Skewness and Kurtosis PDF
Document Details
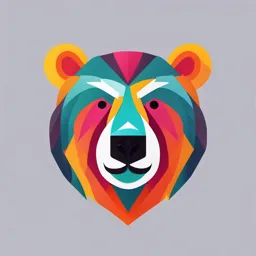
Uploaded by AffordableCombination
Tags
Summary
This document provides an introduction to skewness and kurtosis, which are statistical measures used to describe the shape of probability distributions. It explores different types of skewness and kurtosis including the formulas to calculate them. It also discusses the applications in hypothesis testing and practical scenarios.
Full Transcript
SKEWNESS Skewness â—¦Skewness is a measurement of the distortion of symmetrical distribution or asymmetry in a data set. Skewness is demonstrated on a bell curve when data points are not distributed symmetrically to the left and right sides of the median on a bell curve. â—¦Skewness is used in...
SKEWNESS Skewness ◦Skewness is a measurement of the distortion of symmetrical distribution or asymmetry in a data set. Skewness is demonstrated on a bell curve when data points are not distributed symmetrically to the left and right sides of the median on a bell curve. ◦Skewness is used in hypothesis testing to determine whether a sample of data is normally distributed or not. If the skewness value is close to zero, the data is likely to be normally distributed. If the skewness value is positive or negative, the data is likely to be skewed and may require non-parametric tests for hypothesis testing. ◦Normal distributions have zero skewness. This means that the distribution ends up being symmetrical around the mean. Having said that, there are instances where skewness isn't symmetrical. In these cases, it can be either positive or negative. Below, we highlight what each type of skewness means. Positive Skewness ◦A distribution is positively skewed when its tail is more pronounced on the right side than it is on the left. Since the distribution is positive, the assumption is that its value is positive. As such, most of the values end up being left of the mean. This means that the most extreme values are on the right side. As an investor, you may find that you have some small losses with a positive skew. But you may also end up realizing large gains—albeit fewer. Negative Skewness ◦Negative skewness, on the other hand, occurs when the tail is more pronounced on the left rather than the right side. Contrary to the positive skew, most of the values are found on the right side of the mean when it comes to negative skewness. As such, the most extreme values are found further to the left. Having a negative skew may indicate that you can expect some small gains here and there. But you can generally expect to see a few large losses here and there as an investor. ◦Skewness is a statistical measure that is used to show whether a distribution is distorted or asymmetrical. If the skewness is right-tailed (meaning the right tail is more pronounced than the left), the skew is positive. In this case, the values are more than zero. If the opposite is true and the tail is more pronounced on the left, then the skew is negative, where the values are less than zero. Measuring Skewness ◦There are several ways to measure skewness. Pearson’s first and second coefficients of skewness are two common methods. Pearson’s first coefficient of skewness, or Pearson mode skewness, subtracts the mode from the mean and divides the difference by the standard deviation. Pearson’s second coefficient of skewness, or Pearson median skewness, subtracts the median from the mean, multiplies the difference by three, and divides the product by the standard deviation. ◦High skewness means a distribution curve has a shorter tail on one end a distribution curve and a long tail on the other. The data set follows a normal distribution curve; however, higher skewed data means the data is not evenly distributed. The data points favor one side of the distribution due to the nature of the underlying data. ◦ Kurtosis is a statistical measure that is used to describe distributions. Kurtosis is defined as a measure of ‘peakedness’ and is generally measured relative to normal distributions. Calculating Kurtosis ◦Here we show the formula to calculate the measure of Kurtosis. ◦If the resultant value is zero, it indicates that the distribution is mesokurtic. A value less than 0 indicates platykurtic distribution and a value greater than 0 indicates leptokurtic distribution. Note that this gives an excess of kurtosis relative to a normal distribution. ◦To summarize, we can say that If skewness is less than −1 or greater than +1, the distribution is highly skewed. ◦If skewness is between −1 and −½ or between +½ and +1, the distribution is moderately skewed, and If skewness it is between −½ and +½, the distribution is approximately symmetric. ◦Similarly, a distribution with kurtosis approximately equal to 3 or excess of kurtosis=0 is called mesokurtic. A value of kurtosis less than 0 indicates a platykurtic distribution and a value greater than 3 indicates a leptokurtic distribution. ◦A normal distribution is a mesokurtic distribution. When are skewness and kurtosis applied? ◦We use skewness and kurtosis to assess how far a distribution is from a normal distribution. Skewness is more commonly used and reported as selection of statistical measures is often based on the degree of skewness. Kurtosis is used less often, but is very helpful in assessing the distribution of variables under study. Example ◦Example 1: Calculate Sample Skewness, Sample Kurtosis from the following data 3,13,11,11,5,4,2 x = ∑x/n = 3+13+11+11+5+4+2/7 = 49/7 Mean = 7 SD = √ =√ =√20.33 = 4.51 x ( x- ( x- mean)² ( x- mean) mean)³ 3 3-7= -4 16 -64 256 13 6 36 216 1296 11 4 16 64 256 11 4 16 64 256 5 -2 4 -8 16 4 -3 9 -27 81 2 -5 25 -125 625 N= 7 ∑( x- ∑( x- = mean)²= mean)³ = 2786 122 120 ◦Skewness = = = = 0.22- Symmetric ◦Kurtosis = = = = 0.96 - mesokurtic