Session 4 - Intro to Applied Statistics PDF
Document Details
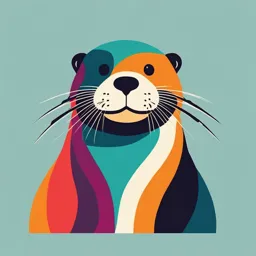
Uploaded by AmenableHurdyGurdy5261
University College London
2024
Asam Saleh
Tags
Related
Summary
This document is lecture notes on applied statistics, specifically focusing on statistical analyses and investigations in the context of mental health research and outcome measurement. It covers continuous outcomes, binary outcomes, count outcomes, and time-to-event outcomes. The document also introduces topics such as hypothesis testing, p-values, confidence intervals, as well as different types of regression models.
Full Transcript
Session 4: 4th Oct - pt2 Created @September 24, 2024 2:53 PM Tags Intro to Applied Statistics: Lead Teachers: Asam Saleh To be covered: 3 most common outcome variables used in mental health research; continuous...
Session 4: 4th Oct - pt2 Created @September 24, 2024 2:53 PM Tags Intro to Applied Statistics: Lead Teachers: Asam Saleh To be covered: 3 most common outcome variables used in mental health research; continuous outcomes, binary outcomes and rates. Using practical concepts to decide which methods to use and how to interpret results in papers. Reading list: PSBS0002: Core Principles of Mental Health Research | University College London (talis.com) Class Prep: Webpage: How to Calculate the Mean Value Webpage: Standard Deviation and Variance Notes: Objectives: To describe the main aims of statistical analyses and investigations To comment on the three most common types of outcome measurement seen in mental health research papers: A. Continuous outcomes B. Binary outcomes C. Count outcomes D. Time-to-event outcomes Session 4: 4th Oct - pt2 1 To understand: A. hypothesis testing B. p-values, and C. confidence intervals Why do we use stats in MH? We want to answer questions about mental health in the population: Description How common are mental health problems? Who is more likely to develop mental health problems? Causation What causes mental health problems? What are the outcomes of mental health problems? Which treatments are effective? Statistics is the science of collecting, summarising, presenting and interpreting data to answer these questions. Patient/problem, intervention, comparison, and outcome: the PICO framework Four step approach to research: 1. Patient/problem 2. Intervention 3. Comparison 4. Outcome Simple framework for understanding research Step 1: Research Question Are adverse childhood experiences [ACEs] associated with psychotic symptoms in adolescence? (risk factor) Session 4: 4th Oct - pt2 2 Is mirtazapine + sertraline (TAU) more effective than sertraline (TAU) in reducing depressive symptoms? (treatment effectiveness Step 2: Define the Comparison We often compare two or more groups Those with (i.e., exposed) vs. those without (i.e., unexposed) a risk factor to a risk factor of interest [experience of ACEs, no ACEs] Those who receive an active treatment (i.e., exposed) or a placebo/TAU (i.e., unexposed) [mirtazapine + sertraline vs. sertraline] Step 3: Collect & Summarise the Data For each group we collect mental health data on: Psychotic symptoms Depressive symptoms As well as other variables and often at more than one time point. Step 4: Test for differences between groups We estimate the size or magnitude of this difference in mental health symptoms (often referred to as effect size or association) Mean-difference Odds-ratio Risk-ratio We estimate the precision of these associations using 95% confidence intervals (CI). The width of the CI indicates how precisely we have estimated these associations. We test the strength (p-value) and precision (95% confidence intervals) of the evidence (e.g., how precisely have we estimated this effect? How confidently can conclude that there is a difference between the groups? How do we test these differences? With ‘Regression Models’: Session 4: 4th Oct - pt2 3 Which one we use depends on the nature of the outcome: A. Continuous outcome → linear regression B. Binary outcome → logistic regression C. Count outcome → Poisson regression A. Continuous Outcome: Comparing two means when the outcome is continuous - Linear Regression Comparison of two means What do we mean by continuous outcome (a.k.a. “quantitative trait”) Depressive (psychotic, eating disorder) symptom score BMI Height Age Blood pressure Real Life Example STEP 1: A research team wish to investigate the effectiveness of combining the antidepressant mirtazapine with other antidepressants (treatment as usual), for treatment resistant depression among adults in primary care STEP 2: Researchers recruit 480 adults with depression and randomize to: a. Usual antidepressant + mirtazapine (active treatment group/exposed) b. Usual antidepressant + placebo (placebo group/unexposed) STEP 3: Participants are followed-up for 12, 24, and 52 weeks and the severity of depressive symptoms was measured using the Beck Depression Inventory STEP 4: analyse the data – how? On the BDI-II, scores range 0-63, higher scores = more severe symptoms Continuous outcome → linear regression Real Life Example: The MIR Trial Session 4: 4th Oct - pt2 4 Linear Regression to Investigate Mean Differences (with a binary exposure) BDI-II scores (outcome) were measured at 12 weeks of follow-up Active treatment group mean BDI-II score: 18.0 (SD: 12.3) Placebo (TAU) group mean BDI-II score: 19.7 (SD: 12.4) Mean Difference = 18.0 – 19.7 = - 1.7 Interpretation: On average, participants assigned to mirtazapine & sertraline had a BDI-II score 1.7 points lower than participants assigned to sertraline. Adjusted mean difference: -1.83 Linear Regression Summary: Mean differences are one of the effect estimates produced from linear regression, widely used in mental health research (and all health research) Using linear regression, we can construct a CI around the mean difference and generate the corresponding p-value We can add other variables (multiple regression e.g., adjust the association for confounders) Session 4: 4th Oct - pt2 5 We can use exposures that are binary, categorical or continuous and we can use multiple exposures if we wish (more in the stats module!) - though the outcome has to be continuous B. Binary Outcomes Comparing two proportions using probability, risks, and odds. Logistic regression. Probability, risk and odds The frequentist definition: the probability of an event equals the proportion of times that it would (or does) occur in a large number of repeated trials Probability can be expressed as a proportion or percentage E.g. the probability of premature mortality in people with severe mental illness [SMI] would be defined as the proportion of times this would occur among a large number of people with SMI. (e.g.: 30 people in 1,000 = 0.03 = 3%) Can also be called the absolute risk of premature mortality in people with SMI Example: A researcher wishes to investigate the association between adverse childhood experiences [ACEs] and depression among young people Her hypothesis is that experiences ACEs in childhood increases the risk of developing depression during adolescence She designs a longitudinal study to test this hypothesis, following 4000 children from age 8 to 16 ACEs (exposure) measured as a binary variable at age 8 and depression diagnoses (outcome) measured as a binary variable at age 16 STEP 1: She designs a longitudinal study to test this hypothesis, following 4000 children from age 8 to 16 STEP 2: The exposure is children who experienced ACEs by age 8 (exposed) vs those who have not experienced ACEs (unexposed) STEP 3: Depression (outcome) is measured as a binary variable at age 16 denoting whether someone has a diagnosis of depression or not Session 4: 4th Oct - pt2 6 Risks: Risk of depression in those with ACEs = 80/800 = 0.1 = 10% Risk of depression in those without ACEs = 160/3,200 = 0.5 = 5% Anything more than 1 is interpreted as ‘higher’ - less than 1 is ‘lower’ Odds: Odds of outcome in the exposed = a / b Odds of the outcome in the unexposed = c / d Session 4: 4th Oct - pt2 7 Odds of depression in those with ACEs = 80/720 = 0.11 Odds of depression in those without ACEs = 160/3,040 = 0.053 Odds ratio is more likely to be used over risks - particularly with Logistic Regression Summary of risks and odds If the ratio is 1 (or close to 1), it suggests no difference or little difference in risk or odds (1 is therefore the null value) A ratio > 1 suggests an increased risk/odds of that outcome in the exposed compared with the unexposed group A ratio < 1 suggests a reduced risk/odds of outcome in the exposed compared with the unexposed group Risk ranges from 0-1 Odds range from 0-infinity Odds more commonly used in health research Read this article for more details on the difference between risk and odds: Ranganathan P, Aggarwal R, Pramesh CS. Common pitfalls in statistical analysis: Odds versus risk. Perspect Clin Res. 2015 Oct-Dec;6(4):222-4. doi: 10.4103/2229-3485.167092. PMID: 26623395; PMCID: PMC4640017. Logistic Regression Odds ratios are the effect estimate produced from logistic regression, widely used in mental health research (and all of health research) Session 4: 4th Oct - pt2 8 Using logistic regression, we can construct a CI around the OR and generate the corresponding p-value We can adjust the association for multiple confounders We can use exposures that are binary, categorical or continuous, and we can use multiple exposures if we wish C. Count Outcome — Rates Comparing two rates using incidence rate ratios. Poisson regression Used mostly in: Cohort Studies People with and without a given exposure are recruited and followed up over time Or People with a common characteristic (e.g. born in the same month/year) are recruited and followed up Information is collected on a number of characteristics that can be used to measures exposures and outcomes Person year at risk (PYAR) Session 4: 4th Oct - pt2 9 Number of events - e.g. heart (1) PYAR - total of Person-years Real-Life Example: Rates vs Risk The rate relates the number of new events in the total observation time Session 4: 4th Oct - pt2 10 This is in contrast to the risk (cumulative incidence) where the number of new events is related to the number at risk at the start of the observation period The longer the observation time, the greater the risk, and the more events People in the study for longer have more time to develop the outcome To reduce bias we need to include time in the denominator Suissa S. Immortal Time Bias in Pharmacoepidemiology. American Journal of Epidemiology. 2008 Jan 7;167(4):492–499 Incidence rate ratio (IRR) Incidence rate in exposed = number of events/PYAR Incidence rate in unexposed = number of events/PYAR Who has a greater rate of depression? Exposure = female, unexposed (control) = male Rates in females 16-20 = 20.92, rates in males = 14.67 IRR = 1.70 — higher than 1 —> Higher rate of depression in females compared to males Summary: Poisson Regression Models The incidence rate ratio (IRR) is the effect estimate produced from Poisson regression, widely used in mental health research Session 4: 4th Oct - pt2 11 Using Poisson regression, we can construct a CI around the IRR and generate the corresponding p value We can adjust the association for multiple confounders or covariates We can use exposures that are binary, categorical or continuous and we can use multiple exposures if we wish - as long as outcome is a count variable D. Cox Regression Model Hypothesis Testing The null-hypothesis, p-values, and CI Hypothesis Testing: The Null-Hypothesis “How confidently can conclude that there is a difference between the groups?” – how do we do this? We start from the null hypothesis that There is no difference in the outcome between the groups Or There is no association of exposure and outcome We try to find evidence to disprove this Suppose we want to investigate if depression always begins during adolescence. We could prove this hypothesis in two ways: 1. by checking that every person with depression had their first episode during adolescence (prove the hypothesis) 2. by finding just one person who had their first episode of depression as an adult (disprove the hypothesis) Generally, it is easier to find evidence against a hypothesis than it is to prove that the hypothesis is correct. The Null-Hypothesis: Mean Difference What would represent the null hypothesis when we are interpreting risk/odds ratio? - i.e. what is the null value? Session 4: 4th Oct - pt2 12 0 — no difference as both groups have the same mean Odds-Ratios and Risk Ratios What value would represent the null hypothesis when we are interpreting risk/odds ratios? - i.e. what is the null value? 1 — as we’re now dividing What is a P-Value? Frequentist statistics tests the strength of evidence against the null hypothesis The p value is defined as the probability of getting a difference at least as big as that observed, if the null hypothesis were true E.g. before considering that a treatment for depression is effective in a placebo controlled RCT, we need to consider whether it could be a chance finding. → We do this by calculating and interpreting a p-value Interpreting P-values P-values range from 0 to 1 The smaller the p-value, the lower the chance of getting a difference as big as the one observed, if the null hypothesis were true The smaller the p-value → the stronger the evidence against the null hypothesis The larger the p-value, the greater the chance of getting a difference as big as the one observed, if the null hypothesis were true The larger the p-value → the weaker the evidence against the null Common Misinterpretations of P-Values It is bad practice to interpret a p value by examining whether it is smaller or larger than a particular threshold E.g. P-values larger than 0.05 are sometimes regarded as “not significant” but 0.05 is an arbitrary threshold (e.g., p=0.035 and p=0.051 represent a very similar strength of evidence against the null) Session 4: 4th Oct - pt2 13 We recommend that precise p values are always reported (e.g. report p=0.035 rather than p