STAT 101 - Week 1 Lecture Notes PDF
Document Details
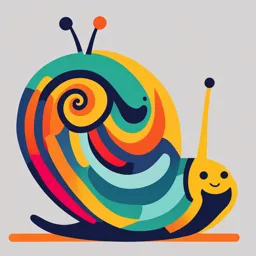
Uploaded by ConsummateWonder8677
Tags
Summary
These STAT 101 lecture notes cover the basics of statistics, including descriptive and inferential statistics. The document reviews data collection methods, basic terminology, and sampling techniques. The notes contain an overview of the main concepts. The lectures cover important topics, such as data collection methods, sources of data (primary and secondary), and the different levels of measurement.
Full Transcript
STAT 101 – Week 1 Lecture Objectives: Upon completion of this lecture, students should be able to: Understand the Basics of Statistics: Define statistics. Differentiate between descriptive and inferential statistics. Identify Sources and Methods of Data Collection: Distinguish between primary a...
STAT 101 – Week 1 Lecture Objectives: Upon completion of this lecture, students should be able to: Understand the Basics of Statistics: Define statistics. Differentiate between descriptive and inferential statistics. Identify Sources and Methods of Data Collection: Distinguish between primary and secondary data, including their advantages and disadvantages. Describe various data collection methods and their advantages and disadvantages. Classify and Distinguish Types of Data: Differentiate between categorical and quantitative data. Differentiate and Identify discrete and continuous data within quantitative data types. Apply Levels of Measurement in Data Analysis: Explain the four levels of measurement: nominal, ordinal, interval, and ratio. Determine the appropriate statistical/mathematical operations for each level of measurement. Understand Fundamental Statistical Terminology: Define key statistical concepts such as population, sample, parameter, statistic, variables, observations, and measurements. Demonstrate Knowledge of Sampling Techniques: Explain and apply different sampling techniques, including random, stratified, cluster, and systematic sampling. Give the advantages and disadvantages of each sampling method. What is Statistics? It is the Collection, Organizing, Analyzing, and Interpretation Of data to make informed decisions. Statistical Terminology Consider the following dataset to understand some fundamental statistical terminology. Participant Gender Educational Age Weight Heig Body ID Level ht Mass Index 1 Male High School 22 138 63.00 24.4 2 Male Primary 27 183 69.75 26.4 3 Female Primary 35 153 65.75 24.9 4 Female Graduate 19 178 70.00 25.5 5 Female Graduate 20 161 70.50 22.8 6 Male Graduate 25 206 70.00 29.6 7 Female High School 32 235 72.00 31.9 8 Male High School 30 151 60.75 28.8 9 Male Primary 22 213 69.00 31.5 10 Female Graduate 24 142 61.00 26.8 Statistical Terminology Population: The entire group you want to study. Sample: A smaller, manageable subset of the population. Parameter: The true value you want to estimate (population-level). Statistic: The value you calculate from your sample to estimate the parameter. 6 Variables: The characteristics you measure (e.g., Gender, Age). Observations: Individual cases or rows in your dataset. Measurements: The recorded values for each variable in your observations. 7 Two Main Areas/Branches of Statistics: Descriptive Statistics: Summarizes and describes the characteristics of a dataset. Inferential Statistics: Makes predictions or inferences about a population based on a sample. An automobile dealer sells: Example: 3 automobiles on Mon 4 automobiles on Tues 5 automobiles on Wed 6 automobiles on Thur 7 automobiles on Fri The average number of automobiles sold per day during this period was: 3+4+5+6+7 = 5, 5 automobiles per day. Is this inferential or descriptive statistics? Sampling Techniques Random Sampling: Every member of the population has an equal chance of being selected. Example: Drawing names from a hat. Advantages: Reduces bias, ensures representativeness. Disadvantages: May require a complete list of the population. 10 Stratified Sampling: The population is divided into subgroups (strata) based on specific characteristics, and samples are taken from each subgroup. Example: Dividing a population into age groups and sampling from each group. Advantages: Ensures representation of all subgroups. Disadvantages: Requires knowledge of population characteristics. 11 Cluster Sampling: The population is divided into clusters, and entire clusters are randomly selected for study. Example: Dividing a city into wards and randomly selecting a few wards to survey. Advantages: Easier and cheaper for large populations. Disadvantages: May introduce bias if clusters are not representative. 12 Systematic Sampling: Selecting every k-th member of the population from a list. Example: Surveying every 2nd person on the list. Advantages: Simple and easy to implement. Disadvantages: Can introduce bias if there’s a hidden pattern in the population. 13 SOURCES OF DATA: PRIMARY DATA Data can be categorized as either primary or secondary. Primary Data is collected directly by the researcher for a specific purpose or study. Examples: ✓ Surveys conducted by a researcher. ✓ Experimental results obtained in a controlled environment. ✓ Observations recorded in real-time. 14 SOURCES OF DATA: PRIMARY DATA Advantages: Tailored to the specific research question. More reliable for the intended purpose since the researcher controls the data collection process. Disadvantages: Time-consuming and costly to collect. Requires careful planning and resources. 15 SOURCES OF DATA: SECONDARY DATA Secondary Data is data that has been collected and published by someone else for a different purpose and reused for the current study with a different purpose. Examples: ✓ Government and Official Reports (e.g., census data). ✓ Research articles or databases. ✓ Industry reports or publicly available datasets. 16 SOURCES OF DATA: SECONDARY DATA Advantages: Easily accessible and cost-effective. Saves time as the data is already collected and often pre-processed. Disadvantages: May not fit the specific needs of the study. Data quality or accuracy might not be guaranteed. Potential for bias if the original source had limitations 17 KEY DISTINCTION BETWEEN PRIMARY AND SECONDARY DATA The classification as primary or secondary data depends on: I. Control over Data Collection: Did you directly influence the data collection process? II. Original Purpose: Was the data collected explicitly for your study, even if by someone else? If the answer to both questions is yes, the data can be viewed as primary. If not, it is secondary. 18 DATA COLLECTION METHODS The techniques or approaches used to gather data for analysis: Surveys and Questionnaires: Structured tools used to collect data from respondents. Examples: Online surveys, in-person interviews. Advantages: ▪ Can gather a large volume of data quickly. ▪ Flexible and customizable. Disadvantages: Risk of non-response or bias. Quality depends on question design. 19 Experiments: Controlled studies where variables are manipulated to observe effects. Examples: Testing a new drug, measuring the effect of teaching methods on student performance. Advantages: ▪ High control over variables. ▪ Establishes cause-and-effect relationships. Disadvantages: ▪ Time-consuming and costly. ▪ May not reflect real-world scenarios. 20 Observational Studies: Data collected by observing subjects without interference. Examples: Monitoring wildlife, observing customer behavior in a store. Advantages: ▪ Natural and realistic data. ▪ Useful when experiments are not feasible. Disadvantages: ▪ Cannot establish causation. ▪ Observer bias may affect results. 21 Interviews and Focus Groups: Interactive methods to gather detailed, qualitative data. Examples: Face-to-face interviews, group discussions. Advantages: ▪ Provides deep insights. ▪ Allows for clarification of responses. Disadvantages: ▪ Time-intensive. ▪ Risk of interviewer bias. 22 Secondary Data Collection: Reusing data collected by others for new research. Examples: Government reports, research articles, organizational records. Advantages: ▪ Time-saving and cost-effective. ▪ Large datasets often available. Disadvantages: ▪ Limited control over data quality. ▪ Data may not perfectly fit the research question. 23 Sensors and Automated Systems: Using technology to collect continuous or real-time data. Examples: Weather stations, IoT devices, traffic cameras. Advantages: ▪ Accurate and consistent data collection. ▪ Suitable for large-scale or high-frequency data. Disadvantages: ▪ High initial setup cost. ▪ Technical malfunctions may lead to data loss. 24 Practical Considerations in Data Collection Ethical Concerns: Ensure confidentiality, consent, and proper use of data. Sampling: Choosing the right sampling method (random, stratified, cluster, etc.). Bias: Avoid biases in data collection methods, such as selection bias or response bias. Validation: Ensuring that the method reliably measures what it intends to measure. 25 Types of Data Qualitative/Categorical data is non-numeric data represented by categories or labels. Quantitative data consists of numerical values and can be further classified into discrete and continuous types. Discrete data is countable (e.g. Number of students in a class, Number of cars in a parking lot.) Continuous data is measurable (e.g. Height, Weight) Types of data Consider the following dataset to understand the types of data. Participant Gender Educational Age Weight Heig Body ID Level ht Mass Index 1 Male High School 22 138 63.00 24.4 2 Male Primary 27 183 69.75 26.4 3 Female Primary 35 153 65.75 24.9 4 Female Graduate 19 178 70.00 25.5 5 Female Graduate 20 161 70.50 22.8 6 Male Graduate 25 206 70.00 29.6 7 Female High School 32 235 72.00 31.9 8 Male High School 30 151 60.75 28.8 9 Male Primary 22 213 69.00 31.5 10 Female Graduate 24 142 61.00 26.8 Level of Measurement Level of measurement refers to the classification of data based on ✓the type of information it represents and ✓the mathematical operations that can be meaningfully performed on it. This is to determine the: ✓type of statistical analysis you can perform. ✓kinds of visualizations you can create. Nominal Level Data is categorized into distinct groups or labels without any inherent order. Characteristics: ▪ Categories are mutually exclusive (each item belongs to one group). ▪ No ranking or ordering of categories. Examples: ▪ Gender (Male, Female, Other). ▪ Hair color (Black, Brown, Blonde). ▪ Types of fruit (Apple, Banana, Orange). Mathematical Operations and Statistical Analysis: ▪ Only counting (frequencies) and mode can be determined. Ordinal Level Data is categorized into groups with a meaningful order, but the differences between categories are not uniform or meaningful. Characteristics: ▪ Categories can be ranked or ordered. ▪ The distance between categories is not measurable. Examples: ▪ Education level (High School, Bachelor’s, Master’s, PhD). ▪ Satisfaction ratings (Very Unsatisfied, Unsatisfied, Neutral, Satisfied, Very Satisfied). ▪ Race rankings (1st, 2nd, 3rd). Mathematical Operations and Statistical Analysis : ▪ Counting, mode, and median can be determined. ▪ Differences or averages are not meaningful. Interval Level Data is measured on a scale where the differences between values are meaningful, but there is no true zero. Characteristics: ▪ Equal intervals between values. ▪ No absolute zero (zero does not mean “none”). Examples: ▪ Temperature in Celsius or Fahrenheit (0℃ ≠ "no temperature"). ▪ Time of day (e.g., 2 PM, 4 PM). ▪ Calendar years (e.g., 1990, 2000, 2010). Mathematical Operations and Statistical Analysis : Addition and subtraction are valid. Mean and standard deviation can be calculated. Ratios (e.g., twice as much) are not meaningful. Ratio Level Data is measured on a scale with a true zero, allowing for meaningful comparisons of both differences and ratios. Characteristics: ▪ Equal intervals between values. ▪ True zero exists (zero means "none" or "absence"). Examples: ▪ Income (e.g., $0 means no income). ▪ Distance (e.g., 0 meters means no distance traveled). ▪ Store inventory (e.g., 0 number of items means there is nothing in the store ) Mathematical Operations and Statistical Analysis : ▪ Addition, subtraction, multiplication, and division are valid. ▪ Ratios are meaningful (e.g., 20 kg is twice as heavy as 10 kg). ▪ Mean, median, mode, variance, etc. can be calculated CLASS EXERCISE Are the following data nominal, ordinal, interval, or ratio data? i. The number of White cats that are in a cage containing cats in a vet’s office. ii. The numbers on the jerseys of football players. iii. Numbered musical exercises in a book of musical exercises. iv. The length and width of an official United States flag. v. Presidential election years in the United States. vi. Checking account numbers.