Survey Research Study Guide PDF
Document Details
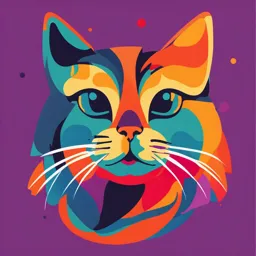
Uploaded by AdoredPlum8449
Tags
Summary
This study guide provides an overview of survey research methods, including different approaches, strengths, weaknesses, and best practices. It explores various survey types and their applications. The guide also examines reliability and validity in surveys, highlights common questionnaire issues, and discusses concepts like levels of measurement.
Full Transcript
1. Survey Research When to Conduct Survey Research: Appropriate: When little information is known about a large group or population, or when generalizable data is needed. It's ideal for studying a large sample efficiently. Comparison to Other Methods: ○ Survey vs. Conten...
1. Survey Research When to Conduct Survey Research: Appropriate: When little information is known about a large group or population, or when generalizable data is needed. It's ideal for studying a large sample efficiently. Comparison to Other Methods: ○ Survey vs. Content Analysis: Surveys collect original data from respondents, while content analysis examines existing media or documents. ○ Survey vs. Experiment: Surveys describe or explore relationships (correlation) without manipulation. Experiments test causality by controlling variables. ○ Survey vs. In-depth Interview: Surveys prioritize breadth over depth, using standardized questions to cover a wide audience, whereas in-depth interviews offer rich, qualitative insights. Common Survey Approaches: 1. Face-to-Face Interviews: High response rate; allows clarification but is costly and time-consuming. 2. Telephone Surveys: Fast and cost-effective but hindered by declining landline use. 3. Mail Surveys: Useful for reaching geographically dispersed populations; slower with lower response rates. 4. Group Administration: Efficient for specific groups (e.g., students in a classroom). 5. Online Surveys: Cost-effective and accessible but prone to low response rates and sampling bias. Strengths and Weaknesses of Survey Research: Strengths: ○ Efficient for large samples. ○ Can be generalized if using probability sampling. ○ Economically sound with wide scope. ○ Non-intrusive and convenient for respondents. Weaknesses: ○ Limited depth (no follow-up questions). ○ Potential sampling errors (nonresponse, self-selection bias). ○ Difficulty in ensuring honest responses due to social desirability or recall issues. Reliability and Validity: Reliability: Consistency of the measurement. Ensured by pretesting, clear questions, and training. Validity: Accuracy of measurement; does it measure what it’s intended to? Improved by well-constructed questions and representative sampling. Issues in Questionnaire Construction: Common Problems: ○ Double-barreled questions (e.g., "Do you like coffee and tea?"). ○ Biased or leading terms (e.g., "Don’t you agree?"). ○ Poorly defined terms or incomplete wording. ○ Overly complex recall or double negatives. Good Practices: ○ Use Gade's Top 10 list: Be clear, concise, and avoid leading or biased language. Avoid absolutes like "always" or "never." Ensure a balance in statements for agree/disagree responses. Levels of Measurement: 1. Nominal: ○ Categories without a logical order (e.g., gender: male/female). ○ Example: "What is your marital status?" (single, married, etc.) 2. Ordinal: ○ Ordered categories but unequal intervals (e.g., Likert scales like satisfaction: satisfied, neutral, dissatisfied). 3. Interval: ○ Ordered with equal intervals; no true zero (e.g., temperature in Celsius). 4. Ratio: ○ Ordered with equal intervals and a true zero (e.g., income, age). Application: ○ Identify the level of measurement in research hypotheses or questions to match with statistical tests. Survey Response Rate: Improvement Strategies: ○ Personalize invitations. ○ Offer incentives. ○ Send follow-up reminders. ○ Ensure survey simplicity and relevance to the audience. Here’s a completed section on Statistical Analysis using the uploaded lecture slides: 2. Statistical Analysis Descriptive vs. Inferential Statistics Descriptive Statistics: ○ Summarize characteristics of the dataset. ○ Types: Central tendency: Mean (average), Median (middle value), Mode (most frequent value). Dispersion: Range, Standard Deviation (SD). Frequency and proportion. ○ Example: A survey reporting that the average age of respondents is 25 years. Inferential Statistics: ○ Used to make inferences about a population from a sample. ○ Tests relationships or differences between variables. ○ Example: Using a sample to predict population behavior, such as voting trends. Null Hypothesis (Ho) Definition: A statement suggesting no relationship or effect exists between variables. Logic: ○ Statistical tests aim to reject the null hypothesis if results are unlikely due to chance. ○ Example: Null hypothesis states "advertising does not affect sales," and statistical analysis seeks evidence to the contrary. P-Value Definition: The probability that the observed results occurred by chance. Usage: ○ If p < 0.05, reject the null hypothesis (statistically significant). ○ If p > 0.05, fail to reject the null hypothesis (not statistically significant). Example: A p-value of 0.03 means a 3% probability the results happened by chance, supporting a real effect. Hypothesis Testing Variables: ○ Independent Variable (IV): The manipulated or categorized factor (e.g., gender, age). ○ Dependent Variable (DV): The measured outcome (e.g., test scores, attitudes). Identifying Variables: ○ RQ: "Is there a difference in TV viewing hours between males and females?" IV: Gender (categorical), DV: Hours of TV (continuous). Statistical Tests Types: ○ Parametric: Require normal distribution (e.g., t-test, ANOVA). ○ Nonparametric: No normality required (e.g., Chi-square). When to Use: ○ T-test: Comparing means between two groups (e.g., males vs. females). ○ Chi-square: Analyzing differences among categorical variables. ○ Correlation: Examining the relationship strength and direction between two continuous variables. ○ Regression: Predicting one variable using another. ○ ANOVA: Comparing means across more than two groups. Interpretation: ○ A t-test result with p < 0.05 indicates a significant difference between groups. Errors in Hypothesis Testing Type I Error (False Positive): ○ Rejecting a true null hypothesis. ○ Example: Concluding a drug works when it doesn’t. Type II Error (False Negative): ○ Failing to reject a false null hypothesis. ○ Example: Concluding a drug doesn’t work when it actually does. Cronbach’s Alpha Definition: Measures internal consistency (reliability) of a scale. Interpretation: ○ Values range from 0 to 1: 0.7: Acceptable. 0.8: Good. 0.9: Excellent (but >0.95 suggests redundancy). Example: A survey measuring "customer satisfaction" with an alpha of 0.85 is reliable. This section summarizes the necessary details for statistical analysis, with examples to help understand concepts like p-value interpretation, hypothesis testing, and statistical tests. Let me know if you’d like practice examples! ○ 3. Content Analysis Definition and Characteristics Definition (Berelson): A research technique for the objective, systematic, and quantitative description of manifest content of communication. ○ Objective: Clear, defined categories ensure different coders reach the same results. ○ Systematic: Procedures are consistently applied to all content. ○ Quantitative: Focuses on numerical representation (e.g., frequencies). ○ Manifest Content: Analyzes explicit content as it appears (not implied meaning). Objectives and Purpose 1. Primary Purpose: Describe content. 2. Infer Gatekeeping: Understand who controls content decisions. 3. Infer Effects: Limited to hypothesizing potential impacts. 4. Test Hypotheses: Explore relationships between variables (e.g., agenda-setting theory). Sampling Issues Sampling mirrors survey techniques but adapts to the research purpose: ○ Probability Samples: Generalizable. ○ Non-probability Samples: Focused on specific contexts (e.g., analyzing news coverage of a single event). ○ Common decisions include: Timeframe (e.g., one week after an event). Type of media (e.g., Fortune 500 companies on Facebook). Unit of Analysis The smallest element studied in the content, such as: ○ News stories, tweets, sentences, ads, or paragraphs. Not to be confused with sampling unit. Often measured by frequencies (e.g., number of sources cited in a news story). Characteristics of Content Analysis Measures Measures typically focus on: 1. Manifest Content: Length, placement, number of sources. 2. Trends Over Time: Changes in coverage or framing. 3. Framing: Attributes or perspectives assigned to issues. 4. Visuals: Gender or minority representations. Steps of Content Analysis Measurement 1. Develop research hypotheses or questions. 2. Review existing literature for prior measures or insights. 3. Adapt or create new measures if needed. 4. Develop clear coding instructions and a codebook. 5. Prepare coding sheets for data entry. 6. Train coders and establish intercoder reliability. Ensuring Reliability in Content Analysis Types of Reliability: ○ Intracoder Reliability: Consistency of one coder over time. ○ Intercoder Reliability: Agreement among multiple coders. Improvement Methods: ○ Multiple coder training sessions. ○ Test with at least 20% of the sample before full analysis. Testing Methods: ○ Krippendorff’s Alpha: Above 0.7 is acceptable. ○ Cohen’s Kappa, Holsti’s Percentage Agreement, Scott’s Pi: Other measures of reliability. 4. Experimental Research Definition and Characteristics Definition: Experimental research determines causality by manipulating independent variables (IVs) and measuring their effects on dependent variables (DVs) while controlling for confounds. When Appropriate: When establishing cause-and-effect relationships (e.g., the effect of ads on purchase behavior). Key Tasks: ○ Manipulate the IV to ensure cause precedes effect. ○ Control for Confounds to eliminate alternative explanations. Experimental Validity Internal Validity: ○ Determines if the IV is the sole cause of the DV. ○ Threats include: Selection Bias: Unequal groups. History: Events outside the study affect results. Testing Effects: Pretests influence outcomes. Maturation: Natural changes over time. Solution: Randomization and control groups. External Validity: ○ The extent to which results can be generalized to other populations or settings. ○ Threats: Artificiality of lab settings. Limited sample diversity. Basic Experimental Designs 1. Posttest-Only with Control Group: ○ Compare experimental and control groups after treatment. ○ Limitation: No pretest comparison. 2. Pretest-Posttest with Control Group: ○ Measure both groups before and after treatment. ○ Limitation: Pretests may influence responses. 3. Solomon Four-Group Design: ○ Combines pretest-posttest and posttest-only designs to control pretest effects. 4. Factorial Design: ○ Examines multiple IVs simultaneously (e.g., 2x2 design for two IVs with two levels each). Controlling for Confounds Randomization: Randomly assign participants to groups. Matching: Measure and statistically control for confounding variables. Manipulation Checks: Ensure IV manipulation worked as intended. Use of Multiple Stimuli: Reduces influence of specific stimuli. Two Types of Experimental Research 1. Lab Experiments: ○ Conducted in controlled environments. ○ Strength: High internal validity. ○ Weakness: Low external validity. 2. Field Experiments: ○ Conducted in natural settings. ○ Strength: High external validity. ○ Weakness: Low internal validity. Strengths and Weaknesses Strengths: ○ Evidence of causality. ○ High control over variables. ○ Cost-effective and replicable. Weaknesses: ○ Artificiality of lab settings. ○ Generalizability challenges (low external validity). 5. Research Essentials Where Essentials Belong in a Research Paper: 1. Introduction: Define the research problem and rationale. State research questions (RQs) or hypotheses (H). 2. Literature Review: Present relevant theories and prior research. Establish the study’s foundation. 3. Methods: Describe the study design and sample. Define variables (IVs, DVs) and explain measurement. Detail experimental procedures and controls. 4. Results: Report findings (e.g., statistical tests, p-values). 5. Discussion/Conclusion: Interpret results, address limitations, and propose implications. Example Interpretations Chi-square Test: Use Chi-square for categorical variables. Interpret if differences between groups are statistically significant (p < 0.05). Correlations: Positive values = positive relationship; negative values = inverse relationship. Close to ±1 indicates strong relationships. t-test: Check means between two groups. Significant differences are indicated by p < 0.05. One-way ANOVA: Compares means across multiple groups. A significant F-value indicates at least one group differs. Post hoc tests identify specific differences.