SPSS User-Friendly Approach for Version 22 PDF
Document Details
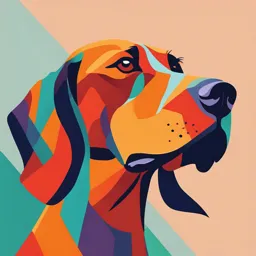
Uploaded by HonoredVampire
Harvard University
2015
Jeffery E. Aspelmeier, Thomas W. Pierce
Tags
Summary
This is a textbook on using SPSS (Statistical Package for the Social Sciences) version 22 for data analysis. It provides a user-friendly approach, covering various statistical techniques like t-tests, ANOVAs, regression, and correlation. The book is aimed at students and researchers in social sciences and related fields.
Full Transcript
SPSS A User-Friendly Approach for Version 22 Jeffery E. Aspelmeier Thomas W. Pierce Radford University Radford University Publisher: Rachel Losh Senior Acquisitions Editor: Daniel DeBonis Editorial Assistant: Katie Pachnos Marketing Manager: Lindsay Johnson Director, Content Management...
SPSS A User-Friendly Approach for Version 22 Jeffery E. Aspelmeier Thomas W. Pierce Radford University Radford University Publisher: Rachel Losh Senior Acquisitions Editor: Daniel DeBonis Editorial Assistant: Katie Pachnos Marketing Manager: Lindsay Johnson Director, Content Management Enhancement: Tracey Kuehn Managing Editor: Lisa Kinne Project Editor: Julio Espin Production Manager: Stacey Alexander Photo Editor: Jennifer Atkins Art Director: Diana Blume Cover Designer: Vicki Tomaselli Art Manager: Matthew McAdams Composition: Linda Harms Printing and Binding: RR Donnelley Library of Congress Control Number: 2014922829 ISBN-10: 1-319-01687-1 ISBN-13: 978-1-319-01687-6 © 2015, 2011, 2009 by Worth Publishers All rights reserved. Printed in the United States of America First printing Worth Publishers 41 Madison Avenue New York, NY 10010 www.macmillanhighered.com About the Authors Jeff Aspelmeier is currently a professor in the Department of Psychology at Radford University, where he has been teaching since 1999. He earned his B.S.Ed. in Secondary Education from Southwest Missouri State University, and his M.A. and Ph.D. in Social Psychology from Kent State University. His research interests focus on adult attachment and social cognition. When not writing statistics textbooks for fun, he likes to travel and ski with his wife Kim, canoe with his dog Cassidy, backpack on the Appalachian Trail with friends, and play banjo with anyone who will tolerate it (which typically does not include Kim or Cassidy). Tom Pierce is a professor in the Department of Psychology at Radford University. He has been teaching statistics and research methods since coming to Radford in 1992. He has a B.A. in Psychology from McGill University and a Ph.D. in Psychology from the University of Maine. He was also a postdoctoral fellow in the Center for the Study of Aging at Duke University Medical Center. His research interests are in the areas of aging and cognitive function, stress and human performance, and time series analysis of behav- ioral and physiological data. iii this page left intentionally blank Brief Contents About the Authors iii Preface xi CHAPTER 1 Introduction to SPSS: A User-Friendly Approach 1 CHAPTER 2 Basic Operations 11 CHAPTER 3 Finding Sums 27 CHAPTER 4 Frequency Distributions and Charts 38 CHAPTER 5 Describing Distributions 65 CHAPTER 6 Compute Statements: Reversing Scores, Combining Scores, and Creating Z-Scores 77 CHAPTER 7 Comparing Means in SPSS (t-Tests) 90 CHAPTER 8 One-Way ANOVA: Means Comparison with Two or More Groups 106 CHAPTER 9 Factorial ANOVA 117 CHAPTER 10 Repeated-Measures Analysis of Variance 137 CHAPTER 11 Regression and Correlation 164 CHAPTER 12 Multiple Regression 178 CHAPTER 13 Chi-Square 199 CHAPTER 14 Reliability 215 CHAPTER 15 Factor Analysis 230 INDEX I-1 v this page left intentionally blank Contents About the Authors iii Preface xi About this Edition xi About the User-Friendly Approach xi Acknowledgments xii CHAPTER 1 Introduction to SPSS: A User-Friendly Approach 1 Don’t Panic! 1 How to Use this Book 2 Data Analysis as a Decision-Making Process 2 Summary 8 Practice Exercises 8 CHAPTER 2 Basic Operations 11 Three Windows 11 Data Editor 12 Syntax Files 19 Output Files 22 Summary 25 Practice Exercises 26 CHAPTER 3 Finding Sums 27 Setting Up the Data 27 Running the Analysis 29 Reading the Output 31 Finding ΣX 2, ΣY 2, and Other Complex Summations 32 Find the Sums and Interpret the Output 35 More Compute Operators 36 Summary 36 Practice Exercises 37 CHAPTER 4 Frequency Distributions and Charts 38 Setting Up the Data 38 Obtaining Frequency Tables 39 Obtaining Frequency Charts 45 vii viii C O N T E N T S Obtaining Charts of Means 55 Charts of Means for Repeated Measures 58 Summary 62 Practice Exercises 62 CHAPTER 5 Describing Distributions 65 Setting Up the Data 65 Measures of Central Tendency: Mean, Median, and Mode 67 Measures of Variability: Range,Variance, and Standard Deviation 70 Measures of Normality: Skewness and Kurtosis 73 Summary 75 Practice Exercises 76 CHAPTER 6 Compute Statements: Reversing Scores, Combining Scores, and Creating Z-Scores 77 Setting Up the Data 77 Reverse Scoring Variables 78 Generating Multi-Item Scale Scores 81 Generating Z-Scores 84 Summary 88 Practice Exercises 89 CHAPTER 7 Comparing Means in SPSS (t-Tests) 90 A Brief Review of Hypothesis Testing 90 The Data 91 Setting Up the Data 92 One-Sample t-test 94 Independent-Samples t-Test 97 Paired-Samples t-Test 101 Summary 104 Practice Exercises 104 CHAPTER 8 One-Way ANOVA: Means Comparison with Two or More Groups 106 Setting Up the Data 106 Running the Analyses 109 Reading the One-Way ANOVA Output 111 Summary 116 Practice Exercises 116 CHAPTER 9 Factorial ANOVA 117 Setting Up the Data 120 Running the Analysis 122 Reading the Output for a Two-Way ANOVA 125 C O N T E N T S ix Simple Effects Testing 130 Summary 135 Practice Exercises 135 References 136 CHAPTER 10 Repeated-Measures Analysis of Variance 137 One-Way Repeated-Measures ANOVA 139 Summary 162 Practice Exercises 162 CHAPTER 11 Regression and Correlation 164 Setting Up the Data 164 Correlation 165 Simple Linear Regression 169 Obtaining the Scatterplot 172 Summary 176 Practice Exercises 176 CHAPTER 12 Multiple Regression 178 Setting Up the Data 178 Simultaneous Entry of Two Predictor Variables 181 Varying the Order of Entry: Hierarchical Multiple Regression 187 Using Automated Strategies for Selecting Predictor Variables 192 Summary 196 Practice Exercises 197 CHAPTER 13 Chi-Square 199 Setting Up the Data 199 Goodness-of-Fit Chi-Square 202 Pearson’s Chi-Square 208 Summary 213 Practice Exercises 213 References 214 CHAPTER 14 Reliability 215 Setting Up the Data 215 Test-Retest and Parallel Forms Reliability 217 Split-Half Reliability 220 Cronbach’s Alpha 224 Concluding Comments on the GSR Scale 227 Summary 228 Practice Exercises 228 x CONTENTS CHAPTER 15 Factor Analysis 230 Setting Up the Data 230 Goals of Factor Analysis 232 Running a Factor Analysis in SPSS 235 Reading the SPSS Output for Factor Analysis 239 Summary 244 Practice Exercises 244 INDEX I-1 Preface C O N T E N T S xi ABOUT THIS EDITION Since the release of the last edition of SPSS: A User-Friendly Approach for Versions 17 and 18, SPSS has gone through four new versions of their product and changed the name of the software to IBM SPSS Statistics. This edition of SPSS: A User-Friendly Approach covers the use of IBM SPSS Statistics 22. If you are using an earlier version of SPSS, you will still find this text to be quite useful, as it covers the same procedures available in ver- sions 19 through 21. We have made some substantive revisions in this edition. Some of the changes we are most proud of involve the introduction of new cartoons. With the help of graphic art- ist and longtime friend David McAdoo (creator of the Red Moon series), we created five original cartoons, which we have used to generate new examples to illustrate statistical procedures. We have also added some new elements to the text. Chapter 5 now covers charts for repeated-measures data, and Chapter 7 offers a brief introduction to hypoth- esis testing (t-tests). Sections labeled “Is the test significant?” have been added to each chapter covering hypothesis tests (t-tests, ANOVA, factorial ANOVA, mixed model ANOVA, correlation, and Chi-Square) to help students decide whether they have ob- tained significant results. Chapter 15 (factor analysis) now covers the use of scree plots in factor identification. We have also made minor improvements throughout the text. ABOUT THE USER-FRIENDLY APPROACH The user-friendly approach to statistics was developed for the average student of statis- tics—specifically, the anxious and trepidatious. My friend and colleague Steve Schacht developed this cartoon-based approach to combat the paralyzing anxiety that math and statistics seemed to induce in his students. I was introduced to this method after using Steve’s book—Statistics: A User-Friendly Approach—in the first statistics classes I taught. I have spent the past 18 years using this approach to teach statistical concepts at both the graduate and undergraduate levels. In 2005, Steve and I co-authored Social and Behavioral Statistics: A User-Friendly Approach. Unfortunately, very shortly after we started work on a new edition, Steve passed away and never saw the book released. Steve and I had always intended to develop a user-friendly guide for teaching computer-based approaches to data analysis, and our work together forms the founda- tion upon which SPSS: A User-Friendly Approach is built. The book embodies that same commitment to making statistics fun and accessible to students while helping them de- velop a sense of empowerment. While planning SPSS: A User-Friendly Approach, I invited my friend Tom Pierce to join the project. I asked Tom for his help largely because, in my opinion, he is a gifted statistics teacher, and I owe a very large portion of my understanding of statistics and xi xii P R E F A C E the teaching of statistics to him. I also asked him to play a part in this project because he shares my appreciation for absurd humor. Together Tom and I have more than 36 years of teaching experience. I think you will see that our collaboration has been quite fruitful, and, though it may be hard to believe, we had tremendous fun writing this book. Although Tom and I are both psychologists, SPSS: A User-Friendly Approach should be attractive to anyone teaching or taking research courses in the social, behavioral, or educational sciences. This book was written to be a compendium to the traditional statistics texts in undergraduate and graduate courses that use SPSS as a data analysis tool. Our intent is to teach students to conduct the statistical analyses typically found in statistics and research methods courses. We also focus on reinforcing the basic statisti- cal concepts that students encounter in these courses. This text covers basic data man- agement techniques, descriptive analyses, and hypothesis-testing approaches, along with more advanced topics like factorial designs, multiple regression, reliability analysis, and factor analysis. We have approached these topics with cartoons and humor, but our main goal is to provide substantive and thorough coverage of these procedures. Both anxious and confident students should find this text to be compelling and informative. It is our sincerest hope that you have as much fun using this book as Tom and I had writing it. Jeff Aspelmeier ACKNOWLEDGMENTS We would like to thank Lisa Underwood, who, on behalf of Steve Schacht’s estate, has allowed us to keep Steve’s work alive. We would also like to thank our editor Dan DeBonis and all the staff at Worth for their creativity and expertise, and for producing a book we are all very proud of. Thanks to Kim for tolerating and indulging an obsession with statistics and cartoons. J.A. Thanks to Ann, Bethany, and Luna. Thanks also to Tom’s parents for modeling and en- couraging curiosity as a lifelong core value. T.W.P. WORTH PUBLISHERS IS PLEASED TO OFFER SPSS: A USER-FRIENDLY APPROACH AS A SUPPLEMENT TO THE FOLLOWING STATISTICS TEXTBOOKS: Using and Interpreting Statistics: A Practical Text for the Behavioral, Social, and Health Sciences by Eric W. Corty (The Pennsylvania State University) is an engaging, easy-to-under- stand textbook that focuses on the needs of behavioral science students encountering sta- tistical practices for the first time. An award- winning master teacher, Corty speaks to stu- dents in their language, with an approachable Cover art by Ikon Images/Corbis © Macmillan Education voice that conveys the basics of statistics step- by-step. Using and Interpreting Statistics: A Practical Text for the Behavioral, Social, and Health Sciences, Second Edition ISBN-10: 1-4292-7860-9 / ISBN-13: 978- 1-4292-7860-7 Statistics for the Behavioral Sciences by Susan A. Nolan (Seton Hall University) and Thomas E. Heinzen (William Paterson University) is a uniquely effective introduction to statis- tics that captivates students with real-world storytelling, its highly visual approach to teaching, its accessible treatment of math- ematical topics, and its helpful, step-by-step worked examples. Cover art by Leslie Wayne, Cover © Macmillan Education Statistics for the Behavioral Sciences, Third Edition ISBN-10: 1-4641-0922-2 / ISBN-13: 978- 1-4641-0922-5 xiii xiv P R E F A C E Essentials of Statistics for the Behavioral Sciences by Susan A. Nolan (Seton Hall University) and Thomas E. Heinzen (William Paterson University) follows the same approach and organization as the full-length Statistics for the Behavioral Sciences. This briefer version makes the mathematics of statistical reasoning acces- sible with engaging stories, careful explana- tions, and helpful pedagogy. Essentials of Statistics for the Behavioral Sciences, Cover art by Margaret Glew, Cover © Macmillan Second Edition ISBN-10: 1-4292-4227-2 / ISBN-13: 978- 1-4292-4227-1 chapter one Introduction to SPSS: A User-Friendly Approach DON’T PANIC! Douglas Adams wrote a very funny and insightful series of novels that center around an electronic book called the Hitchhiker’s Guide to the Galaxy. The Guide contains all relevant information in the known universe, which is nice, but perhaps the most useful piece of advice is featured on the cover: the words “DON’T PANIC.” This is a great suggestion for any occasion, but is especially timely as you open a textbook dedicated to two topics likely to strike feelings of fear, boredom, or both into the hearts of students and faculty members alike: statistics and software. We recognize that many of you are less than thrilled at the prospect of starting a course involving the use of statistical software, and that some of you experience a sizable amount of anxiety at the mere thought of this stuff. To you, especially, we would like to offer the same sage words of advice: don’t panic! You’re going to be okay. We have written this book as a very friendly introduction to SPSS (Statistical Package for the Social Sciences). The central goal of the book is to make potentially complicated concepts and procedures easy to understand, easy to complete, and (when humanly possi- ble) fun to work with. We have found that if you are having fun, it is hard to be anxious. Humor can often distract students from the anxiety they feel, and this in turn gives them a chance to develop their skills as statistical thinkers and develop a sense of confidence in their own abilities. In our approach, every chapter features a cartoon on which humorous (hopefully) and absurd (certainly) data sets are based. A cursory flip through the book will reveal penguin-soliciting pilgrims, college professors from other dimensions, question- naires for lions, zombies taking statistics, and anti-social one-eared bunnies on Prozac. However, this book was not written solely for stats-anxious students. As Cartoon 1.1 clearly shows, some of you will find that SPSS represents a clear path to “Nerdvana.” Cartoon 1.1 DILBERT © 1991 Scott Adams. Used by permission of UNIVERSAL UCLICK. All rights reserved. 1 2 SPSS: A USER-FRIENDLY APPROACH Whether you are totally comfortable with quantitative topics (and come to SPSS in an altered state of geeky bliss) or you are completely stressed out when sitting in front of a computer, we will tell you what you need to know in as clear and concise a manner as we can. A careful reading will reveal that behind the goofy cartoons and examples, you have purchased a very comprehensive introduction to SPSS. It covers the procedures traditionally included in introductory level statistics courses and a number of more ad- vanced topics typically covered in courses at the upper-level undergraduate and Master’s levels. In addition, we have organized this book so that it can serve as both an initial in- troduction to SPSS now and a useful reference tool in the future. Finally, this book was written to reinforce the concepts you are likely to cover in statistics courses in the social, behavioral, and educational sciences. In conjunction with a traditional statistics text- book, this book will help you to develop a firm conceptual and applied understanding of quantitative techniques using one of the most user-friendly software packages available for statistical analysis—SPSS! HOW TO USE THIS BOOK Generally speaking, the chapters in this book are all organized in the same way. Each chapter opens with a brief introduction to the procedure(s) of interest. These introduc- tions focus on the organizational themes for the book: (a) deciding when procedures are appropriate to use, and (b) the types of research questions each procedure is typically used to address. These themes are described in more detail later in this chapter. Following the introduction, we present the steps you need to take in SPSS to conduct the procedures. In each chapter, we will walk you through SPSS procedures using an at-a-glance, step-by-step approach. Usually, a single figure visually presents the windows and dialogue boxes you will encounter when running a particular procedure. In addi- tion, the steps are outlined in greater detail within procedure boxes. This approach is in- tended to make the procedures easy to learn, easy to use, and easy to review. Our goal is to ensure that when you are sitting at your computer doing the analysis, you will always know what to do next. This book emphasizes more than just the mechanics of conducting data analysis in SPSS. For each procedure, we offer a detailed discussion of how to read the generated re- sults (i.e., the Output). Specifically, we direct your attention to the information you need in order to answer your research questions. We also offer a clear description of what the results tell us about the participants in our data set and their behavior. Furthermore, many instructors require their students to learn the hand calculations associated with basic statis- tical procedures. Where appropriate, we illustrate ways in which the results obtained from SPSS can be used to check the results obtained from hand calculations. Finally, a major part of learning is practice. Each chapter concludes with a set of Prac- tice Exercises. These problems are designed to reinforce the major concepts presented in the chapter. DATA ANALYSIS AS A DECISION-MAKING PROCESS Like Calvin in Cartoon 1.2, many students are disappointed to discover that com- puters will not just do the work for you. When using SPSS, you still have to create variables, enter data, request the appropriate procedures, and interpret the results. More INTRODUCTION TO SPSS: A USER-FRIENDLY APPROACH 3 Cartoon 1.2 Reprinted with permission of UNIVERSAL UCLICK. CALVIN AND HOBBES © 1995 Watterson. All rights reserved. importantly, you need to make decisions about what to do with your data. The value of SPSS is that once you know what to do with your data, it becomes quite easy to get the information you want. Unfortunately, we cannot provide a simple mnemonic device or road map approach that will tell you what to do in every data analysis situation. In large part, students de- velop this knowledge over time by working with different types of data. However, we do offer a simplified framework for making common statistical decisions. The rules of thumb we offer here will help you make decisions about the statistical procedures you will likely encounter in statistics and research courses at the undergraduate and Mas- ter’s levels. This decision-making framework serves as the core organizing theme for the chapters that follow. We strongly encourage you to familiarize yourself with this framework and to periodically return to this section as you begin to learn new statistical procedures. WHAT TYPE OF DATA DO YOU HAVE? When choosing an appropriate procedure, keep in mind that each statistical procedure an- swers a particular kind of question, and the kind of question you ask depends on the type of data you have. The first step in choosing a statistic is deciding what type of data you have. Over the years, statisticians have classified data in a variety of ways. A common approach is based on a hierarchy of levels or scales of measurement: nominal, ordinal, interval, and ratio. Many books also refer to a distinction between discrete and continuous data. These and other organizational approaches certainly have their place, and having a fully developed understanding of statistics requires you to make use of these distinctions at various times. However, in the interest of keeping things simple, we focus on a dis- tinction between variables that represent groups and variables that represent scores. As you familiarize yourself with the group/score distinction, you will likely find variables that seem to straddle both categories. In most cases, however, these conflicts can be resolved by considering how you intend to use the measurement. Regardless, in a vast majority of the data analysis problems you will face, this simple distinction is enough to make good decisions about how to analyze your data. By Group Variable, we mean any way of assigning different types of people, events, or outcomes to particular categories. Returning to Cartoon 1.2, assume that we want to study children’s beliefs about computers. We can employ a great variety of group-based variables 4 SPSS: A USER-FRIENDLY APPROACH for this task. We could separately evaluate female children and male children. A group of children (ages 6 to 11) could be compared with a group of adolescents (ages 14 to 17). We could look at children with computers at home and children without. Or we could design an experiment where one group of children is given an opportunity to work with com- puters, while a control group performs a similar task without the use of computers. With respect to children’s beliefs about computers, we could also use group-based variables. For example, we could create categories of beliefs about computers within which the children could be classified. The Youth Inventory of Personal Computer Attitudes and Beliefs—Long Edition (YIPe-CompAttABLE) asks children to pick the statement that best describes their attitudes toward computers, and it groups respondents based on the statements they pick. The groupings are as follows: Which of the following statements is most like you? 1. I think computers are a tool for solving problems more efficiently. 2. I think computers do work that humans do not have the time or motivation to do. 3. I think computers make simple tasks too complicated. 4. I think computers were created by a society of underground mole people in order to distract us so they can steal all the prizes from our boxes of breakfast cereal. Alternatively, a simple yes/no format could be used, which asks children whether they like using computers. This too would represent a group variable. Group variables can also be used to represent random discrete outcomes (either/or). For example, if we had children flip a coin, then the outcomes could be grouped as ei- ther heads or tails. Similarly, children rolling a six-sided die would give us six possible outcomes or groupings: either a 1 or a 2 or a 3, etc. For the most part, variables like this should also be classified as group variables. Score Variables are used when we want to represent how much or how many of some- thing we have. The value of a score variable represents the location of a participant along a numerical range of possible values. With respect to the characteristics of the children in our study, we could use age as a variable. Whether we measure age in months or years, it would represent a numerical range of possible values. Other scores we could use include height, weight, IQ, score on an educational achievement test, grade point aver- age, classroom teacher-to-student ratios, hours per week spent using a computer, family income, number of computers in the home, or the number of tasks correctly completed in a basic computer skills test. Another type of score, which is of particular interest to social and behavioral scientists, consists of attitude and personality ratings. For example, we could ask participants to rate the statement “I like to use computers” using a 5-point scale: (1) Strongly Disagree, (2) Disagree, (3) Neither Disagree nor Agree, (4) Agree, or (5) Strongly Agree. Most attitude/personality measures consist of multiple items, and the ratings for each item are combined to form a single score (usually through averaging or summing; this is covered in Chapters 6 and 14). Now that we have defined group and score variables, it should be noted that variables representing rankings do not really fit into this framework. Ranked variables (also called ordinal variables) reflect categories that have a logical order to them. For example, we could rank the height of 10 children, where 1 is the tallest and 10 is the shortest. Similarly, if we ranked the computer skills of the students in Calvin’s classroom from most skilled to least skilled, then we would have an ordinal level of measurement. Ranked variables typically require special statistical procedures that lie beyond the scope of this text. INTRODUCTION TO SPSS: A USER-FRIENDLY APPROACH 5 MATCHING VARIABLES WITH STATISTICS Once you have identified the type of data with which you are working, you can begin the process of selecting a statistical procedure. Different procedures are available depend- ing on the type of data you have and whether you are describing one variable or the relationship between two or more variables. Figure 1.1 shows the statistical procedures Figure 1.1 Matching Variables with Statistics Group Variables Score Variables Group Variables with One Variable One Variable Score Variables Frequencies (%) Ch. 4 Sum Ch. 3 One Group and Mode Ch. 5 Frequencies (%) Ch. 4 One Score Variable Goodness-of-Fit Central Tendency Ch. 5 Chi-Square Ch. 13 Variability Ch. 5 2 Groups: Normality Ch. 5 Indepdendent Sample Two Variables Z-Scores Ch. 6 t-Tests Ch. 7 Single-Sample 2 or More Groups: Pearson’s Chi- t-Tests Ch. 7 One-Way Square Ch. 13 ANOVA Ch. 8 Two Variables Two or More Group Correlation Ch. 11 Simple Variables and Regression Ch. 11 One Score Variable Two or More Variables Factorial ANOVA Ch. 9 Combined Scores Ch. 6 Multiple Repeated-Measures Regression Ch. 12 Variables Reliability Ch. 14 Factor Analysis Ch. 15 2 Time Points: Paired-Samples t-Test Ch. 7 2 or More Time Points: Repeated-Measures ANOVA Ch. 10 Within-Subjects & Between-Subjects: Mixed Model ANOVA Ch. 10 6 SPSS: A USER-FRIENDLY APPROACH included in this book, along with the chapters in which they are presented. The first column applies to situations where group variables are of interest, the second column applies to variables representing scores, and the third column applies to situations where we are interested in assessing the influence that group variables have on scores. The third column also lists a group of statistics that apply to Repeated-Measures Variables. Repeated- measures procedures represent a unique set of analyses and will be described in a separate section later in this chapter. Evaluating a single variable. Typically, describing or summarizing the characteristics of individual variables is the first step in a larger data analysis plan for a study. For exam- ple, if we have a single group variable that classifies children into two groups—those who have computers at home and those who do not—we could obtain the frequency (number) or percentage of children in each group. We could then use this information to construct tables and charts to visually summarize the number of participants in each category (Chapter 4). We could also determine which group had the most children in it (the modal group; Chapter 5). More ambitiously, we use the Goodness-of-Fit Chi-Square (Chapter 13) to determine whether the ratio of children with computers to children without computers in our sample meaningfully differs from the ratio found (or expected) in the population. A very different set of procedures is available to describe a single variable that repre- sents scores. For example, if we had a variable that represented the number of hours per week that each child uses a computer, the sum procedure (Chapter 3) will calculate the total number of hours that the children as a group use a computer each week. Obtaining the sum of a set of scores is a common first step in completing many other statistical pro- cedures. Frequency Tables or Charts will visually summarize the computer data (Chapter 4). Measures of Central Tendency (Mean, Median, and Mode; Chapter 5) will tell us what amount of time is most representative of how long children use a computer each week. Similarly, measures of Variability (Standard Deviation, Variance, and Range; Chapter 5) and Z-scores (Chapter 6) can help us determine how representative a given score is based on how much children differ in the amount of time they spend using a computer each week. Measures of Normality (Skewness and Kurtosis; Chapter 5) will determine whether the shape of the distribution of scores for Children’s Computer Use resembles the shape of distributions typically collected from populations (e.g., the Normal Curve). Finally, a Single-Sample t-Test (Chapter 7) can determine whether the average amount of time chil- dren in our sample use a computer each week differs from some comparison value, such as the population average. Evaluating associations between two variables. Although describing the characteristics of a single variable is an important first step, frequently the goal of research is to demonstrate how two variables are related to or influence one another. With respect to group vari- ables, we can use Pearson’s Chi-Square (also called the Test of Independence; Chapter 13) to determine whether male and female children differ with respect to the likelihood that they have a computer in their home. Alternatively, with respect to score variables, we can use a Correlation Coefficient (e.g., Pearson’s r) or Simple Linear Regression (Chapter 11) to determine whether children who use computers more frequently also tend to score higher on tests of computer skills (a positive correlation). INTRODUCTION TO SPSS: A USER-FRIENDLY APPROACH 7 We can also assess the relationship between one group variable and one variable rep- resenting scores. First, if we have a group variable that is comprised of two groups (e.g., children with and without computers at home), we can use an Independent Samples t-Test (Chapter 7) or One-Way ANOVA (Analysis of Variance; Chapter 8). These tests will de- termine whether a meaningful difference exists between the two groups with respect to their weekly amount of computer use. However, if the group variable represents more than two groups (e.g., children, adolescents, and adults), then a one-way ANOVA should be used to determine whether significant differences are observed among the groups. Questions with more than two variables. The ability to include more than two variables in a particular analysis allows researchers to address a wide range of complex and interesting questions. Multiple Regression (Chapter 12) can be used to answer a variety of questions regarding how two or more score variables are related to (or predict) the scores of an- other variable. Alternatively, multiple score variables can be combined to form a single score, such as an average or summed score (Chapter 6). Furthermore, Reliability Analyses (Chapter 14) and Factor Analysis (Chapter 15) can be used to determine which combina- tions of scores make the most useful groupings. Factor analysis, reliability analysis, and the ability to form combined scores are all commonly used when working with multi- item measures such as achievement tests, clinical assessments, or attitude and personality measures. When you have two or more group variables and a single variable representing scores, you can use Factorial ANOVA (Chapter 9) to answer questions very similar to the ones asked in one-way ANOVA and t-tests. For example, in a single procedure we could determine whether children who have computers at home differ from children who do not and whether males differ from females with respect to scores on a computer skills test. In addition, factorial ANOVA can address the more complex question of whether the two group variables interact with one another. For example, this same analysis can determine whether differences found between children with and without computers de- pend on whether the child is male or female. Among other potential patterns of results, it may be that males who have computers at home demonstrate greater skill than males who do not have computers at home; but among females, computer skills are high re- gardless of whether they have computers. REPEATED-MEASURES VARIABLES As the name implies, you have repeated-measures variables when you collect the same measurements from the same group (or groups) of people at different points in time. For example, we could give our sample of children a measure of computer skills before training (pre-test), administer a training program, and then measure their computer skills again (post-test).This is generally referred to as a Within-Subjects Design. Had we meas- ured computer skills among two different groups of participants, one that has received training and one that has not, then we would have a Between-Subjects Design (which is just another way of saying we have a group variable). Further, had we taken pre-test and post-test computer skills measurements (within-subjects variable) from one group that receives training and another that does not (between-subjects variable), then we would have a Mixed Design (i.e., the design contains a mixture of within- and between-subjects variables). 8 SPSS: A USER-FRIENDLY APPROACH When you have two measurements taken at different times, then a Paired-Samples t-Test (Chapter 7) or a One-Way Repeated-Measures ANOVA (Chapter 10) can tell you whether the average score taken during the first point in time meaningfully differs from the average score taken during the second point in time. One-way repeated-measures ANOVA can also be used when measurements have been collected from more than two time points. For example, we could compare the computer skills scores of children before training (Time 1), after one training session (Time 2), and after two training sessions (Time 3). Finally, the Mixed-Model Repeated-Measures ANOVA (Chapter 10) can be used with mixed (between-within) designs. Like factorial ANOVA, the mixed- model ANOVA provides a single procedure that will determine whether any differences exist between the groups for the between-subjects variable and whether any differences exist between the times for the within-subjects variable. Further, the procedure will test the interaction of the two independent variables. For example, we can ask whether the size of the differences found in children’s pre-test and post-test scores on a computer skills assessment depends on whether the children are male or female. SUMMARY Making good decisions about what statistical procedures are appropriate for a given situ- ation is a matter of knowing what kind of data you have. If you want to compare groups with groups, then you can evaluate frequencies and chi-square statistics. If you want to compare scores with other scores then correlation, regression, and multiple regression may be appropriate. If you want to compare the scores of different groups, then t-tests, ANOVA, or factorial ANOVA may be appropriate. Finally, if you have given the same measure to the same people on more than one occasion and you want to compare those scores, then a repeated-measures analysis of some sort is called for. We realize this will seem like a lot to cover all at once; after all, you are going to take at least a semester to work your way through these things. Don’t panic! This scheme is intended to help you place each new topic you cover within a relatively simple framework. Do not bother trying to commit the framework to memory, but go back to it periodically until you become more familiar with it. Hopefully, it will come in handy later when you need to pick the right procedure to answer a particular kind of question with a particular type of variable (group or score) or variables. For now, we just want you to be aware of the big picture: Different statistical procedures answer different kinds of questions about differ- ent kinds of data. PRACTICE EXERCISES The following exercises are meant to reinforce the concepts we have introduced in this chapter. If this is your first statistics course, parts B and C of these exercises will probably be quite challenging, especially at the start of the semester. You may want to return to these problems later in the semester to evaluate how much you have learned about how to pick the correct statistical procedure. A. Determine whether the variables described below reflect group variables or score variables. 1. Ethnicity/race. INTRODUCTION TO SPSS: A USER-FRIENDLY APPROACH 9 2. Time spent showering per day. 3. Hair color. 4. Average ratings on a 10-item measure of depression. 5. Political affiliation. 6. Religious affiliation. 7. Years of education. 8. Attitude toward statistics rated using a 7-point scale. 9. Number of pets a person owns. B. For the following, determine whether the design of the study is between- subjects, within-subjects, or a mixed (between-within) design. 10. In an experiment, one-third of the participants are asked to read cartoons for 30 minutes. Another one-third of the participants are asked to watch a cartoon TV show for 30 minutes. The final one-third of the participants are asked to sit quietly for 30 minutes. All participants then completed a measure of statistics attitudes. 11. In an experiment, all participants completed a measure of attitudes toward statistics. Half of the participants then received a 30-minute review of statistics instruction using a cartoon approach. The other half of the participants read cartoons for 30 minutes. All participants were then asked to complete the measure of attitudes toward statistics for the second time. 12. Researchers asked participants (all currently enrolled in a statistics course) to complete a measure of attitudes toward statistics once a week for the entire semester in which they were enrolled in the course. 13. Researchers asked participants (all currently enrolled in a statistics course) to complete a measure of attitudes toward statistics once a week for the entire semester in which they were enrolled in the course. The researchers are interested in testing whether male and female participants differ at and across the various time points. C. For the following, indicate which statistical procedure would be appropriate. 14. Researchers want to know whether male and female children differ in the number of hours per week they use a computer. 15. Researchers want to know whether the age of participants is related to the number of hours per week they use a computer. 16. Researchers want to describe a variable representing the number of different computers participants use in a week. 17. Researchers want to describe a variable representing the type of operating system children use most often (e.g., Mac OS versus Windows). 18. Researchers want to know whether participants’ gender/sex (male versus female) is related to the type of operating system children use most often. 19. Researchers want to compare the computer skills test scores for students from third-, fourth-, and fifth-grade classes. 10 S P S S : A U S E R - F R I E N D L Y A P P R O A C H 20. Researchers want to compare the computer skills test scores for male and female students that come from third-, fourth-, and fifth-grade classes. 21. Researchers want to know whether the number of hours per week children use computers and the number of students in the children’s classrooms are associated with the children’s scores on a computer skills test. chapter two Basic Operations C hapter 1 presented a very conceptual overview of SPSS. The present chap- ter will walk you through the routine procedures that you will use nearly every time you work with SPSS. Specifically, you will learn how to navigate the SPSS environment; enter, save, and retrieve data sets; create, modify, and save text-based records of the procedures you perform (syntax); and generate, navigate, save, and print the results of your data analyses (output). THREE WINDOWS The base version of SPSS can be split into three major parts: the Data Editor (where we enter data and create new variables), the Syntax Editor (where we store and create syntax for our analyses and procedures), and the IBM SPSS Statistics Viewer (where we view the output/results our statistical analyses have generated). The student version of SPSS does not include the Syntax Editor, but the Data Editor and Output Viewer operate in the same manner as the base version. Each of the major parts of SPSS has its own program window in SPSS. When more than one SPSS window is open, separate preview thumb- nails will be available for each SPSS window; thumbnails appear when you click the SPSS button on the Windows task bar (see Figure 2.1). You can navigate between the different windows of SPSS by left-clicking on the appropriate thumbnail. When work- ing in the SPSS windows, if you close either the SPSS Viewer or the Syntax Editor the remaining windows will not be affected. However, closing the Data Editor closes the entire SPSS program, and the SPSS Viewer and Syntax Editor will also close. Figure 2.1 Windows Task Bar 11 12 S P S S : A U S E R - F R I E N D L Y A P P R O A C H DATA EDITOR The first step in any data analysis process is to set up the data file in the Data Editor (you can create variables and enter data in the Syntax Editor, but that is beyond the scope of this text). The SPSS Data Editor is split into two parts (or views): the Variable View and the Data View. The Data View allows you to view and input data. The columns in the Data View represent variables and the rows represent observations/participants/ subjects (often referred to as “cases”). The Variable View allows you to edit variables and add new variables to the data set. Note that in the Variable View, the rows represent each variable and correspond to the columns in the Data View. The columns in the Var- iable View represent different aspects of each variable. Figure 2.2 presents the Variable View and the Data View for a blank Data Editor file. Window A shows the Variable View and Window B shows the Data View. To toggle back and forth between the two views, click on the labeled buttons located at the bottom left-hand corner of the Data Editor spreadsheet. In Figure 2.2, the buttons are marked with the letter C. Figure 2.2 Variable View and Data View for a Blank Data File A C B C B A S I C O P E R A T I O N S 13 CREATING NEW VARIABLES Cartoon 2.1 inspired the data set that you will work with in this chapter. Helga’s state- ment about Hagar implies that he would not know what the well water tastes like be- cause he only drinks beer. Beer holds a special place in the hearts of most statisticians as some of the basic statistical procedures and assumptions used today were developed by William Gossett, an employee of the Guinness brewery in Ireland. In the early 1900s, Gossett developed the Student t-distribution so that he could select the best varieties of barley for producing beer. Thus, without beer there would be no statistics, although you may argue that without statistics there would be little need for beer. It seems likely that both statements are true. Cartoon 2.1 HAGAR© 1991 King Features Syndicate, Inc., World Rights Reserved Assume that a researcher is interested in the drinking habits of different cartoon char- acters. Specifically, she is interested in the types and amounts of beer consumed by car- toon characters. The type of beer would be considered a group variable. The amount in this case is operationalized as the number of beers (12-oz. cans) consumed per week, and this variable represents a set of scores. If the distinction between variables represent- ing groups and scores is unclear to you, then it would be a good idea to go back and review the discussion in Chapter 1. Table 2.1 displays the result of the study. The first column lists the participant number by which each cartoon character is identified. Hagar is participant number 15. The second column presents the number of beers consumed per week by each character. The third column presents the brand of beer preferred by each character. Variable Names. To create new variables on a blank data file, select the Variable View. Give each variable a variable name by typing the desired name in the first open row of the first column, labeled Name. Older versions of SPSS limited variable names to eight characters. Newer versions allow for much longer variable names (approximately 60 characters), though it is a good idea to keep them as short as possible; eight or fewer is ideal. Table 2.2 offers other guidelines for naming variables. For our example, we have named the first variable, representing the number of beers each cartoon character drinks a week, beerweek. Similarly, we have named the second variable, representing the brand of beer that each cartoon character drinks, beerbrnd. Figure 2.3 (on p. 15) presents the variable view with the variable names entered for our example. 14 S P S S : A U S E R - F R I E N D L Y A P P R O A C H Table 2.1 Amount and Brands of Beer Consumed by Cartoon Characters Participant # # of Beers Per Week Brand 1 1 Bongo Beer 2 2 Swiller Light 3 4 Swiller Light 4 4 Lights-Out-Lager 5 5 Lights-Out-Lager 6 6 Lights-Out-Lager 7 7 Lights-Out-Lager 8 7 Lights-Out-Lager 9 9 Budget Brew 10 10 Budget Brew 11 11 Budget Brew 12 12 Belcher’s Pride 13 12 Cirrhosis Light 14 12 Cirrhosis Light (Hagar’s Data) 15 15 Cirrhosis Light Table 2.2 Guidelines for Naming Variables Things you cannot do: Names cannot start with a number (1, 2, 3,…), though they can have numbers in them. Names cannot have spaces or the following symbols: - !%^&*+~ ( ) { }[ ] ?/> greater than ~ not - subtraction < less than ~= not equal to * multiplication >= greater than or equal to & and / division.05, use the Top Row (Equal variances assumed). If Levene’s Sig. <.05, use the Bottom Row (Equal variances not assumed). In this case, the alpha level is.313 (larger than.05), so the first row of t-test results is appropriate, which is circled and labeled B in Figure 7.6. The second test in the Independent Samples Test table is the t-test for Equality of Means. This section provides us with the t obtained, degrees of freedom (df ), the two-tailed level of significance (Sig.), and the mean difference (the difference between the two group means). IS THE TEST SIGNIFICANT? If t-test Sig. (2-Tailed) is >.05, then the difference between means is NOT sig- nificant. If t-test Sig. (2-Tailed) is <.05, then the difference between means IS significant. In this example, we have obtained a t-value of ᎑2.614 and, with 28 degrees of free- dom (df = n – 2), it has an alpha level (Sig.) of.014. Since the alpha level is less than.05, we can say the difference between the means is significant. It appears that humans who have been chased and those who have not are significantly different with respect to their attitudes toward zombies. More specifically, by examining the group means and the mean difference (Group 1 mean − Group 2 mean), we see that the humans who have been chased scored 5.32143 points lower on the ZOM-B than humans who had never been chased. Part C of the Independent Samples Test table provides confidence intervals for the difference between the group means. This interval allows us to estimate the actual mean difference between groups found in the population based on potential sampling error. In this case, with respect to the difference in attitudes toward zombies found between humans who have been chased and those who have not, we can be 95% confident that the actual difference in the population is somewhere between ᎑9.49147 and ᎑1.15139. C O M P A R I N G M E A N S I N S P S S ( t - T E S T S ) 101 PAIRED-SAMPLES t-TEST RUNNING THE PAIRED-SAMPLES t-TEST Like other t-tests, the Paired-Samples t-test allows us to test whether two sample means are significantly different from each other. The Paired-Samples t is appropriate when the means are collected from the same group on two separate occasions (typi- cally called repeated-measures or within-groups designs). Also, the Paired-Samples t may be used when means are collected from two different groups, where each mem- ber of one group has been paired or matched with a member of the other group based on one or more characteristics (e.g., age, IQ, economic status, etc.). In our current example, we have measured attitudes toward zombies twice: once before a sample of humans attended a Zombie Rights Sensitivity Training Workshop (zomb) and once after (zomb_2). With these data, we can test the hypothesis that the average of at- titudes toward zombies before getting sensitivity training differs significantly from the average of attitudes after training. PROCEDURE FOR RUNNING THE PAIRED-SAMPLES T TEST: 1 Select the Analyze pull-down menu. 2 Select Compare Means. 3 Select Paired-Samples T Test... from the side menu. This will open the Paired-Samples T Test dialogue box. 4 Select the first score variable (zomb) by left-clicking on the variable and then left-clicking the boxed arrow. Repeat these steps for the second score variable (zomb_2). The two variables will now appear as Pair 1 in the Paired Variables: field. 5 The order of the variables (which is treated as variable 1 and which is treated as variable 2) can be switched by clicking on the double-headed boxed arrow. If you have requested multiple analyses, you can change the order in which they will appear by using the up and down boxed arrows. 6 Finally, double-check your variables and either select OK to run or Paste to create syntax to run at a later time. If you selected the paste option from the procedure above, you should have generated the following syntax: T-TEST PAIRS= zomb WITH zomb_2 (PAIRED) /CRITERIA=CI(.9500) /MISSING=ANALYSIS. 102 S P S S : A U S E R - F R I E N D L Y A P P R O A C H Figure 7.7 Running the Paired-Samples T Test 1 2 3 4 5 6 READING THE PAIRED-SAMPLES t-TEST OUTPUT Figure 7.8 presents the output for the Paired-Samples t-test. This output consists of three major parts: Paired Samples Statistics, Paired Samples Correlations, and Paired Samples Test. The Paired Samples Statistics table provides the mean, sam- ple sizes (N), standard deviations, and standard error of the mean for each score vari- able. The Paired Samples Correlations table presents a statistic that will be covered in a later chapter on Correlation and Regression. Essentially, this statistic tells us how strongly related our two variables are. In the context of paired-samples t-tests, as the cor- relation value gets closer to 1.00, it indicates that the change observed is more uniformly experienced by participants. The Paired Samples Test table is split into two parts, which we have labeled A and B. Part A presents the basic parts of the formula to obtain the t-value for a paired- samples test. First, the mean difference is reported in the column labeled Mean. The mean difference is the numerator of the Paired-Samples t formula and can be obtained by subtracting the mean for the first measurement from the mean for the second mea- surement. In this case, the mean for the post-sensitivity-training variable (12.8000) is subtracted from the mean for the pre-sensitivity-training attitude variable (10.2667), which produces a value of ᎑2.5333. The second column, labeled Std. Deviation, re- ports the standard deviation of the difference, which is part of the denominator of the C O M P A R I N G M E A N S I N S P S S ( t - T E S T S ) 103 Figure 7.8 Output for the Paired-Samples t-Test T-Test Paired Samples Statistics Mean N Std. Deviation Std. Error Mean Pair 1 Zombie Opinion Measure 10.2667 30 6.09654 1.11307 (Form B) Time 2 Zombie Opinion 12.8000 30 6.42409 1.17287 Measure (Form B) Paired Samples Correlations N Correlation Sig. Pair 1 Zombie Opinion Measure (Form B) & Time 2 Zombie 30.988.000 Opinion Measure (Form B) Paired Samples Test Paired Differences A 95% Confidence Interval of the Difference Mean Std. Deviation Std. Error Mean Lower Upper Pair 1 Zombie Opinion Measure (Form B) - Time 2 Zombie Opinion Measure (Form B) -2.53333 1.04166.19018 -2.92230 -2.14437 Paired Samples Test B t df Sig. (2-tailed) Pair 1 Zombie Opinion Measure (Form B) - Time 2 Zombie Opinion Measure (Form B) -13.321 29.000 Paired-Samples t formula. The third column, labeled Std. Error Mean, reports the standard error of the mean difference, which is obtained by dividing the standard devia- tion of the difference by the square root of n. The standard error of the mean difference is the complete denominator of the Paired-Samples t formula. The last two columns of this table present the boundaries of the 95% confidence interval, within which the true mean difference for the population is expected to fall. Part B of the Paired Samples Test table presents the t obtained, degrees of free- dom, and two-tailed level of significance (alpha). In this case, the t obtained is ᎑13.321, there are 29 degrees of freedom (df = n − 1), and the alpha level is.000. Though SPSS reports a significance level of.000, it is generally inappropriate to report this level be- cause we can never be 100% sure our results did not occur by chance alone. Reporting.001 is the preferred method. IS THE TEST SIGNIFICANT? If Sig. (2-Tailed) is >.05, then the difference between means is NOT significant. If Sig. (2-Tailed) is <.05, then the difference between means IS significant. 104 S P S S : A U S E R - F R I E N D L Y A P P R O A C H In this example, the obtained alpha level of.001 is less than.05, so we can conclude that pre- and post-sensitivity-training means are significantly different. More specifi- cally, based on an examination of the difference between the average of attitudes toward zombies obtained before participating in the Zombie Rights Sensitivity Training Work- shop and the post-workshop attitudes, our humans’ average ZOM-B scores were 2.5333 points higher after attending the workshop compared to their pre-workshop attitudes. SUMMARY In this chapter, we covered the use of several tests that compare group means. Specifi- cally, we demonstrated how to conduct a One Sample t-test within SPSS to compare a sample mean with a population mean, how to conduct an Independent-Samples t-test to compare two sample means, and how to conduct a Paired-Samples t-test to compare the means obtained from a single group at two different points in time (or two groups that have been matched). Subsequent chapters will introduce methods for comparing means when you have more than two groups (ANOVA) or when you want to consider more than one group variable at a time (Factorial ANOVA). However, these procedures will include follow-up tests that are conceptually identical to the t-tests we have just introduced. PRACTICE EXERCISES Assume a researcher is interested in the frequency with which the members of a par- ticular population of zombies are able to catch the humans they are compelled to chase. The researcher draws a random sample of 20 zombies from a single city. The number of humans caught by each zombie is recorded during two separate one-week periods of observation: once before the townspeople implemented a zombie avoidance infor- mation campaign emphasizing the importance of cardio-training, and then once after implementing the campaign. The researcher also classified each zombie in the sample as belonging to one of two groups: Slow Zombies versus Fast Zombies. The results of the study are presented in Table 7.1. Use these data to conduct the analyses that would answer the research questions below. For each analysis, report the appropriate group means, group standard deviations, t-values, degrees of freedom, sample size (N), and level of significance, and then describe the results obtained (e.g., Did the groups signifi- cantly differ with respect to the attitude of interest?). 1. Does the number of humans captured per week by the zombies pre-campaign and post-campaign differ from the average number of humans captured by the entire population of zombies, which is estimated to be 4.00? 2. Does the number of humans captured per week by the zombies before the information campaign differ from the number captured after the information campaign, regardless of which type of zombie has captured them? 3. After the information campaign, do the slow zombies differ from fast zombies with respect to the number of humans they catch per week? 4. Before the information campaign, do the slow zombies differ from fast zombies with respect to the number of humans they catch per week? C O M P A R I N G M E A N S I N S P S S ( t - T E S T S ) 105 Table 7.1 Practice Exercise Data Pre-Zombie Avoidance- Post-Zombie Avoidance- Campaign Campaign Type of Zombie: Slow Number of Humans Number of Humans Zombies vs. Fast Zombie Caught Caught Zombies 1 1 1 Slow Zombies 2 1 1 Fast Zombies 3 2 1 Slow Zombies 4 2 2 Fast Zombies 5 3 1 Slow Zombies 6 3 3 Fast Zombies 7 3 1 Slow Zombies 8 3 3 Fast Zombies 9 4 1 Slow Zombies 10 4 4 Fast Zombies 11 4 2 Slow Zombies 12 4 4 Fast Zombies 13 5 3 Slow Zombies 14 5 5 Fast Zombies 15 5 3 Slow Zombies 16 5 5 Fast Zombies 17 6 4 Slow Zombies 18 6 6 Fast Zombies 19 7 4 Slow Zombies 20 7 7 Fast Zombies chapter eight One-Way ANOVA: Means Comparison with Two or More Groups T he previous chapter demonstrated methods for testing differences between the means of two groups. This chapter presents steps for testing differences between the means of two or more groups using the SPSS ANOVA proce- dures. Procedures are presented for running a One-Way Analysis of Variance (ANOVA), generating Fisher’s LSD Post Hoc Tests, and creating a chart that plots the group means. You should consider using one-way ANOVA when you have one independent variable consisting of two or more groups and one dependent vari- able consisting of scores for all the members of each group. SETTING UP THE DATA Assume that researchers are testing eight-year-olds’ tolerance for spicy foods (see Car- toon 8.1). Thirty children have been randomly assigned to one of three groups. Each group receives one of three types of chili sauce, each made with a different type of chili pepper: poblano (capsicum annuum c.), jalapeño (capsicum annuum c.), and habanero (cap- sicum chinense). The level of spicy heat for each of the three chili peppers is measured in Scoville Units and is found to be 1,000–1,500 Scoville Units for the poblano pep- pers, 2,500–8,000 Scoville Units for the jalapeño peppers, and 100,000–350,000 Scoville Units for the habanero peppers. The researchers measured the time in seconds it took before each of the 30 children had a “volcanic eruption” (i.e., spewed) after tasting the sauce. The data obtained by our scientists are presented in Table 8.1. Cartoon 8.1 CALVIN AND HOBBES © 1990 Watterson. Reprinted with permission of UNIVERSAL UCLICK. All rights reserved. 106 O N E - W A Y A N O V A : M E A N S C O M P A R I S O N W I T H T W O O R M O R E G R O U P S 107 Table 8.1 Volcanic Eruption Data Number of Seconds before Eight-Year-Olds Volcanically Erupt after Tasting Chili Sauce Poblano Peppers Jalapeño Peppers Habanero Peppers 1,000–1,500 su 2,500–8,000 su 100,000–300,000 su 10 8 5 11 9 6 12 10 7 13 11 8 14 12 9 14 12 9 15 13 10 16 14 11 17 15 12 18 16 13 Figure 8.1 presents the Variable View of the SPSS Data Editor, where we have defined two variables. The first variable corresponds to the three different groups of children that received the three different types of chili sauce. This variable is named Figure 8.1 Variable View for Volcanic Eruption Data 108 S P S S : A U S E R - F R I E N D L Y A P P R O A C H chili_group and is labeled “Type of Chili Sauce Each Child Received.” We have as- signed numerical values to each group and supplied value labels. We have paired 1 with the label “Poblano,” 2 with “Jalapeno,” and 3 with “Habanero.” The second variable represents the latency (amount of time) between the child tasting the chili sauce and spewing it. We have given it the variable name eruption and the label “Time (seconds) before ‘Volcanic Eruption’.” Figure 8.2 presents the Data View of the SPSS Data Editor. Here we have en- tered the volcanic eruption data for the 30 children in our sample. Remember that the Figure 8.2 Data View for Volcanic Eruption Data A B O N E - W A Y A N O V A : M E A N S C O M P A R I S O N W I T H T W O O R M O R E G R O U P S 109 columns represent each variable and the rows represent each observation, which in this case is each eight-year-old. For example, the first child (found in part A of the figure) tasted poblano chili sauce and spewed after 10 seconds. Similarly, the 30th child (found in part B of the figure) tasted the habanero chili sauce and spewed after 13 seconds. RUNNING THE ANALYSES To assess eight-year-olds’ tolerance for spicy food, the researchers have designed a study that will determine whether hotter chili sauces will cause children to erupt faster than milder chili sauces. The one-way ANOVA will determine whether the mean eruption time for each group differs from any of the other groups. If the results of the ANOVA are significant, then meaningful group differences exist. However, when you have more than two groups, a statistically significant one-way ANOVA result alone does not indi- cate which groups differ. In order to determine which groups significantly differ, follow- up tests are required. In this chapter, we use Fisher’s LSD t-tests to probe a significant one-way ANOVA result. PROCEDURE FOR RUNNING THE ONE-WAY ANOVA: 1 Select the Analyze pull-down menu. 2 Select Compare Means. 3 Select One-Way ANOVA... from the side menu. This will open the One-Way ANOVA dialogue box. 4 Enter the variable representing scores (eruption) in the Dependent List: field by left-clicking on the variable and left-clicking on the boxed arrow pointing to the Dependent List: field. 5 Enter the variable representing groups (chili_group) in the Factor: field by left-clicking on the variable and left-clicking on the boxed arrow pointing to the Factor: field. 6 Request the LSD t-tests by left-clicking the Post Hoc... button. Figure 8.4 presents the One-Way ANOVA: Post Hoc Multiple Comparisons dialogue box. 7 Select LSD under the Equal Variances Assumed options. 8 Left-click Continue to return to the One-Way ANOVA dialogue box. 9 Left-click the Options... button (shown in Figure 8.3) to request descriptive statistics for each of the groups and a line chart that will plot the group means. This will open the One-Way ANOVA: Options dialogue box (shown in Figure 8.4). 110 S P S S : A U S E R - F R I E N D L Y A P P R O A C H Figure 8.3 Running the One-Way ANOVA 1 2 3 6 4 9 5 13 10 Select Descriptive from the Statistics options. This will provide you with the sample size, mean, standard deviation, standard error of the mean, and range for each group. 11 Select the Means plot option. This will provide you with a line chart that graphs the group means. 12 Left-click Continue to return to the One-Way ANOVA dialogue box. 13 Finally, double-check your variables and options and either select OK to run or Paste to create syntax to run at a later time. If you selected the paste option from the procedure above, you should have generated the following syntax: ONEWAY eruption BY chili_group /STATISTICS DESCRIPTIVES /PLOT MEANS /MISSING ANALYSIS /POSTHOC = LSD ALPHA(.05). O N E - W A Y A N O V A : M E A N S C O M P A R I S O N W I T H T W O O R M O R E G R O U P S 111 Figure 8.4 One-Way ANOVA Procedure Continued 7 10 11 12 8 READING THE ONE-WAY ANOVA OUTPUT The One-Way Anova Output is presented in Figures 8.5, 8.6, and 8.7. This output con- sists of four major parts: Descriptives (in Figure 8.5), ANOVA (in Figure 8.5), Post Hoc Tests (in Figure 8.6), and the Means Plots (in Figure 8.7). READING THE DESCRIPTIVES OUTPUT The Descriptives table (part A of Figure 8.5) provides each groups’ sample size (N), mean, standard deviation, standard error of the mean (the standard deviation divided by the square root of N, which estimates the potential for sampling error), and minimum and maximum scores. This part of the output also presents the confidence intervals within which we are 95% confident that the true population mean of time before eruption for each group will fall. We usually only need to concern ourselves with the means and stand- ard deviations for each group. In this case, the means and standard deviations (presented in parentheses) for the poblano group, the jalapeño group, and the habanero group are 14.00 (2.58199), 12.00 (2.58199), and 9.00 (2.58199), respectively. Eyeballing our data, we be- gin to suspect that the habanero group erupted more quickly than the other two groups. READING THE ANOVA OUTPUT Like ANOVA Summary Tables presented in most textbooks, the three rows of the ANOVA table for our example (part B of Figure 8.5) present the different compo- nents of an ANOVA: Between Groups information, Within Groups information, and 112 S P S S : A U S E R - F R I E N D L Y A P P R O A C H Figure 8.5 ANOVA Output for Volcanic Eruption Data Oneway Time (seconds) before "Volcanic Eruption" Descriptives A 95% Confidence Interval for Mean N Mean Std. Deviation Std. Error Lower Bound Upper Bound Minimum Maximum Poblano 10 14.0000 2.58199.81650 12.1530 15.8470 10.00 18.00 Jalapeno 10 12.0000 2.58199.81650 10.1530 13.8470 8.00 16.00 Habanero 10 9.0000 2.58199.81650 7.1530 10.8470 5.00 13.00 Total 30 11.6667 3.25188.59371 10.4524 12.8809 5.00 18.00 ANOVA B Time (seconds) before "Volcanic Eruption" Sum of Squares df Mean Square F Sig. Between Groups 126.667 2 63.333 9.500.001 Within Groups 180.000 27 6.667 Total 306.667 29 information regarding the Total sample. The first column shows that the Sums of Squares between groups (SSbetween) is 126.667, the Sums of Squares within (SSwithin) is 180.00, and the Sums of Squares for the total sample (SStotal) is 306.667. The second column presents the degrees of freedom (df ) between groups (number of groups minus 1), degrees of freedom within groups (n minus number of groups), and total degrees of freedom (n minus 1). In this case, the dfbetween is 2 because there are three groups; the dfwithin is 27 because there are 30 children in the sample and three groups; and the dftotal is 29 because there are 30 children in the sample. The third column presents the Mean Square (MS) between groups and within groups, respectively. MSbetween is obtained by dividing the SSbetween by the dfbetween. In this case, MSbetween = 63.333. Similarly, MSwithin is obtained by dividing the SSwithin by the dfwithin. In this case MSwithin = 6.667. The fourth and fifth columns present the final F statistic and its associated level