistics and on-base percentage or slugging percentage or some other changes taking place which might account for these differences. Certainly, again, you can see that in many seasons, on-base percentage, the size of the coefficient is larger than the coefficient on slugging percentage. But again, the statistical precision is limited, so we should be cautious about the interpretation of that result. That's when we look it year by year. But as you can see from the last row in each of these tables, the number of observations is relatively small. In each season, we have somewhere between 100 and 150 free agents whose salaries we can look at. You might argue that that's a relatively small dataset in order to identify these effects. A final thing we can do here is pull the data to see whether when we include all of the years simultaneously, whether we can identify a post Moneyball effect in the data. This is a simpler exercise than the functions we've been running up until now, we're just going to run a single regression covering the entire data period. We're going to regress salary on all the variables that we've looked at before. But in addition, we're going to interact each variable with a post Moneyball dummy, a dummy which sees value zero before the publication of Moneyball on one afterwards. What I will do is allow us to see if the relationship between salary and on-base percentage and slugging changes in the post Moneyball era. Let's first create that dataset so that we can run this regression for all of these years simultaneously. We've got that data here, which will just run some summary statistics so we can see what the data is we're looking at. You can see that the top row tells us how many observations we have. We have over 3,000 observations and that enables us to run a regression which is probably going to allow us to generate more statistically precise estimates than the set of small datasets that we've been looking at up until now. Let's run the regression and see what the results look like. Here is the table of results and the list of the coefficients. We're focusing as ever on on-base percentage and slugging. If you look down the column, you can see the first case where on-base percentage and slugging up here, you can see that on-base percentage is statistically insignificant and slugging percentage is highly statistically significant. The slugging percentage coefficient is something like 10 times larger that the on-base percentage coefficient and that's across the entire DataQuery, taking all the 22 seasons into account. But now, let's go down the table and look at the post Moneyball coefficients, the interaction. You can see these are the rows listed, PostMB: OBP, and PostMB: SLG. Those tell us what the relationship was between on-base percentage and salaries and slugging and salaries in the post Moneyball era. The way to think about is add these two, the coefficients of OBP and SLG that we just discussed. That is the impact in the post Moneyball era, the two combined. These individual coefficients measure the change that occurs where the post Moneyball era starts. What you can see here is very striking. The coefficient on on-base percentage post Moneyball goes up by 2.57, a statistically significant amount and the coefficient on slugging goes down by 1.52 also, a statistically significant amount. This says that in the post Moneyball era, there was indeed a rebalancing of the valuation of on-base percentage and slugging to the point where on-base percentage, if anything, has a more significant role in determining player salaries in the post Moneyball era. When we look over this longer period, we find that somewhat more striking confirmation of the money help bull hypothesis running our data right up to the recent period, 2015.