Internet of Things in Marine Environment Monitoring: A Review PDF
Document Details
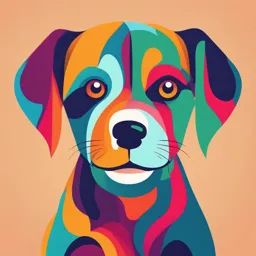
Uploaded by FlawlessMotif7787
Manakula Vinayagar Institute of Technology
Guobao Xu, Yanjun Shi, Xueyan Sun, and Weiming Shen
Tags
Summary
This article reviews the application of the Internet of Things (IoT) in marine environment monitoring. It discusses new technologies like Big Data analytics and their implications for marine protection, along with key challenges and opportunities. The paper covers various application areas, including ocean sensing, water quality monitoring, and others.
Full Transcript
sensors Review Internet of Things in Marine Environment Monitoring: A Review Guobao Xu 1 , Yanjun Shi 2 , Xueyan Sun 3 and Weiming Shen 3, * 1 School of Electronics and Information Engineering, Guangdong Ocean University, Zhanjiang 524088, China; [email protected] 2 School of Mechanical En...
sensors Review Internet of Things in Marine Environment Monitoring: A Review Guobao Xu 1 , Yanjun Shi 2 , Xueyan Sun 3 and Weiming Shen 3, * 1 School of Electronics and Information Engineering, Guangdong Ocean University, Zhanjiang 524088, China; [email protected] 2 School of Mechanical Engineering, Dalian University of Technology, Dalian 116024, China; [email protected] 3 State Key Lab of Digital Manufacturing Equipment and Technology, Huazhong University of Science & Technology, Wuhan 430074, China; [email protected] * Correspondence: [email protected]; Tel.: +86-27-8754-3129 Received: 5 March 2019; Accepted: 6 April 2019; Published: 10 April 2019 Abstract: Marine environment monitoring has attracted more and more attention due to the growing concern about climate change. During the past couple of decades, advanced information and communication technologies have been applied to the development of various marine environment monitoring systems. Among others, the Internet of Things (IoT) has been playing an important role in this area. This paper presents a review of the application of the Internet of Things in the field of marine environment monitoring. New technologies including advanced Big Data analytics and their applications in this area are briefly reviewed. It also discusses key research challenges and opportunities in this area, including the potential application of IoT and Big Data in marine environment protection. Keywords: Internet of Things; Big Data; wireless sensor networks; marine environment monitoring 1. Introduction Although the Internet of Things (IoT) has been defined in various perspectives, there is a common version that is widely accepted by scholars as follows: IoT is a dynamic global network infrastructure with self-configuring capabilities based on standard and interoperable communication protocols, where physical and virtual “things” have identities, physical attributes, and virtual personalities and use intelligent interfaces, and are seamlessly integrated into the information network. Recently, IoT has been widely accepted as a promising paradigm that can transform our society and industry. It can achieve the seamless integration of various devices equipped with sensing, identification, processing, communication, actuation, and networking capabilities. A wireless sensor network (WSN) plays a key role in IoT. It consists of a large number of distributed sensors interconnected through wireless links for physical and environmental monitoring purposes. On the other hand, Big Data is considered as an emerging technology and has become a very active research area, primarily involving topics related to data mining, machine learning, database, and distributed computing. During the past couple of decades, wireless sensor networks (WSNs), as a subset of IoT, have been widely utilized in a variety of smart applications and services, including smart home , smart building [4,5], smart transportation [6,7], smart industrial automation [8,9], smart healthcare , smart grids , and smart cities. Similar IoT-based technologies can certainly be applied to the monitoring and protection of marine environments. With the development of our society and economy, the marine environment has drawn increasing attention from scientists and scholars. Conventional marine environment monitoring systems such as oceanographic and hydrographic research vessels are very expensive. Their data collection and Sensors 2019, 19, 1711; doi:10.3390/s19071711 www.mdpi.com/journal/sensors Sensors 2019, 19, 1711 2 of 21 analysis processes are time-consuming and the collected data have a low resolution. The Internet of Things (IoT) has been evolved from wireless sensor networks (WSNs). Compared with WSNs, IoT has much stronger data processing capabilities, enabling intelligent control of objects. In a typical IoT-based marine environment monitoring system, different sensors are deployed to measure and monitor various physical and chemical parameters like water temperature and pressure, wind direction and speed, salinity, turbidity, pH, oxygen density, and chlorophyll levels. An advanced IoT-based marine environment monitoring and protection system would also be able to control some objects, devices, or equipment within the monitored marine environment, in order to adjust some physical and chemical parameters so as to improve the marine environment. While the design, development, and deployment of an IoT-based marine environment monitoring and protection system is needed to address some critical issues including autonomy, adaptability, scalability, simplicity, and self-healing [13,14], following requirements specific to the harsh marine environments should be considered : (1) High water resistance: Sensor and actuator nodes need to have very high levels of water resistance; (2) Strong robustness in hardware: Hardware or equipment needs stronger robustness due to the aggressive and complex marine environment with currents, waves, tides, typhoons, etc.; (3) Low energy consumption and energy harvesting: Energy conservation and harvesting measures need to be considered due to long communication distances and an environment in constant motion; (4) Stability of Radio signal: Special techniques may be required to ensure the stability of radio signals since the oscillation of the radio antenna can cause an unstable line-of-sight between transmitters and receivers and bad weather conditions can also affect the stability of radio signals; (5) Other issues: Devices and sensor nodes should be highly reliable because of the difficult deployment and maintenance; the need for buoy and mooring devices; sensor coverage needs to be carefully calculated because of large areas ; equipment should be designed against possible acts of vandalism. We conducted a comprehensive review of WSN applications in marine environment monitoring in 2014. When we planned and started this updated review, we wanted to expand the scope from WSN to IoT and to cover the protection aspect in addition to the monitoring because of the characteristics of IoT not only on sensing but also on actuation. However, we did not find enough references related to protection. In this paper, we do not consider the protection aspect as a focus but keep the related discussions. We envision the protection from two perspectives: (1) Results from the advanced data analytics based on the data collected by the monitoring system can be fed back to the marine environment management agencies/control centers for quick decision making and real time manual interventions in order to protect the marine environment from some disasters (e.g., oil spills and bad weather damages); (2) some autonomous vessels or other devices (actuators) can also automatically and quickly react to disasters and other events in order to protect the marine environment. Similarly, we wanted to cover the Big Data analytics and its applications to marine environment monitoring and protection, but we did not find sufficient references. Therefore, we did not make the Big Data a focus of this paper. However, we strongly believe that Big Data analytics will play a more and more important role in this area in the future. The remainder of the paper is organized as follows: Section 2 provides an overview of the fundamentals of IoT-based marine environment monitoring systems; Section 3 presents a summary of some related projects and systems under five application areas; Section 4 reviews a few new technologies applied in the marine environment monitoring systems; Section 5 addresses some research challenges and opportunities in this area; Section 6 provides some concluding remarks. Sensors 2019, 19, 1711 3 of 21 2. Overview of IoT in Marine Environment Monitoring This section provides an overview of IoT in marine environment monitoring, including various applications, common system architectures, typical sensing nodes and sensing parameters, and related wireless communication technologies. 2.1. IoT-Based Marine Environment Monitoring Applications IoT-based marine environment monitoring application areas include: (1) Ocean sensing and monitoring; (2) water quality monitoring; (3) coral reef monitoring; (4) marine (either offshore or deep-sea) fish farm monitoring; (5) wave and current monitoring. Different applications use different IoT system architectures, sensing and control technologies, and communication technologies. An ocean sensing and monitoring system is a general marine environment monitoring system, which existed for a long time, previously using oceanographic and hydrographic research vessels. A water quality monitoring system usually monitors water conditions and qualities, including water temperature, pH, turbidity, conductivity, and dissolved oxygen (DO) for ocean bays, lakes, rivers, and other water bodies. A coral reef monitoring system typically monitors coral reef habitats and the surrounding environments. A marine fish farm monitoring system monitors water conditions and qualities including temperature and pH, measures the amount of fecal waste and uneaten feed for a fish farm, as well as fish conditions and activities including the number of dead fishes. A wave and current monitoring system measures waves and currents for safe and secure waterway navigations. 2.2. Common IoT-Based System Architectures for Marine Environment Monitoring and Protection The Internet of Things is usually to achieve “knowing, thinkable, and controllable” to the surrounding world , which means that the IoT is able to perceive, think, and control the world by collecting, processing, and analyzing the data of the world. It can make intelligent judgments that impact on the outside world. IoT researchers have proposed different IoT system architectures in the research literature. Among them, a five layered system architecture was proposed by Antao et al.. Similarly, we also believe that a typical IoT-based marine environment monitoring and protection system has five layers: Perception and execution layer, transmission layer, data pre-processing layer, application layer, and business layer, as shown in Figure 1. (1) Perception and Execution Layer The perception and execution layer is the bottom layer of the architecture. It includes sensor and actuator devices, with the objective of sensor data collection and command actuation. In IoT-based marine environment monitoring and protection systems, this layer can also include GPS sensors, energy harvesting devices, in addition to regular water condition and quality monitoring sensors. Note that most existing marine environment monitoring systems do not have any execution functions and therefore do not include actuators. (2) Data Transmission Layer The main function of the data transmission layer is to transmit various collected data to the data processing layer via communication networks, mostly mobile or wireless communication networks. At the same time, control measures made by users or intelligent applications (reference engines) are transferred from the application layer to the perception and execution layer, thus that corresponding devices or actuators can take required actions (such as device repositioning, increasing or decreasing temperature settings, releasing food in fish farms). (3) Data Pre-Processing Layer The data pre-processing layer is in the middle of the IoT system architecture, where the raw data received can be stored and pre-processed, using advanced data mining technologies. It also completes Sensors 2019, 19, 1711 4 of 21 information aggregation or disaggregation, data cleaning and fitting or screening, sharing as needed, and sometimes it triggers alerts or warnings based on pre-defined rules. (4) Application Layer The application layer provides services according to different applications requested by users. For example, it provides water condition and quality data as well as the amount of fecal waste and Sensors 2019, 19, x FOR PEER REVIEW 4 of 22 uneaten feed for a fish farm. The main purpose of this layer is to provide smart application services to meet coral users’ reef needs. In monitoring, IoT-based marine marine (either offshoreenvironments, or deep-sea) this later fish farmcovers water quality monitoring, monitoring, wave and current coral reef monitoring, monitoring [21–23]. marine (either offshore or deep-sea) fish farm monitoring, wave and current monitoring [21–23]. (5) Business Layer (5) Business Layer The business layer is the top layer and manages the overall IoT system activities and services, The business including creating layer is the business top layer models, and manages business the overall logic flowcharts, IoT system graphic activities and representations, services, according to including creating business models, business logic flowcharts, graphic representations, according the data received from the application layer. It also monitors and verifies outputs of the other four to the data received layers according from tothe theapplication layer. Itinalso business models monitors order and verifies to enhance outputs services of the other and maintain fourprivacy users’ layers according to the business models in order to enhance services and maintain users’ privacy [21,23]. [21,23]. Business layer Business models Business logic flowcharts Graphic representations Monitoring and verifying outputs Application layer Water quality monitoring Ocean sensing Coral reef monitoring Fish farm monitoring Data pre-processing layer Data mining Machine learning Data cleaning Data fitting Data transmission layer Wireless sensor network LAN Mobile communication network M2M Perception and execution layer Sensors Actuators Figure 1. 1. Common Common layered layered architecture architecture for for Internet Internet of Things (IoT)-based marine environment monitoring and protection applications. Such aa layered Such layeredsystem systemarchitecture architectureprovides providesa good a good picture picture of the of the data/information data/information flowflow in in IoT- IoT-based marine environment monitoring and protection systems. Figure 2 shows a common based marine environment monitoring and protection systems. Figure 2 shows a common physical physical architecture of IoT-based marine environment monitoring and protection systems. It includes sensor nodes and actuator nodes, sink nodes, a base station, a system server, and user terminals. Sensor nodes are used to sense and monitor environmental parameters such as water temperature and pH, salinity, turbidity, oxygen density, and chlorophyll levels, and transmit the collected data to sink nodes via ZigBee or some other wireless communication protocols. Actuator nodes execute Sensors 2019, 19, 1711 5 of 21 architecture of IoT-based marine environment monitoring and protection systems. It includes sensor nodes and actuator nodes, sink nodes, a base station, a system server, and user terminals. Sensor nodes are used to sense and monitor environmental parameters such as water temperature and pH, salinity, turbidity, oxygen density, and chlorophyll levels, and transmit the collected data to sink nodes via ZigBee or some other wireless communication protocols. Actuator nodes execute commands from the upper layers. Communication between a sink node and sensor or actuator nodes is usually point-to-point. A sink node collects data from a group of sensor nodes and sends collected data to the2019, Sensors base19,station or passes x FOR PEER REVIEW execution commands from upper layers to actuator notes, via mobile 5 of 22 communication networks (2G/3G/4G). The base station is connected to a system server through the Internet. Internet. The Thesystem systemserver serverstores storesand and processes processesthe the received received data data from from the the base base station, station, completes completes data data analyses analyses according according totocorresponding corresponding applications, applications, sends sends commands commands to toactuator actuatornodes nodesaccording according to to the the pre-defined pre-defined rules rules as as appropriate. appropriate. Various Various kinds kinds of of user user terminals terminals (desktops, (desktops, laptops, laptops, pads, pads, and and smart smart phones, phones, etc.) etc.) connect connect users users to to the the system system server server over over the the Internet Internet (including (including mobile mobile Internet). Zigbee Zigbee Sensor Actuator node Zigbee Zigbee Internet Sensor Sink Actuator node Zigbee node Zigbee System server Network 1 Actuator Sensor node Sea Wifi Zigbee Zigbee Terminal user 1 Sensor Actuator node Zigbee Zigbee Base station Wifi Sensor Zigbee Sink Zigbee node node Actuator Terminal user n Network 2 Terminal user 2 Actuator Sensor node Common Figure2.2.Common Figure physical physical architecture architecture of IoT-based of IoT-based marine environment marine environment monitoring monitoring and and protection protection systems. systems. The design The design and and development development of of aa durable durable and and scalable scalable IoT IoT system system forfor marine marine environment environment monitoring and protection should carefully consider a number of factors: monitoring and protection should carefully consider a number of factors: The harsh marine The harsh marine environment, the environment, thecommunication communicationprotocols protocolsandandnetwork network topology, topology, thethe number number andand distribution distribution of of nodes, buoys and mooring systems, oceanographic sensors, energy supply and nodes, buoys and mooring systems, oceanographic sensors, energy supply and harvesting options, harvesting options, and so and so on. on. As described As described above, above, an an IoT IoT system system consists consists of of many many sensor sensor and and actuator actuator nodes nodes and and aa gateway gateway for the connection to the Internet. Usually, sensor and actuator nodes are organized for the connection to the Internet. Usually, sensor and actuator nodes are organized into a connectedinto a connected network according network accordingtotoaacertain certaintopology. topology.Physical Physical topology topology andand density density are are entirely entirely dependent dependent on on applications , therefore the design and development of an IoT system applications , therefore the design and development of an IoT system need to consider its need to consider its application and deployment environment. Even though more sensors can application and deployment environment. Even though more sensors can be densely deployed to be densely deployed to enhance data enhance data availability availability and andaccuracy, accuracy, aa dense dense deployment deployment alsoalso brings brings negative negative issues: issues: High Highenergy energy consumption, data collisions, and interferences, etc.. Sensor nodes normally consumption, data collisions, and interferences, etc.. Sensor nodes normally have four typical have four typical kinds of kinds of network network topologies: topologies: Linear, Linear, star, star, cluster/tree, cluster/tree,and andmesh meshtopologies, topologies,asasshown shownininFigure Figure3.3. While we have discussed in detail the star, cluster/tree, and mesh topologies in reference , the linear topology is a series connection of all the nodes. Its main advantage is that it has a simple structure thus that the cost is relatively low. As a consequence, the transmission volume is large, and the speed is slow. Sensors 2019, 19, x FOR PEER REVIEW 6 of 22 Sensors 2019, 19, 1711 6 of 21 linear Star Cluster / Tree Mesh While we have discussed in detail the star, cluster/tree, and mesh topologies in reference , the linear topology is a series connection of all the nodes. Its main advantage is that it has a simple structure thus that the cost is relatively low. As a consequence, the transmission volume is large, and Sensors 2019,is the speed 19,slow. x FOR PEER REVIEW 6 of 22 Sensor node Router node linear Star Cluster / Tree Mesh Sink node Figure 3. Typical wireless sensor network topologies. The selection of a right network topology for a particular application depends on the amount and frequency Sensor of data to be transmitted, the distance of data transmission, the requirement of battery node life as requiredRouter for maintenance, node and the mobility of the sensor nodes. On the other hand, the physical network topology of an IoT system may change during its operation due to its energy Sink node availability, node position variations, equipment or sensor malfunction, node reachability (due to noise, severe weathers, movingFigureobstacles, Figure 3. Typical 3. etc.), and Typical wireless wireless task network sensor sensor details of network sensor nodes. topologies. topologies. The 2.3.AThe selection General Marineof aEnvironment right network topologySensor Monitoring for a particular application depends on the amount and selection of a right network topology for aNode particular application depends on the amount frequency of data to be transmitted, the distance of data transmission, the requirement of battery life and frequency of data to be transmitted, the distance of The general architecture of a marine environment monitoring data transmission, the requirement sensor node is shown inof battery Figure 4. as required for maintenance, and the mobility of the sensor nodes. On the other hand, the physical life as required for maintenance, and the mobility of the sensor nodes. On the other It typically has a buoy device to protect electronic devices against water, and consists of the following hand, the network topology of an IoT system may change during its operation due to its energy availability, physical four mainnetwork modulestopology of an IoT : A sensing system module, may change during a microcontroller, its operation a wireless transceiverduemodule, to its energy and a node position variations, equipment or sensor malfunction, node reachability (due to noise, severe availability, node power supply module. position variations, equipment or sensor malfunction, node reachability (due to weathers, moving obstacles, etc.), and task details of sensor nodes. noise,The severe weathers, sensing moving module obstacles, obtains etc.), and task details the environmental of sensor nodes and equipment.(with associated status amplifiers 2.3. andMarine A General analog-to-digital Environment(A/D) converters). Monitoring The microcontroller receives the data from the Sensor Node 2.3.A sensorGeneral Marine Environment and processes Monitoring the data accordingly. ASensor Node wireless transceiver module includes a radio frequency The general architecture of a marine environment monitoring sensor node is shown in Figure 4. It (RF) The transceiver and an antenna. general architecture A power of a marine supply module environment includes monitoring sensorenergy node storage is showndevices (like in Figure 4. typically has a buoy device to protect electronic devices against water, and consists of the following rechargeable It typically hasbatteries), and ato a buoy device power management protect systemagainst electronic devices with energy water,harvesting and consists devices, of thewhich can following four main modules : A sensing module, a microcontroller, a wireless transceiver module, and a be a main four solar modules panel, a wind : Aenergy sensingharvesting module, adevice, a tidal power microcontroller, generator, a wireless or a seawater transceiver module,power and a power supply module. generator. power Themodule. supply buoy has an anchor to prevent it from moving. The sensing module obtains the environmental and equipment status (with associated amplifiers and analog-to-digital (A/D) converters). The microcontroller receives the data from the sensor and processes the data accordingly. A wireless transceiver module includes a radio frequency (RF) transceiver and an antenna. A power supply module includes energy storage devices (like rechargeable batteries), and a power management system with energy harvesting devices, which can be a solar panel, a wind energy harvesting device, a tidal power generator, or a seawater power generator. The buoy has an anchor to prevent it from moving. Figure 4. General architecture of a marine environment monitoring sensor node. Figure 4. General architecture of a marine environment monitoring sensor node. Sensors 2019, 19, 1711 7 of 21 The sensing module obtains the environmental and equipment status (with associated amplifiers and analog-to-digital (A/D) converters). The microcontroller receives the data from the sensor and processes the data accordingly. A wireless transceiver module includes a radio frequency (RF) transceiver and an antenna. A power supply module includes energy storage devices (like rechargeable batteries), and a power management system with energy harvesting devices, which can be a solar panel, a wind energy harvesting device, a tidal power generator, or a seawater power generator. The buoy has an anchor to prevent it from moving. 2.4. Typical Sensors and Sensing Parameters Sensors are used to respond to changes in their environments by producing electrical signals in the form of electrical voltage, current, or frequency. There are typically two kinds of sensors: Physical sensors and chemical sensors. Physical sensors are used to measure different physical parameters like temperature, humidity, pressure, wind speed, and wind direction. Chemical sensors are used to measure various chemical parameters like salinity, turbidity, pH, nitrate, chlorophyll, and dissolved oxygen (DO). Details can be found in reference. Decisions on the selection of sensors are made according to requirements related to the deployment area and season, measurement range, accuracy, resolution, and power consumption. 2.5. Wireless Communication Technologies A sensor node requires a radio module for wireless communication. The access network, with a communication range from a few hundred meters to several kilometers, includes all the devices between the backbone network and user terminals. For the IoT-based marine environment monitoring and protection systems, wireless communication networks have different requirements than other applications, because of the following reasons: (1) Reliability: Radio antenna oscillations and bad ocean weather conditions can cause instability of radio signals. (2) Energy efficiency: Low power consumption is the key to supporting long-flow and reduced maintenance costs in stand-alone battery-powered equipment. This is particularly critical for devices deployed in remote offshore areas that are difficult and costly to replace. According to the current requirements for IoT applications, the development of wireless communication technologies has already made significant progresses. Various wireless communication standards and technologies have been proposed and developed, including WiFi, ZigBee, Bluetooth, GPRS, GSM, and WiMAX. A summary and brief comparison of these communication standards and technologies can be found be in our previous survey paper. Typically, multiple wireless communication technologies are used in an IoT-based marine environment monitoring and protection system. In some specific applications, underwater acoustic communication technologies are used for data collection and communication among underwater marine environment sensors [30–33]. Generally, a longer-range communication consumes more energy. Selection of the most appropriate wireless communication technology for an application depends on the transmitted data volume, transmission frequency, transmission distance, and available power supply. 3. A Review of Existing Marine Environment Monitoring Projects and Systems Various systems have been developed and deployed for marine environment monitoring during the past couple of decades. Table 1 summarizes the features of reviewed projects and systems under five different application areas as defined in Section 2.1. For completeness and consistency, we kept all the projects and systems summarized in our previous survey and added more from the recent research literature. Sensors 2019, 19, 1711 8 of 21 Table 1. Summary of existing marine environment monitoring projects and systems. Energy Reference Country Sensing Parameters Comm. Protocols Buoy Key Features (Including Testing and Deployment) Harvesting Application Area: Ocean Sensing and Monitoring T, P, salinity, nitrates, velocity, LabVIEW-based user interface using Google Maps; Solar Perez et al. Spain GPRS, ZigBee Special buoy Solar chlorophyll, and turbidity energy harvesting; Special buoy; Deployed in a harbor Simple buoy and Design of an advanced low-cost buoy system; tested in real Voigt et al. Sweden; Germany T, motion, vibration and sound GPRS No king’s buoy environment An autonomous mini-buoy prototype (tested in a pool); Vesecky et al. USA T, wave and location 900 MHz Mobile minibuoy No GPS is used A Perpendicular Intersection (PI) mobile-assisted Liu et al. China T, Sea depth ZigBee Sensor floating No localization scheme; deployed in Hong Kong U of S&T campus and Tsingtao Three-tier communication architecture; transmitting video Macias et al. Spain T, Visible-field, sound ZigBee and acoustic ? ? streaming data; Tested on module of NS-3 T, conductivity, water depth, A multi-layered scalable and adaptive approach of data Roadknight et al. UK ? Single buoy No turbidity management; deployed off Scroby sands Cylinder waterproof Used underwater wireless communication; deployed in the Cella et al. Australia T, illuminance ZigBee Solar buoys Moreton Bay The sleep mechanism and lever buoy; deployed off the Jiang et al. China T, velocity and light ZigBee Lever buoy No seashore Position determination and location verification using GPS Buoys with GPS and Tao et al. China Water T, DO and pH ZigBee ? and PEA (positioning estimation algorithms); tested in two PEA testbeds Seawater luminosity, T and Cylinder waterproof Optimal solar energy harvesting; power-aware and Alippi et al. Italy ZigBee Solar moisture buoys adaptive TDMA protocol; deployed in the Moreton Bay T, P, PAR radiation, pH and Cylinder waterproof A low cost reconfigurable WSN with solar panels; tested in De Marziani et al. Argentina ZigBee Solar salinity buoys San Jorge Gulf A new multisensory buoy system with solar panels; Albaladejo et al. Spain T, P ZigBee Special buoy Solar deployed in Mar Menor Lagoon Marine data acquisition and cartography system based on T, depth, wind speed and MADNET routing VHF; hybrid Mobile Ad-hoc/Delay Tolerant routing Al-Zaidi et al. UK Ship ? direction, humidity, salinity protocol protocol (MADNET); tested in North Sea, and English Channel WiFi, Used autonomous underwater vehicles (AUV), and Ferreira et al. Portugal T, position GPRS/UMTS/LTE, Ship Buoy, ASV No autonomous surface vehicles (ASV); tested in Portuguese Acoustic coast Water T, P, wind speed, wind SentiWordNet is used as an information retrieval tool for Kaur et al. India direction, humidity, cloud GPRS ? processing messages received from nearby marine areas cover, turbidity Sensors 2019, 19, 1711 9 of 21 Table 1. Cont. Energy Reference Country Sensing Parameters Comm. Protocols Buoy Key Features (Including Testing and Deployment) Harvesting Ring Broadcast Mechanism is used to guide searching Hu et al. China T, humility and salinity ? AUV ? direction of sensor nodes; providing self-adaptive dynamic routing mechanism to search the alternative path A framework for spatio-temporal monitoring of Anchors with Mourya et al. UK T, P, salinity, oxygen level Acoustic Solar underwater acoustic sensor networks; anchors are acoustic modems deployed in the ROI inspired by compressive sensing Implementation of the TDA-MAC protocol in practice, and T, P, humidity, optical, distance, Autonomous surface Morozs et al. UK Acoustic No practical issues prompted several crucial modifications to sound, magnetic field, motion vehicle (ASV) the TDA-MAC protocol Underwater positioning algorithm of electing anchor Song et al. China Water T, P, salinity and PH Acoustic Buoy ? nodes and the self-repairing localization algorithm based on anchor nodes failure Application Area: Water Quality Monitoring Various interface circuits; 5 air-based sensor nodes; lab Yang et al. USA pH RF and acoustic PVC housing No testing only Box and polyethylene A LakeNet sensor pod and an altered sampling strategy; Seders et al. USA T, pH, and DO 433 MHz No ring tested a prototype in a small lake T, pH, turbidity, DO and A real-time heterogeneous water quality monitoring; Regan et al. Ireland ZigBee Inshore sensor buoys Solar conductivity deployed in five sites on the River Lee, Ireland A multi-modal environment monitoring network based on O’Connor et al. Ireland T, conductivity and depth ? Buoys ? WSN and visual image; tested in River Lee, Poolbeg Marina and Galway Bay Cylinder waterproof Integrated satellite remote sensing and WSN; deployed in Hadjimitsis et al. Cyprus T, P, salinity and turbidity GPRS No buoy a beach Jin et al. China T, pH, DO, and salinity ZigBee GPRS ? No An early WSN-based water monitoring system ZigBee 802.11 Used ZigBee and 802.11 and a high capacity solar panel; Alkandari et al. Kuwait Water T, DO, and pH ? Solar Ethernet radio tested in a pool T, salinity, conductivity, Two different probe solutions for field covering; tested in Adamo et al. Italy GPRS Self-sufficient buoy ? turbidity and chlorophyll-a Apulia region Application Area: Coral Reefs Monitoring T, P, pH, light, and Bromage et al. USA 900 MHz Watertight housing No Deployed in Monterey Bay conductivity T, ORP, pH, Electrical Remotely Operated Remotely Operated Vehicles with water quality sensors; Berlian et al. Indonesia ? No Conductivity, DO, audio/video Vehicle, buoy Big Data analysis Sensors 2019, 19, 1711 10 of 21 Table 1. Cont. Energy Reference Country Sensing Parameters Comm. Protocols Buoy Key Features (Including Testing and Deployment) Harvesting Application Area: Fish Farm Monitoring A sub-layer-based power consumption algorithm; tested in López et al. Spain T and pH ZigBee ? No a pool Water T, pH value, salinity, DO Multi-hop communication protocol, multiple nodes, and Yang et al. China GPRS ? Solar and COD SMT; tested in an aquatic experimental base T, depth, salinity, position, Fixed on fishing gears, self-powered, autonomous; tested Leblond et al. France GPRS Vessels No catches in Bay of Biscay Lloret et al. Spain Sediment depositions Acoustic Bouy ? Ultrasonic sensor; tested through simulations A multi-level P2MP infrastructure network——OceanNet; Sea surface T, quality of sea Meera et al. India WiFi Fishing vessels No protocol performance comparison of CoAP, AMQP and water, pH, chlorophyll MQTT A group-based underwater WSN for monitoring fecal Lloret et al. Spain Amount of pollution ? Buoy ? waste and uneaten feed; tested on OPNET Modeler network simulator Application Area: Wave and current monitoring ZigBee, Integrated different wave sensors; threshold values Marimon et al. Philippines Acceleration, angle Buoy Solar GSM/GPRS/EDGE generated based on statistics; tested in Manila Bay A temporal evolution model to describe the ocean current Chen et al. China Current velocities ? ? No process based on the temporal correlation of the current velocity. Notes: “T”: Temperature; “P”: Pressure; “DO”: Dissolved Oxygen; “COD”: Chemical Oxygen Demand; “No” under Energy Harvesting: Battery power is used; “?”: Related information is not available from the source. Sensors 2019, 19, 1711 11 of 21 From this long list of systems, we can see that most of the efforts are related to general ocean sensing and monitoring [34–44,46–48] and water quality monitoring [53–60,62]. Some specific efforts have been made for fish farm monitoring [63,65,66,68], coral reef monitoring [61,62], wave and current monitoring , and marine shellfish monitoring. Several projects focus on specific technologies or devices, e.g., buoys [35,36,44,45,47,64], energy saving and harvesting [34,40,43–45,55,59,63,64], routing protocols [46,64], data transmitting approaches [38,46,47], and data analysis. It can also be found that testing places are different among these systems. About half of the developed systems have been tested or deployed in real marine or river environments [34,35, 37,39–41,44–47,55–57,60,61,65,69]; a number of them were experimented in lab settings or indoor environments [38,53,64,68]; some were tested in outdoor pools or small ponds/lakes [36,54,59,63]; and several of them were only tested through simulations [38,66,68]. It is very interesting to note that most projects, systems, and applications have been developed by research groups in a small number of countries, including China [37,41,42,58,64], USA [36,53,54,61], Spain [38,45,63,68], UK , Indonesia , Philippines , India , Portugal , France , and Ireland [55,56]. In these completed systems, most of them occurred on water surfaces [34,35,37,39– 46,48,54–65,68,69] and only a few of them were deployed under water [38,47,53,66]. Please note that underwater wireless sensor network is not the focus of this paper and we will briefly discuss this later in the paper. Even though many researchers discussed different options for energy harvesting including solar, wind, waves, and ocean currents, only solar energy has been used in about a third of these systems and applications. While most systems use GPRS and/or ZigBee for wireless communications, a few systems use underwater acoustic communication [38,47,53,66]. Offshore and deep-sea fish farming has been emerging, which provides more opportunities and also challenges, not only for marine environment monitoring but also for operation controls and marine environment protection. 4. New Technologies for Marine Environment Monitoring and Protection 4.1. Data Analysis Recent fast development and deployment of IoT technologies in marine environment monitoring created huge amounts of data, while the recent advancement of Big Data analytics facilitated the analysis of these marine environment data. As in many other IoT-based data collection systems, dealing with marine environment data also faces some major challenges, particularly the large amount of data and significant bad data. Researchers around the word have been trying to address these challenges. Yang et al. proposed a method to quickly describe the contour of data collected over the Internet of Things (IoT). The distribution of contour lines can be calculated accurately in a short time. Blix and Eltoft proposed an automatic model selection algorithm (AMSA) to determine the best model for a given matchup dataset. It can automatically choose between regression models to estimate the parameter of interest. It also finds out the number and combination of features to be used for obtaining the best model. They used four Machine Learning feature ranking methods and three Machine Learning regression models to estimate oceanic chlorophyll-a in the global and optically complex waters. Zhong et al. developed a fast fuzzy C-means clustering algorithm to analyze water environment monitoring data of the Three Gorges Reservoir Area. The hard cluster center can be treated as the initial value of the fuzzy cluster center to accelerate the speed of convergence and reduce the number of iterations. Addison et al. summarized the challenges in marine environment data management and interpretation caused by the implications of Big Data. They suggested a solution for the management Sensors 2019, 19, 1711 12 of 21 of Big Data, which requires new collaborations between marine practitioners and data scientists with expertise in programming languages and packages like R and Python. Belghith et al. proposed a deep learning-based approach in a marine Big Data setting that enables to classify these diverse acoustic sounds not only considering marine mammals signals. Li et al. presented a support vector regression architecture with smoothness priority for marine sensor data prediction to handle the abruptly fluctuating, multi-noise, non-stationary and abnormal data. The smoother plays the role of preprocessing to handle the outliers and noises in marine sensor data, providing stable initialization values for the next nonlinear approximation based on support vector machines. Since the existing current correlation analysis method for ocean monitoring big data is time consuming and stability is poor, Song et al. developed a new method by sending the collected data to the cloud storage system. Based on the global and local Moran index calculations, the ocean big data of relatively high correlation were saved to the adjacent data center, which reduces the ocean monitoring data correlation analysis time. Radeta et al. developed a low-cost passive acoustic monitoring (PAM) system for nautical citizen science and real-time acoustic augmentation of whale-watching experiences. This paper used machine learning identify vocal acoustic samples of common cetaceans like whales and dolphins with acoustic features (clicks, moans or whistles). It is clear that research and development efforts on the application of Big Data analytics in marine environment monitoring have been growing recently. 4.2. Network Topology Control In wireless sensor networks (WSNs), network topology control capability is a key factor in the performance of the entire network. A reasonable WSN topology control structure can effectively improve the efficiency of network communication protocols and the overall performance of the network. A good network topology helps extend the overall life cycle of the network. Therefore, WSN topology control optimization technology is the key to determining the overall performance of the network, including the network coverage and connectivity. The node selection policy changes the state of the node itself and avoids the communication link redundancy among nodes to form a performance-optimized network structure. Table 2 summarizes the advantages and disadvantages of different topology control algorithms for underwater wireless senor networks (UWSNs). Sensors 2019, 19, 1711 13 of 21 Table 2. Classification of Topology Control Algorithms for underwater wireless senor networks (UWSNs). Category Main Idea Advantages Disadvantages The proper transmission power level is assigned Simple; scalable; May diminish the to each node to conserves energy; does network connectivity; guarantee enough signal not change the sensing Power control based increases the number of strength at the receiver coverage; can overcome hops and end-to-end that it can successfully time-varying acoustic delay. receive and decode the channel quality. transmitted message The wireless interface of nodes alternates between active, sleeping, and Simple; scalable; Changes network Wireless interface mode powered-off modes. This conserves energy relative density; changes routing management based change reduces the channel polling; does not paths from time to time; amount of unnecessary change sensing coverage. increases delay. time a node spends listening to the channel. Some mobile nodes are Improves network Needs trajectory moved to new locations connectivity; deals with planning procedures; in different depths or Mobility assisted based network partitions; increases energy cost for with a predetermined improves data collection mobility; may change trajectory, creating new from hop spots. sensing coverage. interconnections. Even though this classification of topology control algorithms is for underwater wireless sensor networks, it can be well applied to the IoT-based marine environment monitoring in general. Please note that UWSN is not the focus of this paper. For comprehensive reviews of underwater sensor networks and applications, please refer to references [80,81]. It is also interesting to check out the concept of Internet of Underwater Things (IoUT) and its potential applications. The rest of this section provides a review of some examples of interesting approaches proposed and developed in the literature on network topology control. Kim et al. used power control to achieve reliable data delivery based on the sea surface movement that affects the surface signal reflection and the strength of the received signal at a node. Bai et al. proposed an approach to reduce link interference and achieved high throughput by using a correlation matrix to describe the source-destination relationship and conflict relationships among the links. Power control is used to achieve a Signal to Noise Ratio (SNR) larger than the decoding threshold. Su et al. proposed a cycle difference set-based protocol to determine the number and positions of active and sleep intervals in one cycle to guarantee that both the transmitter and receiver are awake for communication. Coutinho et al. developed an optimization model to investigate the performance of the on-the-fly adjustment of the sleep interval in duty-cycled UWSNs to achieve a balanced energy consumption. Khan et al. presented a method to incorporate the AUV resurfacing time in the VoI function and AUV path planning algorithm. Coutinho et al. designed the distributed topology control (DTC) and centralized topology control (CTC) depth adjustment–based topology control algorithms for disconnected and void nodes repositioning to improve network connectivity and data routing. 4.3. New Communication Routing Protocols With the advancement of communication technologies, new communication routing protocols have been proposed and developed for marine environment monitoring systems. Faheem et al. proposed a chromosome (routing path), which consists of sequences of non-negativity integers that denote the IDs of genes (CH nodes) through which a routing path passes. In a routing path, the Sensors 2019, 19, 1711 14 of 21 order of each CH is represented by the locus of chromosome in which the gene of the first locus is always reserved for the source CH nodes. A looping feature of selection (φs), crossover (φc), and mutation (φm) operators is applied on each individual to improve the quality of the solution through the pre-defined probabilities (φp) until the termination criterion is satisfied. The probability of the mutation rate increases from an extremely low value of 0.01 to its maximum value of 0.05. Highly stable small clustering mechanism is used to organize sensor nodes into a connected hierarchy for distributing energy and data traffic load evenly in the network. The protocol proposed by Javaid et al. is called balanced energy adaptive routing (BEAR). It exploits the location information, selects the neighbors, chooses the facilitating and successor nodes based on cost function value, and finally selects the forwarder node that has residual energy more than the average residual energy of the network. BEAR allows nodes to communicate directly with the sink node leading to an increase in the number of packets being dropped. Alageswaran and Swapna proposed an enhanced duty cycled multiple rendezvous multichannel media access control (DMM-MAC) for handling more volume of data in multi-hop underwater wireless sensor networks (UWSNs) for marine eco systems. This protocol distributes bursty sensor data by dynamically tuning the duty cycles, and hence network performance is enhanced dramatically. It is equipped with one modem and the propagation delays or relative distances need not to be known by the other nodes in the network. Less energy is consumed, and it is easy to forward the data based on priority. One cycle consists of several frames and is subdivided into an active section and a sleep section. Each node can use the other time slot when the time slot is free. 5. Major Challenges and Opportunities 5.1. Energy Management Because marine environment monitoring and protection systems work on hash environments as mentioned above, battery replacement is difficult and costly. It is therefore very important to have an efficient energy management system together and preferably with an energy harvesting capability. Typical energy options for sensor nodes include batteries, capacitors, fuel cells, and energy harvesting. A battery is widely used in sensor nodes, and in fact in over half of the systems reviewed under this paper. However, using batteries in sensor nodes has a number of issues including the replacement difficulty, risk of losing power during operation, and environment contamination. It is therefore important to explore alternative power supply options for sensor nodes. Energy harvesting is a natural way to go. In marine environments, energy harvesting options including solar, wind, water waves, and currents. The most outstanding energy harvesting at the moment is photovoltaics (solar energy). Usually, network energy consumption is increasing at a very high rate due to an increase in data rates, increase in the number of Internet-enabled services and rapid growth of Internet connected edge-devices. The drastic increase in IoT devices requires efficient fabrication of batteries because of the uncertainty in battery dissipation. On the other hand, the devices in marine environment monitoring and protection systems are heterogeneous in nature, each with various capabilities and numerous requirements. Hence, the requirement of cost effective and energy efficient routing strategy in terms of space and time arises in future wireless communication networks. 5.2. Standardization With the wider scope of marine environment monitoring and protection, international cooperation projects are emerging one after another. According to the interests of the projects, various application platforms and methods have their own characteristics and cannot be compatible with each other. Even though a number of networking protocols and standards for Internet of Things have been proposed and developed [94,95], they are not sufficient for applications in marine environment monitoring. In addition to the standards of IoT networking, it is also important to provide the industry with standards Sensors 2019, 19, 1711 15 of 21 for IoT devices, equipment, and platforms for marine environment monitoring and protection applications, and providing different levels of governments and marine environment management agencies with standards for marine environment data management, analysis, and reporting. As a result, the standardization of platform development for marine environment monitoring and protection systems brings challenges, including: 1. Standardization of IoT devices specifically for marine environment monitoring and protection, including sensor and actuator nodes, routers and gateways. 2. Standardization of IoT platforms and system technologies for marine environment monitoring and protection, including communication network structures, protocols, and algorithms. 3. Standardization of computing and data storage technologies for marine environment monitoring and protection, including cloud, fog, and edge computing mechanisms, data archiving and warehousing techniques. 4. Standardization of data analysis outputs and reporting formats for exchange among different organizations and governments. 5.3. Marine Environment Protection Currently, the environmental protection issue is one of the most important issues around the world. The ultimate goal of monitoring the ocean is to protect the marine environment. Most of the current marine environment monitoring applications collected and analyzed massive data from the ocean, but there is no action on protection controls yet. With the advancement and sophistication of IoT and Big Data technologies and their wide applications in marine environment monitoring and protection, we are confident that active marine environment protection measures, technologies, and systems will be developed and deployed in the near future. Massive data collected from marine environments will be analyzed using advanced Big Data analytics and the results will be sent to the related marine environment management agencies/control centers for quick decision making and real time manual interventions in order to protect the marine environment from some disasters (e.g., oil spills and bad weather). On the other hand, some autonomous vessels or other devices (actuators) can also automatically and quickly react to disasters and other events in order to protect the marine environment. In the case of a fishing farm application, data collected from the fish farm environment can be analyzed and used to provide the best fish growth condition and the best feeding. 6. Conclusions During the past couple of decades, marine environment monitoring has attracted wide attention. Governments and research organizations have heavily invested in the research and development of new technologies in this area. Advanced information and communication technologies have been applied to the development of various marine environment monitoring technologies and systems. Internet of Things has played an important role in this area. This paper presents an updated review of the related technologies and systems on the application of the Internet of Things in marine environment monitoring. A comprehensive review of about 40 related projects revealed that most systems and applications developed thus far are for ocean sensing and monitoring and water quality monitoring. Some specific efforts have been made for fish farm monitoring, coral reef monitoring, wave and current monitoring. Several projects focused on specific technologies or devices like buoys, energy saving and harvesting devices, routing protocols, data transmitting mechanisms, and data analysis techniques. It can also be noted that testing places are very much different among these projects. About half of the developed systems have been tested or deployed in real marine or river environments; a number of them were experimented in lab settings or indoor environments; a few others were tested in outdoor pools or small ponds and lakes; and several of them were only tested through simulations. Sensors 2019, 19, 1711 16 of 21 While most systems use GPRS and/or ZigBee for wireless communication, a few systems used underwater acoustic communication. Emerging offshore and deep-sea fish farming provides more opportunities and also challenges, not only for marine environment monitoring but also for operation controls and marine environment protection, which is a new area of research. The energy management issue is mainly considered from two aspects: Reducing energy consumption and using alternative renewable energy sources. Optimizing network topologies and developing advanced routing protocols are the ways to reduce energy consumption. Even though many researchers discussed different options for energy harvesting including solar, wind, waves and ocean currents as alternative renewable energy sources, only solar energy has been used in about a third of the developed systems. The results of Big Data analytics can be used not only for feedback to marine environment management agencies and control centers for quick decision making and real time manual interventions but also for autonomous vessels and remotely deployed devices to take real time actions in order to protect the marine environment from some disasters (e.g., oil spills and bad weather). It is a growing area of research and development. This review also identified several research challenges and opportunities, including energy management, standardization of system platforms and technologies, and marine environment protection measures. Author Contributions: All the co-authors have contributed to the conceptualization of the paper and the review of the cited references. As the first author, G.X. prepared the first draft based on his previously published survey paper. X.S. searched and collected the most recent references and provided a first review of these references. Y.S. contributed to the review of new IoT and wireless communication technologies. W.S. defined the paper structure, re-organized the content, and edited the whole paper. Funding: This work was supported in part by the Science Foundation for Enhancing School with Innovation of Guangdong Ocean University (Grant Nos. GDOU2015050207). Conflicts of Interest: No conflict of interests exits in the submission of this manuscript, and the manuscript has been approved by all the co-authors for publication. We would also like to declare that the work described in this paper is original and it has not been published previously, and not under consideration for publication elsewhere, in whole or in part. References 1. Van Kranenburg, R. The Internet of Things: A Critique of Ambient Technology and the All-Seeing Network of Rfid; Institute of Network Cultures: Amsterdam, The Netherlands, 2008. 2. Yang, C.; Shen, W.; Wang, X. Internet of Things in manufacturing: An overview. IEEE SMC Mag. 2018, 4, 6–15. 3. Cook, D.J.; Crandall, A.S.; Thomas, B.L.; Krishnan, N.C. Casas: A smart home in a box. Computer 2013, 46, 62–69. [CrossRef] 4. Finch, E. Is ip everywhere the way ahead for building automation? Facilities 2001, 19, 396–403. [CrossRef] 5. Komninos, N.; Philippou, E.; Pitsillides, A. Survey in smart grid and smart home security: Issues, challenges and countermeasures. IEEE Commun. Surv. Tutor. 2014, 16, 1933–1954. [CrossRef] 6. Talcott, C. Cyber-physical systems and events. In Software-Intensive Systems and New Computing Paradigms; Springer: Berlin/Heidelberg, Germany, 2008; pp. 101–115. 7. Yongfu, L.; Dihua, S.; Weining, L.; Xuebo, Z. A service-oriented architecture for the transportation cyber-physical systems. In Proceedings of the 31st Control Conference (CCC), Hefei, China, 25–27 July 2012; pp. 7674–7678. 8. Ungurean, I.; Gaitan, N.-C.; Gaitan, V.G. An IoT architecture for things from industrial environment. In Proceedings of the 10th International Conference on Communications (COMM), Bucharest, Romania, 29–31 May 2014; pp. 1–4. 9. Wang, C.; Bi, Z.; Da Xu, L. IoT and cloud computing in automation of assembly modeling systems. IEEE Trans. Ind. Inform. 2014, 10, 1426–1434. [CrossRef] Sensors 2019, 19, 1711 17 of 21 10. Catarinucci, L.; De Donno, D.; Mainetti, L.; Palano, L.; Patrono, L.; Stefanizzi, M.L.; Tarricone, L. An IoT-aware architecture for smart healthcare systems. IEEE Internet Things J. 2015, 2, 515–526. [CrossRef] 11. Yan, Y.; Qian, Y.; Sharif, H.; Tipper, D. A survey on smart grid communication infrastructures: Motivations, requirements and challenges. IEEE Commun. Surv. Tutor. 2013, 15, 5–20. [CrossRef] 12. Dameri, R.P.; Rosenthal-Sabroux, C. Smart City: How to Create Public and Economic Value with High Technology in Urban Space; Springer: Cham, Switzerland, 2014. 13. Boonma, P.; Suzuki, J. An adaptive, scalable and self-healing sensor network architecture for autonomous coastal environmental monitoring. In Proceedings of the IEEE Conference on Technologies for Homeland Security, Woburn, MA, USA, 16–17 May 2007; p. 18. 14. Hadim, S.; Mohamed, N. Middleware: Middleware challenges and approaches for wireless sensor networks. IEEE Distrib. Syst. Online 2006, 7, 1. [CrossRef] 15. Albaladejo, C.; Sánchez, P.; Iborra, A.; Soto, F.; López, J.A.; Torres, R. Wireless sensor networks for oceanographic monitoring: A systematic review. Sensors 2010, 10, 6948–6968. [CrossRef] 16. Alippi, C.; Camplani, R.; Galperti, C.; Roveri, M. Effective design of wsns: From the lab to the real world. In Proceedings of the 2008 3rd International Conference on Sensing Technology (ICST), Tainan, Taiwan, 30 November–3 December 2008; pp. 1–9. 17. Cardei, M.; Wu, J. Energy-efficient coverage problems in wireless ad-hoc sensor networks. Comput. Commun. 2006, 29, 413–420. [CrossRef] 18. Xu, G.; Shen, W.; Wang, X. Applications of wireless sensor networks in marine environment monitoring: A survey. Sensors 2014, 14, 16932–16954. [CrossRef] 19. Zhang, X. Application of Internet of Things technology in agricultural production. In Proceedings of the 2018 the International Symposium on Big Data and Artificial Intelligence, Hong Kong, China, 29–30 December 2018; pp. 269–274. 20. Antão, L.; Pinto, R.; Reis, J.; Gonçalves, G. Requirements for testing and validating the industrial Internet of Things. In Proceedings of the 2018 IEEE International Conference on Software Testing, Verification and Validation Workshops (ICSTW), Vasteras, Sweden, 9–13 April 2018; pp. 110–115. 21. Khan, R.; Khan, S.U.; Zaheer, R.; Khan, S. Future Internet: The Internet of Things architecture, possible applications and key challenges. In Proceedings of the 2012 10th International Conference on Frontiers of Information Technology (FIT), Islamabad, India, 17–19 December 2012; pp. 257–260. 22. Yang, Z.; Yue, Y.; Yang, Y.; Peng, Y.; Wang, X.; Liu, W. Study and application on the architecture and key technologies for IoT. In Proceedings of the 2011 International Conference on Multimedia Technology (ICMT), Hangzhou, China, 26–28 July 2011; pp. 747–751. 23. Wu, M.; Lu, T.-J.; Ling, F.-Y.; Sun, J.; Du, H.-Y. Research on the architecture of Internet of Things. In Proceedings of the 2010 3rd International Conference on Advanced Computer Theory and Engineering (ICACTE), Chengdu, China, 20–22 August 2010; pp. V5:484–V485:487. 24. Li, M.; Yang, B. A survey on topology issues in wireless sensor network. In Proceedings of the 2006 International Conference on Wireless Networks (ICWN), Las Vegas, NV, USA, 26–29 June 2006; p. 503. 25. Zeng, Y.; Sreenan, C.J.; Xiong, N.; Yang, L.T.; Park, J.H. Connectivity and coverage maintenance in wireless sensor networks. J. Supercomput. 2010, 52, 23–46. [CrossRef] 26. Flammini, A.; Ferrari, P.; Marioli, D.; Sisinni, E.; Taroni, A. Wired and wireless sensor networks for industrial applications. Microelectron. J. 2009, 40, 1322–1336. [CrossRef] 27. Kim, S.-Y.; Guzide, O.; Cook, S. Towards an optimal network topology in wireless sensor networks: A hybrid approach. In Proceedings of the ISCA First International Conference on Sensor Networks and Applications, San Francisco, CA, USA, 4–6 November 2009. 28. Anastasi, G.; Conti, M.; Di Francesco, M.; Passarella, A. Energy conservation in wireless sensor networks: A survey. Ad Hoc Netw. 2009, 7, 537–568. [CrossRef] 29. Porter, J.; Arzberger, P.; Braun, H.-W.; Bryant, P.; Gage, S.; Hansen, T.; Hanson, P.; Lin, C.-C.; Lin, F.-P.; Kratz, T. Wireless sensor networks for ecology. AIBS Bull. 2005, 55, 561–572. [CrossRef] 30. Jiang, Z. User density in a cluster underwater acoustic network. Int. J. Intell. Control Syst. 2012, 17, 31–40. 31. Jiang, Z. Underwater acoustic networks–issues and solutions. Int. J. Intell. Control Syst. 2008, 13, 152–161. 32. Zhu, Y.; Peng, Z.; Cui, J.-H.; Chen, H. Toward practical mac design for underwater acoustic networks. IEEE Trans. Mobile Comput. 2015, 14, 872–886. [CrossRef] Sensors 2019, 19, 1711 18 of 21 33. Zixuan, Y.; Zhifang, W.; Chang, L. Research on marine environmental monitoring system based on the Internet of Things technology. In Proceedings of the IEEE International Conference on Electronic Information and Communication Technology (ICEICT), Harbin, China, 20–22 August 2016; pp. 121–125. 34. Pérez, C.A.; Jimenez, M.; Soto, F.; Torres, R.; López, J.; Iborra, A. A system for monitoring marine environments based on wireless sensor networks. In Proceedings of the OCEANS 2011 IEEE—Spain, Santander, Spain, 6–9 June 2011; pp. 1–6. 35. Voigt, T.; Osterlind, F.; Finne, N.; Tsiftes, N.; He, Z.; Eriksson, J.; Dunkels, A.; Bamstedt, U.; Schiller, J.; Hjort, K. Sensor networking in aquatic environments-experiences and new challenges. In Proceedings of the 32nd IEEE Conference on Local Computer Networks, Dublin, Ireland, 15–18 October 2007; pp. 793–798. 36. Vesecky, J.F.; Laws, K.; Petersen, S.I.; Bazeghi, C.; Wiberg, D. Prototype autonomous mini-buoy for use in a wireless networked, ocean surface sensor array. In Proceedings of the 2007 IEEE Conference on Geoscience and Remote Sensing Symposium (IGARSS), Barcelona, Spain, 23–27 July 2007; pp. 4987–4990. 37. Liu, K.; Yang, Z.; Li, M.; Guo, Z.; Guo, Y.; Hong, F.; Yang, X.; He, Y.; Feng, Y.; Liu, Y. Oceansense: Monitoring the sea with wireless sensor networks. ACM SIGMOBILE Mobile Comput. Commun. Rev. 2010, 14, 7–9. [CrossRef] 38. Macias, E.; Suarez, A.; Chiti, F.; Sacco, A.; Fantacci, R. A hierarchical communication architecture for oceanic surveillance applications. Sensors 2011, 11, 11343–11356. [CrossRef] 39. Roadknight, C.; Parrott, L.; Boyd, N.; Marshall, I.W. A layered approach to in situ data management on a wireless sensor network. In Proceedings of the 2004 Intelligent Sensors, Sensor Networks and Information Processing Conference, Melbourne, VIC, Australia, 14–17 December 2004; pp. 85–90. 40. Cella, U.M.; Shuley, N.; Johnstone, R. Wireless sensor networks in coastal marine environments: A study case outcome. In Proceedings of the Fourth ACM International Workshop on UnderWater Networks, Berkeley, CA, USA, 3 November 2009; p. 8. 41. Jiang, M.; Guo, Z.; Hong, F.; Ma, Y.; Luo, H. Oceansense: A practical wireless sensor network on the