STA111 Descriptive Statistics Lecture Notes PDF
Document Details
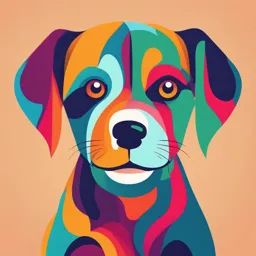
Uploaded by FruitfulMesa
Kwara State University
Tags
Summary
These lecture notes provide an introduction to descriptive statistics, specifically focusing on measures of location, such as averages. It covers calculating and interpreting the arithmetic mean, using examples and methods for both ungrouped and grouped data. The notes also touches upon the concept of a good average.
Full Transcript
STA111: Descriptive Statistics Topic: MEASURES OF LOCATION Once you have collected data, what will you do with it? There are many situations that require that the data be summarized by means of a few descriptive and qualitative measures. For example, we may wi...
STA111: Descriptive Statistics Topic: MEASURES OF LOCATION Once you have collected data, what will you do with it? There are many situations that require that the data be summarized by means of a few descriptive and qualitative measures. For example, we may wish to find a number that describes the average number of days that will take a variety of maize to mature or the average number of eggs that a particular breed of chicken on a particular diet will produce per week. We also often need to find methods of extracting the most important bits of information so that we can use them to describe a set of data or compare different data sets. There are many ways to describe data but not all of them are appropriate for a given sample. In deciding what to use to describe the data needs to consider the type(s) of variables measured – whether they are unordered or ordered categories, counts or measurements. Counts and measurements are quantitative and it is possible to do some arithmetic on their values. In this chapter, you will study numerical ways to describe your data. This area of statistics is called "Descriptive Statistics". You will learn to calculate, and even more importantly, to interpret these measurements. AVERAGES One of the important objectives of statistical analysis is to determine various numerical measures which describe the inherent characteristics of a frequency distribution. The first of such measures is average. The averages are measures which condense a huge unwieldy set of numerical data into single numerical values which are representative of the entire distribution. In the words of Prof. R.A. Fisher: “The inherent inability of the human mind to grasp in its entirety a large body of numerical data compels us to seek relatively few constants that will adequately describe the data”. Averages are one of such few constants. It provides us the gist and gives a bird’s eye view of the huge mass of unwieldy numerical data. Averages are also sometimes referred to measures of location since they enable us to locate the position or place the distribution in question and if the average tends to lie or indicating the centre of the distribution is called measure of central tendency. Averages are very much useful: For describing the distribution in concise manner For comparative study of different distributions For computing various other statistical measures such as dispersion, skewness, and various other basic characteristics of a mass of data. DESIRABLE QUALITIES OF A GOOD AVERAGE Some of the qualities (characteristics) of an average are: It should be easy to calculate and simple to understand It should be clearly defined by a mathematical formula It should not be affected by extreme values It should be based on all the observations It should be capable of further mathematical treatment It should have sample stability ARITHMETIC MEAN 1|Page STA 111 It is the most commonly used average or measure of the central tendency applicable only in case of quantitative data. Arithmetic mean is also simply called “mean”. Arithmetic mean is defined as: “quotient of sum of the given values and number of the given values”. The arithmetic mean can be computed for both ungroup data (raw data: a data without any statistical treatment) and grouped data (a data arranged in tabular form containing different groups). If X is the involved variable, then arithmetic mean of X is abbreviated as A.M of X and denoted by X. The arithmetic mean of X can be computed by any of the following methods. X= x : Ungrouped data and X = fx : Grouped data n f Where x : Indicates values of the variable X n : Indicates number of values of X f : Indicates frequency of different groups : Summation or addition. EXAMPLE 1: The number of calls received in a day by five selected KWASU students is recorded as follows: 10, 5, 15, 8 and 12. Calculate arithmetic mean of the following data. Solution: Let train fare is indicated by x , then the Arithmetic mean of X is X = x , if we decide to use n the above-mentioned formula. From the given data, we have x = 50 and n = 5. Placing these two 50 quantities in above formula, we get the arithmetic mean for given data X = = 10 5 Many times, our data appear in frequency tables where we no longer know the actual values of the observations, but only to which class interval they belong. In these instances, the best we can do is to approximate the sample mean. The mean is given by the expression EXAMPLE 2: The following frequency distribution presents the ages of secondary school students of a particular college. Age (Years) 13 14 15 16 17 Number of Students 2 5 13 7 3 Solution: The given distribution belongs to a grouped data and the variable involved is ages of secondary school students. While the number of students Represent frequencies. Ages (Years) Number of Students ( f ) fx x 13 2 26 14 5 70 15 13 195 16 7 112 17 3 51 Total f = 30 fx = 454 Now we will find the Arithmetic Mean as X = fx = 454 = 15.13 years. f 30 2|Page STA 111 EXAMPLE 3: The following data shows distance covered by 100 persons to perform their routine jobs. Distance (Km) 0-10 10-20 20-30 30-40 Number of Persons 10 20 40 30 Solution: The given distribution belongs to a grouped data and the variable involved is ages of “distance covered”. While the “number of persons” Represent frequencies. Distance (Km) Number of Persons (f) Mid Points (x) fx 0-10 10 5 50 10-20 20 15 300 20-30 40 25 1000 30-40 30 35 1050 Total f = 100 fx = 2400 Now we will find the Arithmetic Mean as X = fx = 2400 = 24 Km. f 100 EXAMPLE 4: The following frequency distribution shows the marks obtained by 50 students in statistics at a certain college. Find the arithmetic mean. Marks 20-29 30-39 40-49 50-59 60-69 70-79 80-89 Frequency 1 5 12 15 9 6 2 SOLUTION: Marks f X Fx 20-29 1 24.5 24.5 30-39 5 34.5 172.5 40-49 12 44.5 534.5 50-59 15 54.5 817.5 60-69 9 64.5 580.5 70-79 6 74.5 447.5 80-89 2 84.5 169.5 Total 50 2745 X= fx = 2745 = 54.9 or 55 Marks f 50 WEIGHTED ARITHMETIC MEAN In calculation of arithmetic mean, the importance of all the items was considered to be equal. However, there may be situations in which all the items under considerations are not equal importance. For example, we 3|Page STA 111 want to find average number of marks per subject who appeared in different subjects like Mathematics, Statistics, Physics and Biology. These subjects do not have equal importance. If we find arithmetic mean by giving Mean. Thus, arithmetic mean computed by considering relative importance of each items is called weighted arithmetic mean. To give due importance to each item under consideration, we assign number called weight to each item in proportion to its relative importance. If for instance, we are interested in finding the mean of several means which are themselves obtained on different numbers of observations, then it is appropriate to weight the means or observations by using weights to depend on the number of observations in each mean. A weighted mean is therefore defined by using following formula: X w = wx w Where: X w Stands for weighted arithmetic mean x Stands for values of the items and w Stands for weight of the item EXAMPLE 5: A student obtained 40, 50, 60, 80, and 45 marks in the subjects of Math, Statistics, Physics, Chemistry and Biology respectively. Assuming weights 5, 2, 4, 3, and 1 respectively for the above mentioned subjects. Find Weighted Arithmetic Mean per subject. Solution: Marks Obtained Weight Wx Subjects (x) (w) Math 40 5 200 Statistics 50 2 100 Physics 60 4 240 Chemistry 80 3 240 Biology 45 1 45 Total wx = 15 wx = 825 Now we will find weighted arithmetic mean as: Xw = wx = 825 = 55 marks/subject. w 15 MERITS AND DEMERITS OF ARITHMETIC MEAN Merits: It is rigidly defined It is easy to calculate and simple to follow It is based on all the observations It is determined for almost every kind of data It is finite and not indefinite It is readily put to algebraic treatment It is least affected by fluctuations of sampling. Demerits: The arithmetic mean is highly affected by extreme values It cannot average the ratios and percentages properly It is not an appropriate average for highly skewed distributions 4|Page STA 111 It cannot be computed accurately if any item is missing The mean sometimes does not coincide with any of the observed value. GEOMETRIC MEAN It is another measure of central tendency based on mathematical footing like arithmetic mean. Geometric mean can be defined in the following terms: Geometric mean is the nth positive root of the product of “n” positive given values. Hence, geometric mean for a value X containing n values such as x1 , x2 ,..., xn is denoted by X g and is as given below: X g = n x1 x 2 ... x n (For Ungrouped Data) If we have a series of n positive values with repeated values such as x1 , x2 ,..., xn are repeated f1 , f 2 ,..., f n times respectively then geometric mean will becomes: X g = n x1 1 x 2 2 ... x n Where n = f1 + f 2 +... + f n f f fn (For Grouped Data) EXAMPLE 6: Find the Geometric Mean of the values 10, 5, 15, 8, 12 Solution: Here x1 = 10 , x2 = 5 , x3 = 15 , x4 = 8 , x 5 = 12 and n = 5 X g = 5 10 5 15 8 12 5 72000 = 9.36 Example 7:Find the Geometric Mean for the following data X 13 14 15 16 17 f 2 5 13 7 3 Solution: We may write it as given below: Here x1 = 13 , x2 = 14 , x3 = 15 , x4 = 16 , x 5 = 17 , f1 = 2 , f 2 = 5 , f 3 = 13 , f 4 = 7 , f 5 = 3 n = f = 30 Using the formula of geometric mean for grouped data, geometric mean in this case will become: X g = n x1 1 x 2 2 ... x n f f fn X g = 30 13 2 14 5 15 13 16 7 17 3 30 2.33292 10 35 15.10 The method explained above for the calculation of geometric mean is useful when the numbers of values in given data are small in number and the facility of electronic calculator is available. When a set of data contains large number of values then we need an alternative way for computing geometric mean. The modified or alternative way of computing geometric mean is given as follow: For Ungrouped Data For Grouped Data log x f log x X g = Anti log X g = Anti log n f Example8 : Find the Geometric Mean of the values 10, 5, 15, 8, 12 X Log x 5|Page STA 111 10 1.0000 log x X g = Anti log n 5 0.6990 4.8573 15 1.1761 X g = Anti log 5 8 0.9031 X g = Anti log (0.9715 ) = 9.36 12 1.0792 Total log x = 4.8573 Example 9: Find the Geometric Mean for the following distribution of students’ marks: Marks 0-30 30-50 50-80 80-100 No. of Students 20 30 40 10 Solution: Marks No. of Students (f) Mid Points (x) f log x 0-30 20 15 23.5218 30-50 30 40 48.0186 50-80 40 65 72.5165 80-100 10 90 19.5424 Total f = 100 f log x = 163.6425 f log x X g = Anti log = Anti log 163.6425 = Anti log (1.6364) = 43.29 f 100 PROPERTIES OF GEOMETRIC MEAN The main properties of geometric mean are: The geometric mean is less than arithmetic mean, G.M