Brain Function and Probabilistic Neuronal Coding PDF
Document Details
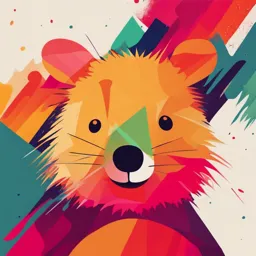
Uploaded by WellBalancedVerism
University of Nottingham
Tags
Summary
This document describes the brain's probabilistic approach to processing sensory information. It discusses how the brain anticipates future events and optimizes actions, using examples like predicting a basketball player's movements. The document focuses on predicting the future as a core function of the brain.
Full Transcript
We think that the brain works through probabilities and probabilistic neuronal coding - means the postsynaptic cell tries to understand the input its getting from the presynaptic cell through a combination of probability resaoning LTP for example can be thought of as an increase in probability that...
We think that the brain works through probabilities and probabilistic neuronal coding - means the postsynaptic cell tries to understand the input its getting from the presynaptic cell through a combination of probability resaoning LTP for example can be thought of as an increase in probability that a receptor becomes activated with the same amount of glutamate - if you've got a presynaptic cell releasing the same amount of glutamate every couple of seconds, you will get a response in the second order neurone on the postynaptic cell, but If you upregulate AMPA receptors on the postsynaptic cell your increasing the probability that the AMPA receptor will be activated - suddenly you have more AMPA receptors and an increased probability that the same amount of glutamate will activate the postsynaptic cell \-\--\> this means the probability of neurotransmission and synaptic strengthening has increased \-- this is essentially what long term potentiation (LTP) is Conversely LTD means that the same amount of glutamate will activate the postsynaptic cell less  The brain is always working on proability and what is the most statistically likely input that has caused that response/EPSP/AP  Our brain essentially generates models of the outside world and then our brain tries to predict the most likely sensory stimulus that caused that particular inputs - for example If you touch a hot kettle and get a painful stimulus your brain is reasoning it must be the kettle causing the pain because you getting visual input that you are touching the kettle and getting a painfull heat stimulus coming in from your hand - the next time you go into the kitchen you are going to be mindful in regards to the tempurature of the kettle - generated a model about the outside world and heat transfers from kettles - our brain needs to generate these models in order to predict the future and protect us from future injury One model that has been superceeded is that the brain passively absorbs sensory input, processes this information in some way and then reacts when controlling our motor and sensory responses to these passively experienced sensory stimuli However we don't just sit there and passively receive sensory information, our brain is constantly trying to predict the future so we can optimise movements and performance in order to carry out whatever task we are doing A more likely hypothesis of sensory information processing is active anticipation - based on the intended goal of a persons actions or movements, the brain actively searches for and anticipates information it expects to be present in that particular environment. The end goal and the persons motivation to activate that outcome will influence how the brain percieves and reacts to constantly changing environmental and sensory stimuli - latter model of perception incorporated the activoty of neural systems that regulate attention, motivation and reward pathways, necessary to acieve a desired outcome  If we look at the basketball player as an example, his goal is to score a basket, but there is so much sensory information coming into the brain, visual, auditory, he is looking around for teammates trying to anticipate their next moves, trying to avoid oncoming apponents and trying to filter out all of the noise of the crowd. He has a very one minded goal - get a basket or pass to a teammate so they can score a basket \-\--\> this action here activates lots of areas of the brain, areas like the ACC keep attention on the primary goal, whilst filtering out the crowd of the sound, the motivation system will be activated with dopamine being released to drive his goals forward, reward pathways are anticipating what will happen if he scores the basket - the NA and the VTA are getting ready to release dopamine for if he wins  Brain is not a passive sponge taking up sensory information and reacting to it, its actively trying to anticipate the future and predict the future so we can make the best movements to achieve our goal Our perception involves the process of probablistic inference The methods our brain uses to process sensory information may have quickly evolved to quickly identify and focus on events and stimuli In the outside world that are somewhat surprising or unpredictable and to adapt them accordingly One hypothesis states that the brain generates models of the world and it continually uses data input form sensory organs to update, refine and optimise generative models We have a model or idea of what a scene should look like, and our brain will naturally go to the unexpected irregularity of the scene as our brain does not like surprises or unpredictable events, so our brain constantly scans and predicts the environment to try and prevent this from happening Anything that is surprising about our external environment will set off alarm bells in our brain - this is known as a prediction error - our brain has predicted what we should see, but if we see something surprising prediction errors will be sent up to the brain and this will put our attention onto the statistical irregulairy When you start something new your usually useless, the brain learns from the mistakes made, and the next time the brain will be able to better predict the motor plans it needs to do in the future This leads to the idea that the brain works on probably inference. Our neural networks need to predict and compute the expected statistical regularities / the recurring patterns of the outside world, and the brain will apply a probabilistic model to generate predictions of future events. The brain may generate motoristic commands based on statistically likely events to occur. Throws up a philosophical question  If we think mathematically and about einsteins theory of relativity, it states that everybody\'s perception of reality is different as we all move relative to ourselves and our perception of reality is very slightly different from everybody else\'s, but we also think our perception of reality is guided by previous knowledge, so all of the things we leant as a child will guide our reality in the future  Where we grow up also likely influences how we perceive events  Do we actually perceive true reality or do we perceive a mixture of reality and what our brain is actually expecting to occur in that instance Illusions are a very good example of explaining how we may not perceive true realty  Thermal grill illusion \--\> we can get a painful cold stimulus if we put our hand over a thermal grill that has very cold steel grates and warm steel grates. The problem is if we have cold and warm stimuli coming into the substantia gelatinosa in the spinal cord and they are synapsing on the same interneurones we can get some opponency processing going on in the spinal cord and we can percieve a painful stimulus where there was no painful input  This is a similar process occurring in visual illusions, our brain is anticipating where it thinks things should be  The brain will try to make sense out of a chaotic world and make order where there is no order We as humans don't like chaos and unstructured things Try to make sense of all of the sensory information coming into the brain and predict the most statistically likely outcome  In the early 1900s the german psychologists behind the Gestalt theory introduced the dalmation scene and said - humans arent passive recipients of incoming imaged, rather we impose a structure on what we see  Brain will fill in the blanks where there are non which means we perceieves things in a different way than intended On the left axis we have the probability of an event occurring (between 0 and 1 / 100%) Look like Gaussian distributions but are in reality called probability density function - the area under the curve is always 1. if you have a probability density function that is very low it will be fatter than the probability density functions that are 1 \-- area under all of the curves will be one, so they will look different based on how high the probability is.  We have a set of generative models which we call prior beliefs - this prior belie system is a model of how we expect the world to behave in a particular situation  Our brain is constantly trying to predict the future based on our prior beliefs and current sensory input. Based on the outcome we can update our prior model - if we predict the outcome with 100% accuracy we don't need to update our model it was completely accurate, but If we get it wrong we need to update our prior model so that hopefully in the future our prior belief will be accurate  If our prior belief is completey wrong as we are in a situation we have never encountered before, the probability density function amplitude decreases, and we have a very imprecise prior based on a novel context. Reliance on visual stimulus is higher in the novel situation Another word for probability is Bayesian inference, and our brain uses this to predict the future We can think of the way that the brain computes information as a probabilistic statistically likely event that is going to occur some time in the future This has lead to lots of people saying the brain processes things in a Bayesian fashion The probablistic model that the brain uses is thought to adhere to bayes theorem The bayesian brain can be conceptuaized as a probability machine that constantly makes predictions about the world and then updates them based on the information it recieves from the sense  Bayes theorem permits the brain to compute an updated probability that something is true, based on the old probablity of something being true, with the addition of new information \--\> all this is saying is that the bayesian brain allows for us to learn and get better at a task as our brain is constantly updating our prior belief system / generative model about that particular situation  Example - get the painful stimulus and perceive this in sensory cortex and the upper neocortex, get lots of action potentials going up to the brain as prediction errors. Nociceptors were activated and pain was percieved, and all of the action potentials they didn't predict going up to the brain were actually prediction errors, what the brain uses these action potentials for is to update its prior model, and in the future will look out for warning signs to avoid this painful stimulus  The bayesian brain allows us to learn and update our generative models so in the future we can minimise the amount of prediction errors that get up to the brain  Its thought that our brain does not want to process all of the sensory information that comes into our brain at any one time as this would be too cost inefficient. The brain only wants to process the action potentials it dosent actually predict that would come up to the neocortex We think this is an energy efficent way to process sensory information by only processing the prediction errors (AP we didn't predict) so the brain can save energy The posterior is the probability that the hypothesis is true given the evidence - the cause of the sensory signals recieved (ie what the brain continuously tries to compute / predict in real time) Were constantly trying to predict the probability that the hypothesis is true given the evidence (this is all of the sensory stimuli coming into the brain  The posterior is equal to the likelihood X our prior knowledge (generative models learnt from experience) / the marginal (probablity the evidence is true)  We can extend bayes theorem denominator to state that the probability the hypothesis is true given the evidence is equal to the probability the hypothesis is truewith prior knowledge x the probability the evidence is true given the hypothesis is true  Can use this mathematical problem to solve real world problems - eg doctors when looking at disease and treatment Trying to solve the probability that the patient has the disease given that the test result is positive  Plugging the numbers in to the extended form of bayes theorem Therefore the updated probability based on the two positive tests is 90.75% This implies there is a 91% chance of the patient having the rare disease For every step in the iterative process the old posterior becomes the new prior - if this happens then we can very quickly learn and update our generative models to be very good in lots of different situations  Our prior (our model of the world) can change with each step and therefore bayesian computation in the brain facilitates learning  Learning and memory is a method used by the brain to ensure that our priors are as accurate as possible, which facilitates predictive coding. So its very likely that our memory has evolved not in order to help us remember events that have happened, but rather our memory is a byproduct of the brain trying to predict the future - rather than the brain having a store of loads of information/experiences, our memory is in fact a byproduct of the brain trying to use bayesian inference in order to optimise and predict the future Brain is constantly trying to predict what is going to happen a few moves ahead \ minimisation of prediction errors drives learning  Precision = the width of the probability density function Accuracy = the difference between the height of your prior knowledge and the height of posterior Ascending feed-forward neural pathways carry stimulus related information from the outside world While long-range feedback connections from higher cognitive centers and local laterally projecting interneuron populations provide natural neuroanatomical checkpoints for processing this external sensory input - we can think about the substantia gelatinosa of the spinal cord in terms of these feet forward projections of the NS which then meet neuronal anatomical checkpoints that we call neuronal ensembles, which are made up of second order and interneurons which are all modulating each other, and the activity of each other  Need to understand what all of this means from a neurophysiological point of view. If we imagine the senrory neurones are bringing in information and releasing glutamate, gluatamte is excitatory so will cause deoplarisations in the second order neurones (these are little EPSPs). The descending pathways will release information onto the interneurones, and these will release GABA and enkephalin which are inhibitory so will induce IPSPs These EPSPs and IPSPs will cancel each other out somewhat. Downward messages from the brain are the predictions which are the inhibitory neurotransmitters Some AP will be generated at the axon hillock, in the form of the spikes, and it is these spikes that are the prediction errors (shown \