Elementary Statistics Lecture Notes PDF - Winter 2024
Document Details
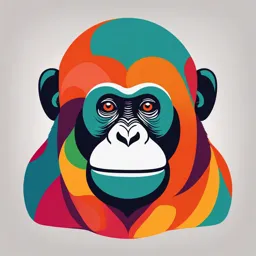
Uploaded by ConstructiveVerdelite2436
2024
Tags
Summary
These lecture notes cover the fundamentals of elementary statistics, focusing on key concepts like sample, population and census. The notes also highlight different classifications of data types like qualitative and quantitative data. The material will be useful to those learning about the science of data analysis.
Full Transcript
Elementary Statistics WINTER 2024 Copyright © 2011 Pearson Education Canada Inc.Canada 1.1 - 1 Copyright © 2010, 2007, 2004 Pearson Education, Inc. 1.1 - 2 Chapter 1 Introduction to Statistics...
Elementary Statistics WINTER 2024 Copyright © 2011 Pearson Education Canada Inc.Canada 1.1 - 1 Copyright © 2010, 2007, 2004 Pearson Education, Inc. 1.1 - 2 Chapter 1 Introduction to Statistics 1.1 - 3 Preview In this context, the terms sample and population have special meaning. Formal definitions for these and other basic terms will be given here. In this section we will look at some of the ways to describe data. 1.1 - 4 Data Data collections of observations (such as measurements, genders, survey responses) 1.1 - 5 Statistics Statistics is the science of planning studies and experiments, obtaining data, and then organizing, summarizing, presenting, analyzing, interpreting, and drawing conclusions based on the data 1.1 - 6 Population Population the complete collection of all individuals (scores, people, measurements, and so on) to be studied; the collection is complete in the sense that it includes all of the individuals to be studied 1.1 - 7 Census versus Sample Census Collection of data from every member of a population Sample Subcollection of members selected from a population 1.1 - 8 Copyright © 2010, 2007, 2004 Pearson Education, Inc. 1.1 - 9 Copyright © 2010, 2007, 2004 Pearson Education, Inc. 1.1 - 10 Key Concept Whether conducting statistical analysis of data that we have collected, or analyzing a statistical analysis done by someone else, we should not rely on blind acceptance of mathematical calculation. We should consider these factors: Copyright © 2010, 2007, 2004 Pearson Education, Inc. 1.1 - 11 Key Concept (continued) Context of the data Source of the data Sampling method Conclusions Practical implications Copyright © 2010, 2007, 2004 Pearson Education, Inc. 1.1 - 12 Context What do the values represent? Where did the data come from? Why were they collected? An understanding of the context will directly affect the statistical procedure used. 1.1 - 13 Source of data Is the source objective? Is the source biased? Is there some incentive to distort or spin results to support some self-serving position? Is there something to gain or lose by distorting results? Be vigilant and skeptical of studies from sources that may be biased. Copyright © 2010, 2007, 2004 Pearson Education, Inc. 1.1 - 14 Sampling Method Does the method chosen greatly influence the validity of the conclusion? Voluntary response (or self-selected) samples often have bias (those with special interest are more likely to participate). These samples’ results are not necessarily valid. Other methods are more likely to produce good results. Copyright © 2010, 2007, 2004 Pearson Education, Inc. 1.1 - 15 Conclusions Make statements that are clear to those without an understanding of statistics and its terminology. Avoid making statements not justified by the statistical analysis. Copyright © 2010, 2007, 2004 Pearson Education, Inc. 1.1 - 16 Practical Implications State practical implications of the results. There may exist some statistical significance yet there may be NO practical significance. Common sense might suggest that the finding does not make enough of a difference to justify its use or to be practical. Copyright © 2010, 2007, 2004 Pearson Education, Inc. 1.1 - 17 Copyright © 2010, 2007, 2004 Pearson Education, Inc. 1.1 - 18 Key Concept The subject of statistics is largely about using sample data to make inferences (or generalizations) about an entire population. It is essential to know and understand the definitions that follow. 1.1 - 19 Parameter Parameter a numerical measurement describing some characteristic of a population. population parameter 1.1 - 20 Statistic Statistic a numerical measurement describing some characteristic of a sample. sample statistic 1.1 - 21 1.1 - 22 1.1 - 23 1.1 - 24 1.1 - 25 1.1 - 26 1.1 - 27 Quantitative Data Quantitative (or numerical) data consists of numbers representing counts or measurements. Example: The weights of supermodels Example: The ages of respondents 1.1 - 28 Qualitative Data Qualitative (Categorical or attribute) data consists of names or labels (representing categories) Example: The genders (male/female) of professional athletes Example: Shirt numbers on professional athletes uniforms - substitutes for names. 1.1 - 29 Copyright © 2010, 2007, 2004 Pearson Education, Inc. 1.1 - 30 1.1 - 31 1.1 - 32 1.1 - 33 Working with Quantitative Data Quantitative data can further be described by distinguishing between discrete and continuous types. 1.1 - 34 Discrete Data Discrete data result when the number of possible values is either a finite number or a ‘countable’ number (i.e. the number of possible values is 0, 1, 2, 3,...) Example: The number of eggs that a hen lays 1.1 - 35 Continuous Data Continuous (numerical) data result from infinitely many possible values that correspond to some continuous scale that covers a range of values without gaps, interruptions, or jumps Example: The amount of milk that a cow produces; e.g. 2.343115 gallons per day 1.1 - 36 Copyright © 2010, 2007, 2004 Pearson Education, Inc. 1.1 - 37 1.1 - 38 1.1 - 39 Levels of Measurement Another way to classify data is to use levels of measurement. Four of these levels are discussed in the following slides. 1.1 - 40 Learning Objectives Understand... The distinction between measuring objects, properties, and indicants of properties. The similarities and differences between the four scale types used in measurement and when each is used. The four major sources of measurement error. The criteria for evaluating good measurement. 1.1 - 41 Types of Scales Nominal Ordinal interval Ratio 1.1 - 42 Levels of Measurement Classification Nominal Ordinal interval Ratio 1.1 - 43 Nominal Scales Mutually exclusive and collectively exhaustive categories Exhibits the classification characteristic only 1.1 - 44 Nominal Level Nominal level of measurement characterized by data that consist of names, labels, or categories only, and the data cannot be arranged in an ordering scheme (such as low to high) Example: Survey responses yes, no, undecided 1.1 - 45 Copyright © 2010, 2007, 2004 Pearson Education, Inc. 1.1 - 46 Four people ran in the race We know their names! Earl Greg Mike Matt 1.1 - 47 Levels of Measurement Classification Nominal Classification Ordinal Order interval Ratio 1.1 - 48 Ordinal Scales Characteristics of nominal scale plus an indication of order Implies statement of greater than and less than 1.1 - 49 Ordinal Level Ordinal level of measurement involves data that can be arranged in some order, but differences between data values either cannot be determined or are meaningless Example: Course grades A, B, C, D, or F 1.1 - 50 Four people ran in the race 1 We know the order they finished in 2 3 4 Earl Greg Mike Matt 1.1 - 51 Levels of Measurement Classification Nominal Classification Ordinal Order Classification Distance interval Order Ratio 1.1 - 52 Interval Scales Characteristics of nominal and ordinal scales plus the concept of equality of interval. Equal distance exists between numbers 1.1 - 53 Interval Level Interval level of measurement like the ordinal level, with the additional property that the difference between any two data values is meaningful, however, there is no natural zero starting point (where none of the quantity is present) Example: Years 1000, 2000, 1776, and 1492 1.1 - 54 Copyright © 2010, 2007, 2004 Pearson Education, Inc. 1.1 - 55 Four people ran in the race 3:15pm We know how far apart they finished, but when did they START? 3:21pm 3:22pm 4:19pm Earl Greg Mike Matt 1.1 - 56 Four people ran in the race 3:15pm We know how far apart they finished, but when did they START? 3:21pm 3:22pm 4:19pm Earl is 1:04 faster than Matt Earl Greg Mike Matt 1.1 - 57 Levels of Measurement Classification Nominal Classification Ordinal Order Classification Distance interval Order Classification Distance Ratio Order Natural Origin 1.1 - 58 Ratio Scales Characteristics of previous scales plus an absolute zero point Examples – Weight – Height – Number of children 1.1 - 59 Ratio Level Ratio level of measurement the interval level with the additional property that there is also a natural zero starting point (where zero indicates that none of the quantity is present); for values at this level, differences and ratios are meaningful Example: Prices of college textbooks ($0 represents no cost, a $100 book costs twice as much as a $50 book) 1.1 - 60 Copyright © 2010, 2007, 2004 Pearson Education, Inc. 1.1 - 61 Four people ran in the race 1:45 The race started at 1:30 P.M. 1:51 1:52 2:49 Earl Greg Mike Matt 1.1 - 62 Four people ran in the race 1:45 The race started at 1:30 P.M. 1:51 1:52 2:49 Earl is 58.23% faster than Matt Earl Greg Mike Matt 1.1 - 63 Copyright © 2010, 2007, 2004 Pearson Education, Inc. 1.1 - 64 1.1 - 65 1.1 - 66 Summary - Levels of Measurement Nominal - categories only Ordinal - categories with some order Interval - differences but no natural starting point Ratio - differences and a natural starting point 1.1 - 67